
“Love is a Healer but who heals love?”
愛の量は無際限ではない。共存共生に至る相互作用の結節点において、私たちが絶えず迫られてきた選択の過程と、その波及効果が蓄積するポテンシャルの総量であり、時に移ろい、時に反転する。

□ Vargas: heuristic-free alignment for assessing linear and graph read aligners
>> https://www.biorxiv.org/content/10.1101/2019.12.20.884676v1.full.pdf
Vargas uses multi-core parallelization and vectorized (SIMD) instructions to make it practical to optimally align large numbers of reads, achieving a maximum speed of 437 billion cell updates per second.
Vargas calculates all possible alignments of read to reference in the course of filling the dynamic programming matrix, and can be used to optimize heuristic alignment accuracy and improve correctness of difficult ChIP-seq reads by 30% over Bowtie 2 most sensitive alignment mode.

□ ICGRM: integrative construction of genomic relationship matrix combining multiple genomic regions for big dataset
>> https://bmcbioinformatics.biomedcentral.com/articles/10.1186/s12859-019-3319-y
ICGRM splits the genome SNPs into several parts and calculate the summary statistics for each part that only needs very few computer RAM; then combines the summary statistics for each part to produce GRM.
ICGRM use Intel Math Kernel Library (Intel MKL) with BLAS routine to perform matrix operation. ICGRM avoids calculating GRM for the whole genome at the same time; thus it makes the construction of the GRM more efficient.
When the number of SNPs is greater than 10 million and number of individual is greater than 10,000, ICGRM solves the problem by splitting the dataset and merging the summary statistics, which reduces the computer memory dramatically.
ICGRM calculates GRM for each segment separately, where users can optionally define the weight of SNP effect and the second command line is to combine each GRM from each segment/loci for generation of the final GRM.


□ NanoCaller for accurate detection of SNPs and small indels from long-read sequencing by deep neural networks:
>> https://www.biorxiv.org/content/10.1101/2019.12.29.890418v1.full.pdf
NanoCaller integrates haplotype structure in deep convolutional neural network for the detection of SNPs/indels from long-read sequencing data, and uses multiple sequence alignment to re-align candidate sites for indels, to improve the performance of variant calling.
NanoCaller uses long-range information to generate predictions for each candidate variant site by considering pileup information of other candidate sites sharing reads. it performs read phasing and carries out local realignment on each set of phased reads to call indels.
NanoCaller uses three convolutional layers with Scaled Exponential Linear Unit (SELU) activation units followed by two different full connection layers for SNP calling. On all genomes, NanoCaller achieves much better performance than Clairvoyante.

□ Smash++: an alignment-free and memory-efficient tool to find genomic rearrangements
>> https://www.biorxiv.org/content/10.1101/2019.12.23.887349v1.full.pdf
Smash++ features improved accuracy, obtained by using multiple finite-context models along with substitution-tolerant Markov models to find fine-grained and coarse-grained chromosomal rearrangements.
Smash++ visualizer allows the visualization of the detected rearrangements along with their self- and relative complexity, by generating an Scalable Vector Graphics.
The Kolmogorov complexity is not computable, hence, an alternative is required to compute it approximately. employ a reference-free compressor to approximate the complexity and, consequently, the redundancy of the found similar regions in the reference and the target sequences.
□ MADOKA: an ultra-fast approach for large-scale protein structure similarity searching
>> https://bmcbioinformatics.biomedcentral.com/articles/10.1186/s12859-019-3235-1
MADOKA performs about 6–100 times faster than existing methods, including TM-align and SAL, in massive alignments.
MADOKA employs a score to select pairs with more similarity to carry out a more accurate fragment-based residue-level alignment.

□ SpecHap: a fast haplotyping method based on spectral graph theory
>> https://www.biorxiv.org/content/biorxiv/early/2019/12/10/870972.full.pdf
SpecHap, a novel approach that adopts spectral graph theory for fast diploid haplotype construction from diverse sequencing protocols.
for 10x linked-reads, barcodes are also examined with its range inferred from the alignment result and hence the linked fragment can cover het-SNVs loci separated by thousands of base pairs; for Hi-C, linkages among het-SNVs apart from millions of base pairs can be extracted.
The unnormalized graph Laplacian is then calculated on the adjacency matrix of the ladder-shape graph, and a cut leading to two haplotype strings guided by the Fielder Vector is performed.
Instead of calculating posterior probability, SpecHap locates conflicting region when the Fielder Vector fails to provide two haplotypes, and cuts the phase block accordingly. SpecHap outputs the VCF file that records phased variants with block identifiers.
Spectral graph theory states that the multiplicity of zero eigenvalues of the graph Laplacian signifies the number of connected components in the graph, and the eigenvector contains the union of spectral signal for all the connected subgraphs.

