

□ DeepGene: An Efficient Foundation Model for Genomics based on Pan-genome Graph Transformer
>> https://www.biorxiv.org/content/10.1101/2024.04.24.590879v1
DeepGene, a model leveraging Pan-genome and Minigraph representations to encompass the broad diversity of genetic language. DeepGene employs the rotary position embedding to improve the length extrapolation in various genetic analysis tasks.
DeepGene is based on a Transformer architecture w/ BPE tokenization for DNA segmentation. The input passes embedding layer and is fed into 12 Rope Transformer blocks to obtain the relative poisition information. DeepGene captures the extensive variability of genomic language.


□ KAN: Kolmogorov-Arnold Networks
>> https://arxiv.org/abs/2404.19756
Kolmogorov-Arnold Networks (KANs) are promising alternatives of Multi-Layer Perceptrons (MLPs). KANs have strong mathematical foundations just like MLPs: MLPs are based on the universal approximation theorem, while KANs are based on Kolmogorov-Arnold representation theorem
KANs have no linear weight matrices at all: instead, each weight parameter is replaced by a learnable 1D function parametrized as a spline. KANs’ nodes simply sum incoming signals without applying any non-linearities.

□ scSimGCL: Graph Contrastive Learning as a Versatile Foundation for Advanced scRNA-seq Data Analysis
>> https://www.biorxiv.org/content/10.1101/2024.04.23.590693v1
scSimGCL combines graph neural networks with contrastive learning, aligning with the GCL paradigm, specifically tailored for scRNA-seq data analysis. The GCL paradigm enables the generation of high-quality representations crucial for robust cell clustering.
scSimGCL uses a cell-cell graph structure learning mechanism that pays attention to the critical parts of the input data using a multi-head attention module for improving the accuracy and relevance of graphs.

□ RecGraph: recombination-aware alignment of sequences to variation graphs
>> https://academic.oup.com/bioinformatics/advance-article/doi/10.1093/bioinformatics/btae292/7658945
RecGraph is an exact approach that implements a dynamic programming algorithm for computing an optimal alignment between a string and a variation graph. Moreover, RecGraph can allow recombinations in the alignment in a controlled (i.e., non heuristic) way.
RecGraph can perform optimal alignment to path not included in the input graphs. This follows directly from the observation that a pangenome graph includes a set of related individuals that are represented as paths of the graph.
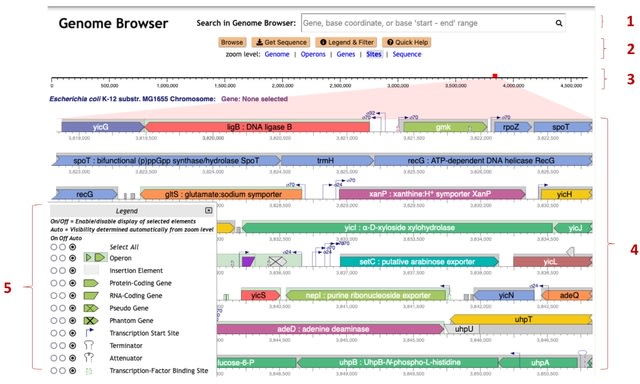
□ The Genome Explorer Genome Browser
>> https://www.biorxiv.org/content/10.1101/2024.04.24.590985v1
Genome Explorer, that provides nearly instantaneous scaling and traversing of a genome, enabling users to quickly and easily zoom into an area of interest. The user can rapidly move between scales that depict the entire genome, individual genes, and the sequence.
Genome Explorer presents the most relevant detail and context for each scale. Genome Explorer diagrams have high information density that provides larger amounts of genome context and sequence information.
Genome Explorer provides optional data tracks for analysis of large-scale datasets and a unique comparative mode that aligns genomes at orthologous genes with synchronized zooming.

□ DISSECT: deep semi-supervised consistency regularization for accurate cell type fraction and gene expression estimation
>> https://genomebiology.biomedcentral.com/articles/10.1186/s13059-024-03251-5
DISSECT can reliably deconvolve cell fractions using a two-step procedure. This approach is adopted because the assumptions underlying each algorithm differ, and there is no significant benefit expected from iteratively deconvolving cell type fractions and gene expression.
DISSECT estimates cell type fractions per spot, which are constrained to sum to 1. To be able to estimate the number of cells per cell type for each spot, and to map single cells, DISSECT estimates can be used as a prior for algorithms such as CytoSpace.

