


□ Quantum mechanical electronic and geometric parameters for DNA k-mers as features for machine learning
>> https://www.biorxiv.org/content/10.1101/2023.01.25.525597v1
A large-scale semi-empirical quantum mechanical (QM) and geometric features calculations for all possible DNA heptamers in their three, B, A and Z, representative conformations. It used the same PM6-DH+ with COSMO solvation.
The DNA structures are optimized by using the semi-empirical Hamiltonian under the restricted Hartree-Fock approach. The procedure is comprised of: the building of the all-atom DNA models / geometry optimisation / feature extraction w/ the corresponding single-point calculations.

□ BLTSA: pseudotime prediction for single cells by Branched Local Tangent Space Alignment
>> https://academic.oup.com/bioinformatics/advance-article/doi/10.1093/bioinformatics/btad054/7000337
BLTSA infers single cell pseudotime for multi-furcation trajectories. By assuming that single cells are sampled from a low-dimensional self-intersecting manifold, BLTSA identifies the tip and branching cells in the trajectory based on cells’ local Euclidean neighborhoods.
A small value of nonlinearity implies a big gap b/n the d-th & (d+1)-th singular values and the neighborhood shows a strong d-dimensional linearity - A large value of nonlinearity implies a small gap b/n 2 singular values and the neighborhood shows a weak d-dimensional linearity.
BLTSA can be used directly from the high dimensional space to one-dimensional space. BLTSA propagates the reliable tangent information from non-branching cells to branching cells. Global coordinates for all the single cells are determined by aligning the local coordinates based on the tangent spaces.

□ Gemini: Memory-efficient integration of hundreds of gene networks with high-order pooling
>> https://www.biorxiv.org/content/10.1101/2023.01.21.525026v1
Gemini uses random walk with restart to compute the diffusion states. Gemini then uses fourth-order kurtosis pooling of the diffusion state matrix as the feature vectors to cluster all networks. Gemini assigns each network a weight inversely proportional to its cluster size.
Gemini randomly samples pairs of networks. These pairs of diffusion state matrices are then mixed-up to create a new simulated network collection. Gemini aggregates the synthetic dataset and perform an efficient singular value decomposition to produce embeddings for all vertices.

□ HQAlign: Aligning nanopore reads for SV detection using current-level modeling
>> https://www.biorxiv.org/content/10.1101/2023.01.08.523172v1
HQAlign (which is based on QAlign), which is designed specifically for detecting SVs while incorporating the error biases inherent in the nanopore sequencing process. HQAlign pipeline is modified to enable detection of inversion variants.
HQAlign takes the dependence of Q-mer map into account to perform accurate alignment with modifications specifically for discovery of SVs. the nucleotide sequences that have indistinguishable current levels from the lens of the Q-mer map are mapped to a common quantized sequence.

□ Ankh ☥: Optimized Protein Language Model Unlocks General-Purpose Modelling: Ankh unlocks the language of life via learning superior representations of its ”letters”, the amino acids.
>> https://www.biorxiv.org/content/10.1101/2023.01.16.524265v1
The Ankh architecture constructs the information flow in the network starting from the input sequences, pre-processing, transformer, and then either a residue-level / protein-level prediction network that only differs in being preceded by a global max pooling layer.
Ankh provides a protein variant generation analysis on High-N and One-N input data scales where it succeeds in learning protein evolutionary conservation-mutation trends and introducing functional diversity while retaining key structural-functional characteristics.

□ FAME: Efficiently Quantifying DNA Methylation for Bulk- and Single-cell Bisulfite Data
>> https://www.biorxiv.org/content/10.1101/2023.01.27.525734v1
FAME, the first bisulfite-aware (BA) mapping method with an index that is tailored for the alignment of BS reads with direct computation of CpGm values. The algorithm is working on the full alphabet (A,C,G,T), resolving the asymmetric mapping problem correctly.
FAME enables ultra-fast and parallel querying of reads without I/O overhead. FAME is built on a novel data structure that exploits gapped k-mer counting within short segments of the genome to quickly reduce the genomic search space.

