


□ LDE: Latent-based Directed Evolution accelerated by Gradient Ascent for Protein Sequence Design
>> https://www.biorxiv.org/content/10.1101/2024.04.13.589381v1
LDE (Latent-based Directed Evolution), the first latent-based method for directed evolution. LDE learns to reconstruct and predict the fitness value of the input sequences in the form of a variational autoencoder (VAE) regularized by supervised signals.
LDE encodes a wide-type sequence into the latent representation, on which the gradient ascent is performed as an efficient offline MBO algorithm that guides the latent codes to reach high-fitness regions on the simulated landscape. LDE integrates latent-based directed evolution.
LDE involves iterative rounds of randomly adding scaled noise to the latent representations, facilitating local exploration around high-fitness regions. The noised latent representations are decoded into sequences and evaluated by the truth oracles.


□ Biological computations: limitations of attractor-based formalisms and the need for transients
>> https://arxiv.org/abs/2404.10369
The attractor-based framework provides an explanation for robustness (i.e. maintaining directional memory when the signal is disrupted) - adaptation to dynamic signals that vary over space and/or time, and thus processing of dynamic signals in real time.
An integrated framework that relies on transient quasi-stable dynamics could potentially enhance our understanding of how single cells actively process information. It could explain how they learn from their continuously changing environment to stabilize their phenotype.


□ CMC: An Efficient and Principled Model to Jointly Learn the Agnostic and Multifactorial Effect in Large-Scale Biological Data
>> https://www.biorxiv.org/content/10.1101/2024.04.12.589306v1
Under the guidance of maximum entropy, Conditional Multifactorial Contingency (CMC) aims to learn the joint probability distribution of each entry in the contingency tensor with the expectations of the margins along each dimension fixed to the observed values.
By applying the Lagrangian method, CMC obtained an unconstrained optimization problem with a much-reduced number of variables. The impact strengths of factors can be well depicted by Lagrange multipliers, which naturally emerge during the optimization process.
CMC avoids the NP-hard problem and results in a theoretically solvable convex problem. The CMC model estimates the distribution based on the marginal totals in each dimension. A marginal total is the sum of all entries corresponding to one index in one dimension.


□ Biology System Description Language (BiSDL): a modeling language for the design of multicellular synthetic biological systems
>> https://bmcbioinformatics.biomedcentral.com/articles/10.1186/s12859-024-05782-x
Biology System Description Language (BiSDL), a computational language for spatial, multicellular synthetic designs. The compiler manages the gap between this high-level biological semantics and the low-level Nets-Within-Nets (NWN) formalism syntax.
The NWN formalism is a high-level PN formalism supporting all features of other high-level PN: tokens of different types and timed and stochastic time delays associated with transitions.
BiSDL supports modularity, facilitating the creation of libraries for knowledge integration in the multicellular synthetic biology DBTL cycle. The TIMESCALE of a module sets the base pace of the system dynamics compared to the unitary step of the discrete-time simulator.

□ scGATE: Single-cell multi-omics analysis identifies context-specific gene regulatory gates and mechanisms
>> https://academic.oup.com/bib/article/25/3/bbae180/7655771
scGATE (single-cell gene regulatory gate), a novel computational tool for inferring TF–gene interaction networks and reconstructing Boolean logic gates
involving regulatory TFs using scRNA-seq data.
scGATE eliminates the need for individual formulations and likelihood calculations for each Boolean rule (e.g. AND, OR, XOR). scGATE applies a Bayesian framework to update prior probabilities based on the data and infers the most probable Boolean rule a posteriori.

□ Deep Lineage: Single-Cell Lineage Tracing and Fate Inference Using Deep Learning
>> https://www.biorxiv.org/content/10.1101/2024.04.25.591126v1
Deep Lineage uses lineage tracing and multi-timepoint scRNA-seq data to learn a robust model of a cellular trajectory such that gene expression and cell type information at different time points within that trajectory can be predicted.
Deep Lineage treats cells and their progenies within a clone as interconnected entities. Drawing inspiration from natural language processing, they conceptualize cellular relationships in terms of "clones" which represent cells ordered within a shared lineage and gene expression.
Deep Lineage uses LSTM, Bi-directional LSTM or Gated Recurrent Units (GRUs) to model complex sequential dependencies and temporal dynamics of a cellular trajectory. An autoencoder-learned embedding captures essential features of the data to simplify input to the LSTM.