□ scTPC: a novel semi-supervised deep clustering model for scRNA-seq data
>> https://academic.oup.com/bioinformatics/advance-article/doi/10.1093/bioinformatics/btae293/7659796
scTPC integrates the triplet constraint, pairwise constraint and cross-entropy constraint based on deep learning. Specifically, the nodel begins by pre-training a denoising autoencoder based on a zero-inflated negative binomial (ZINB) distribution.
Deep clustering is then performed in the learned latent feature space using triplet constraints and pairwise constraints generated from partial labeled cells. Finally, to address imbalanced cell-type datasets, a weighted cross-entropy loss is introduced to optimize the model.

□ Nanomotif: Identification and Exploitation of DNA Methylation Motifs in Metagenomes using Oxford Nanopore Sequencing
>> https://www.biorxiv.org/content/10.1101/2024.04.29.591623v1
Nanomotif offers de novo methylated motif identification, metagenomic bin contamination detection, bin association of unbinned contigs, and linking of MTase genes to methylation motifs.
Nanomotif finds methylated motifs in individual contigs by first extracting windows of 20 bases upstream and downstream of highly methylated positions. Motif candidates are then built iteratively by considering enriched bases around the methylated position.
Afterwards, windows that constitute the specific motif are removed and the process repeated to identify additional motifs in the contig.
Motifs de novo identified in the contig are referred to as 'direct detected'. Afterwards, all direct detected motifs are scored across all contigs to identify missed motifs and referred to as 'indirect detected'.

□ xSiGra: Explainable model for single-cell spatial data elucidation
>> https://www.biorxiv.org/content/10.1101/2024.04.27.591458v1
xSiGra, an interpretable graph-based Al model, designed to elucidate interpretable features of identified spatial cell types, by harnessing multi-modal features from spatial imaging technologies. xSiGra employs hybrid graph transformer models to delineate spatial cell types.
XSiGra integrates a novel variant of Grad-CAM component to uncover interpretable features, including pivotal genes and cells for various cell types, thereby facilitating deeper biological insights from spatial data.

□ siRNADesign: A Graph Neural Network for siRNA Efficacy Prediction via Deep RNA Sequence Analysis
>> https://www.biorxiv.org/content/10.1101/2024.04.28.591509v1
siRNADesign, a GNN framework that thoroughly explores the sequence features of siRNA and mRNA with a specific topological structure. siRNADesign extracts two distinct-type features of RNA, i.e., non-empirical features and empirical-rules-based ones, and integrates them into GNN training.
The non-empirical features incl. one-hot sequence / position encodings, base-pairing / RNA-protein interaction probabilities. The empirical-rules-based features incl. the thermodynamic stability profile, nucleotide frequencies, the G/C percentages, and the rule codes.

□ SharePro: an accurate and efficient genetic colocalization method accounting for multiple causal signals
>> https://academic.oup.com/bioinformatics/advance-article/doi/10.1093/bioinformatics/btae295/7660541
SharePro takes marginal associations (z-scores) from GWAS summary statistics and Linkage Disequilibrium information calculated from a reference panel as inputs and infers posterior probabilities of colocalization. SharePro adopts an effect group-level approach for colocalization.
SharePro uses a sparse projection shared across traits to group correlated variants into effect groups. Variant representations for effect groups are the same across traits so that colocalization probabilities can be directly calculated at the effect group level.

□ Cauchy hyper-graph Laplacian nonnegative matrix factorization for single-cell RNA-sequencing data analysis
>> https://bmcbioinformatics.biomedcentral.com/articles/10.1186/s12859-024-05797-4
Cauchy hyper-graph Laplacian non-negative matrix factorization (CHLNMF) replaces the Euclidean distance used in the original NMF model with CLF, which reduces the impact of noise and improves the stability of the model.
The CHLNMF techniques include regularisation terms for hyper-graphs to maintain the original data's manifold structure. The non-convex optimization issue is changed into an iterative weighted problem using the half-quadratic (HQ) optimization approach.

