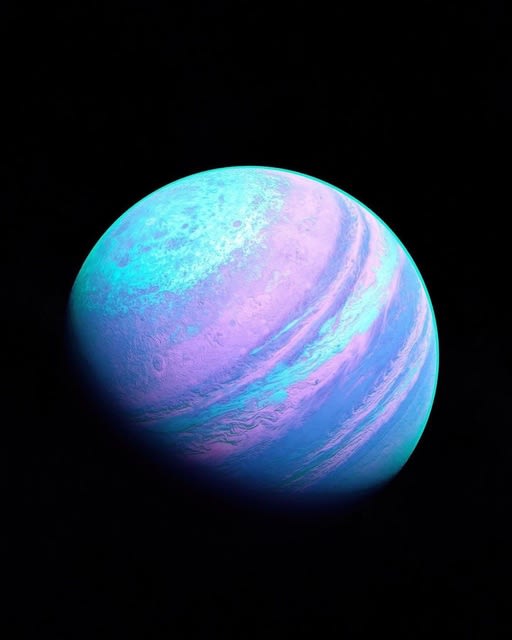
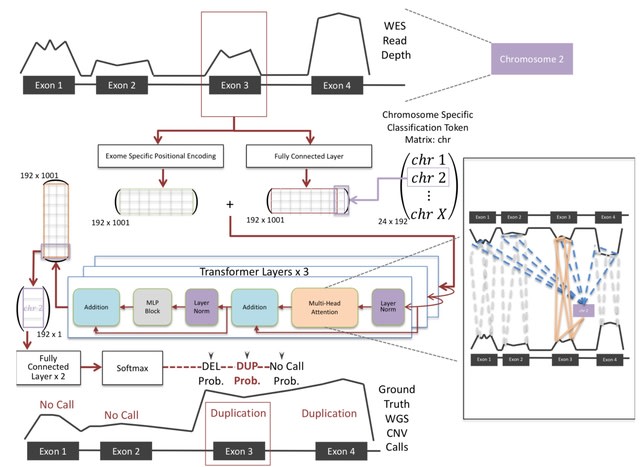
□ ÉCOLE: Learning to call copy number variants on whole exome sequencing data
>> https://www.biorxiv.org/content/10.1101/2022.11.17.516880v1
Based on a variant of the transformer architecture, ÉCOLE learns to call CNVs per exon, using high confidence calls made on matched WGS samples as the semi-ground truth. E ́COLE is able mimic the expert labeling for the first time with 68.7% precision and 49.6% recall.
ÉCOLE processes the read-depth signal over each exon. This information is transformed into a read depth embedding using a multi-layered perceptron. The model uses a positional encoding vector which is summed up w/ the transformed read depth encoding and the classification token.
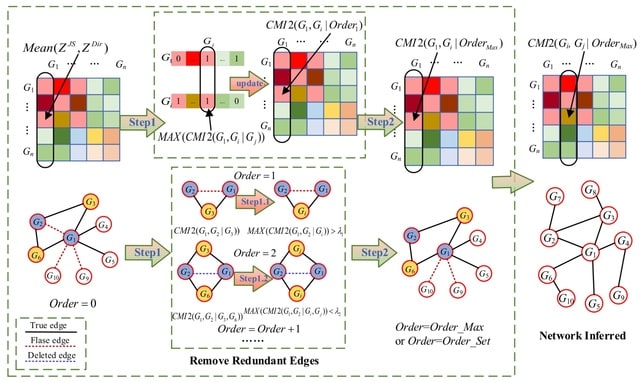
□ MEOMI: An Approach of Gene Regulatory Network Construction Using Mixed Entropy Optimizing Context-Related Likelihood Mutual Information
>> https://academic.oup.com/bioinformatics/advance-article/doi/10.1093/bioinformatics/btac717/6808612
MEOMI combines two entropy estimators to calculate the mutual information between genes. Then, distribution optimization was performed using a context-related likelihood algorithm to eliminate some indirect regulatory relationships and obtain the initial gene regulatory network.
MEOMI uses the conditional mutual inclusive information calculation method to gradually remove redundant edges. The conditional mutual inclusive information of a pair of genes under the influence of multiple related genes is calculated by multi-order traversal algorithm.

