
Und in den Nächten fällt die schwere Erde
aus allen Sternen in die Einsamkeit.
- Rainer Maria Rilke.
そして夜々には 重たい地球が
あらゆる星の群から 寂寥のなかへ落ちる。

□ ENIGMA: Improved estimation of cell type-specific gene expression through deconvolution of bulk tissues with matrix completion
>> https://www.biorxiv.org/content/10.1101/2021.06.30.450493v1.full.pdf
ENIGMA (a dEcoNvolutIon method based on reGularized Matrix completion) requires cell type reference expression matrix (signature matrix), which could be derived from either FACS RNA-seq / scRNA-seq through calculating the average expression value of each gene from each cell type.
ENIGMA applied robust linear regression model to estimate each cell type fractions among samples based on reference matrix derived from the first step. Third, based on reference matrix and cell type fraction matrix.
ENIGMA applied constrained matrix completion algorithm to deconvolute bulk RNA-seq matrix into CSE. ENIGMA inferred CSE, almost all cell types showed improved cell type fractions estimation, as reflected by increased Pearson correlation with the ground truth cell type fractions.
ENIGMA could reconstruct the pseudo-trajectory of CSE. the returned CSE could be used to identify cell type-specific DEG, visualize each gene’s expression pattern on the cell type-specific manifold space.

□ INFIMA leverages multi-omics model organism data to identify effector genes of human GWAS variants
>> https://www.biorxiv.org/content/10.1101/2021.07.15.452422v1.full.pdf
INFIMA, a statistically grounded framework to capitalize on multi-omics functional data and fine-map model organism molecular quantitative trait loci. INFIMA leverages multiple multi-omics data modalities to elucidate causal variants underpinning the DO islet eQTLs.
INFIMA links ATAC-seq peaks and local-ATAC-MVs to candidate effector genes by fine-mapping DO-eQTLs. As the ability to measure inter-chromosomal interactions matures, incorporating trans-eQTLs into INFIMA framework would be a natural extension.

□ CCPE: Cell Cycle Pseudotime Estimation for Single Cell RNA-seq Data
>> https://www.biorxiv.org/content/10.1101/2021.06.13.448263v1.full.pdf
CCPE maps high-dimensional scRNA-seq data onto a helix in three-dimensional space, where 2D space is used to capture the cycle information in scRNA-seq data, and one dimension to predict the chronological orders of cells along the cycle, which is called cell cycle pseudotime.
CCPE learns a discriminative helix to characterize the circular process and estimates pseudotime in the cell cycle. CCPE iteratively optimizes the discriminative dimensionality reduction via learning a helix until convergence.

□ GRIDSS2: comprehensive characterisation of somatic structural variation using single breakend variants and structural variant phasing
>> https://genomebiology.biomedcentral.com/articles/10.1186/s13059-021-02423-x
GRIDSS2 utilises the same high-level approach as the first version of GRIDSS, assembling all reads that potentially support a structural variant using a positional de Bruijn graph breakend assembly algorithm.
GRIDSS2’s ability to phase breakpoints involving short DNA fragments is of great utility to downstream rearrangement event classification and karyotype reconstruction as it exponentially reduces the number of possible paths through the breakpoint graph.
GRIDSS2’s ability to collapse imprecise transitive calls into their corresponding precise breakpoints is similarly essential to complex event reconstruction as these transitive calls result in spurious false positives that are inconsistent with the actual rearrangement structure.

□ VSS: Variance-stabilized signals for sequencing-based genomic signals
>> https://academic.oup.com/bioinformatics/advance-article/doi/10.1093/bioinformatics/btab457/6308936
Most Gaussian-based methods employ a variance-stabilizing transformation to handle the nonuniform mean-variance relationship. They most commonly use the log or inverse hyperbolic sine transformations.
VSS, a method that produces variance-stabilized signals for sequencing- based genomic signals. Having learned the mean-variance relationship, VSS can be generated using the variance-stabilizing transformation.
VSS uses the zero bin for raw and fold enrichment (FE) signals, but not log Poisson p-value (LPPV), which are not zero-inflated. And using variance-stabilized signals from VSS improves annotations by SAGA algorithms.