□ ChatNT: A Multimodal Conversational Agent for DNA, RNA and Protein Tasks
>> https://www.instadeep.com/wp-content/uploads/2024/04/ChatNT_A-Multimodal-Conversational-Agent-for-DNA-RNA-and-Protein-Tasks.pdf
ChatNT is the first framework for genomics instruction-tuning, extending instruction-tuning agents to the multimodal space of biology and biological sequences. ChatNT is designed to be modular and trainable end-to-end.
ChatNT combines a DNA encoder model, pre-trained on raw genome sequencing data and that provides DNA sequence representations. A projection layer maps DNA encoder outputs into the embedding space of English words, enabling use by the English decoder.

□ MOWGAN: Scalable Integration of Multiomic Single Cell Data Using Generative Adversarial Network
>> https://academic.oup.com/bioinformatics/advance-article/doi/10.1093/bioinformatics/btae300/7663468
MOWGAN, a deep learning framework for the generation of synthetic paired multiomics single-cell datasets. The core component is a single Wasserstein Generative Adversarial Network w/ gradient penalty (WGAN-GP). Inputs are data from multi-omics experiment in unpaired observation.
Once trained, the generative network is used to produce a new dataset where the observations are matched between all modalities. The synthetic dataset can be used for downstream analysis, first of all to bridge the original unpaired data.
MOWGAN learns the structure of single assays and infers the optimal couplings between pairs of assays. In doing so, MOWGAN generates synthetic multiomic datasets that can be used to transfer information among the measured assays by bridging.
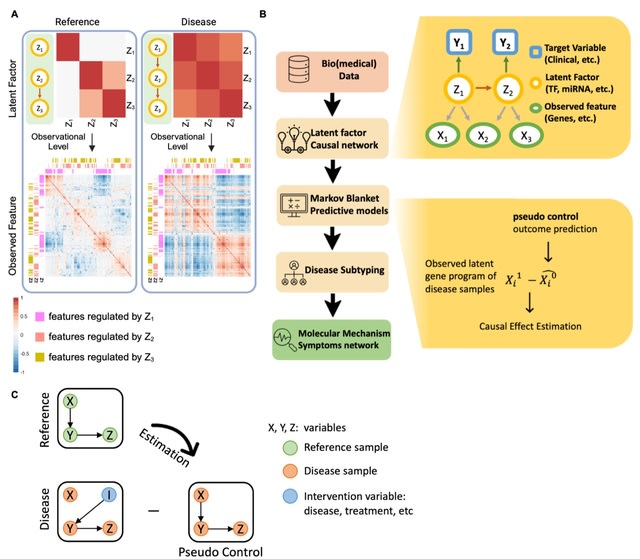
□ LaGrACE: Estimating gene program dysregulation using latent gene regulatory network for biomedical discovery
>> https://www.biorxiv.org/content/10.1101/2024.04.29.591756v1
LaGrACE (Latent Graph-based individuAl Causal Effect Estimation). LaGrACE is a novel approach designed to estimate regulatory network-based pseudo control outcome to characterize gene program dysregulation for samples within treatment (or disease) group.
They build a predictor of a gene program activity by using the variables in its Markov blanket. LaGrACE enables grouping of samples w/ similar patterns of gene program dysregulation, facilitating discovery of underlying molecular mechanisms induced by treatment or disease.
LaGrACE based on LOVE LF exhibited performance comparable to LaGrACE with ground truth latent factors. LaGrACE is robust for subtyping tasks in high-dimensional and collinear datasets.
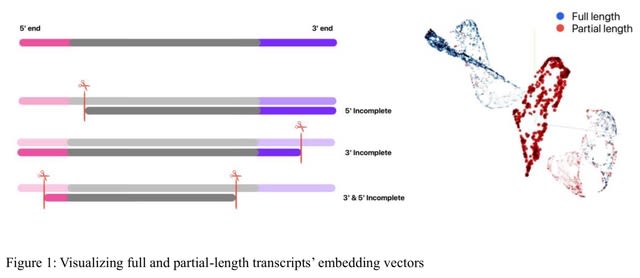
□ ntEmbd: Deep learning embedding for nucleotide sequences
>> https://www.biorxiv.org/content/10.1101/2024.04.30.591806v1
ntEmbd is a nucleotide sequence embedding method for latent representation of input nucleotide sequences. The model is built on a Bi-LSTM autoencoder to summarize data in a fixed-dimensional latent representation, capturing both local and long-range dependencies between features.
ntEmbd employs a 5-fold cross-validation approach where it initializes an Optuna study and records the best parameters for each fold. It aggregates the best hyperparameters across folds using voting strategy for categorical parameters and averaging for continuous parameters.

