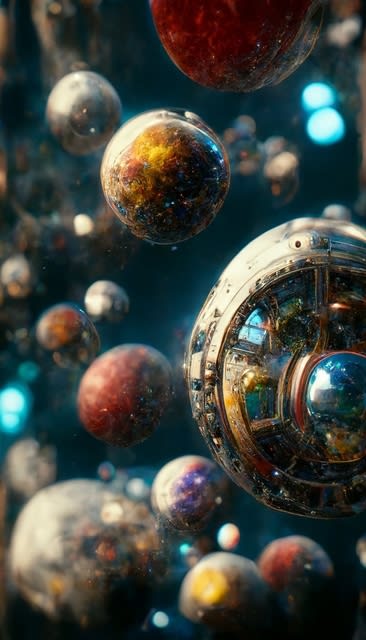
知性とは、関わる人間との相性や環境に依って相互に作用するベクトル量である。一時的な均衡に囚われていても、それは自己淘汰に向かう過程かもしれない。

□ The omnitig framework can improve genome assembly contiguity in practice
>> https://www.biorxiv.org/content/10.1101/2023.01.30.526175v1
Simple omnitigs are walks having a non-branching core, such that all nodes to the right of the core have out-degree one, and all nodes to the left of the core have in-degree one. It significantly improve length and contiguity over unitigs, while almost reaching that of omnitigs.
Simple omnitigs remain safe even when there are multiple linear chromosomes, as long as no chromosome starts or ends inside them. They give a linear output-sensitive time algorithm for finding all simple omnitigs.

□ SHARE-Topic: Bayesian Inerpretable Modelling of Single-Cell Multi-Omic Data
>> https://www.biorxiv.org/content/10.1101/2023.02.02.526696v1
SHARE-Topic, a Bayesian generative model of multi-omic single cell data. SHARE-Topic identifies common patterns of co-variation between different ‘omic layers, providing interpretable explanations for the complexity of the data.
SHARE-Topic extends the cisTopic model of single-cell chromatin accessibility by coupling the epigenomic state with gene expression through latent variables. SHARE-Topic provides a low-dimensional representation of multi-omic data by embedding cells in a topic space.

□ Verkko: Telomere-to-telomere assembly of diploid chromosomes
>> https://www.nature.com/articles/s41587-023-01662-6
To resolve the most complex repeats, this project relied on manual integration of ultra-long Oxford Nanopore sequencing reads with a high-resolution assembly graph built from long, accurate PacBio HiFi reads.
Verkko begins with a multiplex de Bruijn graph built from long, accurate reads and simplifies this graph by integrating ultra-long reads and haplotype-paths. A phased, diploid assembly of both haplotypes, with many chromosomes automatically assembled from telomere to telomere.
□ Genome Gov
>> https://www.genome.gov/news/news-release/nih-software-assembles-complete-genome-sequences-on-demand
.@Genome_gov researchers have developed and released an innovative software tool called Verkko for assembling truly complete genome sequences from a variety of species! Verkko makes assembling complete genome sequences more affordable and accessible.

□ scBGEDA: Deep Single-cell Clustering Analysis via a Dual Denoising Autoencoder with Bipartite Graph Ensemble Clustering
>> https://academic.oup.com/bioinformatics/advance-article/doi/10.1093/bioinformatics/btad075/7025496
scBGEDA preprocesses the high-dimensional sparse scRNA-seq data into compressed low-dimensional data. The second module is a single-cell denoising autoencoder based on a dual reconstruction loss that characterizes the scRNA-seq data by learning the robust feature representations.
scBGEDA comprises a bipartite graph ensemble clustering method used on the learned latent space to obtain the optimal clustering result. The scBGEDA algorithm encodes the scRNA-seq data in a discriminative representation, on which two decoders reconstruct the scRNA-seq data.

