

□ HYFA: Hypergraph factorization for multi-tissue gene expression imputation
>> https://www.nature.com/articles/s42256-023-00684-8
HYFA (hypergraph factorization) is genotype agnostic, supports a variable number of collected tissues per individual, and imposes strong inductive biases to leverage the shared regulatory architecture of tissues and genes.
HYFA employs a custom message-passing neural network that operates on a 3-uniform hypergraph. HYFA infers latent metagene values for the target tissue—a hyperedge-level prediction task—and maps these representations back to the original gene expression space.

□ Charting cellular differentiation trajectories with Ricci flow
>> https://www.biorxiv.org/content/10.1101/2023.07.20.549833v1
Modern interpretations of Waddington's Landscape have re-framed cell fate trajectories via the phase space of transcriptomic dynamics. A framework for employing a discrete Ricci curvature and normalized Ricci flow to predict dynamic trajectories b/n temporally linked GE samples.
Network entropy and the total Forman-Ricci curvature are related quantities but not interchangeable. A positive correlation between network entropy and total discrete curvature of a biological network, by appealing to results on metric-measure spaces.

□ GraphChainer: Chaining for Accurate Alignment of Erroneous Long Reads to Acyclic Variation Graphs
>> https://academic.oup.com/bioinformatics/advance-article/doi/10.1093/bioinformatics/btad460/7231478
A new algorithm to co-linearly chain a set of seeds in a string labeled acyclic graph, together with the first efficient implementation of such a co-linear chaining algorithm into a new aligner of erroneous long reads to acyclic variation graphs, GraphChainer.
GraphChainer connects the anchor paths to obtain a longer path, which is then reported as the answer. GraphChainer splits its solution whenever a path joining consecutive anchors is longer than some parameter g = colinear-gap, and reports the longest path after these splits.

□ GNNome Assembly: Untangling genome assembly graphs with graph neural networks
>> http://talks.cam.ac.uk/talk/index/202234
GNNome Assembly consists of simulating the synthetic reads, generating the assebmly graphs, and decoding edge probabilities with greedy search. The selected path is translated into a contig of reconstructed genome by concatenating the overlapping reads in the path.
GNNome Assembly constructs assembly graphs using Raven. GatedGCN is utilized to compute d-dimensional representations of nodes and edges. An Multi-Layer Perception classifier then outputs a probability indicating whether a given edge can lead to the optimal reconstruction.

□ cloudrnaSPAdes: Isoform assembly using bulk barcoded RNA sequencing data
>> https://www.biorxiv.org/content/10.1101/2023.07.25.550587v1
cloudrnaSPAdes, a novel tool for de novo assembly of full-length isoforms from barcoded RNA-seq data. It constructs a single assembly graph using the entire set of input reads and further derives paths for each read cloud, closing gaps and fixing sequencing errors in the process.
cloudrnaSPAdes is able to accurately reconstruct full-length transcript sequences from read clouds having coverage as low as 1x, including genes with dozens of different expressing isoforms.

□ GRouNdGAN: GRN-guided simulation of single-cell RNA-seq data using causal generative adversarial networks
>> https://www.biorxiv.org/content/10.1101/2023.07.25.550225v1
GRouNdGAN simulates steady-state and transient-state single-cell datasets where genes are causally expressed under the control of their regulating TFs. GRouNdGAN captures non-linear TF-gene dependences and preserves gene identities, cell trajectories and pseudo-time ordering.
The architecture of GRouNdGAN builds on the causal generative adversarial network (CausalGAN) and includes a causal controller, several target generators, a critic, a labeler and an anti-labeler all implemented as separately parameterized neural networks.

