
□ scLKME: A Landmark-based Approach for Generating Multi-cellular Sample Embeddings from Single-cell Data
>>
https://www.biorxiv.org/content/10.1101/2023.11.13.566846v1
scLKME, a landmark-based approach that uses kernel mean embedding to compute vector representations for samples profiled with single-cell technologies. scLKME sketches or sub-selects a limited set of cells across samples as landmarks.
scLKME maps them into a reproducing kernel Hilbert space (RKHS) using kernel mean embedding. The final embeddings are generated by evaluating these transformed distributions at the sampled landmarks, yielding a sample-by-landmark matrix.

□ Cellsig plug-in enhances CIBERSORTx signature selection for multi-dataset transcriptomes with sparse multilevel modelling
>>
https://academic.oup.com/bioinformatics/advance-article/doi/10.1093/bioinformatics/btad685/7413172
cellsig is a Bayesian multilevel generalised linear model tailored to RNA sequencing data. It uses joint hierarchical modelling to preserve the uncertainty of the mean-variability association of the gene-transcript abundance.
cellsig estimates the heterogeneity for cell-type transcriptomes, modelling population and group effects. They organised cell types into a differentiation hierarchy.
For each node of the hierarchy, cellsig allows for missing information due to partial gene overlap across samples (e.g. missing gene-sample pairs). The generated dataset is then input to CIBERSORTx to generate the transcriptional signatures.

□ RabbitKSSD: accelerating genome distance estimation on modern multi-core architectures
>>
https://academic.oup.com/bioinformatics/advance-article/doi/10.1093/bioinformatics/btad695/7424710
RabbitKSSD adopts the Kssd algorithm for estimating the similarities between genomes. In order to accelerate time-consuming sketch generation and distance computation, RabbitKSSD relies on a highly-tuned task partitioning strategy for load balancing and efficiency.
In the RabbitKSSD pipeline, the genome files undergo parsing to extract k-mers, which are subsequently used to generate sketches. Following this, the integrated pipeline computes pairwise distances among these genome sketches by retrieving the unified indexed dictionary.

□ MIXALIME: Statistical framework for calling allelic imbalance in high-throughput sequencing data
>>
https://www.biorxiv.org/content/10.1101/2023.11.07.565968v1
MIXALIME, a versatile framework for identifying ASEs from different types of high-throughput sequencing data. MIXALIME provides an end-to-end workflow from read alignments to statistically significant ASE calls, accounting for copy-number variation and read mapping biases.
MIXALIME offers multiple scoring models, from the simplest binomial to the beta negative binomial mixture, can incorporate background allelic dosage, and account for read mapping bias.
MIXALIME estimates the distribution parameters from the dataset itself, can be applied to sequencing experiments of various designs, and does not require dedicated control samples.

□ Mdwgan-gp: data augmentation for gene expression data based on multiple discriminator WGAN-GP
>>
https://bmcbioinformatics.biomedcentral.com/articles/10.1186/s12859-023-05558-9
MDWGAN-GP, a generative adversarial network model with multiple discriminators, is proposed. In addition, a novel method is devised for enriching training samples based on linear graph convolutional network.
MDWGAN-GP-C (resp. MDWGAN-GP-E) represents the model adopting only Cosine distance (resp. Euclidean distance). Multiple discriminators are adopted prevent mode collapse via providing more feedback signals to the generator.