□ UniPath: A uniform approach for pathway and gene-set based analysis of heterogeneity in single-cell epigenome and transcriptome profiles https://www.biorxiv.org/content/biorxiv/early/2019/12/11/864389.full.pdf
UniPath, for representing single-cells using pathway and gene-set enrichment scores by transformation of their open-chromatin or expression profiles. UniPath also provides consistency and scalability in estimating gene-set enrichment scores for every cell.
UniPath also enables exploiting pathway continuum and dropping known covariate gene-sets for predicting temporal order of single-cells. a novel pseudo-temporal ordering method in UniPath which can use pathway scores and allow dropping gene-sets of known covariates.

□ Explaining the genetic causality for complex diseases via deep association kernel learning
>> https://www.biorxiv.org/content/10.1101/2019.12.17.879866v1.full.pdf
Deep Association Kernel learning (DAK) model to enable automatic causal genotype encoding for GWAS at pathway level. DAK framework incorporates convolutional layers to encode raw SNPs as latent genetic representation.

□ AdaReg: Data Adaptive Robust Estimation in Linear Regression with Application in GTEx Gene Expressions
>> https://www.biorxiv.org/content/biorxiv/early/2019/12/10/869362.full.pdf
constructing a robust likelihood criterion based on weighted densities in the mixture model of Gaussian population distribution mixed with unknown outlier distribution, and developed a data-adaptive γ-selection procedure embedded into the robust estimation.

□ Inferring reaction network structure from single-cell, multiplex data, using toric systems theory
>> https://journals.plos.org/ploscompbiol/article?id=10.1371/journal.pcbi.1007311
The Effective Stoichiometric Spaces (ESS) elucidates network structure from the covariances of single-cell multiplex data, fixed time-point, single-cell data.
eigendecomposition of covariance matrices from sc-data can be interpreted in terms of network stoichiometry and timescales, without model simulation, independent of kinetic parameters, and unhindered by unobserved species.
Simulation of synthetic complex-balanced networks and GRNs suggests ways to tailor reaction network ODEs to better match sc-data. it is possible to predict a partitioning of the eigenspace of Σ without actually simulating the ODE network, under the assumption of toric geometry.
This application of toric theory enables a data-driven mapping of covariance relationships in single-cell measurements into stoichiometric information, one in which each cell subpopulation has its associated Effective Stoichiometric Spaces interpreted in terms of CRN theory.


□ The Euler Characteristic and Topological Phase Transitions in Complex Systems
>> https://www.biorxiv.org/content/biorxiv/early/2019/12/11/871632.full.pdf
theoretically illustrate the emergence of topological phase transitions in three classical network models, namely the Watts-Strogratz model, the Random Geometric Graph, and the Barabasi-Albert model.
Topological phase transitions are characterized by the zeros of the Euler characteristic (EC) or by singularities of the Euler entropy and also indicate signal changes in the mean node curvature of networks and the emergence of a giant k-cycle in a simplicial complex.

□ An Efficient hybrid filter-wrapper metaheuristic-based gene selection method for high dimensional datasets
>> https://www.nature.com/articles/s41598-019-54987-1
a hybrid method based on the IWSSr method and Shuffled Frog Leaping Algorithm (SFLA) is proposed to select effective features in a large-scale gene dataset.
in the filter phase, the Relief method is used for weighting the features. Then, in the wrapper step, by using the SFLA and the IWSSr algorithm, the search is performed to find the best subset of the features.