□ SIVS: Stable Iterative Variable Selection
>> https://academic.oup.com/bioinformatics/advance-article/doi/10.1093/bioinformatics/btab501/6322982
Stable Iterative Variable Selection (SIVS) starts from aggregating the results of multiple multivariable modeling runs using different cross-validation random seeds.
SIVS hired an iterative approach and internally utilizes varius Machine Learning methods which have embedded feature reduction in order to shrink down the feature space into a small and yet robust set. the "true signal" is more effectively captured by SIVS compared to the standard glmnet.

□ Metric Multidimensional Scaling for Large Single-Cell Data Sets using Neural Networks
>> https://www.biorxiv.org/content/10.1101/2021.06.24.449725v1.full.pdf
a neural network based approach for solving the metric multidimensional scaling problem that is orders of magnitude faster than previous state-of-the-art approaches, and hence scales to data sets with up to a few million cells.
metric MDS clustering approach provides a non-linear mapping between high-dimensional points into the low-dimensional space, that can place previously unseen cells in the same embedding.

□ lra: A long read aligner for sequences and contigs
>> https://journals.plos.org/ploscompbiol/article?id=10.1371/journal.pcbi.1009078
lra alignment approach increases sensitivity and specificity for SV discovery, particularly for variants above 1kb and when discovering variation from ONT reads, while having runtime that are comparable (1.05-3.76×) to current methods.
lra is a sequence alignment program that aligns long reads from single-molecule sequencing (SMS) instruments, or megabase-scale contigs from SMS assemblies.
lra implements seed chaining sparse dynamic programming with a concave gap function to read and assembly alignment, which is also extended to allow for inversion cases.
there are O(log(n)) subproblems it is in and in each subproblem EV[j] can be computed from the block structure EB in O(log(n)) time. it takes O((log(n))2) time. Since there are n fragments in total, the time complexity of processing all the points is bounded by O(n log(n)2).
□ SVNN: an efficient PacBio-specific pipeline for structural variations calling using neural networks
>> https://bmcbioinformatics.biomedcentral.com/articles/10.1186/s12859-021-04184-7
The logic behind this hypothesis was, only a small fraction of all reads (less than 1%) are used for SV detection, and these reads are usually mapped harder to the reference compared to normal reads and therefore might share some common characteristics which can be leveraged in a learning model.
SVNN is a pipeline for SV detection that intelligently combines Minimap2 and NGMLR as long read aligners for the mapping phase, and SVIM and Sniffles for the SV calling phase.
<bt />

□ IHPF: Dimensionality reduction and data integration for scRNA-seq data based on integrative hierarchical Poisson factorisation
>> https://www.biorxiv.org/content/10.1101/2021.07.08.451664v1.full.pdf
Integrative Hierarchical Poisson Factorisation (IHPF), an extension of HPF that makes use of a noise ratio hyper-parameter to tune the variability attributed to technical (batches) vs. biological (cell phenotypes) sources.
IHPF gene scores exhibit a well defined block structure across all scenarios. IHPF learns latent factors that have a dual block- structure in both cell and gene spaces with the potential for enhanced explainability and biological interpretability by linking cell types to gene clusters.

□ SEDR: Unsupervised Spatial Embedded Deep Representation of Spatial Transcriptomics
>> https://www.biorxiv.org/content/10.1101/2021.06.15.448542v1.full.pdf
Iterative deep clustering generates a soft clustering by assigning cluster-specific probabilities to each cell, leveraging the inferences between cluster-specific and cell-specific representation learning.
SEDR uses a deep autoencoder to construct a gene latent representation in a low-dimensional latent space, which is then simultaneously embedded with the corresponding spatial information through a variational graph autoencoder.

□ DeeReCT-TSS: A novel meta-learning-based method annotates TSS in multiple cell types based on DNA sequences and RNA-seq data
>> https://www.biorxiv.org/content/10.1101/2021.07.14.452328v1.full.pdf
DeeReCT-TSS uses a meta-learning-based extension for simultaneous transcription start site (TSS) annotation on 10 cell types, which enables the identification of cell-type-specific TSS.
the DNA sequence and the RNA-seq coverage in the 1000bp flanking window were converted into a 1000x4 (one-hot encoding) and 1000x1 vector. Both the DNA sequence and the RNA-seq coverage were fed into the network, resulting in the predicted value for each site in each TSS peak.