□ scmTE: multivariate transfer entropy builds interpretable compact gene regulatory networks by reducing false predictions
>> https://www.biorxiv.org/content/10.1101/2022.11.08.515579v1
scmTE, a new algorithm single-cell multivariate Transfer Entropy. scmTE is the unique algorithm that did not produce a hair-ball structure (due to too many predictions) and recapitulated known ground- truth relationships with high accuracy.
scmTE calculates causal relationships from a gene to a target gene while considering other genes that can influence the target. Similar to TE, mTE relies on the dynamic gene expression changes over time i.e. pseudo-time, the ordered trajectory.

□ scFormer: A Universal Representation Learning Approach for Single-Cell Data Using Transformers
>> https://www.biorxiv.org/content/10.1101/2022.11.20.517285v1
scFormer applies self-attention to learn salient gene and cell embeddings through masked gene modelling. scFormer provides a unified framework to readily address a variety of downstream tasks as data integration, analysis of gene function, and perturbation response prediction.
scFormer employs masked gene modelling to promote the learning of cross-gene relations, inspired by the masked-language modelling in NLM. The self-attention on gene expressions and the introduced MGM and MVC objectives significantly boost the cell-level and gene-level tasks.
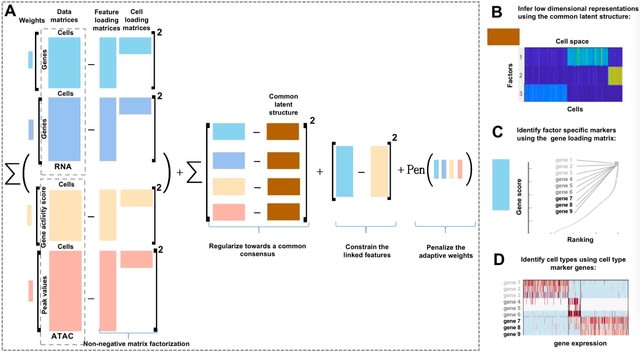
□ scAWMV: an Adaptively Weighted Multi-view Learning Framework for the Integrative Analysis of Parallel scRNA-seq and scATAC-seq Data
>> https://academic.oup.com/bioinformatics/advance-article/doi/10.1093/bioinformatics/btac739/6831091
scAWMV considers both the difference in importance across different modalities in multi-omics data and the biological connection of the features in the scRNA-seq and scATAC-seq data. It generates biologically meaningful low-dimensional representations for the transcriptomic and epigenomic profiles.
scAWMV is minimized via finding the optimal matrix factorization. scAWMV utilizes the linked information b/n the parallel transcriptomic and epigenomic layers. scAWMV uses Louvain clustering and groups the cells in the same clusters in the heatmap of the common latent structure.

□ mtANN: Cell-type annotation with accurate unseen cell-type identification using multiple references
>> https://www.biorxiv.org/content/10.1101/2022.11.17.516980v1
mtANN (multiple-reference-based scRNA-seq data annotation) learns multiple deep classification models from multiple reference datasets, and the multiple prediction results are used to calculate the metric for unseen cell-type identification and to vote for the final annotation.
mtANN integrates multiple references to enrich cell types in the reference atlas to alleviate the unseen cell-type problem. This metric is defined by three entropy indexes which are calculated from the prediction probability of multiple base classification and vote probability.

□ PAST: latent feature extraction with a Prior-based self-Attention framework for Spatial Transcriptomics
>> https://www.biorxiv.org/content/10.1101/2022.11.09.515447v1
PAST, a variational graph convolutional auto-encoder for ST, which effectively integrates prior information via a Bayesian neural network, captures spatial patterns via a self-attention mechanism, and enables scalable application via a ripple walk sampler strategy.
PAST identifies k nearest neighbors (k-NN) for each spot using spatial coordinates in a Euclidean space, and adopts GCNs to aggregate spatial patterns from each spot’s neighbors.
PAST restricts the distance of latent embeddings between neighbors through metric learning, the insight of which is that spatially close spots are more likely to be positive pairs to show similar latent patterns.