□ ASTRAL-Pro: quartet-based species tree inference despite paralogy
>> https://www.biorxiv.org/content/10.1101/2019.12.12.874727v1.full.pdf
ASTRAL-Pro is more accurate than alternative methods when gene trees differ from the species tree due to the simultaneous presence of gene duplication, gene loss, incomplete lineage sorting, and estimation errors.
ASTRAL-Pro (ASTRAL for PaRalogs and Orthologs), a new quartet-based species tree inference method. ASTRAL-Pro defines a measure in a principled manner and show how to optimize it using dynamic programming.

□ Overcoming high nanopore basecaller error rates for DNA storage via basecaller-decoder integration and convolutional codes
>> https://www.biorxiv.org/content/10.1101/2019.12.20.871939v1.full.pdf
a novel approach which overcomes the high error rates in basecalled sequences by integrating a Viterbi error correction decoder with the basecaller, enabling the decoder to exploit the soft information available in the deep learning based basecaller pipeline.
To match the sequential Markov nature of the nanopore sequencing and basecalling process, using a convolutional coding scheme as the inner code and achieve 3x lower reading cost than the state-of- the-art works at similar writing cost.
The decoding of the convolutional code using the transition probabilities generated by the basecaller, rather than the final basecalled sequence. The code structure dictates the possible transitions while the probabilities from the basecaller are used for scoring possible paths.
□ HyPo: Super Fast & Accurate Polisher for Long Read Genome Assemblies
>> https://www.biorxiv.org/content/10.1101/2019.12.19.882506v1.full.pdf
HyPo – a Hybrid Polisher– that utilises short as well as long reads within a single run to polish a long reads assembly of small and large genomes.
Hypo generates significantly more accurate polished assembly in about one-third of the time with only about half the memory requirements in comparison to Racon.
HyPo exploits unique genomic kmers to selectively polish segments of contigs using Partial Order Alignment of selective read-segments.

□ FRASER: Detection of aberrant splicing events in RNA-seq data with FRASER
>> https://www.biorxiv.org/content/10.1101/2019.12.18.866830v1.full.pdf
FRASER is based on a count distribution and multiple testing correction, reducing the number of calls by two orders of magnitude over commonly applied z score cutoffs, with a minor sensitivity loss.
The optimal dimension for the latent space was determined by maximizing the area under the precision-recall curve when calling artificially injected aberrant values independently for each splicing metric.
The method was robust to the exact choice of the encoding dimension, as the performance for recalling artificial outliers typically plateaued around the optimal dimension.
□ M3S: a comprehensive model selection for multi-modal single-cell RNA sequencing data
>> https://bmcbioinformatics.biomedcentral.com/articles/10.1186/s12859-019-3243-1
For a gene fitted with multiple peaks in a drop-seq data set, considering the zero expressions as well as those expressions falling into the lowest peak as insignificant expressions, while the rest of the expressions in larger peaks as different levels of true expressions.
Zero Inflated Mixture Gaussian achieved best fitting for 10x genomics data. error of the non-zero expressions are hard to be modeled due to the varied experiment resolutions, ZIMG utilizes a Gaussian distribution to cover the variation of the errors of the lowly expressed genes.
□ The genome polishing tool POLCA makes fast and accurate corrections in genome assemblies
>> https://www.biorxiv.org/content/10.1101/2019.12.17.864991v1.full.pdf
The hybrid strategy can be pursued either by incorporating the short-read data into the early phase of assembly, during the read correction step, or by using short reads to “polish” the consensus built from long reads.
POLCA (POLishing by Calling Alternatives) is more accurate than Pilon, and comparable in accuracy to Racon.

□ Feature selection and dimension reduction for single-cell RNA-Seq based on a multinomial model
>> https://genomebiology.biomedcentral.com/track/pdf/10.1186/s13059-019-1861-6
The multinomial model adequately describes negative control data, and there is no need to model zero inflation.
A simple multinomial methods, including generalized principal component analysis (GLM-PCA) for non-normal distributions, and feature selection using deviance.

□ Representation learning of genomic sequence motifs with convolutional neural networks
>> https://journals.plos.org/ploscompbiol/article?id=10.1371/journal.pcbi.1007560
Deep convolutional neural networks designed to foster hierarchical representation learning of sequence motifs—assembling partial features into whole features in deeper layers—tend to learn distributed representations, i.e. partial motifs.
Max-pooling and convolutional filter size modulates information flow, controlling the extent that deeper layers can build features hierarchically.


