
(Artwork by Joey Camacho)

□ HyperHMM: Efficient inference of evolutionary and progressive dynamics on hypercubic transition graphs
>> https://www.biorxiv.org/content/10.1101/2022.05.09.491130v1.full.pdf
Hypercubic transition path sampling (HyperTraPS) uses biased random walkers to estimate this likelihood, which is then embedded in a Bayesian framework using Markov chain Monte Carlo for parameter estimation.
HyperHMM, an adapted Baum-Welch (expectation maximisation) algorithm for inferring dynamic pathways on hypercubic transition graphs, and can be combined with resampling for quantify uncertainty.

□ Ultima Genomics RT
>> https://www.ultimagenomics.com
Ultima Genomicsが第三勢力となり得る、新しいシーケシング・プラットフォームを2023年にリリース。既にステルスで6億ドルを調達。蛍光フローベースに基づき1ドル/Gbのデータ生成を実現。Sentieon・DeepVariantとも提携、高精度のバリアントコールも実装する。
Today Ultima Genomics emerged from stealth mode with a new high-throughput, low-cost sequencing platform that delivers the $100 genome. Ultima’s goal is to unleash a new era in genomics-driven research and healthcare, and it has secured approximately $600 million in backing from leading investors who share this vision.
□ Joseph Replogle
$1/Gb? I had a great experience collaborating w/ Ultima genomics to sequence genome-scale Perturb-seq libraries on their new open fluidics sequencing platform: biorxiv.org/content/10.110… (see Figure S13 for comparison)
>> https://www.biorxiv.org/content/10.1101/2021.12.16.473013v3
□ Albert Viella
Cost-efficient whole genome-sequencing using novel mostly natural sequencing-by-synthesis chemistry and open fluidics platform biorxiv.org/content/10.110… #UltimaGenomics
>> https://www.biorxiv.org/content/10.1101/2022.05.29.493900v1

□ SUBATOMIC: a SUbgraph BAsed mulTi-OMIcs Clustering framework to analyze integrated multi-edge networks
>> https://www.biorxiv.org/content/10.1101/2022.06.01.494279v1.full.pdf
SUBATOMIC, a SUbgraph BAsed mulTi-Omics Clustering framework to construct and analyze multi-edge networks. SUBATOMIC investigates statistically the connections in between modules as well as between modules and regulators such as miRNAs and transcription factors.
SUBATOMIC integrates all networks into one multi-edge network and decomposes it into two- and three-node subgraphs using ISMAGS. The resulting subgraphs are further categorized according to their type and clustered into modules using the hyperedge clustering algorithm SCHype.

□ AEON: Exploring attractor bifurcations in Boolean networks
>> https://bmcbioinformatics.biomedcentral.com/articles/10.1186/s12859-022-04708-9
A a computational framework employing advanced symbolic graph algorithms that enable the analysis of large networks with hundreds of Boolean variables. A comprehensive methodology for automated attractor bifurcation analysis of parametrised BNs, fully implemented in AEON.
AEON computes the attractors for all valid parametrisations. AEON assigns each parametrisation its behaviour class. This bifurcation function can be displayed as a simple table which obtains witness instantiations for each behaviour class and inspect their attractor state space.

□ Eulertigs: minimum plain text representation of k-mer sets without repetitions in linear time
>> https://www.biorxiv.org/content/10.1101/2022.05.17.492399v1.full.pdf
A formalisation of arc-centric bidirected de Bruijn graphs and prove that it accurately models the k-mer spectrum. The algorithm constructs the de Bruijn graph in the length of the input strings. Then it uses a Eulerian-cycle-based algorithm to compute the minimum representation.
Computing a Hamiltonian cycle in a de Bruijn graph is polynomial. de Bruijn graphs are a subclass of adjoint graphs, in which solving the Hamiltonian cycle problem is equivalent to solving the Eulerian cycle problem in the adjoint graph, which can be computed in linear time.


□ N-ACT: An Interpretable Deep Learning Model for Automatic Cell Type and Salient Gene Identification
>> https://www.biorxiv.org/content/10.1101/2022.05.12.491682v1.full.pdf
N-ACT (Neural-Attention for Cell Type identification) accurately predicts preliminary annotations with no prior knowledge about the system, providing a valuable complementary framework to experimental studies and computational pipelines.
N-ACT learns complex mappings, outputs are non-linearly “activated” through a Point-Wise Feed Forward Neural Network. N-ACT consists of flexible stages that can be modified for different objectives. N-ACT minimizes a cross entropy loss using the Adam gradient-based optimizer.

