

□ starTracer: An Accelerated Approach for Precise Marker Gene Identification in Single-Cell RNA-Seq Analysis
>> https://www.biorxiv.org/content/10.1101/2023.09.21.558919v1
starTracer seamlessly accepts input in various formats, including Seurat objects, sparse expression matrices with annotation tables, or average expression matrix of each cell type.
StarTracer provides option to search marker genes from highly variable genes to further increase the calculation speed. A non-redundant matrix of marker genes will be presented according to the number of marker genes in each cluster.

□ veloVI: Deep generative modeling of transcriptional dynamics for RNA velocity analysis in single cells
>> https://www.nature.com/articles/s41592-023-01994-w
veloVI (velocity variational inference), a deep generative model for estimating RNA velocity. VeloVI reformulates the inference of RNA velocity via a model that shares information b/n all cells/genes, while learning the same quantities, namely kinetic parameters and latent time.
veloVI returns an empirical posterior distribution: matrix of cells by genes by posterior samples. veloVI illuminates cell states that have estimated with high uncertainty, which adds a notion of confidence to the velocity stream and highlights regions of the phenotypic manifold.

□ Divide-and-conquer quantum algorithm for hybrid de novo genome assembly of short and long reads
>> https://www.biorxiv.org/content/10.1101/2023.09.19.558544v1
Due to the path conflicts brought by repetitive sequences and sequencing errors, it is not feasible to directly determine an Eulerian path within the de Bruijn graph that faithfully reconstructs the original sequences.
A hybrid assembly quantum algorithm using high-accuracy short reads and error-prone long reads. It integrates short reads from next-generation sequencing technology and long reads from third-generation sequencing technology to address assembly path conflicts.
Using simulations of 10-qubit quantum computers, the algorithm addresses problems as large as 140 qubits, yielding optimal assembly results. The convergence speed is significantly improved via the problem-inspired ansatz based on the known information about the assembly problem.
This algorithm builds upon the variational quantum eigensolver and utilizes divide-and-conquer (VQE) strategies to approximate the ground state of larger Hamiltonian while conserving quantum resources.

□ CellPolaris: Decoding Cell Fate through Generalization Transfer Learning of Gene Regulatory Networks
>> https://www.biorxiv.org/content/10.1101/2023.09.25.559244v1
CellPolaris, a computational system that leverages transfer learning algorithms. Diverging from conventional GRN inference models, which heavily rely on integrating epigenomic data with transcriptomic information or adopt causal strategies through gene co-expression networks.
CellPolaris uses the transfer network to analyze single-cell transcriptomic data in the development or differentiation process and a Probabilistic Graphical Model (PGM) to predict the impact of TF perturbations on cell fate.

□ Finding related sequences by a simple sum over alignments
>> https://www.biorxiv.org/content/10.1101/2023.09.26.559458v1
A simplest-possible change to standard alignment sums probabilities of alternative alignments. It is easy to use in typical sequence-search software. It is also easy to calculate the probability of an equal or higher score between random sequences, based on a clear conjecture.
This method is a variant of "hybrid alignment”. Hybrid alignment has been neglected; the model produces different alignments with different probabilities. The method generalizes to different kinds of alignment e.g. DNA-versus-protein with frameshifts.

□ scBridge embraces cell heterogeneity in single-cell RNA-seq and ATAC-seq data integration
>> https://www.nature.com/articles/s41467-023-41795-5
scBridge models the discriminability and confidence of scATAC-seq cells with a Gaussian Mixture. scBridge achieves accurate scRNA-seq and scATAC-seq data integration, as well as label transfer with heterogeneous transfer learning.
scBridge uses the deep neural encoder and classifier. scBridge computes the ATAC prototypes as the weighted average of scATAC-seq cells with the same predicted cell type and aligns them with the RNA prototypes to achieve integration.

□ Uncertainty-aware single-cell annotation with a hierarchical reject option
>> https://www.biorxiv.org/content/10.1101/2023.09.25.559294v1
Hierarchical annotation in comparison to flat annot, leads to fewer label rejections under the full rejection, and these rejections are less severe under partial rejection. Consequently, if the rejection is implemented, hierarchical annotation proves to be the superior method.
With greedy label assignment, only the path with the highest probability scores in the hierarchy is followed. With non-greedy label assignment, all possible prediction paths are traversed and only the end score is considered for the final label assignment.

