

□ ZODIAC: database-independent molecular formula annotation using Gibbs sampling reveals unknown small molecules
>> https://www.biorxiv.org/content/biorxiv/early/2019/11/16/842740.full.pdf
ZODIAC: database-independent molecular formula annotation using Gibbs sampling reveals unknown small molecules https://www.biorxiv.org/content/biorxiv/early/2019/11/16/842740.full.pdf
SIRIUS has become a powerful tool for the interpretation of tandem mass spectra, and shows outstanding performance for identifying the molecular formula of a query compound, being the first step of structure identification.
ZODIAC reranks SIRIUS' molecular formula candidates, combining fragmentation tree computation with Bayesian statistics using Gibbs sampling.

□ Quantifying pluripotency landscape of cell differentiation from scRNA-seq data by continuous birth-death process
>> https://journals.plos.org/ploscompbiol/article?id=10.1371/journal.pcbi.1007488
the Landscape of Differentiation Dynamics (LDD) method, which calculates cell potentials and constructs their differentiation landscape by a continuous birth-death process from scRNA-seq data.
From the viewpoint of stochastic dynamics, exploiting the features of the differentiation process and quantified the differentiation landscape based on the source-sink diffusion process.
Using LDD to compute both the pseudo-time and directed differentiation paths, which are also known as the differentiation landscape. Additionally, the reverse of the pseudo-time could be calculated for each cell type.

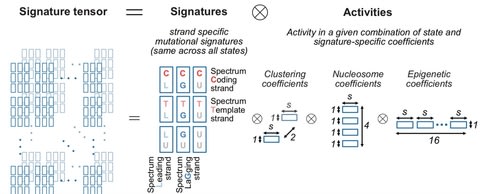
□ TensorSignatures: Learning mutational signatures and their multidimensional genomic properties
>> https://www.biorxiv.org/content/biorxiv/early/2019/11/21/850453.full.pdf
Matrix-based mutational signature analysis proved to be powerful in deconvolving mutational spectra into mutational signatures, yet it is limited in characterizing them with regard to their genomic properties.
TensorSignatures, an algorithm to learn mutational signatures jointly across all variant categories and their genomic context.
TensorSignatures is a multidimensional tensor factorisation framework, incorporating the aforementioned features for a more comprehensive and robust extraction of mutational signatures using an overdispersed statistical model.
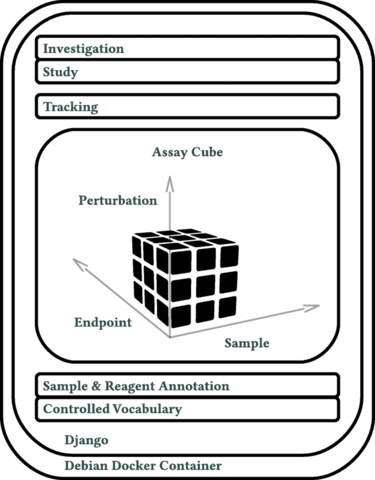
□ Annot: a Django-based sample, reagent, and experiment metadata tracking system
>> https://bmcbioinformatics.biomedcentral.com/articles/10.1186/s12859-019-3147-0
The cornerstone of Annot’s implementation is a json syntax-compatible file format, which can capture detailed metadata for all aspects of complex biological experiments.
Annot can store detailed information about diverse reagents and sample types, each defined by a “brick.” Annot is implemented in Python3 and utilizes the Django web framework, Postgresql, Nginx, and Debian.