□ CReSIL: Accurate Identification of Extrachromosomal Circular DNA from Long-read Sequences.
>> https://www.biorxiv.org/content/10.1101/2022.05.13.491700v1.full.pdf
CReSIL (Construction-based Rolling-circle amplification for eccDNA Sequence Identification and Location) constructed directed graphs with the information of regions, terminals, and strands; an individual region contained 4 nodes and multiple edges derived from linkages.
CReSIL relies on the reference genome read alignment result, enabling construction of linkages among regions. CReSIL generated consensus sequences and variants of eccDNA, and assess potential phenotypic effects of eccDNA when variations on the chromosomes are generated.

□ scMinerva: a GCN-featured Interpretable Framework for Single-cell Multi-omics Integration with Random Walk on Heterogeneous Graph
>> https://www.biorxiv.org/content/10.1101/2022.05.28.493838v1.full.pdf
scMinerva, an unsupervised Single-Cell Multi-omics INtegration method with GCN on hEterogeneous graph utilizing RandomWAlk, that can adapt to any number of omics with efficient computational consumption.
Considering the structure and biological insight of this multi-omics integration problem, to learn the cell property on top of multi-omics information and the cell neighbors, they accordingly design the model on a new random walk strategy.
scMinerva process any number of omics and has an explicit probabilistic interpretability, and a Graph Convolutional Network (GCN), which considers the spatial information of nodes and endows the method a strong robustness to noises.

□ scDeepC3: scRNA-seq Deep Clustering by A Skip AutoEncoder Network with Clustering Consistency
>> https://www.biorxiv.org/content/10.1101/2022.06.05.494891v1.full.pdf
scDeepC3, a novel deep clustering model containing an AutoEncoder with adaptive shortcut connection and using deep clustering loss with consistency constraint for clustering analysis of scRNA-seq data.
scDeepC3 can effective extract embedded representations, which is optimized for clustering, of the high-dimensional input through a nonlinear mapping. The optimal mapping function can be efficiently computed by the Hungarian algorithm.

□ MARGARET: Inference of cell state transitions and cell fate plasticity from single-cell
>> https://academic.oup.com/nar/advance-article/doi/10.1093/nar/gkac412/6593121
MARGARET employs a novel measure of connectivity to assess connectivity between the inferred clusters in the first step and constructs a cluster-level undirected graph to represent a trajectory topology.
MARGARET contructs a kNN graph between all cells and prunes it with reference to the undirected graph computed previously. MARGARET assigns a pseudotime to each cell in the pruned kNN graph denoting the position of this cell in the underlying trajectory.

□ RISER: real-time in silico enrichment of RNA species from nanopore signals
>> http://nanoporetech.com/resource-centre/video/lc22/riser-real-time-in-silico-enrichment-of-rna-species-from-nanopore-signals
RISER, the first method for realtime in silico enrichment of RNA species during direct RNA sequencing (DRS). RISER accurately classifies protein-coding from non-coding species directly from four seconds of raw DRS signal.
RISER has been integrated with the Read Until API to enact real-time sequencing decisions that allow enrichment of mRNAs or non-coding RNAs, as well as real-time tagging of reads with RNA species.

□ Last-train: Finding rearrangements in nanopore DNA reads with LAST and dnarrange
>> https://www.biorxiv.org/content/10.1101/2022.05.30.494079v1.full.pdf
The LAST and dnarrange software packages can resolve complex re- lationships between DNA sequences, and characterize changes such as gene conversion, processed pseudogene insertion, and chromosome shattering.
Last-train learns the rates (probabilities) of deletions, insertions, and each kind of base match and mismatch. These probabilities are then used to find the most likely sequence relationships/alignments, which is especially useful for DNA with unusual rates.

□ inClust: a general framework for clustering that integrates data from multiple sources
>> https://www.biorxiv.org/content/10.1101/2022.05.27.493706v1.full.pdf
inClust provides a general and flexible framework, which can be applied to various tasks with different modes. inClust perform information integration and clustering jointly, meanwhile it could utilize the labeling information from data as regulation information.
inClust encode scRNA-seq data and batch information (or other covariates and auxiliary information) into latent space, respectively. So, the influence of the batch and other covariates is explicitly eliminated by vector arithmetic in latent space.