□ PG-SGD: Pangenome graph layout by Path-Guided Stochastic Gradient Descent
>> https://www.biorxiv.org/content/10.1101/2023.09.22.558964v1
PG-SGD (the Path Guided Stochastic Gtadient Descent) moves pairs of nodes in parallel applying a modified HOGWILD! strategy. The algorithm computes the pangenome graph layout that best reflects the nucleotide sequences in the graph.
PG-SGD stores node coordinates in a vector of atomic doubles. PG-SGD can be extended to any number of dimensions. It can be seen as a graph embedding algorithm that converts high-dimensional, sparse pangenome graphs into low-dimensional, dense, and continuous vector spaces.

□ DeepCCI: a deep learning framework for identifying cell-cell interactions from single-cell RNA sequencing data
>> https://academic.oup.com/bioinformatics/advance-article/doi/10.1093/bioinformatics/btad596/7281356
DeepCCI, a graph convolutional network (GCN) based deep learning framework for CCI identification. DeepCCI learns an embedding function that jointly projects cells into a shared embedding space using Autoencoder (AE) and GCN.
DeepCCI predicts intercellular crosstalk between any pair of clusters. It captures the essential hidden information of cells and makes full use of the topological relationships. DeepCCI determines the number of clusters before clustering, using the Louvain algorithm.

□ GPFN: Prior-Data Fitted Networks for Genomic Prediction
>> https://www.biorxiv.org/content/10.1101/2023.09.20.558648v1
A Genomic Prior-Data Fitted Network (GPFN), a new paradigm for GP. GPFNs perform amortized Bayesian inference by drawing hundreds of thousands or millions of synthetic breeding populations during the prior fitting phase.
GPFN fits the prior using a transformer model with 12 layers, an internal dimensionality of 2048, a hidden layer size of 2048, and a single attention head. Overfitting is no longer an issue, as training data is practically infinite.

□ LEOPARD: Missing view completion for multi-timepoints omics data via representation disentanglement and temporal knowledge transfer
>> https://www.biorxiv.org/content/10.1101/2023.09.26.559302v1
LEOPARD (missing view completion for multi-timepoints omics data via representation disentanglement and temporal knowledge transfer) extends representation disentanglement and style transfer techniques to the application of missing view completion in longitudinal omics data.
LEOPARD factorizes omics data from different timepoints into omics-specific content and timepoint-specific knowledge via contrastive learning. The generator learns mappings b/n two views, while temporal knowledge is injected into content representation via the AdalN operation.
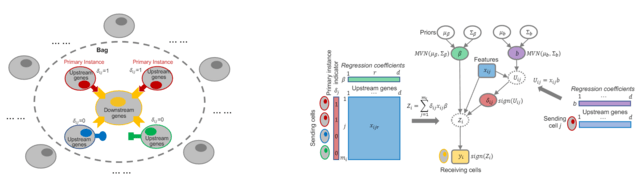
□ Spacia: Mapping Cell-to-cell Interactions from Spatially Resolved Transcriptomics Data
>> https://www.biorxiv.org/content/10.1101/2023.09.18.558298v1
Spacia, a Bayesian framework to detect cell-cell communication (CCC) from SRT data, by fully exploiting their unique spatial modality, which dramatically increased the accuracy of the detection of CCC.
Spacia uses cell-cell proximity as a constraint and prioritizes cell-cell interactions that cause a downstream change. Spacia employs a Multi-instance learning (MIL) to assess CCC. Spacia allows spatial information to minimize the number of assumptions and arbitrary parameters.

□ Spectra: Supervised discovery of interpretable gene programs from single-cell data
>> https://www.nature.com/articles/s41587-023-01940-3
Spectra (supervised pathway deconvolution of interpretable gene programs) receives a gene expression count matrix with cell-type labels for each cell as well as predefined gene sets, which it converts to a gene–gene graph.
Spectra fits a factor analysis model using a loss function that optimizes reconstruction of the count matrix and guides factors to support the input gene–gene graph. Spectra provides factor loadings and gene programs corresponding to cell types and cellular processes.