□ Bambu: Context-Aware Transcript Quantification from Long Read RNA-Seq data
>> https://www.biorxiv.org/content/10.1101/2022.11.14.516358v1
Bambu estimates the likelihood that a novel transcript is valid, allowing the filtering of transcript candidates with a single, interpretable parameter, the novel discovery rate, that is calibrated to guarantee a reproducible maximum false discovery rate across different samples.
Bambu then employs a statistical model to assign reads to transcripts that distinguishes full-length and non full-length (partial) reads, as well as unique and non-unique reads, thereby providing additional evidence from long read RNA-Seq to inform downstream analysis.

□ SCARP: Single-Cell ATAC-seq analysis via Network Refinement with peaks location information
>> https://www.biorxiv.org/content/10.1101/2022.11.18.517159v1
SCARP utilizes the genomic information of peaks, which contributed to characterizing co-accessibility of peaks. SCARP used network to model the accessible relationships between cells and peaks, aggregated information with the diffusion method.
The output matrix derived from SCARP can be further processed by the dimension reduction method to obtain low-dimensional embeddings of cells and peaks, which can benefit the downstream analyses such as the cells clustering and cis-regulatory relationships prediction.

□ iEnhancer-DCLA: using the original sequence to identify enhancers and their strength based on a deep learning framework
>> https://bmcbioinformatics.biomedcentral.com/articles/10.1186/s12859-022-05033-x
iEnhancer − 2 L uses pseudo k-tuple nucleotide composition (PseKNC) as the encoding method of sequence characteristics. iEnhancer -ECNN uses one-hot encoding and k-mers to process the data, and uses CNN to construct the ensemble model.
iEnhancer -XG combines k-spectrum profile, mismatch k-tuple, subsequence profile and position-specific scoring matrix, and constructs a two-layer predictor using XGBoost. iEnhancer-EBLSTM uses 3-mer to encode the input DNA sequences and predicts enhancers by bidirectional LSTM.
iEnhancer-DCLA uses word2vec to convert k-mers into number vectors to construct an input matrix. Secondly, It uses convolutional neural network and BiLSTM network to extract sequence features, and finally uses the attention mechanism to extract relatively important features.

□ INSIDER: Interpretable Sparse Matrix Decomposition for Bulk RNA Expression Data Analysis
>> https://www.biorxiv.org/content/10.1101/2022.11.10.515904v1
INSIDER decomposes variation from different biological variables into a shared low-rank latent space. In particular, it considers interactions between biological variables and introduces the elastic net penalty to induce sparsity, thus facilitating interpretation.
INSIDER computes the adjusted expression that controls for variation in other confounders or covariates. The variation is decomposed into a shared latent space of rank K by matrix factorization. INSIDER incorporates the interaction b/n covariates and the gene representation V.

□ The geometry of Coherent topoi and Ultrastructures
>> https://arxiv.org/abs/2211.03104v1
The geometric properties of coherent topoi with respect to flat embeddings, and let the notion of ultrastructure emerge naturally from general considerations on the topology of flat embeddings.
Ultrastructures were defined to condense the main properties of the category of models of a first order theory. This technology provides a reconstruction theorem for first order logic that goes under the name of conceptual completeness.

□ scSSA: A clustering method for single cell RNA-seq data based on semi-supervised autoencoder
>> https://www.sciencedirect.com/science/article/abs/pii/S1046202322002298
scSSA is based on semi-supervised autoencoder, Fast Independent Component Analysis and Gaussian mixture clustering. It is an autoencoder based on depth counting, which aims to learn a lower dimensional space so that the original space can be reconstructed accurately.
scSSA also attaches a supervised target. The Gaussian mixture clustering model performs cell clustering on the low dimensional matrix, obtains the clustering results and identifies the cell type, and obtains the clustering visualization through FastICA.

□ DeepCCI: a deep learning framework for identifying cell-cell interactions from single-cell RNA sequencing data
>> https://www.biorxiv.org/content/10.1101/2022.11.11.516061v1
DeepCCI provides two deep learning models: a GCN-based unsupervised model for cell clustering, and a GCN-based supervised model for CCI identification.
DeepCCI learns an embedding function that jointly projects cells into a shared embedding space using Autoencoder and GCN. DeepCCI predicts intercellular crosstalk between any pair of clusters.

