
(Created with Midjourney V6 ALPHA)
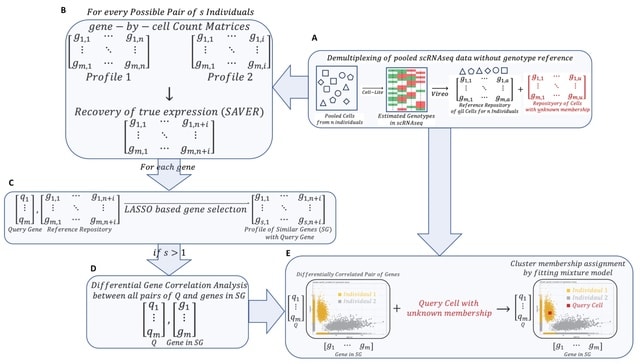
□ scDIV: Demultiplexing of Single-Cell RNA sequencing data using interindividual variation in gene expression
>> https://academic.oup.com/bioinformaticsadvances/advance-article/doi/10.1093/bioadv/vbae085/7690196
Interindividual differential co-expression genes provide a distinct cluster of cells per individual and display the enrichment of cellular macromolecular super-complexes.
scDIV (Single Cell RNA Sequencing Data Demultiplexing using Inter-individual Variations) uses Vireo (Variational Inference for Reconstructing Ensemble Origin) for donor deconvolution using expressed SNPs in multiplexed scRNA-seq data.
scDIV generates gene-cell count matrix using the 10X cellranger. The scDIV function uses SAVER (single-cell analysis via expression recovery), an expression recovery method for Unique Molecule Index based scRNA-seq data to provide accurate expression estimates for all genes.

□ SpaCEX: Learning context-aware, distributed gene representations in spatial transcriptomics
>> https://www.biorxiv.org/content/10.1101/2024.06.07.598026v1
SpaCEX (context-aware, self-supervised learning on Spatially Co-EXpressed genes) features in utilizing spatial genomic context inherent in ST data to generate gene embeddings that accurately represent the condition-specific spatial functional and relational semantics of genes.
SpaCEX treats gene spatial expressions (SEs) as images and lever-ages a masked-image model (MIM), which excels in extracting local-context perceptible and holistic visual features, to yield initial gene embeddings.
These embeddings are iteratively refined through a self-paced pretext task aimed at discerning genomic contexts by contrastingSE patterns among genes, drawing genes with similar SEs closer in the latent embedding space, while distancing those with divergent patterns.

□ CPMI: comprehensive neighborhood-based perturbed mutual information for identifying critical states of complex biological processes
>> https://bmcbioinformatics.biomedcentral.com/articles/10.1186/s12859-024-05836-0
CPMI, a novel computational method based on the neighborhood gene correlation network, to detect the tipping point or critical state during a complex biological process.
A CPMI network is constructed at each time point through the computation of a modified version of the Mahalanobis distance between gene pairs. Next, the nearest neighbor genes of the central gene in the local network are selected based on the top genes in terms of distance.
Subsequently, based on reference samples, case samples are separately introduced at each time point, and the perturbed neighbourhood mutual information for the combined samples is calculated, providing insights into changes for each gene at each moment.

□ Space Omics and Medical Atlas (SOMA) across orbits
>> https://www.nature.com/immersive/d42859-024-00009-8/index.html
The SOMA package represents a milestone in several other respects. It features a over 10-fold increase in the number of next-generation sequencing (NGS) data from spaceflight, a 4-fold increase in the number of single-cells processed from spaceflight.
Launching the first aerospace medicine biobank, the first-ever direct RNA sequencing data from astronauts, the largest number of processed biological samples from a mission, and the first ever spatially-resolved transcriptome data from astronauts.

□ Fundamental Constraints to the Logic of Living Systems
>> https://www.preprints.org/manuscript/202406.0891/v1
The space of possible proteins with a length of 1000 amino acids is 20^1000, a space so large that it could never be explored in our universe. The space of possible molecular configurations of molecules within an organism is yet astronomically larger.
Considering the thermodynamic properties of living systems, the linear nature of molecular information / building blocks of life / multicellularity and development / threshold nature of computations in cognitive systems, and the discrete nature of the architecture of ecosystems.