□ CRISPR-GPT: An LLM Agent for Automated Design of Gene-Editing Experiments
>> https://www.biorxiv.org/content/10.1101/2024.04.25.591003v1
CRISPR-GPT, an LLM agent augmented with domain knowledge and external tools to automate and enhance the design process of CRISPR-based gene-editing experiments.
CRISPR-GPT leverages the reasoning ability of LLMs to facilitate the process of selecting CRISPR systems, designing guide RNAs, recommending cellular delivery methods, drafting protocols, and designing validation experiments to confirm editing outcomes.

□ CopyVAE: a variational autoencoder-based approach for copy number variation inference using single-cell transcriptomics
>> https://academic.oup.com/bioinformatics/advance-article/doi/10.1093/bioinformatics/btae284/7658946
CopyVAE takes count matrix as input and is trained to learn latent representations for cells. Diploid cells are identified using k-means clustering and auto-correlation comparison.
The baseline expression levels are calculated from the expression profiles of identified diploid cells, and a pseudo copy matrix is generated for approximate copy number estimation.
Copy VAE takes pseudo copy matrix as input and is trained to refine copy number estimation, followed by a likelihood-based segmentation algorithm to integrate copy number profiles within aneuploid clones and call breakpoints individually for each clone.
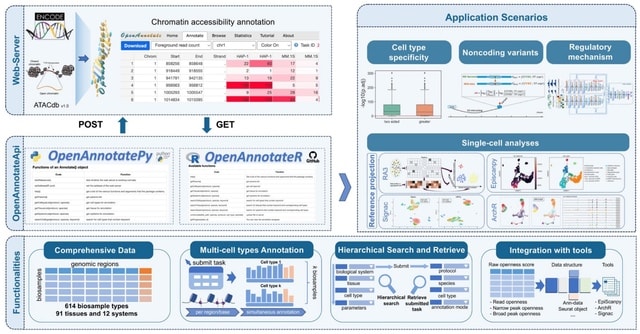
□ OpenAnnotateApi: Python and R packages to efficiently annotate and analyze chromatin accessibility of genomic regions
>> https://academic.oup.com/bioinformaticsadvances/article/4/1/vbae055/7643533
OpenAnnotateApi comprises two toolkits, the R version and Python version, operating together as the command-line iteration of OpenAnnotate, which efficiently annotates chromatin accessibility signals across diverse bio-sample types.
OpenAnnotateApi holds extensive applicability, particularly in single-cell data analysis. It can integrate openness scores from OpenAnnotateApi into models to predict and discover regulatory elements, and even construct regulatory networks.

□ Figeno: multi-region genomic figures with long-read support
>> https://www.biorxiv.org/content/10.1101/2024.04.22.590500v1
figeno, an application for generating publication-quality FIgures for GENOmics. Figeno particularly focuses on multi-region views across genomic breakpoints and on long reads with base modifications.
Figeno can plot one or multiple regions simultaneously. Although some tracks will be plotted independently for each region, other tracks can show interactions across regions; ATAC / ChIP-seq / HiC, as well as whole genome sequencing data with copy numbers and structural variants.

□ Imbalance and Composition Correction Ensemble Learning Framework (ICCELF): A novel framework for automated scRNA-seq cell type annotation
>> https://www.biorxiv.org/content/10.1101/2024.04.21.590442v1
Comprehensive benchmarking of classification algorithms identified XGBoost as the optimal classifier compatible with ICCELF. XGBoost significantly outperformed other methods like random forests, support vector machines, and neural networks on real PBMC datasets.
ICCELF generates layered synthetic training sets by combining real scRNA-seq data with oversampled minority classes. This structure is well-suited for XGBoost's boosting approach.