□ LongStitch: High-quality genome assembly correction and scaffolding using long reads https://www.biorxiv.org/content/10.1101/2021.06.17.448848v1.full.pdf
LongStitch runs efficiently and generates high-quality final assemblies. Long reads are used to improve upon an input draft assembly from any data type. If a project solely uses long reads, the LongStitch is able to further improve upon de novo long-read assemblies.
LongStitch incorporates multiple tools developed by our group and runs in up to three stages, which includes initial assembly correction using Tigmint-long, followed by two incremental scaffolding stages using ntLink and ARKS-long.

□ ECHO: Characterizing collaborative transcription regulation with a graph-based deep learning approach
>> https://www.biorxiv.org/content/10.1101/2021.07.01.450813v1.full.pdf
ECHO, a graph-based neural network, to predict chromatin features and characterize the collaboration among them by incorporating 3D chromatin organization from 200-bp high-resolution Micro-C contact maps.
ECHO, which mainly consists of convolutional layers, is more interpretable compared to ChromeGCN. ECHO leveraged chromatin structures and extracted information from the neighborhood to assist prediction.

□ Pheniqs 2.0: accurate, high-performance Bayesian decoding and confidence estimation for combinatorial barcode indexing
>> https://bmcbioinformatics.biomedcentral.com/articles/10.1186/s12859-021-04267-5
Pheniqs computes the full posterior decoding error probability of observed barcodes by consulting basecalling quality scores and prior distributions, and reports sequences and confidence scores in Sequence Alignment/Map (SAM) fields.
Pheniqs achieves greater accuracy than minimum edit distance or simple maximum likelihood estimation, and it scales linearly with core count to enable the classification of over 11 billion reads.

□ CStreet: a computed Cell State trajectory inference method for time-series single-cell RNA sequencing data
>> https://academic.oup.com/bioinformatics/advance-article/doi/10.1093/bioinformatics/btab488/6312549
CStreet estimates the connection probabilities of the cell states and visualizes the trajectory, which may include multiple starting points and paths, using a force-directed graph.
CStreet uses a distribution-based parameter interval estimation to measure the transition probabilities of the cell states, while prior approaches used scoring, such as the percentages of votes or the mutual information of the cluster pathway enrichment used by Tempora.
The Hamming–Ipsen–Mikhailov (HIM) score is a combination of the Hamming distance and the Ipsen- Mikhailov distance to quantify the difference in the trajectory topologies.

□ scGCN is a graph convolutional networks algorithm for knowledge transfer in single cell omics
>> https://www.nature.com/articles/s41467-021-24172-y
scGCN nonlinearly propagates feature information from neighboring cells in the hybrid graph, which learns the topological cell relations and improves the performance of transferring labels by considering higher-order relations between cells.
scGCN learns a sparse and hybrid graph of both inter- and intra-dataset cell mappings using mutual nearest neighbors of canonical correlation vectors. scGCN projects different datasets onto a correlated low-dimensional space.
□ scSGL: Signed Graph Learning for Single-Cell Gene Regulatory Network Inference
>> https://www.biorxiv.org/content/10.1101/2021.07.08.451697v1.full.pdf
scSGL incorporates the similarity and dissimilarity between observed gene expression data to construct gene networks. scSGL is formulated as a non-convex optimization problem and solved using an efficient ADMM framework.
scSGL reconstructs the GRN under the assumption that graph signals admit low-frequency representation over positive edges, while admitting high-frequency representation over negative edges.
□ StrobeAlign: Faster short-read mapping with strobemer seeds in syncmer space
>> https://www.biorxiv.org/content/10.1101/2021.06.18.449070v1.full.pdf
Canonical syncmers can be created for specific parameter combinations and reduce the computational burden of computing the non-canonical randstrobes in reverse complement. Strobealign aligns short reads 3-4x faster than minimap2 and 15-23x faster than BWA and Bowtie2.
Strobealign and Accel-Align achieves the speedup at different stages in the alignment pipeline, -Strobealign in the seed-ing stage and Accel-Align in the filtering stage, they have the potential to be combined.
□ SDDScontrol: A Near-Optimal Control Method for Stochastic Boolean Networks
>> https://www.ncbi.nlm.nih.gov/pmc/articles/PMC8208226/
The method requires a set of control actions such as the silencing of a gene or the disruption of the interaction between two genes. An optimal control policy defined as the best intervention at each state of the system can be obtained using existing methods.
the complexity of the proposed algorithm does not depend on the number of possible states of the system, and can be applied to large systems. And uses approximation techniques from the theory of Markov decision processes and reinforcement learning.
the method generates control actions that approximates the optimal control policy with high probability with a computational efficiency that does not depend on the size of the state space.
□ causalDeepVASE: Causal inference using deep-learning variable selection identifies and incorporates direct and indirect causalities in complex biological systems
>> https://www.biorxiv.org/content/10.1101/2021.07.17.452800v1.full.pdf
causalDeepVASE identifies associated variables in a pairwise Markov Random Field or undirected graphical model.
causalDeepVASE develops a penalized regression function with the interaction terms connecting the response variable and each of the other variables and maximizes the likelihood with sparsity penalties.