□ COSMIC: Molecular Conformation Space Modeling in Internal Coordinates with an Adversarial Framework
>> https://pubs.acs.org/doi/10.1021/acs.jcim.3c00989
COSMIC, a novel generative adversarial framework COSMIC for roto-translation invariant conformation space modeling. The proposed approach benefits from combining internal coordinates and a fast iterative refinement on pairwise distances.
COSMIC combines two adversarial models, the WGAN-GP and the AAE, which share a generator/decoder part. They also introduce a fast energy-based metric RED that exposes the physical plausibility of generated conformations by accounting for conformation energy.

□ ZX-calculus is Complete for Finite-Dimensional Hilbert Spaces
>> https://arxiv.org/pdf/2405.10896
The ZX-calculus is a graphical language for reasoning about quantum computing and quantum information theory. ZXW- and ZW-calculus enable complete reasoning for both qudits and finite-dimensional Hilbert spaces.
The finite-dimensional ZX-calculus generalizes the qudit ZX-calculus by introducing a mixed-dimensional Z-spider. The completeness of this generalization can be proved by translating to the complete finite-dimensional ZW-calculus, and showing that this translation is invertible.

□ Leaf: an ultrafast filter for population-scale long-read SV detection
>> https://genomebiology.biomedcentral.com/articles/10.1186/s13059-024-03297-5
Leaf (LinEAr Filter) employs a canonical binning module for quickly clustering patterns in long reads. It takes long reads as input and outputs clustered anchors of matched patterns. Additionally, Leaf consists of an adversarial autoencoder (AAE) for screening discordant anchors.
Leaf uses the generative model to generate the most likely assembly of fragments from which the given read is sequenced. The core idea is to use likelihood functions instead of score functions to compute the optimal assembly of fragments.

□ Chimera: Effectively Modeling Multivariate Time Series with 2-Dimensional State Space Models
>> https://arxiv.org/pdf/2406.04320
Chimera, an expressive variation of the 2-dimensional SSMs with careful design of parameters to maintain high expressive power while keeping the training complexity linear.
Using two SSM heads with different discretization processes and input-dependent parameters, Chimera is provably able to learn long-term progression, seasonal patterns, and desirable dynamic autoregressive processes.

□ PRESENT: Cross-modality representation and multi-sample integration of spatially resolved omics data
>> https://www.biorxiv.org/content/10.1101/2024.06.10.598155v1
PRESENT can simultaneously capture spatial dependency and complementary multi-omics information, obtaining interpretable cross-modality representations for various downstream analyses, particularly the spatial do main identification.
PRESENT also offers the potential to incorporate various reference data to address issues related to the low sequencing depth and signal-to-noise ratio in spatial omics data.
PRESENT is built on a multi-view autoencoder and extracts spatially coherent biological variations contained in each omics layer via an omics-specific encoder consisting of a graph attention neural network (GAT) and a Bayesian neural network.
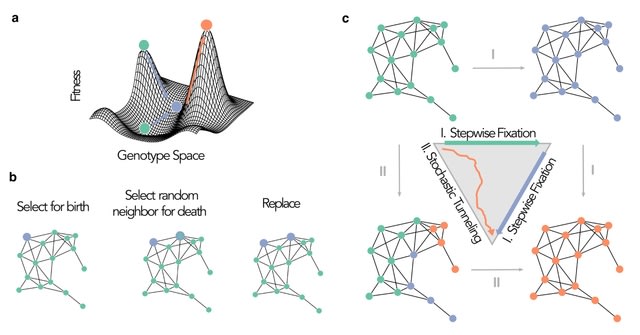
□ Evolutionary graph theory beyond single mutation dynamics: on how network-structured populations cross fitness landscapes
>> https://academic.oup.com/genetics/article/227/2/iyae055/7651240
The role of network topologies in shaping multi-mutational dynamics and probabilities of fitness valley crossing and stochastic tunneling.
The total probability of crossing the fitness landscape is the sum of the probabilities of acquiring the second mutation under the two independent evolutionary processes.
When the first mutant is strongly deleterious, the population depends on the second mutation appearing in time to cross the fitness landscape and the acceleration factor of the network changes the rate of fitness valley crossing by a factor of λ^-1.

□ Analysis-ready VCF at Biobank scale using Zarr
>> https://www.biorxiv.org/content/10.1101/2024.06.11.598241v1
VCF is at its core an encoding of the genotype matrix, where each entry describes the observed genotypes for a given sample at a given variant site, interleaved with per-variant information and other call-level matrices.
The data is largely numerical and of fixed dimension, and is therefore a natural mapping to array-oriented or "tensor" storage. The VCF Zarr specification maps the VCF data model into an array-oriented layout using Zarr. Each field in a VCF is mapped to a separately-stored array, allowing for efficient retrieval and high levels of compression.