□ xAtlas: scalable small variant calling across heterogeneous next-generation sequencing experiments
>> https://academic.oup.com/gigascience/article/doi/10.1093/gigascience/giac125/6987867
xAtlas, a lightweight and accurate single- sample SNV and small indel variant caller. xAtlas includes fea- tures that allow it to easily scale to population-scale sample sets, incl. support for CRAM and gVCF file formats, minimal computational requirements, and fast runtimes.
xAtlas determines the most likely genotype and reports the candidate variant. xAtlas supplies to the SNV and indel logistic regression models were compiled. xAtlas reports only the variant at that position with the greatest number of reads supporting the variant sequence.

□ TransImp: Towards a reliable spatial analysis of missing features via spatially-regularized imputation
>> https://www.biorxiv.org/content/10.1101/2023.01.20.524992v1
TransImp leverages a spatial auto-correlation metric as a regularization for imputing missing features in ST. Evaluation results from multiple platforms demonstrate that TransImp preserves the spatial patterns, hence substantially improving the accuracy of downstream analysis.
TransImp learns a mapping function to translate the scRNA-seq reference to ST data. Related to the Tangram model, TransImp learns a linear mapping matrix from the ST data. One can view it as a multivariate regression model, by treating gene as sample and cell as dimension.

□ scGREAT: Graph-based regulatory element analysis tool for single-cell multi-omics data
>> https://www.biorxiv.org/content/10.1101/2023.01.27.525916v1
scGREAT can generate the regulatory state matrix, which is a new layer of information. With the graph-based correlation scores, scGREAT filled the gap in multi-omics regulatory analysis by enabling labeled and unlabeled analysis, functional annotation, and visualization.
Using the same KNN graph constructed in the sub-clustering process, trajectory analysis was performed with functions in scGREAT utilizing diffusion pseudo-time implemented by Scanpy , and the pseudo-time labels were transferred back to single-cell data.


□ VIMCCA: A multi-view latent variable model reveals cellular heterogeneity in complex tissues for paired multimodal single-cell data
>> https://academic.oup.com/bioinformatics/advance-article/doi/10.1093/bioinformatics/btad005/6978155
VIMCCA uses a common latent variable to interpret the common source of variances in two different data modalities. VIMCCA jointly learns an inference model and two modality-specific non-linear models via variational optimization and multilayer neural network backpropagation.
VIMCCA projects the single latent factor into multi-modal observation spaces by modality-specific non-linear functions. VIMCCA allows us to directly integrate raw peak counts of scATAC-seq and gene expression of scRNA-seq without converting peak counts into gene activity matrix.

□ MetaCortex: Capturing variation in metagenomic assembly graphs
>> https://academic.oup.com/bioinformatics/advance-article/doi/10.1093/bioinformatics/btad020/6986127
MetaCortex, a de Bruijn graph metagenomic assembler that is built upon data structures and graph-traversal algorithms developed for the Cortex assembler.
MetaCortex captures variation by looking for signatures of polymorphisms in the de Bruijn graph constructed from the reads and represents this in sequence graph format (both FASTG and GFA v2), and the usual FASTA format.
MetaCortex generates sequence graph files that preserve intra-species variation (e.g. viral haplotypes), and implements a new graph traversal algorithm to output variant contig sequences.