□ stRainy: assembly-based metagenomic strain phasing using long reads
>> https://www.biorxiv.org/content/10.1101/2023.01.31.526521v1
stRainy, an algorithm for phasing and assembly of closely-related strains. stRainy takes a sequence graph as input, identifies graph regions that represent collapsed strains, phases them and represents the results in an expanded and simplified assembly graph.
stRainy works with either a linear reference or a de novo assembly graph as input, and supports long reads. Because the strain variants are often unevenly distributed, regions of high and low heterozygosity may interleave in the assembly graph, which leads to tangles.

□ SATURN: Towards Universal Cell Embeddings: Integrating Single-cell RNA-seq Datasets across Species
>> https://www.biorxiv.org/content/10.1101/2023.02.03.526939v1
SATURN (Species Alignment Through Unification of Rna and proteiNs), a deep learning approach that integrates cross-species scRNA-seq datasets by coupling gene expression with protein embeddings generated by large protein language models.
SATURN introduces a concept of macrogenes defined as groups of functionally related genes. The strength of associations of genes to macrogenes are learnt to reflect the similarity of their corresponding protein embeddings.

□ PLANET: A Multi-Objective Graph Neural Network Model for Protein-Ligand Binding Affinity Prediction
>> https://www.biorxiv.org/content/10.1101/2023.02.01.526585v1
PLANET (Protein-Ligand Affinity prediction NETwork) was trained through a multi-objective process as multi-objective training has been proven useful for improving the performance and generalization of binding affinity prediction models.
PLANET is essentially a GNN model that captures protein–ligand interactions from the input structures, while deriving the intra-ligand distance matrix helps PLANET to capture 3D features from the 2D structural graph of the ligand.

□ Protein Sequence Design by Entropy-based Iterative Refinement
>> https://www.biorxiv.org/content/10.1101/2023.02.04.527099v1
An iterative sequence refinement pipeline, which can refine the sequence generated by existing sequence design. It retains reliable predictions based on the model’s confidence in predicted distributions, and decodes the residue type based on a partially visible environment.
Computing the entropy of the predicted distribution at each position and select the positions with low entropy, with the assumption that models are more confident with low-entropy predictions.
This method can remove a large portion of noise in the input residue environment, which improves both the generated sequences and the converging speed. The final prediction will be the averaged prediction from every iteration weighted by their entropy.

□ Metaphor - A workflow for streamlined assembly and binning of metagenomes
>> https://www.biorxiv.org/content/10.1101/2023.02.09.527784v1
Metaphor, a fully-automated workflow for GRM. Metaphor differs from GRM workflows by offering flexible approaches for the assembly and binning of the input data, and by combining multiple binning algorithms with a bin refinement step to achieve high quality genome bins.
Metaphor processes multiple datasets in a single execution, performing assembly and binning in separate batches for each dataset, and avoiding the need for repeated executions with different input datasets.
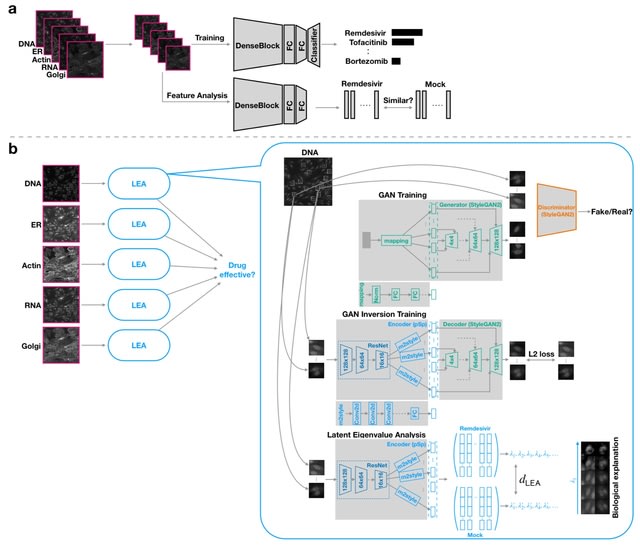
□ LEA: Latent Eigenvalue Analysis in application to high-throughput phenotypic profiling
>> https://www.biorxiv.org/content/10.1101/2023.02.10.528026v1
By quantifying the multi-dimensional eigenvalue difference, sorted eigenvalues can provide informative measurements along principal axes and facilitate a more complete analysis of data heterogeneity.
LEA learns robust latent representations with a residual-based encoder for reconstructing these single-cell images. LEA can refine the high-throughput cell-based drug analysis to single-cell and single-organelle granularity.