□ Panacus: fast and exact pangenome growth and core size estimation
>> https://www.biorxiv.org/content/10.1101/2024.06.11.598418v1
Panacus (pangenome-abacus), a tool designed for rapid extraction of information from pangenomes represented as pangenome graphs in the Graphical Fragment Assembly (GFA) format.
Panacus not only efficiently generates pangenome growth and core curves but also provides estimates of the pangenome's expansion. Since a path can represent multiple types of sequence, a contig or even an entire chromosome, Panacus offers the option to group paths together.
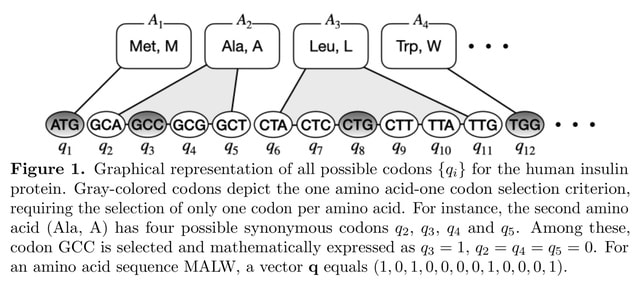
□ Quantum-classical hybrid approach for codon optimization and its practical applications
>> https://www.biorxiv.org/content/10.1101/2024.06.08.598046v1
An advanced protocol based on a quantum classical hybrid approach, integrating quantum annealing with the Lagrange multiplier method, to solve practical-size codon optimization problems formulated as constrained quadratic-binary problems.
This protocol converts each amino acid from the protein sequence into a set of binary variables representing all possible synonymous codons of the amino acid.

□ VILOCA: Sequencing quality-aware haplotype reconstruction and mutation calling for short- and long-read data
>> https://www.biorxiv.org/content/10.1101/2024.06.06.597712v1
VILOCA (VIral LOcal haplotype reconstruction and mutation CAlling for short and long read data), a statistical model and computational tool for single-nucleotide variant calling and local haplotype reconstruction from both short-read and long-read data.
VILOCA employs a finite Dirichlet Process mixture model that clusters reads according to their unobserved haplotypes. Reads are assigned to the most suitable haplotype using a sequencing error process that takes into account the sequencing quality scores specific to each read.

□ Exon Nomenclature and Classification of Transcripts (ENACT): Systematic framework to annotate exon attributes
>> https://www.biorxiv.org/content/10.1101/2024.06.07.597685v1
ENACT (Exon Nomenclature and Annotation of Transcripts) centralizes exonic loci while integrating protein sequence per entity with tracking and assessing splice site variability. ENACT enables exon features to be tractable, facilitating a systematic analysis of isoform diversity.
These include splice site variations, coding/noncoding exon property, and their combinations with exonic loci incorporated through genomic and coding genomic coordinates.
ENACT provides ways to assess proteome impact of exon variations (including indels) from transcriptomic and translational processes, especially inadequacies promulgated by AS, ATRI/ATRT, and ATLI/ATLT.

□ nipalsMCIA: Flexible Multi-Block Dimensionality Reduction in R via Nonlinear Iterative Partial Least Squares
>> https://www.biorxiv.org/content/10.1101/2024.06.07.597819v1
nipalsMCIA uses an extension with proof of monotonic convergence of Non-linear Iterative Partial Least Squares (NIPALS) to solve the Multiple co-inertia analysis (MCIA) optimization problem. This implementation shows significant speed-up over existing SVD-based approaches.
nipalsMCIA removes the dependence on an eigendecomposition for calculating the variance explained. nipalsMCIA offers users several options for pre-processing and deflation to customize algorithm performance, methodology to perform out-of-sample global embedding.

□ pyRforest: A comprehensive R package for genomic data analysis featuring scikit-learn Random Forests in R
>> https://www.biorxiv.org/content/10.1101/2024.06.09.598161v1
pyRforest, an R package that integrates the scikit-learn RandomForestClassifier algorithm. pyRforest enables users familiar with R to leverage the machine learning strengths of Python without requiring any Python coding knowledge.
pyRforest offers several innovative features, including a novel rank-based permutation method for identifying significantly important features, which estimates and visualizes p-values for individual features.
pyRforest includes methods for calculating and visualizing SHapley ADditive Explanations (SHAP) values while supporting comprehensive downstream analysis for gene ontology and pathway enrichment with cluster Profiler and g: Profiler.