□ Distance Indexing and Seed Clustering in Sequence Graphs
>> https://www.biorxiv.org/content/10.1101/2019.12.20.884924v1.full.pdf
a minimum distance algorithm with run time that is linear in the depth of the snarl tree. The distance is also more strictly defined than the previous implementation of distance in vg and is faster than other distance algorithms on queries of arbitrary distance.
The minimum distance algorithm will also work with any sequence graph, whereas the preexisting vg distance algorithm required pre-specified paths.
□ A Metric Space of Ranked Tree Shapes and Ranked Genealogies
>> https://www.biorxiv.org/content/10.1101/2019.12.23.887125v1.full.pdf
The metrics provide the basis for a decision-theoretic statistical inference that can be constructed by finding the best-ranked genealogy that minimizes the expected error or loss function, which, in turn, is a function of the tree distance.
This metric space provides a tool for evaluating convergence and the mixing of Markov chain Monte Carlo procedures on ranked genealogies.
The proposed distances inherit the properties of L1 and L2 distances of symmetric positive definite (SPD) matrices, and define a distance on ranked unlabeled isochronous trees, with all samples obtained at the same point in time.

□ TandemMapper and TandemQUAST: mapping long reads and assessing/improving assembly quality in extra-long tandem repeats
>> https://www.biorxiv.org/content/10.1101/2019.12.23.887158v1.full.pdf
The tandemMapper algorithm is inspired by the minimap2 and Flye mappers. TandemQUAST uses general metrics for evaluating ETRs of any kind and centromeric metrics designed specially to account for HOR structure of centromeric ETR.
TandemQUAST consists of the read mapping module that identifies positions of read alignments to the assembly, polishing module for improving the quality of an assembly based on the identified read alignments, and the quality assessment module.

□ GCNG: Graph convolutional networks for inferring cell-cell interactions https://www.biorxiv.org/content/10.1101/2019.12.23.887133v1.full.pdf
GCNG firstly processed the single cell spatial expression data as one matrix encoding cell locations, and one more matrix encoding gene expression, then feed them into a five-layer graph convolutional neural network to predict gene relationships between cells.
The core structure of GCN is its graph convolutional layer, which enables it to combine graph structure (cell location) and node information (gene expression in specific cell) as inputs to a neural network.
on spatial transcriptomics data, GCNG improves upon prior methods suggested for this task and can propose novel pairs of extracellular interacting genes, and the output of GCNG can also be used for down-stream analysis including functional assignment.

□ Normalization and variance stabilization of single-cell RNA-seq data using regularized negative binomial regression
>> https://genomebiology.biomedcentral.com/articles/10.1186/s13059-019-1874-1
an unconstrained negative binomial model may overfit scRNA-seq data, and overcome this by pooling information across genes with similar abundances to obtain stable parameter estimates.
This procedure omits the need for heuristic steps including pseudocount addition or log-transformation and improves common downstream analytical tasks such as variable gene selection, dimensional reduction, and differential expression.
calculate the Pearson residuals of this model, representing a variance-stabilization transformation that removes the inherent dependence between a gene’s average expression and cell-to-cell variation.
And constructing a generalized linear model (GLM) for each gene with UMI counts as the response and sequencing depth as the explanatory variable.
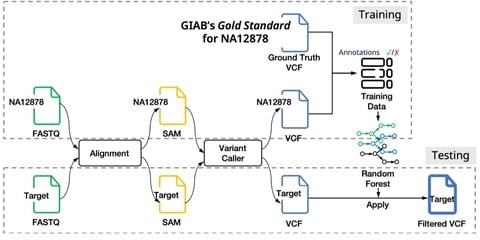
□ VEF: a Variant Filtering tool based on Ensemble methods
>> https://academic.oup.com/bioinformatics/advance-article/doi/10.1093/bioinformatics/btz952/5686383
VEF, a variant filtering tool based on decision tree ensemble methods that overcomes the main drawbacks of VQSR and HF.
VEF is based on ensemble methods that use decision trees as base learners, and trains the model on a variant call set from a sample for which a high- confidence set of “true” variants (i.e., a ground truth of gold standard) is available.
□ SMART: SuperMaximal Approximate Repeats Tool
>> https://academic.oup.com/bioinformatics/advance-article/doi/10.1093/bioinformatics/btz953/5686382
Supermaximal k-mismatch repeats, which are linear in n and capture all maximal k-mismatch repeats: every maximal k-mismatch repeat is a substring of some supermaximal k-mismatch repeat.
SMART employs recent algorithmic advances in approximate string matching under Hamming distance to compute supermaximal k-mismatch repeats without explicitly computing all maximal repeated pairs.
□ A robustness metric for biological data clustering algorithms
>> https://bmcbioinformatics.biomedcentral.com/articles/10.1186/s12859-019-3089-6
if ground truth is largely unknown, or if hierarchical structure is implicit in the data under study, then hierarchical clustering can serve at least as a good starting candidate given its excellent robustness, relative simplicity and intuitive appeal.
For more complex clustering tasks, however, endorse instead a graph-theoretical method such as paraclique due its solid overall robustness and its much improved potential for biological fidelity.