□ m6Anet: Detection of m6A from direct RNA sequencing using a multiple instance learning framework
>> https://www.nature.com/articles/s41592-022-01666-1
m6Anet, a MIL-based neural network model that takes in signal intensity and sequence features to identify potential m6A sites from direct RNA-Seq data.
m6Anet takes into account the mixture of modified and unmodified RNAs and outputs the m6A-modification probability at any given site for all DRACH fivemers represented in the training data.
m6Anet learns a high-dimensional representation of individual reads from each candidate site before aggregating them together to produce a more accurate prediction of m6A sites.

□ metaMIC: reference-free misassembly identification and correction of de novo metagenomic assemblies
>> https://genomebiology.biomedcentral.com/articles/10.1186/s13059-022-02810-y
metaMIC is a fully automated tool for identifying and correcting misassemblies of (meta)genomic assemblies with the following three steps. Firstly, metaMIC extracts various types of features from the alignment between paired-end sequencing reads and the assembled contigs.
The features extracted in the first step will be used as input of a random forest classifier for identifying misassemblies. metaMIC will localize misassembly breakpoints for each misassembled contig and then correct misassemblies by splitting into parts at the breakpoints.

□ End-to-end learning of multiple sequence alignments with differentiable Smith-Waterman
>> https://academic.oup.com/bioinformatics/advance-article/doi/10.1093/bioinformatics/btac724/6820925
SMURF (Smooth Markov Unaligned Random Field), a new method that jointly learns an alignment and the parameters of a Markov Random Field for unsupervised contact prediction. SMURF takes as input unaligned sequences and jointly learns an MSA via LAM.
A Smooth Smith-Waterman (SSW) formulation in which the probability that any pair of residues is aligned can be formulated as a derivative.
LAM (Learned Alignment Module), a fully differentiable module for constructing MSAs and hence can be trained in conjunction with another differentiable downstream model. LAM employs a smooth and differentiable version of the Smith-Waterman algorithm.

□ Destin2: integrative and cross-modality analysis of single-cell chromatin accessibility data
>> https://www.biorxiv.org/content/10.1101/2022.11.04.515202v1
Destin2 is a statistical framework for cross-modality dimension reduction, clustering, and trajectory reconstruction of single-cell ATAC-seq data.
Destin2 integrates cellular-level epigenomic profiles from peak accessibility, motif deviation score, and pseudo-gene activity and learns a shared manifold using the multimodal input, followed by clustering and/or trajectory inference.

□ G2Φnet: Relating genotype and biomechanical phenotype of tissues with deep learning
>> https://journals.plos.org/ploscompbiol/article?id=10.1371/journal.pcbi.1010660
G2Φnet directly provides a functional expression for a parameterized constitutive relation based on the neural operator architecture. G2Φnet formulates the sample feature w/ a limited dimension, which together with the injected genotype feature composes the material parameters.
G2Φnet formulation is formally similar to the classical approach of constitutive modeling by analytical expressions, hence endowing the method with generalizability and transferability across different specimens in multiple material classes.

□ DELFOS oracle: Managing the evolution of genomics data over time: a conceptual model-based approach
>> https://bmcbioinformatics.biomedcentral.com/articles/10.1186/s12859-022-04944-z
Updating the DELFOS Oracle so that its architecture can manage the temporal dimension. the Delfos module to change from a static-data perspective to a dynamic-data perspective,
The DELFOS oracle consists of four interconnected modules (HERMES, ULISES, DELFOS, SIBILA) that implement each one of the stages of SILE (Search, Identification, Load, and Exploitation). SIBILA, a genomic information system automatizes the Exploitation stage of the SILE method.