□ A Sequence Distance Graph framework for genome assembly and analysis
>> https://f1000research.com/articles/8-1490
The Sequence Distance Graph (SDG) is a framework to work with genome graphs and sequencing data. It provides a workspace built around a Sequence Distance Graph, datastores for paired, linked and long reads, read mappers, and k-mer counters.
SDG framework works with genome assembly graphs and raw data from paired, linked and long reads. It includes a simple deBruijn graph module, and can import graphs using the graphical fragment assembly (GFA) format.
□ OLOGRAM: Determining significance of total overlap length between genomic regions sets
>> https://academic.oup.com/bioinformatics/advance-article/doi/10.1093/bioinformatics/btz810/5613178
The Python GTF toolkit (pygtftk) package comes with a set of UNIX commands that can be accessed through the gtftk program. The gtftk program proposes several atomic tools to filter, convert, or extract data from GTF files.
OLOGRAM (OverLap Of Genomic Regions Analysis using Monte Carlo) computes overlap statistics between region and inter-region to fit a negative binomial model of the total overlap length, and annotation derived from Gene centric features enclosed in a Gene Transfer Format (GTF).
□ Exploring High-Dimensional Biological Data with Sparse Contrastive Principal Component Analysis
>> https://www.biorxiv.org/content/biorxiv/early/2019/11/09/836650.full.pdf
a combination of these techniques, sparse constrastive PCA (scPCA), which draws on cPCA to remove technical eects and on SPCA for sparsification of the loadings, thereby extracting interpretable, stable, and uncontaminated signal from high-dimensional biological data.
SPCA provides a transparent method for the sparsification of loading matrices, its development stopped short of providing means by which to identify the most relevant directions of variation, presenting an obstacle to its efficacious use in biological data exploration.
SPCA generates interpretable and stable loadings in high dimensions, with most entries of the matrix being zero.
□ NanoSatellite: accurate characterization of expanded tandem repeat length and sequence through whole genome long-read sequencing on PromethION
>> https://genomebiology.biomedcentral.com/articles/10.1186/s13059-019-1856-3
NanoSatellite, a novel pattern recognition algorithm, which bypasses base calling and alignment, and performs direct Tandem Repeats analysis on raw PromethION squiggles. achieved more than 90% accuracy and high precision (5.6% relative standard deviation).
NanoSatellite is based on consecutive rounds of Dynamic Time Warping (DTW), a dynamic programming algorithm to find the optimal alignment between two (unevenly spaced) time series.
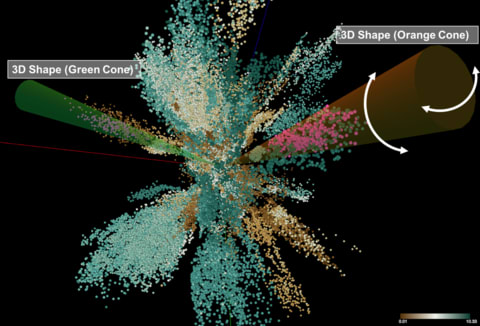
□ Visual Analytics for Deep Embeddings of Large Scale Molecular Dynamics Simulations
>> https://www.biorxiv.org/content/biorxiv/early/2019/11/04/830844.full.pdf
specific dimensionality reduction algorithm using deep learning technique has been employed here to embed the high-dimensional data in a lower-dimension latent space that still preserves the inherent molecular characteristics i.e. retains biologically meaningful information.
This system enables exploration and discovery of meaningful and semantic embedding results and supports the understanding and evaluation of results by the quantitatively described features of the Molecular Dynamics simulations.

□ AtacWorks: A deep convolutional neural network toolkit for epigenomics
>> https://www.biorxiv.org/content/biorxiv/early/2019/11/04/829481.full.pdf
AtacWorks performs denoising at single-base pair resolution, and adapts transcription factor “footprinting”, that leverages the fact that transcription factor-bound DNA is inaccessible in order to identify characteristic insertion signatures and predict binding across the genome.
AtacWorks uses a Resnet (residual neural network) model consisting of multiple stacked residual blocks composed of 1-dimensional convolutional layers and ReLU activation functions.
AtacWorks trained models using high-coverage (100 million reads) ATAC-seq data from FACS-sorted NK cells2 , downsampled to a range of lower sequencing depths (0.2 - 70 million reads), and tested them on ATAC-seq data from HSCs downsampled to the same sequencing depths.

□ DeepPheno: Predicting single gene knockout phenotypes
>> https://www.biorxiv.org/content/biorxiv/early/2019/11/13/839332.full.pdf
DeepPheno is a method for predicting gene-phenotype associations from gene functional annotations. DeepPheno annotations can be used to prioritize gene– disease associations whereas the naive annotations do not perform better than a random classifier.
the naive classifier achieves an Fmax close to DeepPheno and other phenotype prediction models because of the propagation of annotations using the hierarchical structure.

□ ASTAR-Seq: Parallel Bimodal Single-cell Sequencing of Transcriptome and Chromatin Accessibility
>> https://www.biorxiv.org/content/biorxiv/early/2019/11/04/829960.full.pdf
ASTAR-Seq (Assay for Single-cell Transcriptome and Accessibility Regions) integrated with automated microfluidic chips, which allows for parallel sequencing of transcriptome and chromatin accessibility within the same single-cell.
Multilayers of information collected by ASTAR-Seq allows for the identification of regulatory regions and the genes being regulated, which together contribute to the cellular heterogeneity.
□ Diversification of Reprogramming Trajectories Revealed by Parallel Single-cell Transcriptome and Chromatin Accessibility Sequencing
>> https://www.biorxiv.org/content/biorxiv/early/2019/11/04/829853.full.pdf
Parallel scRNA-Seq and scATAC-Seq analysis reveals that the cells undergoing reprogramming proceed in an asynchronous trajectory and diversify into heterogeneous sub-populations.
The toolkits to decipher the intermediate cells with different stemness capacity, will help deepen our understanding of the regulatory phasing of reprogramming process.