□ seq2cells: Single-cell gene expression prediction from DNA sequence at large contexts
>> https://www.biorxiv.org/content/10.1101/2023.07.26.550634v1
seq2cells uses a transfer learning framework that utilizes Enformer as a pre-trained epigenomic model, to create gene embeddings that capture the sequence logic of transcriptional regulation.
seq2cells can in principle use as a seq2emb module any model that embeds the DNA sequence of the TSS. The Enformer trunk takes as input a one-hot encoded 196,608 base pair DNA sequence and outputs 3,072 dimensional sequence embedding of the central 896 sequence windows.
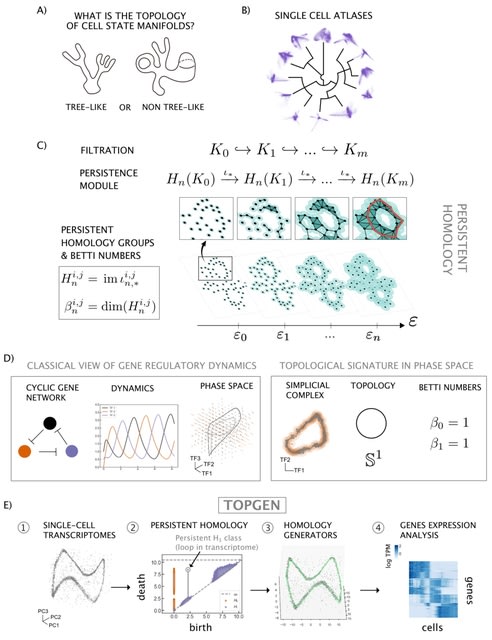
□ TopGen: Unraveling cell differentiation mechanisms through topological exploration of single-cell developmental trajectories
>> https://www.biorxiv.org/content/10.1101/2023.07.28.551057v1
TopGen, a method that uses the representatives of homology groups to analyze gene expression patterns. In essence, the method involves establishing a common basis for the kernel and image of consecutive boundary maps via the Smith Normal Form.
By calculating the n-th Betti number, we can determine the homology group generator from this shared basis. By hypothesis, cyclic topologies would have oscillatory genes that are transiently active in different parts of the cycle.
The eigenfunctions of the Laplace-Beltrami operator encodes the geometry of a manifold in an orthogonal basis of harmonic functions. The discrete version of these harmonic eigenfunctions also turn out to have oscillatory behavior and are eigenvectors of the discrete Laplacian.

□ Theory and models of (∞,ω)-categories
>> https://arxiv.org/abs/2307.11931
The models of (∞,ω)-categories. The main result is to establish a Quillen equivalence between Rezk's complete Segal Θ-spaces and Verity's complicial sets.
The (∞,1)-category corresponding to these two model structures, denoted by (∞,ω)-cat. Its connection with Rezk's complete Segal Θ-spaces allows us to use the globular language, while its connection with complicial sets gives us access to a fundamental operation, the Gray tensor product.
The objective will be to implement standard categorical constructions in the context of (∞,ω)-categories. A special emphasis will be placed on the Grothendieck construction.

□ LegNet: a best-in-class deep learning model for short DNA regulatory regions
>> https://academic.oup.com/bioinformatics/advance-article/doi/10.1093/bioinformatics/btad457/7230784
LegNet, an EfficientNetV2-inspired convolutional network for modeling short gene regulatory regions. LegNet can be used in diffusion generative modeling as a step toward the rational design of gene regulatory sequences.
LegNet-Generator corrects the artificial noise by reverting back point mutations introduced in sequences with known expression levels.
Iterative generation by applying LegNet-Generator induces substitutions in a completely random sequence, i.e. by tricking the model to correct "errors" in the provided random sequence so that upon full correction the resulting promoter provides a desired expression level.

□ LPHash: Locality-preserving minimal perfect hashing of k-mers
>> https://academic.oup.com/bioinformatics/article/39/Supplement_1/i534/7210438
LPHash achieves very compact space by exploiting the fact that consecutive k-mers share overlaps of k - 1 symbols. This allows LPHash to actually break the theoretical log 2(e) bit/key barrier for MPHFs.
One used to build a BBHash function over the k-mers and spend 3 bits/k-mer and 100-200 ns per lookup. This work shows that it is possible to do significantly better than this when the k-mers come from a spectrum-preserving string set: less than 0.6-0.9 bits/k-mer and 30-60 ns.

□ DeepDynaForecast: Phylogenetic-informed graph deep learning for epidemic transmission dynamic prediction
>> https://www.biorxiv.org/content/10.1101/2023.07.17.549268v1
DeepDynaForecast, a cutting-edge deep learning algorithm designed for forecasting pathogen transmission dynamics. DeepDynaForecast was trained on in-depth data and used more information from the phylogenetic tree, allowing classification of samples according to their dynamics.
DeepDynaForecast incorporates the Primal-Dual Graph Long Short-Term Memory learning architecture. The Phylogenetic tree is modeled as a bi-directed graph. DeepDynaForecast can predict near-future transmission dynamics for the external nodes.

