

□ STT: Spatial transition tensor of single cells
>> https://www.nature.com/articles/s41592-024-02266-x
STT, a spatial transition tensor approach to reconstruct cell attractors in spatial transcriptome data using unspliced and spliced mRNA counts, to allow quantification of transition paths between spatial attractors as well as analysis of individual transitional cells.
STT assumes the coexistence of multiple attractors in the joint unspliced (U)–spliced (S) counts space. A 4-dimensional transition tensor across cells, genes, splicing states and attractors is constructed, with attractor-specific quantities associated with each attractor basin.
By iteratively refining the tensor estimation and decomposing the tensor-induced and spatial-constrained cellular random walk, STT connects the scales between local gene expression and splicing dynamics as well as the global state transitions among attractors.


□ D3 - DNA Discrete Diffusion: Designing DNA With Tunable Regulatory Activity Using Discrete Diffusion
>> https://www.biorxiv.org/content/10.1101/2024.05.23.595630v1
DNA Discrete Diffusion (D3), a generative framework for conditionally sampling regulatory sequences with targeted functional activity levels. D3 can accept a conditioning signal, a scalar or vector, alongside the data as input to the score network.
D3 generates DNA sequences that better capture the diversity of cis-regulatory grammar. D3 employs a similar method with a different function for Bregman divergence.

□ PHOENIX: Biologically informed NeuralODEs for genome-wide regulatory dynamics
>> https://genomebiology.biomedcentral.com/articles/10.1186/s13059-024-03264-0
PHOENIX (Prior-informed Hill-like ODEs to Enhance Neuralnet Integrals with eXplainability), an innovative NeuralODE architecture that inherits the universal function approximation property (and thus the flexibility) of neural networks while resembling Hill-Langmuir kinetics.
PHOENIX operates on the original gene expression space and performs without any dimensional reduction. PHOENIX plausibly predicted continued periodic oscillations in gene expression, even though the training data consisted of only two full cell cycles.
PHOENIX incorporates two levels of back-propagation to parameterize the neural network while inducing domain knowledge-specific properties. PHOENIX estimates the local derivative, and an ODE solver integrates this value to predict expression at subsequent time points.

□ Spatial Coherence of DNA Barcode Networks
>> https://www.biorxiv.org/content/10.1101/2024.05.12.593725v1
"Spatial Coherence" follows Euclidean geometric laws. Spatial coherence is a feature of well-behaved spatial networks, and is reduced by introducing random, non-spatially-correlated edges b/n nodes in the network and is impacted by sparse or incomplete sampling of the network.
Spatial coherence is a measurable, ground-truth agnostic property that can be used to assess how well spatial information is captured in sequencing-based microscopy networks, and could aid in benchmark comparison, or provide a metric of confidence in reconstructed images.


□ LiftOn: Combining DNA and protein alignments to improve genome annotation
>> https://www.biorxiv.org/content/10.1101/2024.05.16.593026v1
LiftOn implements a two-step protein-maximization algorithm to find the best annotations at protein-coding gene loci. LiftOn uses a chaining algorithm, to find the exon-intron boundaries of protein coding transcripts.
LiftOn combines both DNA and protein sequence alignment to generate protein-coding gene annotations that maximize similarity to the reference proteins. LiftOn resolves issues such as overlapping gene loci and multi-mapping for genes.

□ HERRO: Telomere-to-telomere phased genome assembly using error-corrected Simplex nanopore reads
>> https://www.biorxiv.org/content/10.1101/2024.05.18.594796v1
HERRO, a framework based on a deep learning model capable of correcting Simplex nanopore regular and ultra-long reads. Combining HERRO with Hifiasm and Verkko for diploid and La Jolla Assembler, It achieves phased genomes with many chromosomes reconstructed T2T.
HERRO is optimised for both R9.4.1. and R10.4.1 pores and chemistry. HERRO achieves up to 100-fold improvement in read accuracy while keeping intact the most important sites, including haploid-specific variation and variations between segments in tandem duplications.