□ High throughput, error corrected Nanopore single cell transcriptome sequencing
>> https://www.biorxiv.org/content/biorxiv/early/2019/11/05/831495.full.pdf
a UMI assignment strategy that tolerates sequencing errors where we compare the Nanopore UMI reads with the UMIs defined with high accuracy for the same gene and the same cell by Illumina sequencing.
Single cell Nanopore sequencing with UMIs (ScNaUmi-seq), an approach that combines Oxford Nanopore sequencing with unique molecular identifiers to obtain error corrected full length sequence information with the 10xGenomics single cell isolation system.


□ DRAMS: A Tool to Detect and Re-Align Mixed-up Samples for Integrative Studies of Multi-omics Data
>> https://www.biorxiv.org/content/biorxiv/early/2019/11/06/831537.full.pdf
DRAMS uses a logistic regression model followed by a modified topological sorting algorithm to identify the potential true IDs based on data relationships of multi-omics.
DRAMS estimates pairwise genetic relatedness among all the data generated, and cluster all the highly related data and consider from one cluster have only one potential ID. Then, using a “majority vote” strategy to infer the potential ID for individuals in each cluster.
□ MKpLMM: Multi-kernel linear mixed model with adaptive lasso for prediction analysis on high-dimensional multi-omics data
>> https://academic.oup.com/bioinformatics/advance-article-abstract/doi/10.1093/bioinformatics/btz822/5613801
MKpLMM can capture not only the predictive effects from each layer of omics data but also their interactions via using multiple kernel functions.
MkPLMM adopts a data-driven approach to select predictive regions as well as predictive layers of omics data, and achieves robust selection performance.

□ PhenomeXcan: Mapping the genome to the phenome through the transcriptome
>> https://www.biorxiv.org/content/biorxiv/early/2019/11/06/833210.full.pdf
a novel Bayesian colocalization method, fastENLOC, to prioritize the most likely causal gene-trait associations.
Its resource, PhenomeXcan, synthesizes 8.87 million variants from GWAS on 4,091 traits with transcriptome regulation data from 49 tissues in GTEx v8 into an innovative, gene-based resource including 22,255 genes.
□ Hopper: A Mathematically Optimal Algorithm for Sketching Biological Data
>> https://www.biorxiv.org/content/biorxiv/early/2019/11/08/835033.full.pdf
Hopper realizes the optimal polynomial-time approximation of the Hausdorff distance between the full and downsampled dataset, ensuring that each cell is well-represented by some cell in the sample.
Hopper, a single-cell toolkit that both speeds up the analysis of single-cell datasets and highlights their transcriptional diversity by intelligent subsampling, or sketching.

□ scGAIN: Single Cell RNA-seq Data Imputation using Generative Adversarial Networks
>> https://www.biorxiv.org/content/biorxiv/early/2019/11/12/837302.full.pdf
scGAIN suites large scRNA-seq datasets with thousands and millions of cells which is infeasible for most statistical approaches to deal with.
using capabilities of GANs for accurately and efficiently imputing zero gene expressions due to technical dropdown and dropouts in scRNA-seq data using scGAIN model.

□ Neural Gene Network Constructor: A Neural Based Model for Reconstructing Gene Regulatory Network
>> https://www.biorxiv.org/content/biorxiv/early/2019/11/14/842369.full.pdf
The design of multi-layer perceptron (MLP), dynamic learner in NGNC model could approximate the underlying mechanism of gene regulation without any restriction on model of regulation such as linearality.
It consists a network generator which incorporating gumbel softmax technique to generate candidate network structure, and adopts multiple feedforward neural networks on dynamics learning.
□ Assessing the shared variation among high-dimensional data matrices: a modified version of the Procrustean correlation coefficient https://www.biorxiv.org/content/biorxiv/early/2019/11/14/842070.full.pdf
The main advantage of IRLs over other matrix correlation coefficients is that it allows for estimating shared variation between two matrices according to the classical definition of variance partitioning used with linear models.
The second advantage of IRLs is that its definition implies that the variance/co-variance matrix of a set of matrices is positive-definite. That allows for estimating partial correlation coefficients matrix by inverting the variance/co-variance matrix.
□ DNA-BOT: A low-cost, automated DNA assembly platform for synthetic biology
>> https://www.biorxiv.org/content/biorxiv/early/2019/11/15/832139.full.pdf
The ability to explore a genetic design space by building extensive libraries of DNA constructs is essential for creating programmed biological systems that perform the desired functions.
the DNA-BOT platform, which combines highly accurate, open source Biopart Assembly Standard for Idempotent Cloning (BASIC) DNA assembly with the low-cost Opentrons OT-2 for automated DNA assembly.