□ The transcriptome dynamics of single cells during the cell cycle
>> https://www.biorxiv.org/content/10.1101/2019.12.23.887570v1.full.pdf
The linearity of this algorithm is in contrast to non-linear analysis and visualization methods (k-nearest-neighbors, UMAP, tSNE), which can be used to flatten more complex manifolds onto a two-dimensional space.
It is generally accepted that single-cell transcriptomic profiles characterize an expression manifold embedded in the expression space of all genes.
if cells have evolved “optimality principles” to traverse the cell cycle, it is tempting to speculate that similar optimality principles of gene expression trajectories may have evolved for a large variety of biological systems.

□ VariantStore: A Large-Scale Genomic Variant Search Index
>> https://www.biorxiv.org/content/10.1101/2019.12.24.888297v1.full.pdf
the scalability of VariantStore by indexing genomic variants from the TCGA- BRCA project containing 8640 samples and 5M variants in ≈ 4 Hrs and the 1000 genomes project containing 2500 samples and 924M variants in ≈ 3 Hrs.
VariantStore outperformed VG toolkit by 3× in terms of memory-usage and construction time and uses 25% less disk space although VG toolkit does not support variant queries. In future, VariantStore will support dynamic updates following the LSM-tree design.
□ Efficient computation of stochastic cell-size transient dynamics
>> https://bmcbioinformatics.biomedcentral.com/articles/10.1186/s12859-019-3213-7
a continuous time Markov chain (CTMC) which transient dynamics can be estimated numerically using the finite state projection (FSP) approach.
Finite state projection maps the infinite set of the states n∈N of a Markov chain onto a set with a finite number of states. The transient probability distribution of such finite state Markov chain can approximated by using standard numerical ODE solvers.
Continuous rate models consider, besides discrete division events, the cell cycle dynamics. This class of models describes the division as a continous-time stochastic process w/ an associated division rate that sets the probability of division into an infinitesimal time interval.

□ Sanity: Bayesian inference of the gene expression states of single cells from scRNA-seq data
>> https://www.biorxiv.org/content/10.1101/2019.12.28.889956v1.full.pdf
the prior distribution over LTQs for a gene is the least assuming, i.e. maximum entropy, distribution that is consistent with only a given mean and variance.
Indeed, even though methods such as SAVER, DCA, MAGIC, and scVI specifically normalize for the total UMI count per cell, their normalized expression levels show strong correlations (and anti-correlations) with total UMI count.
Sanity (SAmpling Noise corrected Inference of Transcription activitY) is deterministic, has zero tunable parameters, and provides error-bars for all its estimates.

□ A novel algorithm for alignment of multiple PPI networks based on simulated annealing
>> https://bmcgenomics.biomedcentral.com/articles/10.1186/s12864-019-6302-0
an efficient algorithm based on graph feature vectors to globally align multiple PPI networks. A target scoring function was used to evaluate the quality of network alignment, which integrates both topology and sequence information.
□ Empirical decomposition of the explained variation in the variance components form of the mixed model
>> https://www.biorxiv.org/content/10.1101/2019.12.28.890061v1.full.pdf
a novel coefficient of determination which is dimensionless, has an intuitive and simple definition in terms of variance explained, is additive for several random effects and reduces to the adjusted coefficient of determination in the linear model.
※コメント投稿者のブログIDはブログ作成者のみに通知されます