□ ReGeNNe: Genetic pathway-based deep neural network using canonical correlation regularizer for disease prediction
>>
https://academic.oup.com/bioinformatics/advance-article/doi/10.1093/bioinformatics/btad679/7420211
ReGeNNe, an end-to-end deep learning framework incorporating the biological clustering of genes through pathways and further capturing the interactions between pathways sharing common genes through Canonical Correlation Analysis.
ReGeNNe’s Canonical Correlation based neural network modeling captures linear/ nonlinear dependencies between pathways, projects the features from genetic pathways into the kernel space, and ultimately fuses them together in an efficient manner for disease prediction.
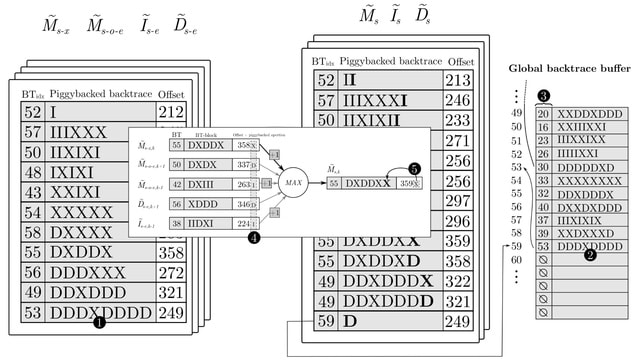
□ WFA-GPU: Gap-affine pairwise read-alignment using GPUs
>>
https://academic.oup.com/bioinformatics/advance-article/doi/10.1093/bioinformatics/btad701/7425447
WFA-GPU, a GPU-accelerated implementation of the Wavefront Alignment algorithm for exact gap-affine pairwise sequence alignment. It combines inter-sequence and intra-sequence parallelism to speed up the alignment computation.
A heuristic variant of the WFA-GPU that further improves its performance. WFA-GPU uses a bit-packed encoding of DNA sequences using 2 bits per base. It reduces execution divergence and the total number of instructions executed, which translates into faster execution times.

□ BELB: a Biomedical Entity Linking Benchmark
>>
https://academic.oup.com/bioinformatics/advance-article/doi/10.1093/bioinformatics/btad698/7425450
BELB, a Biomedical Entity Linking Benchmark providing access in a unified format to 11 corpora linked to 7 knowledge bases and 6 entity types: gene, disease, chemical, species, cell line and variant. BELB reduces preprocessing overhead in testing BEL systems on multiple corpora.
Using BELB they perform an extensive evaluation of six rule-based entity-specific systems and three recent neural approaches leveraging pre-trained language models.
Results of neural approaches do not transfer across entity types, with specialized rule-based systems still being the overall best option on entity-types not explored by neural approaches, namely genes and variants.

□ A new paradigm for biological sequence retrieval inspired by natural language processing and database research
>>
https://www.biorxiv.org/content/10.1101/2023.11.07.565984v1
This benchmarking study comparing the quality of sequence retrieval between BLAST and the HYFT methodology shows that BLAST is able to retrieve more distant homologous sequences with low percent identity than the HYFT-based search.
HYFT synonyms increases the recall. The HYFT methodology is extremely scalable as it does not rely on sequence alignment to find similars, but uses a parsing-sorting-matching scheme. The HYFT-based indexing is a solution to the biological sequence retrieval in a Big Data context.

□ ORCA: OmniReprodubileCellAnalysis: a comprehensive toolbox for the analysis of cellular biology data.
>>
https://www.biorxiv.org/content/10.1101/2023.11.07.565961v1
OmniReproducibleCellAnalysis (ORCA), a new Shiny Application based in R, for the semi-automated analysis of Western Blot (WB), Reverse Transcription-quantitative PCR (RT-qPCR), Enzyme-Linked ImmunoSorbent Assay (ELISA), Endocytosis and Cytotoxicity experiments.
ORCA allows to upload raw data and results directly on the data repository Harvard Dataverse, a valuable tool for promoting transparency and data accessibility in scientific research.

□ TBtools-II: A “one for all, all for one” bioinformatics platform for biological big-data mining
>>
https://www.cell.com/molecular-plant/fulltext/S1674-2052(23)00281-2
TBtools-II has the plugin mode to better meet personalized data analysis needs. Although there are methods available for quickly packaging command-line tools, such as PyQT, wxPython, and Perl/Tk, they often require users to be proficient with a programming language.
TBtools-II simplifies this process with its plugin “CLI Program Wrapper Creator”, making it easy for users to develop plugins in a standardized manner.
TBtools-II uses SSR Miner for the rapid identification of SSR (Simple Sequence Repeat) loci at the whole-genome level. To compare two genome sequences of two species or two haploids, users can also apply the “Genome VarScan” plugin to quickly identify structure variation regions.

□ A model-based clustering via mixture of hierarchical models with covariate adjustment for detecting differentially expressed genes from paired design
>>
https://bmcbioinformatics.biomedcentral.com/articles/10.1186/s12859-023-05556-x
A novel mixture of hierarchical models with covariate adjustment in identifying differentially expressed transcripts using high-throughput whole genome data from paired design. In their models, the three gene groups allow to have different coefficients of covariates.
In future, they plan to try the hybrid algorithm of the DPSO (Discrete Particle Swarm Optimization) and the EM approach to improve the global search performance.