□ WMDS.net: a network control framework for identifying key players in transcriptome programs
>> https://academic.oup.com/bioinformatics/advance-article/doi/10.1093/bioinformatics/btad071/7023921
The weight of WMDS.net (the weighted minimum dominating set network) integrates the degree of nodes in the network and the significance of gene co-expression difference between two physiological states into the measurement of node controllability of the transcriptional network.

□ NIAPU: Network-Informed Adaptive Positive-Unlabeled learning for disease gene identification
>> https://academic.oup.com/bioinformatics/advance-article/doi/10.1093/bioinformatics/btac848/7023926
A set of network-based features to be used in a novel Markov diffusion-based multi-class labeling strategy. NIAPU is formed by the computation of the NeDBIT (Network diffusion and biology-informed topological) and the usage of APU (Adaptive Positive-Unlabelled label propagation).
The NIAPU classification is almost perfect since NeDBIT features allow those classes to be properly separated from the others since they grasp the topological aspects of the set of seed genes as a whole, assigning lower and lower weights to genes that are progressively far.

□ cvlr: finding heterogeneously methylated genomic regions using ONT reads
>> https://academic.oup.com/bioinformaticsadvances/article/3/1/vbac101/6998217
cvlr, a software which can be run from the command line on the output of Nanopore sequencing to cluster reads based on methylation patterns. Internally, the algorithm sees the data as a binary matrix, w/ n rows representing reads and d columns corresponding to genomic positions.
Reads are clustered (into k clusters) via a mixture of multivariate Bernoulli distributions. cvlr uses an EM algorithm. cvlr can be run to detect subpopulation of reads regardless of whether they are due to an allelic effect and does not need a preliminary phasing step.

□ A cloud-based pipeline for analysis of FHIR and long-read data
>> https://academic.oup.com/bioinformaticsadvances/article/3/1/vbac095/6994207
A full pipeline for working with both PacBio sequencing data and clinical FHIR data, from initial data to tertiary analysis. It performs variant calling on long-read PacBio HiFi data using Cromwell on Azure.
Both data formats are parsed, processed and merged in a single scalable pipeline which securely performs tertiary analyses using cloud-based Jupyter notebooks.

□ HiMAP2: Identifying phylogenetically informative genetic markers from diverse genomic resources
>> https://onlinelibrary.wiley.com/doi/10.1111/1755-0998.13762
HiMAP2 is a tool designed to identify informative loci from diverse genomic and transcriptomic resources in a phylogenomic framework. HiMAP2 identifies informative loci for phylogenetic studies, but it can also be used more widely for comparative genomic tasks.
HiMAP2 facilitates exploration of the final filtered exons by incorporating phylogenetic inference of individual exon trees with RAxML-NG as well as the estimation of a species tree using ASTRAL.

□ ClusterSeg: A crowd cluster pinpointed nucleus segmentation framework with cross-modality datasets
>> https://www.sciencedirect.com/science/article/abs/pii/S1361841523000191
ClusterSeg tackles nuclei clusters, which consists of a convolutional-transformer hybrid encoder and a 2.5-path decoder for precise predictions of nuclei instance mask, contours, and clustered-edges.
The instance-level segmentation performance adopts the prevalent Aggregated Jaccard Index (AJI), to evaluate connected components instead of pixels which penalizes over-segmentation, under-segmentation, as well mis-segmentation.