□ Unified fate mapping in multiview single-cell data
>> https://www.biorxiv.org/content/10.1101/2023.07.19.549685v1
CellRank 2 models cell-state dynamics from multiview single-cell data. It automatically determines initial and terminal states, computes fate probabilities, charts trajectory-specific gene expression trends, and identifies putative driver genes.
CellRank2 employs a probabilistic system description wherein each cell constitutes one state in a Markov chain with edges representing cell-cell transition probabilities.
CellRank 2 provides a set of diverse kernels that derive transition probabilities. CellRank 2 generalizes earlier concepts to arbitrary pseudotimes and atlas-scale datasets with the PseudotimeKernel and CytoTRACEKernel. The RealTimeKernel combines across time point transitions.

□ PINNACLE: Contextualizing protein representations using deep learning on protein networks and single-cell data
>> https://www.biorxiv.org/content/10.1101/2023.07.18.549602v1
PINNACLE (Protein Network-based Algorithm for Contextual Learning), a self-supervised geometric deep learning model adept at generating protein representations through the analysis of protein interactions within various cellular contexts.
In total, PINNACLE's unified multi-scale embedding space comprises 394,760 protein representations, 156 cell type representations, and tissue representations.
PINNACLE generates a distinct representation for each cell type in which a protein-coding gene is activated. PINNACLE learns the topology of proteins, cell types, and tissues by optimizing a unified latent representation space.


□ FraSICL: Molecular Property Prediction by Semantic-invariant Contrastive Learning
>> https://academic.oup.com/bioinformatics/advance-article/doi/10.1093/bioinformatics/btad462/7233069
FraSICL (Fragment-based Semantic-Invariant Contrastive Learning), a semantic-invariant view generation method by properly breaking molecular graphs into fragment pairs.
FraSICL is an asymmetric model with two branches, the molecule view branch and the fragment view branch. FraSICL is trained by both NT-Xent contrastive loss and an auxiliary similarity loss. In the contrastive loss, two projections of a molecule are treated as a positive pair.

□ XA4C: eXplainable representation learning via Autoencoders revealing Critical genes
>> https://www.biorxiv.org/content/10.1101/2023.07.16.549209v1
XA4C offers optimized autoencoders to process gene expressions at two levels: whole transcriptome (global) autoencoder, and single pathway (local) autoencoders. The decoder is symmetrical to the encoder counterpart to recover the gene expressions.
XA4C disentangles the black box of the neural network of an autoencoder by providing each gene's contribution to the latent variables. XA4C quantifies the Critical index of a gene by averaging the absolute values of its SHapley Additive exPlanations value to all latent variable.

□ cycle_finder: de novo analysis of tandem and interspersed repeats based on cycle-finding
>> https://www.biorxiv.org/content/10.1101/2023.07.17.549334v1
cycle_finder constructs a graph structure from low-cost short-read data and constructs units of both types of repeats. The tool can detect cycles with branching and corresponding tandem repeats, and can construct interspersed repeats by exploring non-cycle subgraphs.
cycle_finder can estimate sequences w/ large copy-number differences. Tandem repeats detected from de Bruin graphs are output as different sequences if they contain even a single nucleotide difference, a large number of sequences are detected from sequences in the same cluster.

□ RECOMBINE: Recurrent composite markers of cell types and states
>> https://www.biorxiv.org/content/10.1101/2023.07.17.549344v1
RECOMBINE, a novel framework, recurrent composite markers for biological identities with neighborhood enrichment. RECOMBINE is a data-driven approach for unbiased selection of composite markers that characterize discrete cell types and continuous cell states in tissue ecosystems.
RECOMBINE selects an optimized set of markers that discriminate hierarchical cell subpopulations. RECOMBINE identifies recurrent composite markers (RCMs) for not only discrete cell types but also continuous cell states with high granularity.

□ AE-TWAS: Autoencoder-transformed transcriptome improves genotype-phenotype association studies
>> https://www.biorxiv.org/content/10.1101/2023.07.23.550223v1
AE-TWAS, which adds a transformation step before conducting standard TWAS. The transformation is composed of two steps by first splitting the whole transcriptome into co-expression networks and then using autoencoder to reconstruct the transcriptome data within each module.
This transformation removes noise (including nonlinear ones) from the transcriptome data, paving the path for downstream TWAS. After transformation, the transcriptome data enjoy higher expression heritability at the low-heritability spectrum and possess higher connectivity.