□ TAGET: a toolkit for analyzing full-length transcripts from long-read sequencing
>> https://www.nature.com/articles/s41467-023-41649-0
TAGET uses polished high-quality transcripts in fasta format as input (Fig. 1) for full-length transcriptome analysis. Following the Iso-seq data analysis protocol, TAGET only considers transcripts supported by at least two circular consensus sequences (CCS).
TAGET aligns transcripts to the reference genome by integrating alignment results from long and short reads and improves splice site prediction using Convolutional Neural Network. TAGET annotates transcripts by comparing with reference DBs and classifies them into seven classes.
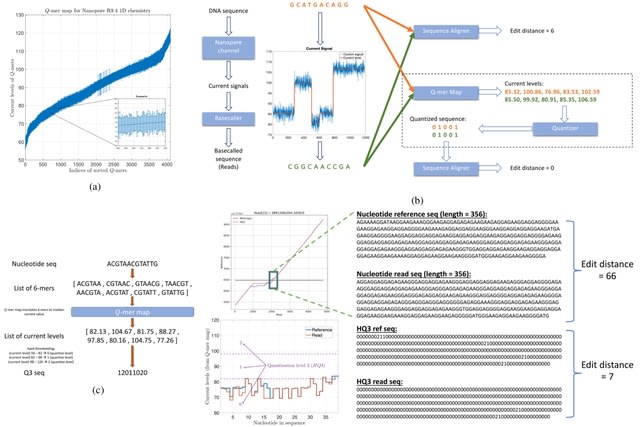
□ HQAlign: Aligning nanopore reads for SV detection using current-level modeling
>> https://academic.oup.com/bioinformatics/advance-article/doi/10.1093/bioinformatics/btad580/7280145
HQAlign is a hybrid mechanism with two steps of alignment. In the initial alignment step, the reads are aligned onto the genome in the nucleotide space using minimap2 to determine the region of interest where a read can possibly align.
In the hybrid step, the read is realigned to the region of interest on the genome in the quantized space. An alignment of the read-to-genome is maintained w/o dropping the frequently occurring seed matches, while the error biases are taken into account thru quantized sequences.

□ skani: Fast and robust metagenomic sequence comparison through sparse chaining
>> https://www.nature.com/articles/s41592-023-02018-3
skani is a program for calculating average nucleotide identity (ANI) from DNA sequences (contigs/MAGs/genomes). skani uses an approximate mapping method without base-level alignment to get ANI. This allows for sequence identity estimation using k-mers on only the shared regions between two genomes avoiding the pitfalls of alignment-ignorant sketching methods.

□ scPipe: An extended preprocessing pipeline for comprehensive single-cell ATAC-Seq data integration in R/Bioconductor
>> https://www.biorxiv.org/content/10.1101/2023.09.25.559230v1
scPipe is able to take FASTQ format as input, which are demultiplexed based on quality and Ns, aligned to the reference genome and filtered based on various quality metrics such as mapping rate, fraction of reads mapping and the number of duplicate or high-quality reads.

□ CellOT: Learning single-cell perturbation responses using neural optimal transport
>> https://www.nature.com/articles/s41592-023-01969-x
CellOT, a new approach that predicts perturbation responses of single cells by directly learning and uncovering maps between control and perturbed cell states, thus explicitly accounting for heterogeneous subpopulation structures in multiplexed molecular readouts.
CellOT models cell responses as deterministic trajectories. CellOT learns an optimal transport map for each perturbation in a fully parameterized and highly scalable manner. CellOT parameterizes a pair of dual potentials with input convex neural networks.

□ imply: improving cell-type deconvolution accuracy using personalized reference profiles
>> https://www.biorxiv.org/content/10.1101/2023.09.27.559579v1
imply can utilize personalized reference panels to precisely deconvolute cell type proportions using longitudinal or repeatedly measured data. It borrows information across the repeatedly measured transcriptome samples w/in each subject, to recover personalized reference panels.
imply utilizes support vector regression within a mixed-effect modeling framework to retrieve personalized reference panels, based on subjects’ phenotypical information. Then, it uses the recovered personalized reference panels to estimate cell type proportions.

□ MuDCoD: Multi-Subject Community Detection in Personalized Dynamic Gene Networks from Single Cell RNA Sequencing
>> https://academic.oup.com/bioinformatics/advance-article/doi/10.1093/bioinformatics/btad592/7281355
MuDCoD (Multi-subject Dynamic Community Detection), infers gene communities per subject and per time point by extending the temporal smoothness assumption to the subject dimension.
MuDCoD builds on the spectral clustering and promotes information sharing among the networks of the subjects AWA networks at different time points. It clusters genes in the personalized dynamic gene networks and reveals gene communities that are variable across time / subjects.