□ Mowgli: Paired single-cell multi-omics data integration
>> https://www.biorxiv.org/content/10.1101/2023.02.02.526825v1
Multi-Omics Wasserstein inteGrative anaLysIs (Mowgli), a novel method for the integration of paired multi-omics data with any type and number of omics. Of note, Mowgli combines integrative Nonnegative Matrix Factorization (NMF) and Optimal Transport.
Mowgli employs integrative NMF, popular in computational biology due to its intuitive representation by parts and further enhances its interpretability. Mowgli uses the entropic regularization of Optimal Transport as a reconstruction loss.

□ CATE: A fast and scalable CUDA implementation to conduct highly parallelized evolutionary tests on large scale genomic data.
>> https://www.biorxiv.org/content/10.1101/2023.01.31.526501v1
CATE (CUDA Accelerated Testing of Evolution) is capable of conducting evolutionary tests such as Tajima’s D, Fu and Li's, and Fay and Wu’s test statistics, McDonald–Kreitman Neutrality Index, Fixation Index, and Extended Haplotype Homozygosity.
CATE attempts to solve the problem of latency in conducting evolutionary tests through two key innovations: a unique file hierarchy together with a novel search algorithm (CIS) and GPU level parallelisation with the Prometheus mode.
The Prometheus architecture focuses mainly on batch processing of multiple query regions at the same time, whereas in normal mode CATE will process only a single query region at a time.

□ KmerCamel🐫: Masked superstrings as a unified framework for textual 𝑘-mer set representations
>> https://www.biorxiv.org/content/10.1101/2023.02.01.526717v1
Masked superstrings combines the idea of representing 𝑘-mer sets via a string that contains the 𝑘-mers as substrings, with masking out positions of the newly emerged “false positive” 𝑘-mers. This allows to remove the limitation of using (𝑘 − 1)-long overlap only.
KmerCamel🐫, which first reads a user-provided FASTA file with genomic sequences, computes the corresponding 𝑘-mer set, computes a masked superstring using a user-specified heuristic and core data structure, and prints it in the enc2 encoding.

□ The local topology of dynamical network models for biology
>> https://www.biorxiv.org/content/10.1101/2023.01.31.526544v1
Network motifs/anti-motifs are local structures that appear unusually often/rarely in a network. Their likelihood is quantified based on their average occurrence in randomizations of the network that preserve the degree of each node.
Slight differences are present in the literature about the tresholds and the randomizations involved in the quantitative definition of a motif. This work only considers fully connected triads, i.e. fully connected subsets of three nodes.

□ SNEEP: A statistical approach to identify regulatory DNA variations
>> https://www.biorxiv.org/content/10.1101/2023.01.31.526404v1
SNEEP is fast method to identify regulatry non-coding SNPs (rSNPs) that modify the binding sites of Transcription Factors (TFs) for large collections of SNPs provided by the user.
A modified Laplace distribution can adequately approximate the empirical distributions. It can derive a p-value for the maximal differential TF binding score in constant time.

□ Helixer: de novo Prediction of Primary Eukaryotic Gene Models Combining Deep Learning and a Hidden Markov Model.
>> https://www.biorxiv.org/content/10.1101/2023.02.06.527280v1
Helixer takes DNA sequence as input, makes base-wise predictions for genic class and phase with pre-trained Deep Neural Networks, and processes these predictions with a Hidden Markov Model to into primary gene models.
The optimal scoring path through this Markov Model for a given underlying sequence and set of base-wise predictions is determined with the Viterbi algorithm. The system penalizes discrepancies where the state of the Markov Model differs from the base-wise predictions by Helixer.