□ TRAPT: A multi-stage fused deep learning framework for transcriptional regulators prediction via integrating large-scale epigenomic data
>> https://www.biorxiv.org/content/10.1101/2024.05.17.594242v1
By leveraging two-stage self-knowledge distillation to extract the activity embedding of regulatory elements, TRAPT (Transcription Regulator Activity Prediction Tool) can predicts key regulatory factors for sets of query genes through a fusion strategy.
TRAPT calculates the epigenomic regulatory potential (Epi-RP) and the transcriptional regulator regulatory potential. It then predicts the downstream regulatory element activity of each TR and the context-specific upstream regulatory element activity of the queried gene set.

□ Gene2role: a role-based gene embedding method for comparative analysis of signed gene regulatory networks
>> https://www.biorxiv.org/content/10.1101/2024.05.18.594807v1
Gene2role, a gene embedding method for signed GRNs, employing the frameworks from SignedS2V and struc2vec. Gene2role leverages multi-hop topological information from genes within signed GRNs.
Gene2role efficiently captures the intricate topological nuances of genes using GRNs inferred from four distinct data sources. Then, applying Gene2role to integrated GRNs allowed us to identify genes with significant topological changes across cell types or states.

□ scDecorr: Feature decorrelation representation learning with domain adaptation enables self-supervised alignment of multiple single-cell experiments
>> https://www.biorxiv.org/content/10.1101/2024.05.17.594763v1
scDecorr takes as input single-cell gene-expression matrix coming from different studies (Domains) and uses a self-supervised feature decorrelation approach using a siamese twin model to obtain an optimal data representation.
scDecorr learns cell representations in a self-supervised fashion via a joint embedding of distorted gene profiles of a cell. It accomplishes this by optimizing an objective function that maximizes similarity among the distorted embeddings while also decorrelating their components.
scDecorr learns batch-invariant representations using the domain adaptation (DA) framework. It is responsible for projecting samples from multiple domains to a common manifold such that similar cell samples from all the domains lie close to each other.

□ DeepDive: estimating global biodiversity patterns through time using deep learning
>> https://www.nature.com/articles/s41467-024-48434-7
DeepDive (Deep learning Diversity Estimation), a framework to estimate biodiversity trajectories consisting of two main modules: 1) a simulation module that generates synthetic biodiversity and fossil datasets and 2) a deep learning framework that uses fossil data.
The simulator generates realistic diversity trajectories, encompassing a broad spectrum of regional heterogeneities. Simulated data also include fossil occurrences and their distribution across discrete geographic regions and through time.

□ CellWalker2: multi-omic discovery of hierarchical cell type relationships and their associations with genomic annotations
>> https://www.biorxiv.org/content/10.1101/2024.05.17.594770v1
CellWalker2 is a graph diffusion-based method for single-cell genomics data integration. It takes count matrices as inputs specifically gene-by-cell and/or peak-by-cell matrices from scRNA-Seq and scATAC-Seq respectively.
CellWalker2 builds a graph that integrates these inputs, plus a cell type ontology and optionally genome coordinates for regions of interest. The algorithm then conducts a random walk with restarts on this graph and computes an influence matrix.
From sub-blocks of the influence matrix, CellWalker2 learns relationships between different nodes. CellWalker2 can map genomic regions to cell ontologies, enabling precise annotation of elements derived from bulk data, such as enhancers, genetic variants, and sequence motifs.

□ bulk2sc: Generating Synthetic Single Cell Data from Bulk RNA-seq Using a Pretrained Variational Autoencoder
>> https://www.biorxiv.org/content/10.1101/2024.05.18.594837v1
bulk2sc, a bulk to single cell framework which utilizes a Gaussian mixture variational autoencoder (GMVAE) to generate representative, synthetic single cell data from bulk RNA-seq data by learning the cell type-specific means, variances, and proportions.
bulk2sc is composed of three parts: a single cell GMVAE (scGMVAE) that learns cell type specific Gaussian parameters, a bulk RNA-seq VAE (Bulk VAE) that learns the cell type specific means, variances and proportion (passed from the scGMVAE) using bulk RNA-seq data as input.
bulk2sc reconstructs the scRNA data using a bulk-to-single-cell encoder-decoder (genVAE) composed of the encoder-decoder components from Bulk VAE, which generates synthetic, representative scRNA-seq from bulk RNA-seq data.