□ APARENT2: Deciphering the impact of genetic variation on human polyadenylation
>> https://genomebiology.biomedcentral.com/articles/10.1186/s13059-022-02799-4
APARENT2, a residual neural network model that can infer 3′-cleavage and polyadenylation from DNA sequence more accurately than any previous model. This model generalizes to the case of alternative polyadenylation (APA) for a variable number of polyadenylation signals.
APARENT2 was considerably better at variant effect size estimation for cryptic variants outside of the CSE. APARENT2 can score cis-regulatory stability elements near the PAS, but that a more general stability model such as Saluki is beneficial for 3′ UTRs with long isoforms.
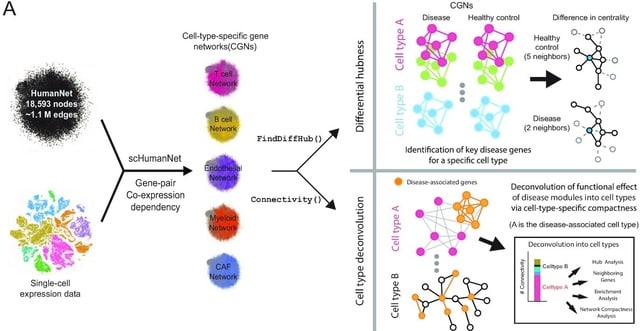
□ scHumanNet: a single-cell network analysis platform for the study of cell-type specificity of disease genes
>> https://academic.oup.com/nar/advance-article/doi/10.1093/nar/gkac1042/6814446
scHumanNet enables cell-type specific networks with scRNA-seq data. The SCINET framework takes a single cell gene expression profile and the “reference interactome” HumanNet v3, to construct a list of cell-type specific network.
HumanNet v3 with 1.1 million weighted edges are used as a scaffold information to infer the likelihood of each gene interactions. scHumanNet could prioritize genes associated with particular cell types using CGN centrality and identified the differential hubness of CGNs.

□ Multiple Sequence Alignment based on deep Q network with negative feedback policy
>> https://www.sciencedirect.com/science/article/abs/pii/S1476927122001608
Leveraging the Negative Feedback Policy (NFP) to enhance the performance and accelerate the convergence of the model. A new profile algorithm is developed to compute the sequence from aligned sequences for the next profile-sequence alignment to facilitate the experiment.
Compared to six state-of-the-art methods, three different genetic algorithms, Q-learning, ClustalW, and MAFFT, this method exceeds these methods in terms of Sum-of-Pairs score and Column Score scores on most datasets in which the increased range of SP score is from 2 to 1056.

□ scAN10: A reproducible and standardized pipeline for processing 10X single cell RNAseq data
>> https://www.biorxiv.org/content/10.1101/2022.11.07.515546v1
scAN10, a processing pipeline of 10X single cell RNAseq data, that inherits the ability to be executed on most computational infrastructures, thanks to Nextflow DSL2.
Filtrating the GTF by removing unwanted genes based on 10X reference had a major impact both on the number of genes but also on gene counts. When using Kallisto-bustools instead of Cellranger the impact of the count numbers for specific genes seemed to be small but meaningful.
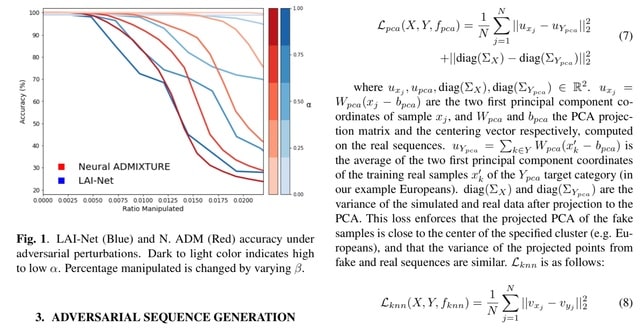
□ Adversarial Attacks on Genotype Sequences
>> https://www.biorxiv.org/content/10.1101/2022.11.07.515527v1
A gradient-based adversarial attack to change the prediction of commonly used genotype classification and segmentation methods (i.e. global and local ancestry inference), while minimally modifying the input sequences.
A d-dimensional binary ’mutation mask’ indicates which positions of the DNA sequence need to be changed. When the adversarial sequences are used as input, each method outputs the category specified as target label (EUR for PCA, AHG for k-NN, AMR for LAI-Net, and OCE for N. ADM).