□ PEPSDI: Scalable and flexible inference framework for stochastic dynamic single-cell models
>> https://journals.plos.org/ploscompbiol/article?id=10.1371/journal.pcbi.1010082
PEPSDI (Particles Engine for Population Stochastic DynamIcs), a flexible modelling framework which infers unknown model parameters from dynamic data for single-cell dynamic models that account for both intrinsic and extrinsic noise.
For the Ornstein-Uhlenbeck stochastic differential equation model, the likelihood approximation has a small variance and exact Bayesian inference is possible because the likelihood can be exactly calculated using the Kalman filter.
PEPSDI modularity facilitates modelling of intrinsic noise by the SSA, Extrande, tau-leaping or Langevin stochastic simulators. New particle filters for the pseudo-marginal modules can be added. Like the one used for the Schlögl model, are particularly statistically efficient.

□ NanoSplicer: Accurate identification of splice junctions using Oxford Nanopore sequencing
>> https://academic.oup.com/bioinformatics/advance-article/doi/10.1093/bioinformatics/btac359/6594111
NanoSplicer utilises the raw ouput from nanopore sequencing. Instead of identifying splice junctions by mapping basecalled reads, nanosplicer compares the squiggle from a read with the predicted squiggles of potential splice junctions to identify the best match and likely junction.
NanoSplicer adapts Dynamic Time Warping to align the two squiggles. NanoSplicer identifies all possible canonical splice junctions within 10 bases. The NanoSplicer model provides assignment probabilities for each candidate by quantifying the squiggle similarity of each alignment.

□ scMoMaT: Mosaic integration of single cell multi-omics matrices using matrix trifactorization
>> https://www.biorxiv.org/content/10.1101/2022.05.17.492336v1.full.pdf
scMoMaT (single cell Multi-omics integration using Matrix Trifactorization) makes it possible to uncover the cell type specific bio-markers at the same time when learning a unified cell representation. Moreover, scMoMaT can integrate cell batches with unequal cell type composition.
scMoMaT uses a matrix tri-factorization framework, which treats each single cell data matrix as a relationship matrix between the cell and feature entity. It factorizes a data matrix into batch-specific cell factor, feature factor, and a factor association matrix.

□ sshash: On Weighted K-Mer Dictionaries
>> https://www.biorxiv.org/content/10.1101/2022.05.23.493024v1.full.pdf
SSHash, a sparse and skew hashing scheme for k-mers – a compressed dictionary that relies on k-mer minimizers and minimal perfect hashing in both random and streaming query modality in succinct space.
Enriching the SSHash data structure with the weight information. by exploiting the order of the k-mers represented in SSHash, the compressed exact weights take only a small extra space on top of the space of SSHash.
This extra space is proportional to the number of runs (maximal sub-sequences formed by all equal symbols) in the weights and not proportional to the number of distinct k-mers. The weights are represented in a much smaller space than the empirical entropy lower bound.

□ Lossless indexing with counting de Bruijn graphs
>> https://genome.cshlp.org/content/early/2022/05/23/gr.276607.122.abstract
Counting de Bruijn graphs (Counting DBGs), a notion generalizing annotated de Bruijn graphs by supplementing each node-label relation with one or many attributes.
Counting DBGs index k-mer abundances from 2,652 human RNA-seq samples in over 8-fold smaller and yet faster. The full RefSeq collection, Counting DBGs generates a lossless and fully queryable index that is 4.6-fold smaller than the corresponding MegaBLAST index.
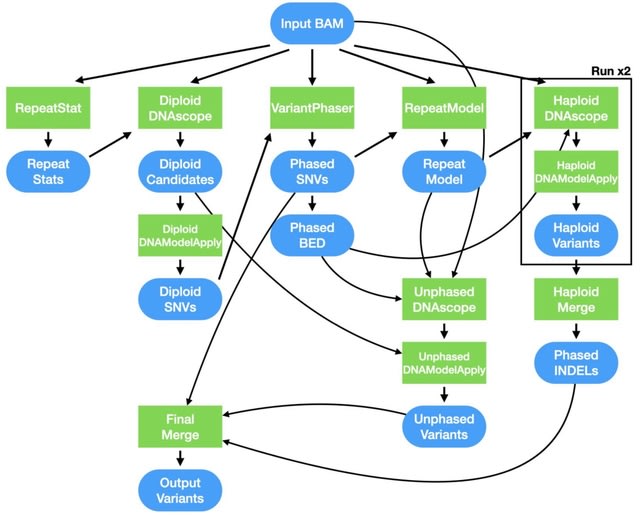
□ Sentieon DNAscope LongRead - A highly Accurate, Fast, and Efficient Pipeline for Germline Variant Calling from PacBio HiFi reads
>> https://www.biorxiv.org/content/10.1101/2022.06.01.494452v1.full.pdf
The core variant calling pipeline calls DNAscope across the phased or unphased regions of the genome and uses DNAModelApply to perform model-informed variant genotyping. Small Python scripts are used for VCF manipulation.
DNAscope LongRead is computationally efficient, calling variants from 30x HiFi samples in under 4 hours on a 16-core machine (120 virtual core-hours) with precision and recall on the most recent GIAB benchmark dataset exceeding 99.83% for HiFi samples sequenced at 30x coverage.