□ StarFunc: fusing template-based and deep learning approaches for accurate protein function prediction
>> https://www.biorxiv.org/content/10.1101/2024.05.15.594113v1
StarFunc, a composite approach that integrates state-of-the-art deep learning models seamlessly with template information from sequence homology, protein-protein interaction partners, proteins with similar structures, and protein domain families.
StarFunc’s structure-based component adds a fast Foldseek-based structure prefiltering stage to select the subset of related templates for full length TM-align alignment, providing both the efficiency of Foldseek and the sensitivity of TM-align for structural template detection.

□ CellAgent: An LLM-driven Multi-Agent Framework for Automated Single-cell Data Analysis
>> https://www.biorxiv.org/content/10.1101/2024.05.13.593861v1
CellAgent, a zero-code LLM-driven multi-agent collaborative framework for scRNA-seq data analysis. CellAgent can directly comprehend natural language task descriptions, completing complex tasks with high quality through effective collabo-ration, autonomously.
CellAgent introduces a hierarchical decision-making mechanism, with upper-level task planning via Planner, and lower-level task execution via Executor.
CellAgent uses a self-iterative optimization mechanism, encouraging Executors to autonomously optimize the planning process by incorporating automated evaluation results and accounting for potential code execution exceptions.


□ ESM All-Atom: Multi-scale Protein Language Model for Unified Molecular Modeling
>> https://www.biorxiv.org/content/10.1101/2024.03.04.583284v2.full.pdf
ESM-AA (ESM All-Atom), which achieves multi-scale unified molecular modeling through pre-training on multi-scale code-switch protein sequences and describing relationships among residues and atoms using a multi-scale position encoding.
ESM-AA generates multi-scale code-switch protein sequences by randomly unzipping partial residues. ESM-AA uses 12 stacked Transformer layers, each with 20 attention heads. The model dimension and feed-forward dimension of each Transformer layer are 480 and 1920.

□ COCOA: A Framework for Fine-scale Mapping Cell-type-specific Chromatin Compartmentalization Using Epigenomic Information
>> https://www.biorxiv.org/content/10.1101/2024.05.11.593669v1
COCOA (mapping chromatin compartmentalization with epigenomic information), a method that predict the cell-type-specific correlation matrix (CM) using six types of accessible epigenomic modification signals.
COCOA employs the cross attention fusion module to fuse bi-directional epigenomic track features. The cross attention fusion module mainly contains two attention feature fusion layers. Each AFF layer has: global feature extraction, local feature extraction and attention fusion.
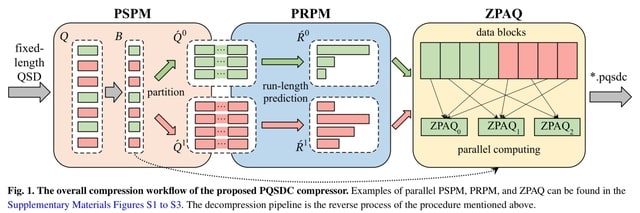
□ CLEAN-Contact: Contrastive Learning-enabled Enzyme Functional Annotation Prediction with Structural Inference
>> https://www.biorxiv.org/content/10.1101/2024.05.14.594148v1
CLEAN-Contact framework harnesses the power of ESM-2, a pretrained protein language model responsible for encoding amino acid sequences, and ResNet, a convolutional neural network utilized for encoding contact maps.
Sequence and structure representations are combined and projected into high-dimensional vectors using the projector. Positive samples are those with the same EC number as the anchor sample and negative samples are chosen from EC numbers with cluster centers close to the anchor.