□ Bering: joint cell segmentation and annotation for spatial transcriptomics with transferred graph embeddings
>> https://www.biorxiv.org/content/10.1101/2023.09.19.558548v1
Bering, a graph deep learning model that leverages transcript colocalization relationships for joint noise-aware cell segmentation and molecular annotation in 2D and 3D spatial transcriptomics data.
The prediction outcome is binary classification, indicating whether the edges connect intercellular or intracellular spots. Molecular connectivity graphs are then constructed, and community detection algorithms such as Leiden Clustering are employed to identify cell borders.

□ CoalNN: Inference of coalescence times and variant ages using convolutional neural networks
>> https://academic.oup.com/mbe/advance-article/doi/10.1093/molbev/msad211/7279051
CoalNN uses a simulation-trained convolutional neural network (CNN) to jointly predict pairwise TMRCAs and recombination breakpoints, and further utilizes these predictions to estimate the age of genomic variants.
CoalNN is trained through simulation and can be adapted to varying parameters, such as demographic history, using transfer learning. CoalNN remains computationally efficient when applied to pairwise TMRCA inference, improving upon optimized coalescent Hidden Markov Models.

□ HAVAC: An FPGA-based hardware accelerator supporting sensitive sequence homology filtering with profile hidden Markov models
>> https://www.biorxiv.org/content/10.1101/2023.09.20.558701v1
Hardware Accelerated single segment Viterbi Additional Coprocessor (HAVAC), an FPGA-based hardware accelerator. The core HAVAC kernel calculates the SSV matrix at 1739 GCUPS on a Xilinx Alveo U50 FPGA accelerator card, ~227x faster than the optimized SSV implementation in nhmmer.

□ GammaGateR: semi-automated marker gating for single-cell multiplexed imaging
>> https://www.biorxiv.org/content/10.1101/2023.09.20.558645v1
GammaGateR provides estimates of marker-positive cell proportions and soft clustering of marker-positive cells. The model incorporates user-specified constraints that provide a consistent but slide-specific model fit.

□ Phylociraptor - A unified computational framework for reproducible phylogenomic inference
>> https://www.biorxiv.org/content/10.1101/2023.09.22.558970v1
Phylociraptor (the rapid phylogenomic tree calculator) performs all steps of typical phylogenomic workflows from orthology inference, MSA, trimming, and concatenation, to gene tree, supermatrix- and species tree reconstructions, complemented with various filtering steps.
Phylociraptor is organised into separate modules, which are executed consecutively, adhering to the principial stages of phylogenomic analyses. phylociraptor align creates MSAs using MAFFT, Clustal Omega and MUSCLE for each gene.

□ BaRDIC: robust peak calling for RNA-DNA interaction data
>> https://www.biorxiv.org/content/10.1101/2023.09.21.558815v1
BaRDIC (Binomial RNA-DNA Interaction Caller), that utilizes a binomial model to identify genomic regions significantly enriched in RNA-chromatin interactions, or "peaks", in All-To-All and One-To-All data.

□ GIA: A genome interval arithmetic toolkit for high performance interval set operations
>> https://www.biorxiv.org/content/10.1101/2023.09.20.558707v1
GIA (Genomic Interval Arithmetic) and BEDRS, a novel command-line tool and a rust library that significantly enhance the performance of genomic interval analysis.
Internally, both forms are treated as numeric, but during named serialization, gia calculates and stores a thin mapping of chromosome names to numeric indices - drastically reducing memory requirements and runtimes in most genomic interval contexts.

□ GET: a foundation model of transcription across human cell types
>> https://www.biorxiv.org/content/10.1101/2023.09.24.559168v1
GET (the general expression transformer) remarkable adaptability across new sequencing platforms and assays, enabling regulatory inference across a broad range of cell types and conditions, and uncovering universal and cell type specific transcription factor interaction networks.
GET learns transcriptional regulatory syntax from chromatin accessibility data acrs hundreds of diverse cell types. GET offers zero-shot prediction of reporter assay readout in new cell types, potentiating itself as a prescreening tool for cell type specific regulatory elements.

□ Modeling and interpretation of single-cell proteogenomic data
>> https://arxiv.org/abs/2308.07465
Single-cell proteogenomics will help connect single-cell genomics with the numerous post-transcriptional mechanisms - such as dynamically regulated protein synthesis, degradation, translocation, and post-translational modifications - that shape cellular phenotypes.