□ D’or: Deep orienter of protein-protein interaction networks
>> https://academic.oup.com/bioinformatics/advance-article/doi/10.1093/bioinformatics/btae355/7691287
D'or uses sets (or distributions) of proximity scores from available cause-effect pairs as input to a deep learning encoder, which is trained in a supervised fashion to generate features for orientation prediction.
A key novelty of D'or is its ability to learn a general function of proximity scores rather than using arbitrary measures such as a sum, used by D2D to aggregate node scores, or a ratio, used by D2D to contrast causes with effects.


□ Omnideconv: Benchmarking second-generation methods for cell-type deconvolution of transcriptomic data
>> https://www.biorxiv.org/content/10.1101/2024.06.10.598226v1
Omnideconv offers five tools: the R package omnideconv providing a unified interface to deconvolution methods, the pseudo-bulk simulation method SimBu, the deconvData data repository, the deconvBench benchmarking pipeline in Nextflow and the web-app deconvExplorer.
For signature-based methods, some determinants of deconvolution performance can be investigated in the characteristics of the derived signature matrix. As the deconvolution step was fast for most methods, reusing signatures can speed up deconvolution of similar bulk datasets.

□ Ragas: integration and enhanced visualization for single cell subcluster analysis
>> https://academic.oup.com/bioinformatics/advance-article/doi/10.1093/bioinformatics/btae366/7691991
Ragas, an R package that integrates multi-level subclustering objects for streamlined analysis and visualization. A new data structure was implemented to seamlessly connect and assemble miscellaneous single cell analyses from different levels of subclustering.
A re-projection algorithm was developed to integrate nearest-neighbor graphs from multiple subclusters in order to maximize their separability on the combined cell embeddings, which significantly improved the presentation of rare and homogeneous subpopulations.

□ CAraCAl: CAMML with the integration of chromatin accessibility
>> https://bmcbioinformatics.biomedcentral.com/articles/10.1186/s12859-024-05833-3
The CAMML (Cell typing using variance Adjusted Mahalanobis distances with Multi-Labeling) method was developed as a cell typing technique for scRNA-seq data that leverages the single-cell gene set enrichment analysis method Variance Adjusted Mahalanobis (VAM).
CAraCAl performs cell typing by scoring each cell for its enrichment of cell type-specific gene sets. These gene sets are composed of the most upregulated or downregulated genes present in each cell type according to projected gene activity.

□ PyMulSim: a method for computing node similarities between multilayer networks via graph isomorphism networks
>> https://bmcbioinformatics.biomedcentral.com/articles/10.1186/s12859-024-05830-6
pyMulSim uses a Graph Isomorphism Network (GIN) for the representative learning of node features, that uses for processing the embeddings and computing the similarities between the pairs of nodes of different multilayer networks.
The key-issue addressed in pyMulSim concerns how much each node in a source multilayer network is similar to a node of a target one, maintaining the layered structure in which these may coexist.
Layers are the fundamental components that perform information propagation and transformation, and the GIN class combines these layers to create a complete neural network.

□ The Comparative Genome Dashboard
>> https://www.biorxiv.org/content/10.1101/2024.06.11.598546v1
The Comparative Genome Dashboard is a component of the Pathway Tools software. Pathway Tools powers the BioCyc website and is used to construct the organism-specific databases, called Pathway/Genome Databases (PGDBs), that make up the BioCyc database collection.
Users can interactively drill down to focus on subsystems of interest and see grids of compounds produced or consumed by each organism, specific GO term assignments, pathway diagrams, and links to more detailed comparison pages.
For example, the dashboard enables users to compare the cofactors that a set of organisms can synthesize, the metal ions that they are able to transport, their DNA damage repair capabilities.

□ Dyport: dynamic importance-based biomedical hypothesis generation benchmarking technique
>> https://bmcbioinformatics.biomedcentral.com/articles/10.1186/s12859-024-05812-8
Dyport is a novel benchmarking framework for evaluating biomedical hypothesis generation systems. Utilizing curated datasets, this approach tests these systems under realistic conditions, enhancing the relevance of the evaluations.
Dyport integrates knowledge from the curated databases into a dynamic graph, accompanied by a method to quantify discovery importance. Applicability of Dyport benchmarking process is demonstrated on several link prediction systems applied on biomedical semantic knowledge graphs.