□ WIMG: WhatIsMyGene: Back to the Basics of Gene Enrichment
>>
https://www.biorxiv.org/content/10.1101/2023.10.31.564902v1
WhatIsMyGene database (WIMG) will be the single largest compendium of transcriptomic and micro-RNA perturbation data. The database also houses voluminous proteomic, cell type clustering, IncRNA, epitranscriptomic (etc.) data.
WIMG generally outperforms in the simple task of reflecting back to the user known aspects of the input set (cell type, the type of perturbation, species, etc.), enhancing confidence that unknown aspects of the input may also be revealed in the output.
The WIMG database contains 160 lists based on WGCNA clustering. Typically, studies that utilize this procedure involve single-cell analysis, requiring large matrices to generate reliable gene-gene co-expression patterns.

□ SillyPutty: Improved clustering by optimizing the silhouette width
>>
https://www.biorxiv.org/content/10.1101/2023.11.07.566055v1
SillyPutty is a heuristic algorithm based on the concept of silhouette widths. Its goal is to iteratively optimize the cluster assignments to maximize the average silhouette width.
SillyPutty starts with any given set of cluster assignments, either randomly chosen, or obtained from other clustering methods. SillyPutty enters a loop where it iteratively refines the clustering. The algorithm calculates the silhouette widths for the current clustering.
SillyPutty identifies the data point with the lowest silhouette width. The algorithm reassigns this data point to the cluster to which it is closest. The loop continues until all data points have non-negative silhouette widths, or an early termination condition is reached.

□ flowVI: Flow Cytometry Variational Inference
>>
https://www.biorxiv.org/content/10.1101/2023.11.10.566661v1
flow VI, Flow Cytometry Variational Inference, an end-to-end multimodal deep generative model, designed for the comprehensive analysis of multiple MPC panels from various origins.
Flow VI learns a joint probabilistic representation of the multimodal cytometric measurements, marker intensity and light scatter, that effectively captures and adjusts for individual noise variances, technical biases inherent to each modality, and potential batch effects.

□ GeneToCN: an alignment-free method for gene copy number estimation directly from next-generation sequencing reads
>>
https://www.nature.com/articles/s41598-023-44636-z
GeneToCN counts the frequencies of gene-specific k-mers in FASTQ files and uses this information to infer copy number of the gene. GeneToCN allows estimating copy numbers for individual samples without the requirement of cohort data.
GeneToKmer script has the flexibility to either treat them separately or to define all 3 copies as a single gene. In the first case, GeneToCN uses the k-mers specific to each different copy, whereas in the latter case, GeneToCN uses only k-mers that are present in all 3 copies.

□ ENCODE-rE2G: An encyclopedia of enhancer-gene regulatory interactions in the human genome
>>
https://www.biorxiv.org/content/10.1101/2023.11.09.563812v1
ENCODE-rE2G, a new predictive model that achieves state-of-the-art performance across multiple prediction tasks, demonstrating a strategy involving iterative perturbations and supervised machine learning to build increasingly accurate predictive models of enhancer regulation.
Using the ENCODE-rE2G model, they build an encyclopedia of enhancer-gene regulatory interactions in the human genome, which reveals global properties of enhancer networks, identifies differences in the functions of genes that have more or less complex regulatory landscapes.

□ SEAMoD: A fully interpretable neural network for cis-regulatory analysis of differentially expressed genes
>>
https://www.biorxiv.org/content/10.1101/2023.11.09.565900v1
SEAMoD (Sequence-, Expression-, and Accessibility-based Motif Discovery), implements a fully interpretable neural network to relate enhancer sequences to differential gene expression.
SEAMoD can make use of epigenomic information provided in the form of candidate enhancers for each gene, with associated scores reflecting local chromatin accessibility, and automatically search for the most promising enhancer among the candidates.
SEAMoD is a multi-task learner capable of examining DE associated with multiple biological conditions, such as several differentiated cell types compared to a progenitor cell type, thus sharing information across the different conditions in its search for underlying TF motifs.