□ DEFND-seq: Scalable co-sequencing of RNA and DNA from individual nuclei
>> https://www.biorxiv.org/content/10.1101/2023.02.09.527940v1
DNA and Expression Following Nucleosome Depletion sequencing (DEFND- seq), a scalable method for co-sequencing RNA and DNA from single nuclei that uses commercial droplet microfluidics to achieve a high-throughput.
DEFND-seq treats nuclei with lithium diiodosalicylate to disrupt the chromatin and expose genomic DNA. Tagmented nuclei are loaded into a microfluidic generator, which co-encapsulates nuclei, beads cont. genomic barcodes, and reverse transcription reagents into single droplets.

□ SeqScreen-Nano: a computational platform for rapid, in-field characterization of previously unseen pathogens.
>> https://www.biorxiv.org/content/10.1101/2023.02.10.528096v1
The SeqScreen-Nano pipeline is based on the SeqScreen pipeline with substantial additions to deal with the complexity of long-read sequences Briefly, it is built upon; Initialize, SeqMapper, Protein / Taxonomic Identification, Functional Annotation and, Report generation.
SeqScreen-Nano can identify Open Reading Frames (ORFs) across the length of raw ONT reads and then use the predicted ORFs for accu- rate functional characterization and taxonomic classification.

□ Olivar: fully automated and variant aware primer design for multiplex tiled amplicon sequencing of pathogen genomes
>> https://www.biorxiv.org/content/10.1101/2023.02.11.528155v1
Olivar, an end-to-end pipeline for rapid and automatic design of primers for PCR tiling. Olivar accomplishes this by introducing the concept of the risk of primer design at the single nucleotide level, enabling fast evaluation of thousands of potential tiled amplicon sets.
Olivar looks for designs that avoid regions with high-risk scores based on SNPs, non- specificity, GC contents, and sequence complexity. Olivar also implements the SADDLE algorithm to optimize primer dimers in parallel and provides a separate validation module.

□  Complet+: a computationally scalable method to improve completeness of large-scale protein sequence clustering
>> https://peerj.com/articles/14779/
Complet+, a novel method to increase the completeness of clusters obtained using large-scale biological sequence clustering methods. Complet+ addresses a key problem with large-scale clustering methods, such as mmSeqs2 clustering and CD-HIT.
Complet+ utilizes the fast search capabilities of MMSeqs2 to identify reciprocal hits between the representative sequences, which may be used to reform clusters and reduce the number of singletons and small clusters and create larger clusters.

□ MDLCN: A multimodal deep learning model to infer cell-type-specific functional gene networks
>> https://bmcbioinformatics.biomedcentral.com/articles/10.1186/s12859-023-05146-x
MDLCN, a multimodal deep learning model, for predicting cell-type-specific FGNs by leveraging single-cell gene expression data with a global protein interaction network.
Gene expression signatures of a gene pair were first transformed to a co-expression matrix that captures the joint density of co-expression patterns of the gene pair across the cells in a particular cell type.
The co-expression matrix and the vector of proximity features were exploited as two modalities in the model, incl. a co-expression-processor modality to extract representations from the co-expression matrix and a proximity-processor modality to extract representations.

□ ChromDL: A Next-Generation Regulatory DNA Classifier
>> https://www.biorxiv.org/content/10.1101/2023.01.27.525971v1
ChromDL, a neural network architecture combining bidirectional gated recurrent units (BiGRU), CNNs, BiLSTM, which significantly improves upon a range of prediction metrics compared to its predecessors in TFBS, histone modification, and DNase-I hypersensitive site (DHS) detection.
ChromDL contains eleven layers. In total, the model contained 10,414,957 parameters, with 512 non-trainable parameters. ChromDL detects a significantly higher proportion of weak TFBS ChIP-seq peaks and demonstrates the potential to more accurately predict TF binding affinities.