□ OrthoRefine: automated enhancement of prior ortholog identification via synteny
>> https://bmcbioinformatics.biomedcentral.com/articles/10.1186/s12859-024-05786-7
OrthoRefine automates the task of using synteny information to refine the HOGs identified by OrthoFinder into groups of syntenic orthologs, orthologs grouped based on evidence of synteny.
OrthoRefine requires only the output from OrthoFinder and genome annotations. OrthoRefine can refine the output of other programs that provide an initial clustering of homologous genes if the output is formatted to match OrthoFinder’s.

□ demuxSNP: supervised demultiplexing scRNAseq using cell hashing and SNPs
>> https://www.biorxiv.org/content/10.1101/2024.04.22.590526v1
demuxSNP is a performant demultiplexing approach that uses hashing and SNP data to demultiplex datasets with low hashing quality where biological samples are genetically distinct.
The genetic variants (SNPs) of the subset of cells assigned with high confidence using a probabilistic hashing algorithm are used to train a KNN classifier that predicts the demultiplexing classes of unassigned or uncertain cells.

□ GENA-Web - GENomic Annotations Web Inference using DNA language models
>> https://www.biorxiv.org/content/10.1101/2024.04.26.591391v1
GENA-Web, a web service for inferring sequence-based features using DNA transformer models. GENA-Web generates DNA annotations as specified by the user, offering outputs both as downloadable files and through an interactive genome browser display.
GENA-Web hosts models tailored for annotating promoters, splice sites, epigenetic features, and enhancer activities, as well as to highlight sequence determinants that underlying model prediction.

□ MooViE – Engine for single-view visual analysis of multivariate data
>> https://www.biorxiv.org/content/10.1101/2024.04.26.591357v1
MooViE is an easy-to-use tool to display multidimensional data with input-output semantics from all research domains. MooViE supports researcher in studying the mapping of several inputs to several outputs in large multivariate data sets.

□ MPH: fast REML for large-scale genome partitioning of quantitative genetic variation
>> https://academic.oup.com/bioinformatics/advance-article/doi/10.1093/bioinformatics/btae298/7660542
MPH (MINQUE for Partitioning Heritability) is designed for efficient genome partitioning analyses using restricted maximum likelihood. MPH integrates several algorithms to facilitate fast REML estimation of VCs.
First, the REML estimates are computed using Fisher's scoring method, and their corresponding analytical standard errors are derived from the Fisher information matrix. Second, the trust-region dogleg method is implemented to overcome possible convergence failures in REML resulting from non-positive definite.
MPH utilizes a stochastic trace estimator to accelerate trace term evaluations in REML, contrasting with direct computations conventionally employed by software like GCTA and LDAK.

□ VCF2PCACluster: a simple, fast and memory-efficient tool for principal component analysis of tens of millions of SNPs
>> https://bmcbioinformatics.biomedcentral.com/articles/10.1186/s12859-024-05770-1
VCF2PCACluster can easily calculate Kinship matrix and perform PCA and clustering analysis, and yield publication-ready 2D and 3D plots based on the variant call format (VCF) formatted SNP data in a fast and low-memory usage.
VCF2PCACluster enables users to perform analysis on a subset of samples defined in the VCF input using the (-InSubSample) parameter. It also enables comparisons between the prior sample group labels with the unsupervised clustering result through the (-InSampleGroup) parameter.

□ NPmatch: Latent Batch Effects Correction of Omics data by Nearest-Pair Matching
>> https://www.biorxiv.org/content/10.1101/2024.04.29.591524v1
NPmatch (Nearest-Pair Matching) relies on distance-based matching to deterministically search for nearest neighbors with opposite labels, so-called “nearest-pair”, among samples. NPmatch requires knowledge of the phenotypes but not of the batch assignment.
NPmatch does not rely on specific models or underlying distribution. NPmatch is based on the simple rationale that samples sharing a biological state (e.g., phenotype, condition) should empirically pair based on distance in biological profiles, such as transcriptomics profiles.