□ Petasearch: Efficient parallelized peta-scale protein database search
>> https://github.com/steineggerlab/petasearch
Petasearch depends on block-aligner for fast computation of Smith-Waterman alignments in the blockalign module. format. You can use convert2sradb to convert a FASTA/FASTQ file or a MMseqs2 database into a srasearch database.

□ netSGCCA: Integrating multi-omics and prior knowledge: a study of the Graphnet penalty impact
>> https://academic.oup.com/bioinformatics/advance-article/doi/10.1093/bioinformatics/btad454/7230780
This work focuses on studying the effect of the injection of a prior graphical knowledge as a penalty into a parsimonious variant of the Regularised Generalised Canonical Correlation (RGCCA) model, namely the Sparse Generalised Canonical Correlation Analysis (SGCCA).
Contrary to Elastic-Net, GraphNet penalty can select a reasonable set of genes and yields informative interpretation from the pathway enrichment analysis. The co-selection of variables is not primarily influenced by the structure of the graph, but rather by its overall density.

□ L-GIREMI uncovers RNA editing sites in long-read RNA-seq
>> https://genomebiology.biomedcentral.com/articles/10.1186/s13059-023-03012-w
L-GIREMI (long-read GIREMI) effectively handles sequencing errors and biases in the reads and uses a model-based approach to score RNA editing sites. L-GIREMI allows investigation of RNA editing patterns of single RNA molecules, co-occurrence of multiple RNA editing events.
L-GIREMI examines the linkage patterns between sequence variants in the same reads, complemented by a model-driven approach, to predict RNA editing sites. L-GIREMI affords high accuracy as reflected by the high fraction of A-to-G sites or known REDIportal sites in its predictions.

□ Voyager: exploratory single-cell genomics data analysis with geospatial statistics
>> https://www.biorxiv.org/content/10.1101/2023.07.20.549945v1
Voyager implements plotting functions for gene expression, cell attributes, and spatial analysis results. The documentation website includes tutorials that demonstrate ESDA on data from multiple spatial -omics, incl. Visium, Slide-seq, Xenium, CosMX, MERFISH, seqFISH, and CODEX.
Voyager is built on the SFEdata structure, which bundles geometries such as cell segmentation polygons with gene expression data. While Vovager is focused on spatial data, neighborhood view ESDA methods can be applied to the k-nearest-neighbor graph in gene expression PCA space.

□ LOCLA: A Novel Genome Optimization Tool for Chromosome-Level Assembly across Diverse Sequencing Techniques
>> https://www.biorxiv.org/content/10.1101/2023.07.20.549842v1
LOCLA (Local Optimization for Chromosome-Level Assembly) identifies reads and contigs aligned locally with high quality on gap flanks or scaffold boundaries of draft assemblies for gap filling and scaffold connection. LOCLA applies to both de novo and reference-based assemblies.
LOCLA can utilize reads produced by diverse sequencing techniques, e.g., 10x Genomics Linked-Reads, and PacBio HiFi reads. LOCLA enhances the draft assemblies by recovering 27.9 million bases and 35.7 million bases of the sequences discarded by the reference-guided assembly tool.

□ MAGICAL: Mapping disease regulatory circuits at cell-type resolution from single-cell multiomics data
>> https://www.nature.com/articles/s43588-023-00476-5
MAGICAL (Multiome Accessibility Gene Integration Calling and Looping), a hierarchical Bayesian approach that leverages paired scRNA-seq and transposase-accessible chromatin sequencing from different conditions to map disease-associated TFs and genes as regulatory circuits.
Using Gibbs sampling, MAGICAL iteratively estimates variable values and optimizes the states of circuit TF–peak–gene linkages.
MAGICAL introduces hidden variables for explicitly modeling the transcriptomic and epigenetic signal variations between conditions and optimization against the noise in both scRNA-seq and scATAC-seq datasets. MAGICAL reconstructs regulatory circuits at cell-type resolution.

□ ISLET: individual-specific reference panel recovery improves cell-type-specific inference
>> https://genomebiology.biomedcentral.com/articles/10.1186/s13059-023-03014-8
ISLET (Individual Specific celL typE referencing Tool) estimates the cell-type-specific gene expression reference panel for each participant. The unobserved panel per subject are estimated by the expectation-maximization (EM) algorithm in a mixed-effect regression model.
ISLET leverages multiple or temporal observations of each subject, to construct a likelihood-based statistics for csDEG inference. This is the first statistical framework to recover the subject-level reference panel by employing multiple samples per subject.