□ DSINMF: Detecting cell type from single cell RNA sequencing based on deep bi-stochastic graph regularized matrix factorization
>> https://www.biorxiv.org/content/10.1101/2022.05.16.492212v1.full.pdf
Sparsity is a significant characteristics of single cell data, in other word, scRNA-seq data have a large number of zero entries. It also restricted the application of cluster method in single-cell data analysis.
DSINMF reduces redundant features. The structure of multi-layer matrix factorization is utilized to extract the deep hidden features which can obtain the features in different layers. The deep matrix factorization with bi-stochastic graph regularization is employed to clustering.
<be />

□ DeepPHiC: Predicting promoter-centered chromatin interactions using a novel deep learning approach
>> https://www.biorxiv.org/content/10.1101/2022.05.24.493333v1.full.pdf
DeepPHiC, a supervised multi-modal deep learning model, which utilizes a comprehensive set of features including genomic sequence, epigenetic signals and anchor distance to predict tissue/cell type-specific genome-wide promoter-enhancer and promoter-promoter interactions.
DeepPHiC utilizes a comprehensive set of informative features, ranging from genomic sequence, epigenetic signal in the anchors and anchor distance. DeepPHiC adopts a ResNet-style structure with skip connections, wherein previous layers are connected to all subsequent layers.

□ Sequence UNET: High-throughput deep learning variant effect prediction
>> https://www.biorxiv.org/content/10.1101/2022.05.23.493038v1.full.pdf
Sequence UNET, a highly scalable variant effect predictors (VEP) that uses a fully Convolutional Neural Network architecture to achieve computational efficiency and independence from length. Convolutional kernels also naturally integrate information from nearby amino acids.
Sequence UNET optimises performance for position specific scoring matrix (PSSM) prediction using a softmax output layer and Kullbeck-Leibler divergence loss and variant frequency classification using a sigmoid output and binary cross entropy.

□ CSCD: More accurate estimation of cell composition in bulk expression through robust integration of single-cell information
>> https://www.biorxiv.org/content/10.1101/2022.05.13.491858v1.full.pdf
Many computational tools have been developed and reported in the literature. However, they fail to appropriately incorporate the covariance structures in both scRNA-seq and bulk RNA-seq datasets in use.
CSCD, a covariance-based single-cell decomposition that estimates cell-type proportions in bulk data through building a reference expression profile based on a single-cell data, and learning gene-specific bulk expression transformations using a constrained linear inverse model.

□ isopret: An algorithmic framework for isoform-specific functional analysis
>> https://www.biorxiv.org/content/10.1101/2022.05.13.491897v1.full.pdf
isopret, a new paradigm for isoform function prediction based on the expectation-maximization framework. isopret leverages the relationships between sequence and functional isoform similarity to infer isoform specific functions in a highly accurate fashion.
isopret predicts isoform annotations w/o using isoform-specific labels, learns directly from isoform sequences w/o using gene elements, and assigns GO to isoforms through a global optimization algorithm, thus avoiding inconsistencies due to local isoform-by-isoform predictions.

□ MAGCNSE: predicting lncRNA-disease associations using multi-view attention graph convolutional network and stacking ensemble model
>> https://bmcbioinformatics.biomedcentral.com/articles/10.1186/s12859-022-04715-w
MAGCNSE uses disease semantic similarity (DSS) and disease Gaussian interaction profile kernel similarity (DGS). And for views of lncRNAs, MAGCNSE uses lncRNA functional similarity, lncRNA sequence similarity and lncRNA Gaussian interaction profile kernel similarity.
MAGCNSE then concatenates the representations of lncRNAs and diseases according to the lncRNA-disease association matrix. MAGCNSE employs a stacking ensemble classifier, consisting of multiple traditional machine learning classifiers, to make the final prediction.