□ Optimal control of gene regulatory networks for morphogen-driven tissue patterning
>>
https://www.sciencedirect.com/science/article/pii/S2405471223002922
An alternative framework using optimal control theory to tackle the problem of morphogen-driven patterning: intracellular signaling is derived as the control strategy that guides cells to the correct fate while minimizing a combination of signaling levels and time.
This approach recovers observed properties of patterning strategies and offers insight into design principles that produce timely, precise, and reproducible morphogen patterning. This framework can be combined w/ dynamical-Waddington-like-landscape models of cell-fate decisions.

□ OrthoRep: Continuous evolution of user-defined genes at 1-million-times the genomic mutation rate
>>
https://www.biorxiv.org/content/10.1101/2023.11.13.566922v1
OrthoRep, a new orthogonal DNA replication system that durably hypermutates chosen genes at a rate of over 104 substitutions per base in vivo.
OrthoRep obtained thousands of unique multi-mutation sequences with many pairs over 60 amino acids apart (over 15% divergence), revealing known and new factors influencing enzyme adaptation.
The fitness of evolved sequences was not predictable by advanced machine learning models trained on natural variation. OrthoRep systems would take 100 generations (8-12 days for the yeast host of OrthoRep) just to sample an average of 1 new mutation in a typical 1 kb gene.

□ RUBic: rapid unsupervised biclustering
>>
https://bmcbioinformatics.biomedcentral.com/articles/10.1186/s12859-023-05534-3
RUBic converts the expressions into binary data using mixture of left truncated Gaussian distribution model (LTMG) and find the biclusters using novel encoding and template searching strategy and finally generates the biclusters in two modes base and flex.
RUBic generates maximal biclusters in base mode, and in flex mode results less and biological significant clusters. The average of maximum match scores of all biclusters generated by RUBic with respect to the BiBit algorithm and vice-versa are exactly the same.

□ EUGENe: Predictive analyses of regulatory sequences
>>
https://www.nature.com/articles/s43588-023-00544-w
EUGENe (Elucidating the Utility of Genomic Elements with Neural nets) transforms sequence data from many common file formats; tains diverse model architectures; and evaluates and interpreting model behavior.
EUGENe provides flexible functions for instantiating common blocks and towers that are composed of heterogeneous sets of layers. EUGENe supports customizable fully connected, convolutional, recurrent and Hybrid architectures that can be instantiated from single function calls.

□ pUMAP: Robust parametric UMAP for the analysis of single-cell data
>>
https://www.biorxiv.org/content/10.1101/2023.11.14.567092v1
pUMAP is capable of efficiently projecting future data onto the same space as the training data. The effect of negative sample strength on the overall structure of the low dimensional embedding produced by trained pUMAP for pancreatic.
pUMAP uses neural networks to parameterize complex functions that map GE data onto a lower dimensional space of an arbitrary dimension. pUMAP constructs a KNN graph from the high dimensional space and computes a weight for the edge that points that scales w/ their local distance.

□ Hierarchical annotation of eQTLs enables identification of genes with cell-type divergent regulation
>>
https://www.biorxiv.org/content/10.1101/2023.11.16.567459v1
A network-based hierarchical model to identify cell-type specific eQTLs in complex tissues with closely related and nested cell types. This model extends the existing CellWalkR modell to take a cell-type hierarchy as input in addition to cell-type labels and scATAC-seq data.
Briefly, the cell type hierarchy is taken as prior knowledge, and it is implemented as edges between leaf nodes that represent specific cell types and internal nodes that represent broader cell types higher in the hierarchy.
The cell type nodes are then connected to nodes representing cells based on how well marker genes correspond to each cell's chromatin accessibility, and cells are connected to each other based on the similarity of their genome-wide chromatin accessibility.
A random walk with random restarts model of node to each other node. In particular, this includes the probability that a walk starting at each cell node ends at each cell type node as well as each internal node representing portions of the cell-type hierarchy.

□ A Method for Calculating the Least Mutated Sequence in DNA Alignment Based on Point Mutation Sites
>>
https://www.biorxiv.org/content/10.1101/2023.11.14.567125v1
The Least mutated sequence calculates the transition/transversion ratio for each sequence in an DNA alignment. It can be used as a rough measure for estimating selection pressure and evolutionary stability for a sequence.
By parsimony principle, the least mutated sequence should be the phylogenetic root for all the other sequences in an alignment result. This method is a non-parameter method and uses the point mutation sites in a DNA alignment result for calculation.
This method only needs a very small proportion of sequences to find the root sequence under random sampling, and quite robust against reverse mutation and saturation mutation, whose accuracy rises with the increasing number of sampling sequences.