□ Gentrius: Identifying equally scoring trees in phylogenomics with incomplete data
>> https://www.biorxiv.org/content/10.1101/2023.01.19.524678v1
Gentrius - a deterministic algorithm to generate binary unrooted trees from incomplete unrooted subtrees. For a tree inferred with any phylogenomic method and a species per locus presence-absence matrix, Gentrius generates all trees from the corresponding stand.
Gentrius systematically assesses the influence of missing data on phylogenomic analysis and enhances the confidence of evolutionary conclusions. When all trees from a stand are generated, one can subsequently study their topological differences employing phylogenetic routine.
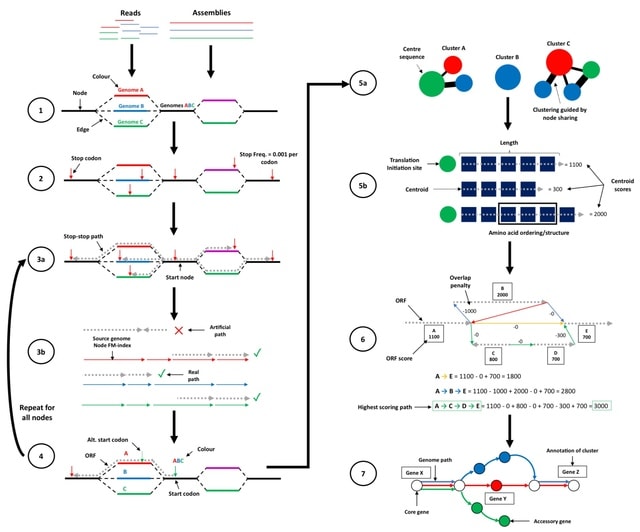
□ ggCaller: Accurate and fast graph-based pangenome annotation and clustering
>> https://www.biorxiv.org/content/10.1101/2023.01.24.524926v1
ggCaller (graph gene-caller), a population-wide gene-caller based on De Bruijn Graphs . ggCaller uses population-frequency information to guide gene prediction, aiding the identification of homologous start codons across orthologues, and consistent scoring of orthologues.
ggCaller traverses Bifrost graphs constructed from genomes to identify putative gene sequences, known as open reading frames (ORFs). ggCaller can be applied in pangenome-wide association studies (PGWAS), enabling reference- agnostic functional inference of significant hits.

□ RawHash: Enabling Fast and Accurate Real-Time Analysis of Raw Nanopore Signals for Large Genomes
>> https://www.biorxiv.org/content/10.1101/2023.01.22.525080v1
RawHash provides the mechanisms for generating hash values from both a raw nanopore signal and a reference genome such that similar regions between the two can be efficiently and accurately found by matching their hash values.
RawHash combines multiple consecutive quantized events into a single hash value. RawHash uses a chaining algorithm that find colinear matching hash values generated from regions that are close to each other both in the reference genome and the raw nanopore signal.

□ HNNVAT: Adversarial dense graph convolutional networks for single-cell classification
>> https://academic.oup.com/bioinformatics/advance-article/doi/10.1093/bioinformatics/btad043/6994183
HNNVAT, a hybrid neural network that not only extracts both low-order and high-order features of the data but also adaptively balances the features of the data extracted by different convolutional layers with self-attention mechanism.
HNNVAT uses virtual adversarial training to improve the generalization and robustness. A convolutional network structure w/ a dense connectivity mechanism is developed to extract comprehensive cell features and expression relationships b/n cells and genes in different dimensions.

□ ResActNet: Secure Deep Learning on Genomics Data via a Homomorphic Encrypted Residue Activation Network
>> https://www.biorxiv.org/content/10.1101/2023.01.16.524344v1
ResActNet, a novel homomorphic encryption (HE) scheme to address the nonlinear mapping issues in deploying secure deep models utilizing HE. ResActNet is built on a residue activation layer to fit the nonlinear mapping in hidden layers of deep models.
ResActNet employs a scaled power function as nonlinear activation, where a scalar term is worked for tuning the convergence of network. ResActNet deploys a residue activation strategy. ResActNet constraints the Scaled Power Activation (SPA) on the residue of latent vector.

□ EMERALD: Sensitive inference of alignment-safe intervals from biodiverse protein sequence clusters
>> https://www.biorxiv.org/content/10.1101/2023.01.11.523286v1
EMERALD effectively explores suboptimal alignment paths within the pairwise dynamic programming matrix. EMERALD embraces the diversity of possible alignment solutions, by revealing alignment-safe intervals of the two sequences.
EMERALD projects the safety intervals (safety windows) back to the representative sequence, thereby annotating the sequence intervals that are robust across all possible alignment configurations within the suboptimal alignment space.

□ PS-SNC: A partially shared joint clustering framework for detecting protein complexes from multiple state-specific signed interaction networks
>> https://www.biorxiv.org/content/10.1101/2023.01.16.524205v1
PS-SNC, a partially shared non-negative matrix factorization model to identify protein complexes in two state-specific signed PPI networks jointly. PS-SNC can not only consider the signs of PPIs, but also identify the common and unique protein complexes in different states.
PS-SNC employs the Hilbert-Schmidt Independence Criterion (HSIC) to construct the diversity constraint. HSIC can measure the dependence of variables by mapping variables to a Reproducing Kernel Hilbert Space (RKHS), which can measure more complicated correlations.