□ CellSNAP: Cross-domain information fusion for enhanced cell population delineation in single-cell spatial-omics data
>> https://www.biorxiv.org/content/10.1101/2024.05.12.593710v1
CellSNAP (Cell Spatio- and Neighborhood-informed Annotation and Patterning), an unsupervised information fusion algorithm, broadly applicable to different single-cell spatial-omics data modalities, for learning cross-domain integrative single-cell representation vectors.
CellSNAP uses SNAP-GNN-duo, they train a pair of graph neural networks with an overarching multi-layer perceptron (MLP) head to predict each cell's neighborhood-composition-plus-cell-cluster vectors, using both its feature expressions and its local tissue image encoding.

□ MetaGraph: Indexing All Life's Known Biological Sequences
>> https://www.biorxiv.org/content/10.1101/2020.10.01.322164v3
MetaGraph can index biological sequences of all kinds, such as raw DNA/RNA sequencing reads, assembled genomes, and protein sequences. The MetaGraph index consists of an annotated sequence graph that has two main components:
The first is a k-mer dictionary representing a De Bruijn graph. The k-mers stored in this dictionary serve as elementary tokens in all operations on the MetaGraph index. The second is a representation of the metadata encoded as a relation b/n k-mers and any categorical features.
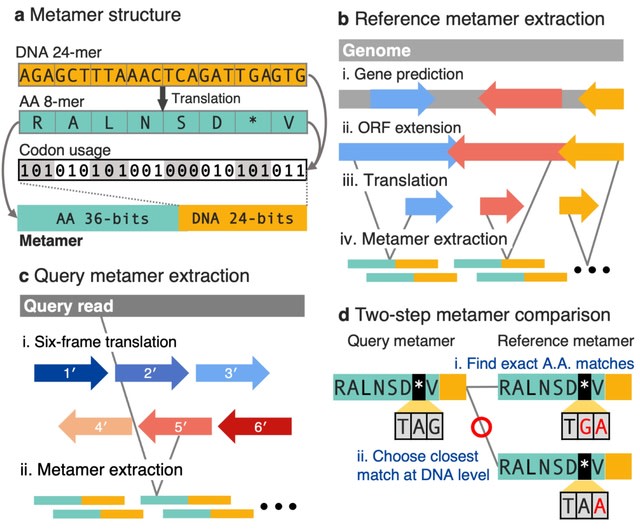
□ Metabuli: sensitive and specific metagenomic classification via joint analysis of amino acid and DNA
>> https://www.nature.com/articles/s41592-024-02273-y
Metabuli is metagenomic classifier that jointly analyze both DNA and amino acid (AA) sequences. DNA-based classifiers can make specific classifications, exploiting point mutations to distinguish close taxa.

□ IFDlong: an isoform and fusion detector for accurate annotation and quantification of long-read RNA-seq data
>> https://www.biorxiv.org/content/10.1101/2024.05.11.593690v1
IFDlong, an Isoform Fusion Detector that was tailored for long-RNA-seq data for the annotation and quantification of isoform and fusion transcripts.
IFDlong employs multiple selection criteria to control FP in the detection of novel isoforms and fusion transcripts. IFDlong enhances the accuracy of fusion detection by filtering out fusion candidates involving pseudogenes, genes from the same family, and readthrough events.

□ Parallel maximal common subgraphs with labels for molecular biology
>> https://www.biorxiv.org/content/10.1101/2024.05.10.593525v1
The parallel algorithms to compute the Maximal Common Connected Partial Subgraphs (MCCPS) over shared memory, distributed memory, and a hybrid approach.
A novel memory-efficient distributed algorithm that allows to exhaustively enumerate all Maximal Common Connected Partial Subgraphs when considering backbones, canonical and noncanonical contacts, as stackings
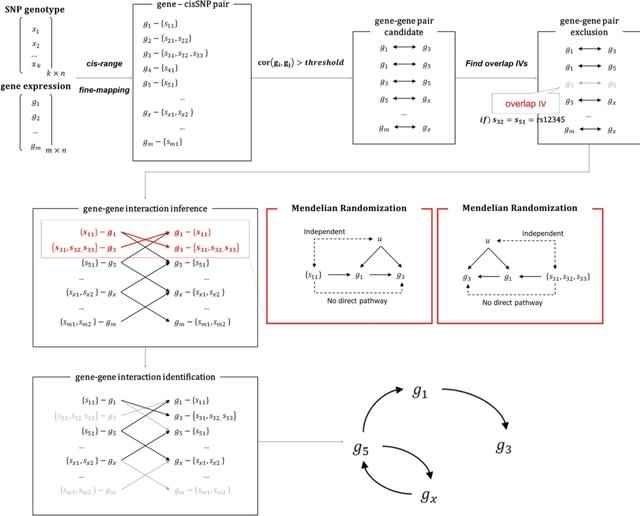
□ MR-GGI: accurate inference of gene–gene interactions using Mendelian randomization
>> https://bmcbioinformatics.biomedcentral.com/articles/10.1186/s12859-024-05808-4
MR-GGI requires gene expression and the genotype of the data. MR-GGI identifies gene–gene interaction by inferring causality between two genes, where one gene is used as an exposure, the other gene is used as an outcome, and causal cis-SNP(s) for the genes are used as IV(s).