□ Single-cell lineage capture across genomic modalities with CellTag-multi reveals fate-specific gene regulatory changes
>> https://www.nature.com/articles/s41587-023-01931-4
An in situ reverse transcription (isRT) step is used to selectively reverse transcribe CellTag barcodes inside intact nuclei. The CellTag construct is modified to flank the random barcode with Nextera Read 1 and Read 2 adapters.
Direct lineage reprogramming presents a unique paradigm of cell identity conversion, with cells often transitioning through progenitor-like states or acquiring off-target identities. CellTag-multi identifies the distinct iEP reprogramming trajectories.

□ intNMF: Scalable joint non-negative matrix factorisation for paired single cell gene expression and chromatin accessibility data
>> https://www.biorxiv.org/content/10.1101/2023.09.25.559293v1
intNMF implements the accelerated hierarchical alternating least squares (acc-HALS) method, which they modified to jointly factorise two matrices. HALS is a block coordinate descent method where the optimisation problem is broken up into smaller sub-problems.
For the RNA modality this typically involved library sized normalisation followed by log(x + 1) transformation and for the ATAC modality Term Frequency-Inverse Document Frequency (TF-IDF) transforming the data. TF-IDF transformation is implemented in the intNMF package.

□ compleasm: a faster and more accurate reimplementation of BUSCO
>> https://academic.oup.com/bioinformatics/advance-article/doi/10.1093/bioinformatics/btad595/7284108
compleasm, an efficient tool for assessing the completeness of genome assemblies. Compleasm utilizes the miniprot protein-to-genome aligner and the conserved orthologous genes from BUSCO.
A complete gene is considered to have a single-copy in the assembly if it only has one alignment, or duplicated if it has multiple alignments. Compleasm reports the proportion of genes falling into each of the four categories as the assessment of assembly completeness.

□ GeneCompass: Deciphering Universal Gene Regulatory Mechanisms with Knowledge-Informed Cross-Species Foundation Model
>> https://www.biorxiv.org/content/10.1101/2023.09.26.559542v1
GeneCompass, a knowledge-informed cross-species foundation model pre-trained on scCompass-126M, a currently largest corpus encompassing over 120 million single-cell transcriptomes.
Inspired by self-supervised learning in natural language processing (NLP) domain, GeneCompass employs the masked language modeling (MLM) strategy to randomly mask gene tokens.

□ SeqVerify: A quality-assurance pipeline for whole-genome sequencing data
>> https://www.biorxiv.org/content/10.1101/2023.09.27.559766v1
SeqVerify, a computational pipeline designed to take raw WGS data and a list of intended edits, and verify that the edits are present and that there are no abnormalities.
SeqVerify operates on three main types of input for the majority of its results. These are paired-end short-read sequencing data, a reference genome to align this data to, and a list of "markers" - untargeted or targeted sequences.

□ StableMate: a new statistical method to select stable predictors in omics data
>> https://www.biorxiv.org/content/10.1101/2023.09.26.559658v1
StableMate, a flexible regression and variable selection framework based on the recent theoretical development of stabilised regression. Stabilised regression considers data collected from different 'environments'. i.e. technical or biological conditions.
□ Integrated DNA Technologies Reveals Launch of xGen™ Products for Ultima Genomics
>> https://sg.idtdna.com/pages/about/news/2023/09/26/integrated-dna-technologies-reveals-launch-of-xgen-products-for-ultima-genomics
xGen Universal Blocking Oligos for Ultima Genomics— proprietary blockers designed specifically for the platform’s native adapters, to reduce non-specific adapter interaction during probe hybridization and increase on-target capture performance.
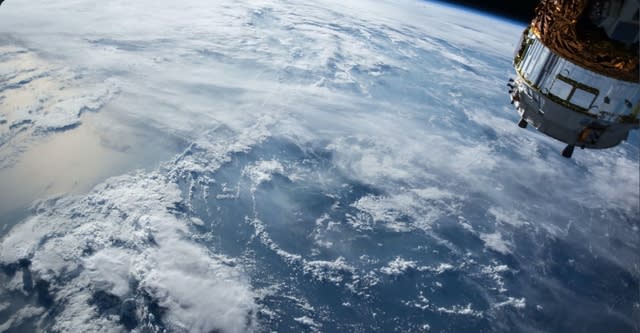
□ Invest in Estonia
>> https://investinestonia.com/estonian-space-startup-kappazetta-leaps-forward-with-additional-funding/
🛰️ #Estonian #space technology #startup #KappaZeta has secured a new round to further help farmers and set sights on forest #carbon stock assessment.