□ micrographs of 1D anatase-like materials, or 1DA, with each dot representing a Ti atom. (Cell Press)

□ NGC 346, one of the most dynamic star-forming regions in nearby galaxies. (esawebb)

□ EUROfusion
>> https://www.mpg.de/19734973/brennpunkte-der-kernfusion
#fusionenergy promises to be a clean and practically inexhaustible #energy source. But how do the different fusion designs compare?

□ UPP2: Fast and Accurate Alignment of Datasets with Fragmentary Sequences
>> https://academic.oup.com/bioinformatics/advance-article/doi/10.1093/bioinformatics/btad007/6982552
UPP2, a direct improvement on UPP (Ultra-large alignments using Phylogeny-aware Profiles). The main advance is a fast technique for selecting HMMs in the ensemble that allows us to achieve the same accuracy as UPP but with greatly reduced runtime.
UPP2 computes a set of subset alignments by hierarchically decomposing the backbone tree at a centroid edge. UPP2 builds an HMM on each set created during this decomposition, incl. the full set, thus producing a collection of ensemble of HMMs (eHMM) for the backbone alignment.

□ scDCCA: deep contrastive clustering for single-cell RNA-seq data based on auto-encoder network
>> https://academic.oup.com/bib/advance-article/doi/10.1093/bib/bbac625/6984787
By increasing the similarities between positive pairs and the differences between negative ones, the contrasts at both the instance and the cluster level help the model learn more discriminative features and achieve better cell segregation.
scDCCA extracts valuable features and realizes cell segregation end-to-end by introducing contrastive learning and denoising ZINB -based auto-encoder into a deep clustering framework. scDCCA incorporates a dual contrastive learning module to capture the pairwise cell proximity.

□ SemiBin2: self-supervised contrastive learning leads to better MAGs for short- and long-read sequencing
>> https://www.biorxiv.org/content/10.1101/2023.01.09.523201v1
SemiBin2 uses self-supervised learning to learn feature embeddings from the contigs. SemiBin2 can reconstruct 8.3%–21.5% more high-quality bins and requires only 25% of the running time and 11% of peak memory usage in real short-read sequencing samples.

□ xcore: an R package for inference of gene expression regulators
>> https://bmcbioinformatics.biomedcentral.com/articles/10.1186/s12859-022-05084-0
Xcore provides a flexible framework for integrative analysis of gene expression and publicly available TF binding data to unravel putative transcriptional regulators and their activities.
Xcore takes promoter or gene expression counts matrix as input, the data is then filtered for lowly expressed features, normalized for the library size and transformed into counts per million.
Xcore intersected the peaks with promoter regions and used linear ridge regression to infer the regulators associated with observed gene expression changes.

□ SiFT: Uncovering hidden biological processes by probabilistic filtering of single-cell data
>> https://www.biorxiv.org/content/10.1101/2023.01.18.524512v1
SiFT (SIgnal FilTering) uncovers underlying processes of interest. Utilizing existing prior knowledge and reconstruction tools for a specific biological signal, such as spatial structure, SiFT filters the signal and uncovers additional biological attributes.
SiFT computes a probabilistic cell-cell similarity kernel, which captures the similarity between cells according to the biological signal we wish to filter. Using this kernel, we obtain a projection of the cells onto the signal in gene expression space.

□ skani: Fast and robust metagenomic sequence comparison through sparse chaining with skani
>> https://www.biorxiv.org/content/10.1101/2023.01.18.524587v1
skani, a method for calculating average nucleotide identity (ANI) using sparse approximate alignments. skani is more accurate than FastANI for comparing incomplete, fragmented MAGs.
skani uses a very sparse k-mer chaining procedure to quickly find orthologous regions between two genomes. skani’s fast ANI filter first computes the max-containment index for a very sparse set of marker FracMin-Hash k-mers to approximate ANI.

□ VAG: Visualization and review of reads alignment on the graphical pan-genome
>> https://www.biorxiv.org/content/10.1101/2023.01.20.524849v1
VAG includes multifunctional modules integrated into a single command line and an online visualization platform supported through a web server. VAG can extract specific sequence regions from a graph pangenome and display read alignments on different paths of a graph pangenome.
The utilization of mate-pair information in VAG provides a reliable reference for variation identification. VAG enables to display inversions of the graph pangenome and the direction of read alignments on the forward or reverse strands.