□ Tempora: cell trajectory inference using time-series single-cell RNA sequencing data
>> https://www.biorxiv.org/content/biorxiv/early/2019/11/18/846907.full.pdf
Tempora takes as input a preprocessed gene expression matrix from a time-series scRNAseq and cluster labels for all cells, and calculates the average GE profiles, or centroids, of all clusters before transforming the data from GE space to pathway enrichment space using GSVA.
Tempora aligns cell types and states across time points using available batch and data set alignment methods, as well as biological pathway information, then infers trajectory relationships between these cell types using the available temporal ordering information.

□ GsVec: Comprehensive biological interpretation of gene signatures using semantic distributed representation
>> https://www.biorxiv.org/content/biorxiv/early/2019/11/18/846691.full.pdf
GsVec (Gene signature Vector) is a semantic method by taking a distributed representation method of sentences in natural language processing (NLP), extracting the biological gene signature, and comparing it with the gene signature to be interpreted to clarify the relevance.
a score to reduce the weight of genes that appeared in various signatures was calculated by determining the value obtained by dividing the total number of signatures for each gene by the number of signatures that contained the gene from the 1-hot vector - Inverse Signature Factor.
□ SWAPCounter: Counting Kmers for Biological Sequences at Large Scale
>> https://link.springer.com/article/10.1007%2Fs12539-019-00348-5
SWAPCounter is embedded with an MPI streaming I/O module for loading huge data set at high speed, and a counting bloom filter module for both memory and communication efficiency.
By overlapping all the counting steps, SWAPCounter achieves high scalability with high parallel efficiency. On Cetus supercomputer, SWAPCounter scales to 32,768 cores with 79% parallel efficiency (using 2048 cores as baseline) when processing 4 TB sequence data of 1000 Genomes.
□ G-Graph: An interactive genomic graph viewer
>> https://www.biorxiv.org/content/biorxiv/early/2019/11/18/803015.full.pdf
At the core of G-Graph is a custom-built generic scatterplot graphing module which is designed to be extensible.
G-Graph delivers smooth and rapid scrolling and zooming even for datasets with millions of points and line segments.
□ BRM: A statistical method for QTL mapping based on bulked segregant analysis by deep sequencing
>> https://academic.oup.com/bioinformatics/advance-article-abstract/doi/10.1093/bioinformatics/btz861/5631910
Bulked segregant analysis by deep sequencing (BSA-seq) has been widely used for QTL mapping. Determination of significance threshold, the key point for QTL identification, remains to be a problem that has not been well solved due to the difficulty of multiple testing correction.
Block Regression Mapping (BRM) is a statistical method for QTL mapping based on bulked segregant analysis by deep sequencing. BRM is robust to sequencing noise and is applicable to the case of low sequencing depth.

□ SSIPs: Semi-supervised identification of cell populations in single-cell ATAC-seq
>> https://www.biorxiv.org/content/biorxiv/early/2019/11/19/847657.full.pdf
Nodes of the first type represent cells from scATAC-seq with edges between them encoding information about cell similarity. A second set of nodes represents “supervising” datasets connected to cell nodes with edges that encode the similarity between that data and each cell.
Via global calculations of network influence, SSIPs allows us to quantify the influence of bulk data on scATAC-seq data and estimate the contributions of scATAC-seq cell populations to signals in bulk data.
□ alignparse: A Python package for parsing complex features from high-throughput long-read sequencing
>> https://www.biorxiv.org/content/biorxiv/early/2019/11/21/850404.full.pdf
alignparse is designed to align long sequencing reads (such as those from PacBio circular consensus sequencing) to targets, filter these alignments based on user-provided specifications, and parse out user-defined sequence features.
alignparse allows for the parsing of additional sequence features necessary for validating the quality of deep mutational scanning libraries, such as the presence of terminal sequences or other identifying tags.