□ vamos: variable-number tandem repeats annotation using efficient motif sets
>> https://genomebiology.biomedcentral.com/articles/10.1186/s13059-023-03010-y
VNTR Annotation Using Efficient Motifs Set (vamos) finds efficient motif sets using a reference panel of diversity genomes. StringDecomposer algorithm is integrated into vamos to annotate new genomes sequenced from aligned LRS reads or their assemblies using efficient motif sets.
vamos to create a combined VNTR callset across the HGSVC and HPRC assemblies to quantify diversity of VNTR sequences, and compared this to the diversity measured by a separate approach that combines calls based on merging similar variants.

□ Artificial intelligence-aided protein engineering: from topological data analysis to deep protein language models
>> https://arxiv.org/abs/2307.14587
Transformer-based protein language models provide new opportunities for building global evolutionary models. A variety of Transformer-based models have been developed such as evolutionary scale modeling (ESM), ProGen, ProteinBERT, Tranception and ESM-2.
Persistent hypergraph Laplacians enable the topological description of internal structures or organizations in data. Persistent hyperdigraph Laplacians further allow for the topological Laplacian modeling of directed hypergraphs
A similar algebraic topology structure is shared by persistent Hodge Laplacians and persistent Laplacians, but the former is a continuum theory for volumetric data and the latter is a discrete formulation for point cloud.

□ getphylo: rapid and automatic generation of multi-locus phylogentic trees from genbank files
>> https://www.biorxiv.org/content/10.1101/2023.07.26.550493v1
getphylo, a tool to automatically generate multi-locus phylogenetic trees from GenBank files. It has a low barrier to entry with minimal dependencies. getphylo uses a parallelised, heuristic workflow to keep runtime and system requirements as low as possible.
getphylo consistently produces trees with topologies comparable to other tools in less time. Furthermore, as getphylo does not rely on reference databases, it has a virtually unlimited scope in terms of taxonomy and genetic scale.

□ Gradient-based implementation of linear model outperforms deep learning models
>> https://www.biorxiv.org/content/10.1101/2023.07.29.551062v1
ZINB-Grad uses a scalable algorithm, reminiscent of alternating least squares, for fitting ZINB-WaVE models. In implementing this algorithm, it borrows the stochastic gradient descent-based model fitting machinery used in deep learning.
ZINB-Grad entropy of batch mixing is better than ZINB-WaVE and comparable to scVI performance. ZINB-Grad has biologically meaningful latent space performing as good as scVI4 and ZINB-WaVE regarding data imputation and accountability for technical variations.

□ COMPASS: joint copy number and mutation phylogeny reconstruction from amplicon single-cell sequencing data
>> https://www.nature.com/articles/s41467-023-40378-8
COMPASS (COpy number and Mutation Phylogeny from Amplicon Single-cell Sequencing), a probabilistic model and inference algorithm that can reconstruct the joint phylogeny of SNVs and CNAs from single-cell amplicon sequencing data.
COMPASS models amplicon-specific coverage fluctuations and that it can efficiently process high-throughput data of thousands of cells. COMPASS vastly outperforms BiTSC in settings where coverage variability resembles targeted scDNAseq.

□ GVP-MSA: Learning protein fitness landscapes with deep mutational scanning data from multiple sources
>> https://www.cell.com/cell-systems/fulltext/S2405-4712(23)00210-7
Geometric Vector Perceptron (GVP)-MSA, a deep learning network to learn the fitness landscapes, in which a 3D equivariant graph neural network was used to extract features from protein structure and a pre-trained model MSA Transformer was applied to embed MSA constraints.
Proof-of-concept trials are designed to validate this training scheme in three aspects: random and positional extrapolation for single-variant effects, zero-shot fitness predictions for new proteins, and extrapolation for higher-order variant effects from single-variant effects.

□ scASfind: Mining alternative splicing patterns in scRNA-seq data
>> https://www.biorxiv.org/content/10.1101/2023.08.19.553947v1
scASfind utilizes an efficient data structure to store the percent spliced-in value for each splicing event. This makes it possible to search for patterns among all differential splicing events, identify marker events, mutually exclusive events, and large blocks of exons.