□ HapHiC: Chromosome-level scaffolding of haplotype-resolved assemblies using Hi-C data without reference genomes
>>
https://www.biorxiv.org/content/10.1101/2023.11.18.567668v1
HapHiC, a Hi-C-based scaffolding tool that enables allele-aware chromosome scaffolding of autopolyploid assemblies without reference genomes. They conducted a comprehensive investigation into the factors that may impede the allele-aware scaffolding of genomes.
HapHiC conducts contig ordering and orientation by integrating the algorithms from 3D-DNA and ALLHiC. HapHiC employs the "divide-and-conquer" strategy to isolate their negative impacts between the two steps.

□ DisCoPy: the Hierarchy of Graphical Languages in Python
>>
https://arxiv.org/abs/2311.10608
DisCoPy is a Python toolkit for computing w/ monoidal categories. It comes w/ two flexible data structures for string diagrams: the first one for planar monoidal categories based on lists of layers, the second one for symmetric monoidal categories based on cospans of hypergraphs.
Algorithms for functor application then allow to translate string diagrams into code for numerical computation, be it differentiable, probabilistic or quantum.

□ SpaGRN: investigating spatially informed regulatory paths for spatially resolved transcriptomics data
>>
https://www.biorxiv.org/content/10.1101/2023.11.19.567673v1
SpaGRN, a statistical framework for predicting the comprehensive intracellular regulatory network underlying spatial patterns by integrating spatial expression profiles with prior knowledge on regulatory relationships and signaling paths.
SpaGRN identifies spatiotemporal variations in specific regulatory patterns, delineating the cascade of events from receptor stimulation to downstream transcription factors and targets, revealing synergetic regulation mechanism during organogenesis.

□ Snapper: high-sensitive detection of methylation motifs based on Oxford Nanopore reads
>>
https://academic.oup.com/bioinformatics/advance-article/doi/10.1093/bioinformatics/btad702/7429397
Snapper, a new highly-sensitive approach to extract methylation motif sequences based on a greedy motif selection algorithm. It collects normalized signal levels for this k-mer from multi-fast5 files for both native and WGA samples.
The algorithm directly compares the collected signal distributions. Using the Kolmogorov-Smirnov test in order to select k-mers that most likely contain a modified base. The result of the first stage is an exhaustive set of all potentially modified k-mers.
Next, the greedy motif enrichment algorithm implemented in Snapper iteratively extracts potential methylation motifs and calculates corresponding motif confidence levels.

□ Centre: A gradient boosting algorithm for Cell-type-specific ENhancer-Target pREdiction
>>
https://academic.oup.com/bioinformatics/advance-article/doi/10.1093/bioinformatics/btad687/7429396
CENTRE is a machine learning framework that predicts enhancer target interactions in a cell-type-specific manner, using only gene expression and ChIP-seq data for three histone modifications for the cell type of interest.
CENTRE extracts all the cCRE-ELS within 500KB of target genes and computes CT-specific and generic features for all potential ET pairs. ET feature vectors are then fed to a pre-trained XGBOOST classifier, and a probability of an interaction is assigned to ET pairs.

□ CellSAM: A Foundation Model for Cell Segmentation
>>
https://www.biorxiv.org/content/10.1101/2023.11.17.567630v1
CellSAM, a foundation model for cell segmentation that generalizes across diverse cellular imaging data. CellSAM builds on top of the Segment Anything Model (SAM) by developing a prompt engineering approach to mask generation.
CellFinder, a transformer-based object detector that uses the Anchor DETR framework. It automatically detects cells and prompt SAM to generate segmentations.

□ Extraction and quantification of lineage-tracing barcodes with NextClone and CloneDetective
>>
https://www.biorxiv.org/content/10.1101/2023.11.19.567755v1
NextClone and CloneDetective, an integrated highly scalable Nextflow pipeline and R package for efficient extraction and quantification of clonal barcodes from scRNA-seq data and DNA sequencing data tagged with lineage-tracing barcodes.
NextClone is particularly engineered for high scalability to take full advantage of the vast computational resources offered by HPC platforms. CloneDetective is an R package to interrogate clonal abundance data generated using lineage tracing protocol.