□ PVS: Pleiotropic Variability Score: A Genome Interpretation Metric to Quantify Phenomic Associations of Genomic Variants
>> https://www.biorxiv.org/content/10.1101/2021.07.18.452819v1.full.pdf
PVS uses ontologies of human diseases and medical phenotypes, namely human phenotype ontology (HPO) and disease ontology (DO), to compute the similarities of disease and clinical phenotypes associated with a genetic variant based on semantic reasoning algorithms.
The Stojanovic method does not need to traverse through the entire ontology to derive the similarity but the computation will terminate upon finding a common parent term using shortest path.
PVS provides a single metric by wrapping the entire compendium of scoring methods to capture phenomic similarity to quantify pleiotropy.

□ GraphCS: A Robust and Scalable Graph Neural Network for Accurate Single Cell Classification
>> https://www.biorxiv.org/content/10.1101/2021.06.24.449752v1.full.pdf
GraphCS, a robust and scalable GNN-based method for accurate single cell classification, where the graph is constructed to connect similar cells within and between labelled and unlabelled scRNA-seq datasets for propagation of shared information.
To overcome the slow information propagation of GNN at each training epoch, the diffused information is pre-calculated via the approximate Generalized PageRank algorithm, enabling sublinear complexity for a high speed and scalability on millions of cells.
□ Klarigi: Explanations for Semantic Groupings
>> https://www.biorxiv.org/content/10.1101/2021.06.14.448423v1.full.pdf
Hypergeometric gene enrichment is a univariate method, while Klarigi produces sets of terms which, considered individually or together, exclusively characterises multiple groups.
Klarigi is based upon the ε-constraints solution, retaining overall inclusivity as the objective function.
Klarigi creates semantic explanations for groups of entities described by ontology terms implemented in a manner that balances multiple scoring heuristics. As such, it presents a contribution to the reduction of unexplainability in semantic analysis.

□ seqgra: Principled Selection of Neural Network Architectures for Genomics Prediction Tasks
>>
seqgra, a deep learning pipeline that incorporates the rule-based simulation of biological sequence data and the training and evaluation of models, whose decision boundaries mirror the rules from the simulation process.
seqgra can serve as a testbed for hypotheses about biological phenomena or as a means to investigate the strengths and weaknesses of various feature attribution methods across different NN architectures that are trained on data sets with varying degrees of complexity.
□ Nanopore callers for epigenetics from limited supervised data
>> https://www.biorxiv.org/content/10.1101/2021.06.17.448800v1.full.pdf
DeepSignal outperforms a common HMM approach (Nanopolish) in the incomplete data setting. Amortized-HMM is a novel hybrid HMM-DNN approach that outperforms both the pure HMM and DNN approaches on 5mC calling when the training data are incomplete.
Amortized-HMM reduces the substantial computational burden, all reported experiments used architecture searches only from the k-mer-complete setting using DeepSignal. Amortized-HMM uses the Nanopolish HMM, w/ any missing modified k-mer emission distributions imputed by the FDNN.

□ splatPop: simulating population scale single-cell RNA sequencing data
>> https://www.biorxiv.org/content/10.1101/2021.06.17.448806v1.full.pdf
The splatPop model utilizes the flexible framework of Splatter to simulate data with complex experimental designs, including designs with batch effects, multiple cell groups (e.g., cell-types), and individuals with conditional effects.
splatPop can simulate populations where there are no batches, where all individuals are present in multiple batches, or where a subset of individuals are present in multiple batches as technical replicates.