□ SVJedi: Genotyping structural variations with long reads
>> https://www.biorxiv.org/content/biorxiv/early/2019/11/21/849208.full.pdf
SVJedi is a structural variation (SV) genotyper for long read data. Based on a representation of the different alleles, it estimates the genotype of each variant from specific alignements obtained.
The approach is implemented in the SVJedi software for the moment for the most common and studied types of SVs, deletion and insertion variants, which represent to date 99% of dbVar referenced SVs.
□ A Fast and Memory-Efficient Implementation of the Transfer Bootstrap
>> https://academic.oup.com/bioinformatics/advance-article/doi/10.1093/bioinformatics/btz874/5637754
Transfer Bootstrap allows to calculate TBE support metrics on extremely taxon-rich phylogenies, without constituting a computational limitation.
using a single thread on dataset D with 31, 749 taxa and 100 bootstrap trees, this implementation can compute TBE support values in under two minutes, while booster requires 916 minutes.
Transfer Bootstrap implementation show that RAxML-NG is two orders of magnitude faster than booster on all datasets, while RAxML-NG uses considerably less memory than booster.

□ Manhattan++: displaying genome-wide association summary statistics with multiple annotation layers
>> https://bmcbioinformatics.biomedcentral.com/articles/10.1186/s12859-019-3201-y
Most existing scripts generate a graph in a landscape orientation, which is not enough with ever-increasing number of discovered GWAS loci.
Manhattan++ software tool reads the genome-wide summary statistic on millions of variants and generates the transposed Manhattan++ plot with user defined annotations like gene-names, allele frequencies, variant consequence and summary statistics of loci.

□ Multiversal SpaceTime (MSpaceTime) Not Neural Network as Source of Intelligence in Generalized Quantum Mechanics, Extended General Relativity, Darwin Dynamics for Artificial Super Intelligence Synthesis
>> https://www.biorxiv.org/content/biorxiv/early/2019/11/29/858423.full.pdf
generalize the 4-Dimensional Hilbert Space based Discrete Quantum SpaceTime to N-Dimensional Hilbert Space based Discrete MSpaceTime as part of MSpaceTime.
a T-Symmetry extension and extending the 4-Dimensional Pseudo-Riemannian Manifold based Continuous Curved SpaceTime as part of MSpaceTime to N-Dimensional Pseudo-Riemannian Manifold based Continuous MSpaceTime extension, in modeling of Artificial Super Intelligence.
Holographic Complexity modeling and reduction of holographic computing and Holographic Learning.
Multiversal Synthesis-based Artificial Design Automation (ADA) categorizes all related concepts including Holographic Supersymmetry, Holographic Entanglement, Holographic Entropy as Holographic Mapping.
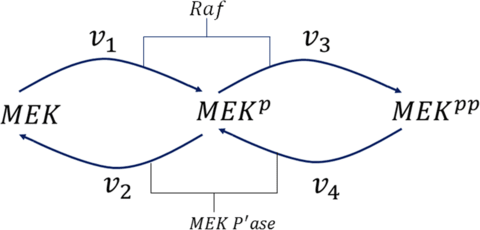
□ Detection of biological switches using the method of Gröebner bases
>> https://bmcbioinformatics.biomedcentral.com/articles/10.1186/s12859-019-3155-0
Analysis is based on the method of Instability Causing Structure Analysis. A necessary condition for fixed-point state bistability is for the Gröbner basis to have three distinct solutions for the state. A sufficient condition is provided by the eigenvalues of the Jacobians.
for a bistable system, the necessary conditions for output switchability can be derived using the Gröebner basis. theoretically, it is possible to have an output subspace of an n-dimensional bistable system where certain variables cannot switch.
□ wg-blimp: an end-to-end analysis pipeline for whole genome bisulfite sequencing data
>> https://www.biorxiv.org/content/biorxiv/early/2019/11/30/859900.full.pdf
wg-blimp integrates established algorithms for alignment, quality control, methylation calling, detection of differentially methylated regions, and methylome segmentation, requiring only a reference genome and raw sequencing data as input.
Since visualization of genomic data is often employed when inspecting analysis results, access links to alignment data for use with the Integrative Genomics Viewer (IGV) are also provided, as IGV provides a bisulfite mode for use with WGBS data.

□ Mini-batch optimization enables training of ODE models on large-scale datasets
>> https://www.biorxiv.org/content/biorxiv/early/2019/11/30/859884.full.pdf
combining mini-batch optimization with advanced numerical integration methods for parameter estimation of ODE models can help to overcome some major limitations.
adapt, apply, and benchmark mini-batch optimization for ordinary differential equation (ODE) models thereby establishing a direct link between dynamic modeling and machine learning.
□ MAtCHap: an ultra fast algorithm for solving the single individual haplotype assembly problem
>> https://www.biorxiv.org/content/biorxiv/early/2019/12/02/860262.full.pdf
MAtCHap, an ultra-fast algorithm that is capable to reconstruct the haplotype structure of a diploid genome, from a 30x sequencing coverage long read.
Based on a novel formulation of the haplotype assembly problem that aims to infer the two haplotypes that maximize the number of allele co-occurrence in the input fragments.