□ SeqCAT: Sequence Conversion and Analysis Toolbox
>> https://academic.oup.com/nar/advance-article/doi/10.1093/nar/gkae422/7683049
SeqCAT provides 14 distinct functionalities and 3 info points. SeqCAT offers a variety of information endpoints from other resources, including amino acid structure and biochemical properties, reverse complementary transcripts, and pathway visualization.
Notable examples are 'Convert Protein to DNA Position' for translation of amino acid changes into genomic single nucleotide variants, or 'Fusion Check' for frameshift determination in gene fusions.

□ LRTK: a platform agnostic toolkit for linked-read analysis of both human genome and metagenome
>> https://academic.oup.com/gigascience/article/doi/10.1093/gigascience/giae028/7692299
Linked-Read ToolKit (LRTK), a unified and versatile toolkit for platform agnostic processing of linked-read sequencing data from both human genome and metagenome.
LRTK provides functions to perform linked-read simulation, barcode-aware read alignment and metagenome assembly, reconstruction of long DNA fragments, taxonomic classification and quantification, and barcode-assisted genomic variant calling and phasing.

□ GenoFig: a user-friendly application for the visualisation and comparison of genomic regions
>> https://academic.oup.com/bioinformatics/advance-article/doi/10.1093/bioinformatics/btae372/7693070
GenoFig allows the personalized representation of annotations extracted from GenBank files in a consistent way across sequences, using regular expressions. It also provides several unique options to optimize the display of homologous regions between sequences.
In GenoFig, annotated features can be drawn in a variety of styles defined by the user. Global specifications can be applied to each feature type (CDS, tRNA, mobile element), but a key component of GenoFig is to propose feature-specific configurations using word-matching queries.

□ isoLASER: Long-read RNA-seq demarcates cis- and trans-directed alternative RNA splicing
>> https://www.biorxiv.org/content/10.1101/2024.06.14.599101v1
isoLASER, enables a clear segregation of cis- and trans-directed splicing events for individual samples. The genetic linkage of splicing is largely individual-specific, in stark contrast to the tissue-specific pattern of splicing profiles.
isoLASER successfully uncovers cis-directed splicing in the highly polymorphic HLA system, which is difficult to achieve with short-read sequencing data.
isoLASER conducts variant calling using the long-read RNA-seq data. It uses a local reassembly approach based on de Bruin graphs to identify nucleotide variation at the read level, followed by a multi-layer perceptron classifier to discard false positives.
isoLASER carries out gene-level phasing to identify haplotypes. isoLASER employs an approach based on k-means read clustering, using the variant alleles as values and weighted by the variant quality score. It simultaneously phases the variants into their corresponding haplotypes.

□ splitcode: Flexible parsing, interpretation, and editing of technical sequences
>> https://academic.oup.com/bioinformatics/advance-article/doi/10.1093/bioinformatics/btae331/7693695
splitcode is a flexible solution with a low memory and computational footprint that can reliably, efficiently, and error-tolerantly preprocess technical sequences based on a user-supplied structure of how those sequences are organized within reads.
splitcode simultaneously trims technical sequences, parse combinatorial barcodes that are variable in length and inconsistent in location w/in a read, and extract UMIs that are defined in location w/ respect to other technical sequences rather than at a set position w/in a read.

□ TADGATE: Uncovering topologically associating domains from three-dimensional genome maps
>> https://www.biorxiv.org/content/10.1101/2024.06.12.598668v1
TADGATE employs a graph attention auto-encoder to accurately identify TADs even from ultra-sparse contact maps and generate the imputed maps while preserving or enhancing the underlying topological structures.
TADGATE captures specific attention patterns, pointing to two types of units with different characteristics. These units are closely associated with chromatin compartmentalization, and TAD boundaries in different compartmental environments exhibit distinct biological properties.
TADGATE also utilize a two-layer Hidden Markov Model to functionally annotate the TADs and their internal regions, revealing the overall properties of TADs and the distribution of the structural and functional elements within TADs.

□ DOT: a flexible multi-objective optimization framework for transferring features across single-cell and spatial omics
>> https://www.nature.com/articles/s41467-024-48868-z
DOT is a versatile and scalable optimization framework for the integration of scRNA-seq and SRT for localizing cell features by solving a multi-criteria mathematical program. DOT leverages the spatial context in a local manner without assuming a global correlation.
DOT employs several alignment objectives to locate the cell populations and the annotations therein in the spatial data. The alignment objectives ensure a high-quality transfer from different perspectives.