□ wpLogicNet: logic gate and structure inference in gene regulatory networks
>> https://academic.oup.com/bioinformatics/advance-article/doi/10.1093/bioinformatics/btad072/7039679
wpLogicNet proposes a framework to infer the logic gates among any number of regulators, with a low time-complexity. This distinguishes wpLogicNet from the existing logic-based models that are limited to inferring the gate between two genes or TFs.
wpLogicNet applies a Bayesian mixture model to estimate the likelihood of the target gene profile and to infer the logic gate a posteriori. In structure-aware mode, wpLogicNet reconstructs the logic gates in TF-gene or gene-gene interaction networks with known structures.
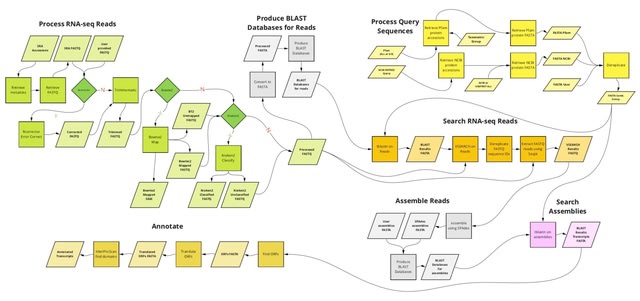
□ kakapo: Easy extraction and annotation of genes from raw RNA-seq reads
>> https://www.biorxiv.org/content/10.1101/2023.02.13.528395v1
kakapo (ka ̄ka ̄po ̄) is a python-based pipeline that allows users to extract and assemble one or more specified genes or gene families. It flexibly uses original RNA-seq read or GenBank SRA accession inputs without performing assembly of entire transcriptomes.
kakapo determines the genetic code for each sample, based on the sample
origin (NCBI TaxID) and the genomic source. kakapo can be employed to extract arbitrary loci, such as those commonly used for phylogenetic inference in systematics or candidate genes and gene families.

□ TRcaller: Precise and ultrafast tandem repeat variant detection in massively parallel sequencing reads
>> https://www.biorxiv.org/content/10.1101/2023.02.15.528687v1
TRcaller implements a novel algorithm for calling TR allele sequences from both short- and long-read sequences, generated from either whole genome and targeted sequences, and achieves greater accuracy and sensitivity than existing tools.
TRcaller uses an alignment strategy to define the boundaries of TRs. TRcaller takes an aligned sequence in indexed BAM format (with a BAI index) and a target TR loci file in BED format as input, and outputs the TR allele length/size, allele sequences, and supported read counts.

□ Five-letter seq: Simultaneous sequencing of genetic and epigenetic bases in DNA
>> https://www.nature.com/articles/s41587-022-01652-0
A whole-genome sequencing methodology capable of sequencing the four genetic letters in addition to 5mC and 5hmC to provide an accurate six-letter digital readout in a single workflow.
The processing of the DNA sample is entirely enzymatic and avoids the DNA degradation and genome coverage biases of bisulfite treatment. The five-letter seq workflow unambiguously resolves the four genetic bases and the epigenetic modifications, 5mC or 5hmC, termed hither to as modC.
Six-letter seq calls unmodC, 5mC and 5hmC when the true state is unmodC, 5mC and 5hmC. A critical requirement is to disambiguate 5mC from 5hmC without compromising genetic base calling within the same sample fragment.

□ baseLess: lightweight detection of sequences in raw MinION data
>> https://academic.oup.com/bioinformaticsadvances/article/3/1/vbad017/7036850
BaseLess reduces the MinION sequencing device to a simple species detector. As a trade-off, it runs on inexpensive computational hardware like single-board computers.
BaseLess deduces the presence of a target sequence by detecting squiggle segments corresponding to salient short sequences, k-mers, using an array of convolutional neural networks. baseLess can determine whether a read can be mapped to a given sequence or not.

□ REPAC: analysis of alternative polyadenylation from RNA-sequencing data
>> https://genomebiology.biomedcentral.com/articles/10.1186/s13059-023-02865-5
REPAC, a novel framework to detect differential alternative polyadenylation (APA) using regression of polyadenylation compositions which can appropriately handle the compositional nature of this type of data while allowing for complex designs.