□ Readsynth: short-read simulation for consideration of composition-biases in reduced metagenome sequencing approaches
>> https://bmcbioinformatics.biomedcentral.com/articles/10.1186/s12859-024-05809-3
Readsynth first reads each input genome assembly individually to capture the set of possible fragments and calculate the probability of each sequence fragment surviving to the final library.
Fragments resulting from any combination of palindromic restriction enzyme motifs are modeled probabilistically to account for partial enzyme digestion.
The probability of a fragment remaining at the end of digestion is calculated based on the probability of an enzyme cut producing the necessary forward and reverse adapter-boundary sites, adjusted accordingly for fragments harboring internal cut sites.
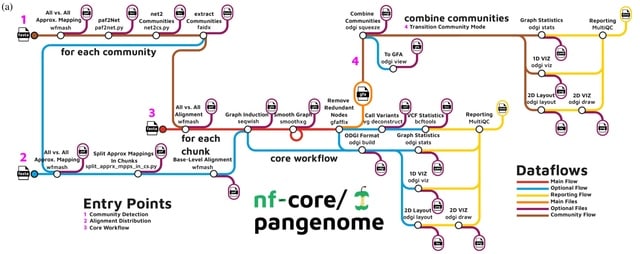
□ Cluster efficient pangenome graph construction with nf-core/pangenome
>> https://www.biorxiv.org/content/10.1101/2024.05.13.593871v1
nf-core/pangenome, an easy-to-install, portable, and cluster-scalable pipeline for the unbiased construction of pangenome variation graphs. It is the first pangenomic nf-core pipeline enabling the comparative analysis of gigabase-scale pangenome datasets.
nf-core/pangenome can distribute the quadratic all-to-all base-level alignments across nodes of a cluster by splitting the approximate alignments into problems of equal size using the whole-chromosome pairwise sequence aligner WMASH.

□ SANGO: Deciphering cell types by integrating scATAC-seq data with genome sequences
>> https://www.nature.com/articles/s43588-024-00622-7
SANGO, a method for accurate single-cell annotation by integrating genome sequences around the accessibility peaks. The genome sequences of peaks are encoded into low-dimensional embeddings, and iteratively reconstruct the peak statistics through a fully connected network.
SANGO was demonstrated to consistently outperform competing methods on 55 paired scATAC-seq datasets across samples, platforms and tissues. SANGO was also shown to be able to detect unknown tumor cells through attention edge weights learned by the graph transformer.

□ Flawed machine-learning confounds coding sequence annotation
>> https://www.biorxiv.org/content/10.1101/2024.05.16.594598v1
An assessment of nucleotide sequence and alignment-based de novo protein-coding detection tools. The controls we use exclude any previous training dataset and include coding exons as a positive set and length-matched intergenic and shuffled sequences as negative sets.
<r />