□ Structured Joint Decomposition (SJD) identifies conserved molecular dynamics across collections of biologically related multi-omics data matrices
>> https://www.biorxiv.org/content/10.1101/2022.11.07.515489v1
SJD focuses specifically on within experiment variation and protects against warping of a single jointly learned manifold by between experiment variation that is often related to technological and/or batch effects.
SJD can process matrices from any data modality that uses systematic row names that map across matrices. Prior to running the SJD decomposition functions, the sjdWrap() function can be used to automatically find shared rows across all the input matrices.

□ SparkEC: speeding up alignment-based DNA error correction tools
>> https://bmcbioinformatics.biomedcentral.com/articles/10.1186/s12859-022-05013-1
SparkEC, a new parallel tool based on Apache Spark aimed at correcting errors in genomic reads that relies on accurate algorithms based on multiple sequence alignment strategies. SparkEC also uses a novel split-based processing strategy with a two-step k-mers distribution.
SparkEC relies on a hash-based partitioning strategy, which partitions the data based on the hashcode of the Resilient Distributed Datasets (RDD) elements. SparkEC defines the hashcode of the RDD elements in such a way that they get oddly distributed.
□ GTS: Genome Transformation Subprograms
>> https://github.com/go-gts/gts

□ Quasic: Reliable and accurate gene expression quantification with subpopulation structure-aware constraints for single-cell RNA sequencing
>> https://www.biorxiv.org/content/10.1101/2022.11.08.515740v1
Quasic, a novel scRNA-seq quantification pipeline which examines the potential cell subpopulation information during quantification, and uses the information to calculate the gene expression level.
Quasic uses the Louvain algorithm to perform clustering. Quasic could separate the doublet and the purified cell type cluster. Quasic not only correctly reinforced the cell signatures, but also identified the corresponding cell subpopulations and biological pathways accurately.

□ HCLC-FC: A novel statistical method for phenome-wide association studies
>> https://journals.plos.org/plosone/article?id=10.1371/journal.pone.0276646
HCLC-FC (Hierarchical Clustering Linear Combination with False discovery rate Control), a novel and powerful multivariate method, to test the association between a genetic variant with multiple phenotypes for each phenotypic category in PheWAS.
HCLC-FC uses the bottom-up Hierarchical Clustering Method (HCM) to partition a large number of phenotypes into disjoint clusters within each category.
The CLC combines test statistics within each phenotypic category and obtain p-values from each phenotypic category. A false discovery rate control based on a large-scale association testing procedure w/ theoretical guarantees for FDR control under flexible correlation structures.

□ Hybran: Hybrid Reference Transfer and ab initio Prokaryotic Genome Annotation
>> https://www.biorxiv.org/content/10.1101/2022.11.09.515824v1
Hybran, a hybrid reference-based and ab initio prokaryotic genome annotation pipeline that transfers features from a curated reference annotation and supplements unannotated regions with ab initio predictions.
Hybran uses the Rapid Annotation Transfer Tool (RATT) to transfer as many annotations as possible from reference genome annotation based on conserved synteny b/n the nucleotide genome sequences. Hybran then supplements unannotated regions with ab initio predictions from Prokka.

□ FASSO: An AlphaFold based method to assign functional annotations by combining sequence and structure orthology
>> https://www.biorxiv.org/content/10.1101/2022.11.10.516002v1
FASSO combines both sequence- and structure-based reciprocal best hit approaches to obtain a more accurate and complete set of orthologs across diverse species. FASSO provides confidence labels on ortholog predictions and flags potential misannotations in existing proteomes.
FASSO uses Diamond, FoldSeek, and FATCAT to find reciprocal best hits and aggregates those results for a final set of ortholog predictions. FASSO merges the results from each method, assigns confidence labels based on the level agreement, and removes conflicting predictions.

□ Moonlight: An Automatized Workflow to Study Mechanistic Indicators for Driver Gene Prediction
>> https://www.biorxiv.org/content/10.1101/2022.11.18.517066v1
Moonlight2 provides the user with the mutation-based mechanistic indicator to streamline the analyses of this second layer of evidence. The Moonlight Process Z-scores indicate if the activity of the process is increased or decreased based on literature reportings and gene expression levels.
One of the strengths of Moonlight is its classification of driver genes into TSGs and OGs which allows for the prediction of dual role genes - genes that are predicted as TSGs in one biological context but as OGs in another context.