□ NORTA: Investigating the Complexity of Gene Co-expression Estimation for Single-cell Data
>> https://www.biorxiv.org/content/10.1101/2023.01.24.525447v1
Zero-inflated Gaussian (ZI-Gaussian) assumes non-zero values of the normalized gene expression matrix following a Gaussian distribution. This strategy generates a co-expression network and constructs a partial correlation matrix (i.e., the inverse of the covariance matrix).
Zero-inflated Poisson (ZI-Poisson) generates a gene expression matrix through a linear combination. In order to have zeros, it then multiplies each element in the GE matrix with a Bernoulli random variable.
NORmal-To-Anything (NORTA) is based on the normal-to-anything approach that transformes multivariate Gaussian samples to samples with any given marginal distributions while preserving a given covariance.
Single-cell ExpRession of Genes In silicO (SERGIO) models the stochasticity of transcriptions and regulators with stochastic differential equations (SDEs). Concretely, it first generates dense gene expression matrix in logarithmic scale at stationary state.

□ Species-aware DNA language modeling
>> https://www.biorxiv.org/content/10.1101/2023.01.26.525670v1
In MLM, parts of an input sequence are hidden (masked) and a model is tasked to reconstruct them. Models trained in this way learn syntax and semantics of natural language and achieve state-of-the-art performance on many downstream tasks.
A state space model for language modeling in genomics. A species-aware masked nucleotide language model trained on a large corpus of species genomes can be used to reconstruct known RNA binding consensus motifs significantly better than chance and species-agnostic models.

□ DeepSelectNet: deep neural network based selective sequencing for oxford nanopore sequencing
>> https://bmcbioinformatics.biomedcentral.com/articles/10.1186/s12859-023-05151-0
DeepSelectNet is a deep neural network-based method capable of classifying species DNA directly using nanopore current signals with superior classification accuracy. DeepSelectNet is built on a convolutional architecture based on ResNet’s residual blocks.
DeepSelectNet utilizes one-dimensional convolutional layers to perform 1D convolution over nanopore current signals in the time domain. Additionally, DeepSelectNet relies on neural net regularization to minimise model complexity thereby reducing the overfitting of data.

□ Co-evolution integrated deep learning framework for variants generation and fitness prediction
>> https://www.biorxiv.org/content/10.1101/2023.01.28.526023v1
EVPMM (evolutionary integrated viral protein mutation machine), a co-evolution profiles integrated deep learning framework for dominant variants forecasting, vital mutation sites prediction and fitness landscape depicting.
EVPMM consists of a position detector to directly detect the functional positions as well as a mutant predictor to depict fitness landscape. Moreover, pairwise dependencies between residues obtained by a Markov Random Field are also incorporated to promote reasonable variant generation.

□ SSWD: A clustering method for small scRNA-seq data based on subspace and weighted distance
>> https://peerj.com/articles/14706/
SSWD follows the assumption that the sets of gene subspace composed of similar density-distributing genes can better distinguish cell groups. SSWD uses a new distance metric EP_dis, which integrates Euclidean and Pearson distance through a weighting strategy.
Each of the gene subspace’s clustering results was summarized using the consensus matrix integrated by PAM clustering. The relative Calinski-Harabasz (CH) index was used to estimate the cluster numbers instead of the CH index because it is comparable across degrees of freedom.

□ scDASFK: Denoising adaptive deep clustering with self-attention mechanism on single-cell sequencing data
>> https://academic.oup.com/bib/advance-article-abstract/doi/10.1093/bib/bbad021/7008799
scDASFK, a new adaptive fuzzy clustering model based on the denoising autoencoder and self-attention mechanism. It implements the comparative learning to integrate cell similar information into the clustering method and uses a deep denoising network module to denoise the data.
scDASFK consists of a self-attention mechanism for further denoising where an adaptive clustering optimization function for iterative clustering is implemented. scDASFK uses a new adaptive feedback mechanism to supervise the denoising process through the clustering.