□ Bioteque Integrating and formatting biomedical data in the Bioteque, a comprehensive repository of pre-calculated knowledge graph embeddings
>> https://www.biorxiv.org/content/10.1101/2022.05.11.491490v1.full.pdf
Bioteque, a resource of unprecedented size and scope that contains pre-calculated biomedical embeddings derived from a gigantic knowledge graph, displaying more than 450 thousand biological entities and 30 million relationships.
Bioteque descriptors can be easily recycled as node features, transferring the learning encoded from orthogonal biomedical datasets to more complex, attribute-aware models. The Bioteque provides information on the specific sources used to construct each metapath.

□ OMEN: Network-based Driver Gene Identification using Mutual Exclusivity
>> https://academic.oup.com/bioinformatics/advance-article-abstract/doi/10.1093/bioinformatics/btac312/6585332
Propagation-based methods in contrast allow recovering rare driver genes, but the interplay between network topology and high-scoring nodes often results in spurious predictions.
OMEN is a logic programming framework based on random walk semantics. OMEN presents a number of novel concepts. In particular, its design is unique in that it presents an effective approach to combine both gene-specific driver properties and gene-set properties.

□ FastIntegration: a fast and high-capacity version of Seurat Integration for large-scale integration of single-cell data
>> https://www.biorxiv.org/content/10.1101/2022.05.10.491296v1.full.pdf
FastIntegration can integrate large single-cell RNA-seq datasets and outputting batch corrected gene expression. Its capacity for large scale batch integration with 4 million cells in 48 hours runtime through good multicore scaling.
Seurat computes a fixed number of kNN to construct the weight matrix of anchor while FastIntegration fits a Gaussian distribution. FastIntgeration removes outlier GE values and keep the sparsity of data, avoiding problem of long vector being unsupported in large sparse matrices.

□ DeSP: a systematic DNA storage error simulation pipeline
>> https://bmcbioinformatics.biomedcentral.com/articles/10.1186/s12859-022-04723-w
DeSP, a systematic DNA storage error Simulation Pipeline, which simulates the errors generated from all DNA storage stages and systematically guides the optimization of encoding redundancy.
DeSP covers both the sequence lost and the within-sequence errors in the particular context of the data storage channel. A systematic model is desired which covers all the key stages of the storage process to reveal how errors are generated / propagated to form final sequencing.

□ INSISTC: Incorporating Network Structure Information for Single-Cell Type Classification
>> https://www.biorxiv.org/content/10.1101/2022.05.17.492304v1.full.pdf
INSISTC utilizes the SIOMICS approach to generate a GRN with its TF-target relationships identified through de novo DNA regulatory motif discovery. SIOMICS is capable of considering both TFs and their cofactors for motif prediction.
INSISTC adopts a random-walk-based graph algorithm to represent the GRN structural information. INSISTC incorporates genes and GRN structural information by creating a Latent Dirichlet Allocation (LDA)-based topic model.
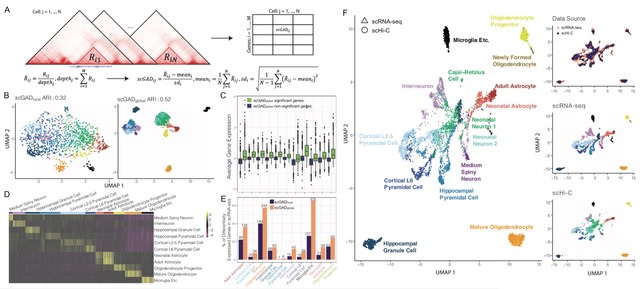
□ scGAD: single-cell gene associating domain scores for exploratory analysis of scHi-C data
>> https://academic.oup.com/bioinformatics/advance-article-abstract/doi/10.1093/bioinformatics/btac372/6598798
scGAD enables summarization at the gene unit while accounting for inherent gene-level genomic biases. Low-dimensional projections with scGAD capture clustering of cells based on their 3D structures.
scGAD facilitates the integration of scHi-C data with other single-cell data modalities by enabling its projection onto reference low-dimensional embeddings. scGAD facilitated an accurate projection of cells onto this larger space.

□ Quantization of algebraic invariants through Topological Quantum Field Theories
>> https://arxiv.org/pdf/2206.00709v1.pdf
Providing necessary conditions for quantizability based on Euler characteristics and, in the case of surfaces, also sufficient conditions in terms of almost-TQFTs and almost-Frobenius algebras.
The E-polynomial of G-representation varieties is not a quantizable invariant by means of a monoidal TQFTs, for any algebraic group G of positive dimension.