□ DeepMP: a deep learning tool to detect DNA base modifications on Nanopore sequencing data
>> https://www.biorxiv.org/content/10.1101/2021.06.28.450135v1.full.pdf
DeepMP, a convolutional neural network (CNN)-based model that takes information from Nanopore signals and basecalling errors to detect whether a given motif in a read is methylated or not.
DeepMP introduces a threshold-free position modification calling model sensitive to sites methylated at low frequency across cells. DeepMP achieved a significant separation compared to Megalodon, DeepSignal, and Nanopolish.
DeepMP's architecture: The sequence module involves 6 1D convolutional layers w/ 256 1x4 filters. The error module comprises 3 1D layers & 3 locally connected layers both w/ 128 1x3 filters. Outputs are finally concatenated and inputted into a fully connected layer w/ 512 units.
□ Hamiltonian Monte Carlo method for estimating variance components:
>> https://onlinelibrary.wiley.com/doi/10.1111/asj.13575
Hamiltonian Monte Carlo is based on Hamiltonian dynamics, and it follows Hamilton's equations, which are expressed as two differential equations.
In the sampling process of Hamiltonian Monte Carlo, a numerical integration method called leapfrog integration is used to approximately solve Hamilton's equations, and the integration is required to set the number of discrete time steps and the integration stepsize.
□ CALLR: a semi-supervised cell-type annotation method for single-cell RNA sequencing data
>> https://academic.oup.com/bioinformatics/article/37/Supplement_1/i51/6319673
CALLR (Cell type Annotation using Laplacian and Logistic Regression) combines unsupervised learning represented by the graph Laplacian matrix constructed from all the cells and super- vised learning using sparse logistic regression.
The implementation of CALLR is based on general and rigorous theories behind logistic regression, spectral clustering and graph- based Merriman–Bence–Osher scheme.
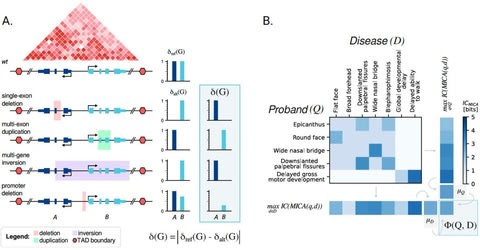
□ SvAnna: efficient and accurate pathogenicity prediction for coding and regulatory structural variants in long-read genome sequencing
>> https://www.biorxiv.org/content/10.1101/2021.07.14.452267v1.full.pdf
Structural Variant Annotation and Analysis (SvAnna) assesses all classes of SV and their intersection with transcripts and regulatory sequences in the context of topologically associating domains, relating predicted effects on gene function with clinical phenotype data.
SvAnna filters out common SVs and calculates a numeric priority score for the remaining rare SVs by integrating information about genes, promoters, and enhancers with phenotype matching to prioritize potential disease-causing variants.
□ scQcut: A completely parameter-free method for graph-based single cell RNA-seq clustering
>> https://www.biorxiv.org/content/10.1101/2021.07.15.452521v1.full.pdf
scQcut employs a topology-based criterion to guide the construction of KNN graph, and then applies an efficient modularity-based community discovery algorithm to predict robust cell clusters.
scQcut computes a distance matrix (or similarity matrix) using a given distance metric, and then computes a series of KNN graphs with different values of k. scQcut ambiguously determines the optimal co-expression network, and subsequently the most appropriate number of clusters.
□ AGTAR: A novel approach for transcriptome assembly and abundance estimation using an adapted genetic algorithm from RNA-seq data
>> https://www.sciencedirect.com/science/article/abs/pii/S0010482521004406
the adapted genetic algorithm (AGTAR) program, which can reliably assemble transcriptomes and estimate abundance based on RNA-seq data with or without genome annotation files.
Isoform abundance and isoform junction abundance are estimated by an adapted genetic algorithm. The crossover and mutation probabilities of the algorithm can be adaptively adjusted to effectively prevent premature convergence.
□ OMclust: Finding Overlapping Rmaps via Gaussian Mixture Model Clustering
>> https://www.biorxiv.org/content/10.1101/2021.07.16.452722v1.full.pdf
OMclust, an efficient clustering-based method for finding related Rmaps with high precision, which does not require any quantization or noise reduction.
OMclust performs a grid search to find the best parameters of the clustering model and replaces quantization by identifying a set of cluster centers and uses the variance of the cluster centers to account for the noise.