□ Telogator2: Characterization of telomere variant repeats using long reads enables allele-specific telomere length estimation
>> https://bmcbioinformatics.biomedcentral.com/articles/10.1186/s12859-024-05807-5
Telogator2, a method for reporting ATL and TVR sequences from long read sequencing data. Telogator2 can identify distinct telomere alleles in the presence of sequencing errors and alignments where reads may be mapped to chromosome arms different from where they originated.
Telogator2 extracts a subset of reads containing a minimum number of canonical repeats. Telomere region boundaries are estimated based on the density of telomere repeats, and reads that terminate in telomere sequence on one end and non-telomere sequence on the other are selected.
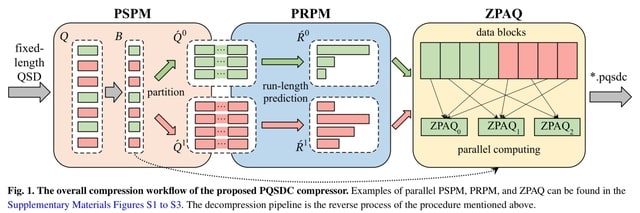
□ PQSDC: a parallel lossless compressor for quality scores data via sequences partition and Run-Length prediction mapping
>> https://academic.oup.com/bioinformatics/advance-article/doi/10.1093/bioinformatics/btae323/7676123
PQSDC (Parallel QSD Compressor), a novel parallel lossless QSD-dedicated compression algorithm. PQSDC is robust when compress QSD w/ varying data distributions. This is attributed to the proposed PRPM model, which integrates the strengths of mapping and dynamic run-length coding.

□ mosGraphGen: a novel tool to generate multi-omic signaling graphs to facilitate integrative and interpretable graph AI model development
>> https://www.biorxiv.org/content/10.1101/2024.05.15.594360v1
mosGraphGen (multi-omics signaling graph generator), a novel computational tool that generates multi-omics signaling graphs of individual samples by mapping the multi-omics data onto a biologically meaningful multi-level background signaling network.

□ iSeq: An integrated tool to fetch public sequencing data
>> https://www.biorxiv.org/content/10.1101/2024.05.16.594538v1
iSeq automatically detects the accession format and fetches metadata from the appropriate source, prioritizing ENA among the partner organizations of INSDC or GSA due to their extensive data availability.
iSeq can merge multiple FASTQ files from the same experiment into a single file for single-end (SE) sequencing data, or maintain the order and consistency of read names in two files for paired-end (PE) sequencing data.

□ SCIITensor: A tensor decomposition based algorithm to construct actionable TME modules with spatially resolved intercellular communications
>> https://www.biorxiv.org/content/10.1101/2024.05.21.595103v1
SCIlTensor, a framework that decomposes the patterns of ME units and the spatial interaction modules based on NTD, an unsupervised method that can identify spatial patterns and modules from multidimensional matrices.
SCIlTensor constructs a three-dimensional matrix by stacking intensity matrices of interactions in each TME unit, and it is decomposed by NTD. The decomposed patterns in each dimension indicate events related to specific cellular and molecular function modules within TME modules.

□ SpatialDiffusion: Predicting Spatial Transcriptomics with Denoising Diffusion Probabilistic Models
>> https://www.biorxiv.org/content/10.1101/2024.05.21.595094v1
stDiffusion adapts Denoising Diffusion Probabilistic Models principles. stDiffusion learns ST data from a single slice and predict heldout slices, effectively interpolating b/n a finite set of ST slices.
stDiffusion incorporates an embedding layer for cell types and a linear transformation for spatial coordinates. An embedding layer for cell type classification allows the model to interpret cell types as dense vectors of a specified dimension.

□ BioInformatics Agent (BIA): Unleashing the Power of Large Language Models to Reshape Bioinformatics Workflow
>> https://www.biorxiv.org/content/10.1101/2024.05.22.595240v1
BIA is operationalized via textual interactions with Large Language Models (LLMs). Overall, the engagement with the LLM is orchestrated via four structured narrative segments: the Thought segment instigates a reflective assessment of the task's progression;
the Action and Action Input segments direct the LLM to invoke a particular tool and specify its required inputs, thereby promoting instrumental engagement; finally, the Observation phase permits the LLM to interpret the result from the executed tool.
※コメント投稿者のブログIDはブログ作成者のみに通知されます