□ Partial Fitch Graphs: Characterization, Satisfiability and Complexity
>> https://www.biorxiv.org/content/10.1101/2024.04.30.591842v1
The characterization of partial Fitch graphin terms of Fitch-satisfiable tuples directly leads to the polynomial-time recognition algorithm. This algorithm yields a Fitch-cotree, which in turn defines a Fitch graph that contains the given partial Fitch graph as a subgraph.
The related Fitch completion problem, which in addition requires optimization of the score function, on the other hand is NP-hard. They provide a greedy-heuristic for "optimally" recovering Fitch graphs from partial ones.

□ Decipher: A computational pipeline to extract context-specific mechanistic insights from single-cell profiles
>> https://www.biorxiv.org/content/10.1101/2024.05.01.591681v1
Decipher is a modular pipeline that connects intercellular signalling between ligand/receptor pairs with downstream intracellular responses mediated by transcription factors and their target genes in a data-driven manner.
Decipher systematically integrates distinct layers of biological networks to tailor, enrich and extract mechanistic insights based on the context of interest. Decipher also produces global cell-to-cell signaling maps that are easy to interpret.

□ Cross-modality Matching and Prediction of Perturbation Responses with Labeled Gromov-Wasserstein Optimal Transport
>> https://arxiv.org/abs/2405.00838
Extending two Gromov-Wasserstein Optimal Transport methods to incorporate the perturbation label for cross-modality alignment. The alignment is employed to train a predictive model that estimates cellular responses to perturbations observed w/ only one measurement modality.
Conducting a nested 5-fold cross-validation by splitting treatments into a train, validation, and test sets. The best hyperparameters for prediction tasks were independently selected from the inner CV. They performed a Hyperparameter search for the entropic regularizer.

□ Locityper: targeted genotyping of complex polymorphic genes
>> https://www.biorxiv.org/content/10.1101/2024.05.03.592358v1
Locityper is a targeted genotyping tool designed for structurally-variable polymorphic loci. For every target region, Locityper finds a pair of haplotypes (locus genotype) that explain input whole genome sequencing (WGS) dataset in a most probable way.
Locus genotyping depends solely on the reference panel of haplotypes, which can be automatically extracted from a variant call set representing a pangenome (VCF format), or provided as an input set of sequences (FASTA format).
Before genotyping, Locityper efficiently preprocesses the WGS dataset and probabilistically describes read depth, insert size, and sequencing error profiles. Next, Locityper uses haplotype minimizers to quickly recruit reads to all target loci simultaneously.
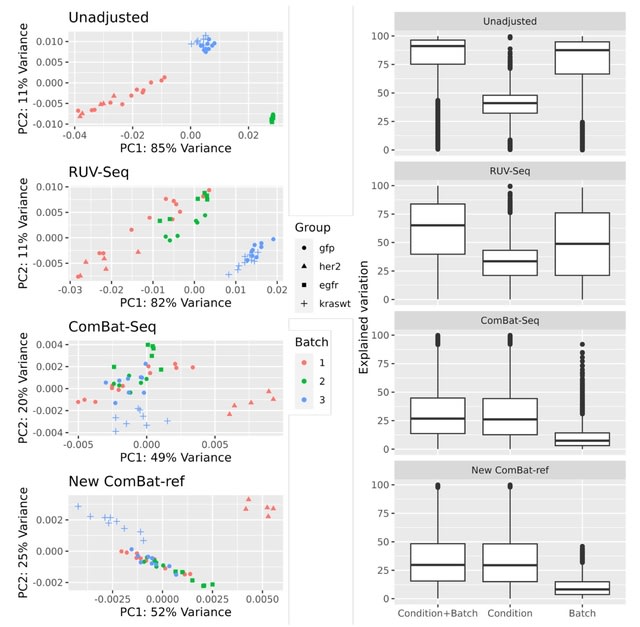
□ Highly Effective Batch Effect Correction Method for RNA-seq Count Data
>> https://www.biorxiv.org/content/10.1101/2024.05.02.592266v1
ComBat-ref, a modified version of the batch effect adjustment method, which models the RNA-seq count data using a negative binomial distribution similar to ComBat-seq, but with important changes in data adjustment.
ComBat-ref estimates a pooled (shrunk) dispersion for each batch and selects the batch with the minimum dispersion as the reference, to which the count data of other batches are adjusted.
※コメント投稿者のブログIDはブログ作成者のみに通知されます