□ NextDenovo: an efficient error correction and accurate assembly tool for noisy long reads
>> https://genomebiology.biomedcentral.com/articles/10.1186/s13059-024-03252-4
NextDenovo first detects the overlapping reads, then filters out the alignments caused by repeats, and finally splits the chimeric seeds based on the overlapping depth. NextDenovo employs the Kmer score chain (KSC) algorithm to perform the initial rough correction.
NextDenovo used a heuristic algorithm to detect these low-score regions (LSRs) during the traceback procedure within the KSC algorithm. For the LSRs, a more accurate algorithm, derived by combining the partial order alignment (POA) and KSC.
NextDenovo calculates dovetail alignments by two rounds of overlapping, constructs an assembly graph, removes transitive edges, tips, and generates contigs. Finally, NextDenovo maps all seeds to contigs and breaks a contig if it possesses low-quality regions.
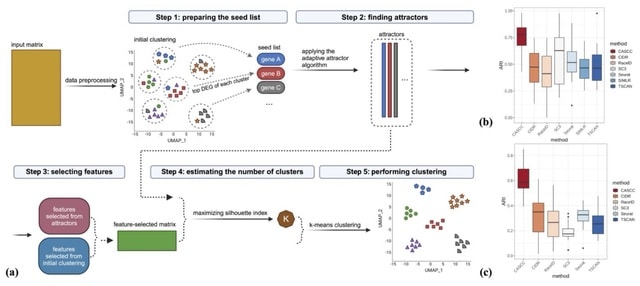
□ CASCC: a co-expression assisted single-cell RNA-seq data clustering method
>> https://academic.oup.com/bioinformatics/advance-article/doi/10.1093/bioinformatics/btae283/7658302
CASCC, a clustering method designed to improve biological accuracy using gene co-expression features identified using an unsupervised adaptive attractor algorithm. Briefly, the algorithm starts from a "seed" gene and converges to an "attractor" gene signature.
Each signature is defined by a list of ranked genes. Following an initial low computational complexity graph-based clustering, the top-ranked DEGs of each cluster are selected as features and as potential seeds used for the adaptive attractor method.
The final number of clusters, K, is determined based on the attractor output. Lastly, K-means clustering is performed on the feature-selected expression matrix, in which the cells with the highest expression levels of attractors are chosen as the initial cluster centers.

□ RiboDiffusion: Tertiary Structure-based RNA Inverse Folding with Generative Diffusion Models
>> https://arxiv.org/abs/2404.11199
RiboDiffusion, a generative diffusion model for RNA inverse folding based on tertiary structures. RoboDiffusion formulates the RNA inverse folding problem as learning the sequence distribution conditioned on fixed backbone structures, using a generative diffusion model.
RiboDiffusion captures multiple mappings from 3D structures to sequences through distribution learning. With a generative denoising process for sampling, RiboDiffusion iteratively transforms random initial RNA sequences into desired candidates under tertiary structure conditioning.

□ KMAP: Kmer Manifold Approximation and Projection for visualizing DNA sequences
>> https://www.biorxiv.org/content/10.1101/2024.04.12.589197v1
KMAP is based on the mathematical theories for describing the kmer manifold. They examined the probability distribution, introduced the concept of Hamming ball, and developed a motif discovery algorithm, such that we could sample relevant kmers to depict the full kmer manifold.
KMAP performs transformations to the kmer distances based on the kmer manifold theory to mitigate the inherent discrepancies between the kmer mmanifold and the 2D Euclidean space.

□ STREAMLINE: Topological benchmarking of algorithms to infer Gene Regulatory Networks from Single-Cell RNA-seq Data
>> https://academic.oup.com/bioinformatics/advance-article/doi/10.1093/bioinformatics/btae267/7646844
STREAMLINE is a refined benchmarking strategy for GRN Inference Algorithms that focuses on the preservation of topological graph properties as well as the identification of hubs.
The classes of networks we consider are Random, Small-World, Scale-Free, and Semi-Scale-Free Networks. Random or Erdös-Renyi networks include a set of nodes in which each node pair has the same probability of being connected by an edge.
SINCERITIES is a causality-based method that uses a linear regression model on temporal data, similar to Granger causality, which is known to have high false positive rates when its underlying assumptions are violated, as is the case in complex datasets with nonlinear dynamics.
SINCERITIES emerges as the top-performing algorithm for estimating the Average Shortest Path Length and produces more disassortative and centralized networks. This causes it to underestimate Assortativity and overestimate Centralization across all types of synthetic networks.

□ State-Space Systems as Dynamic Generative Models
>> https://arxiv.org/html/2404.08717v1
A probabilistic framework to study the dependence structure induced by deterministic discrete-time state-space systems between input and output processes.
Formulating general sufficient conditions under which solution processes exist and are unique once an input process has been fixed, which is the natural generalization of the deterministic echo state property.
State-space systems can induce a probabilistic dependence structure between input and output sequence spaces even without a functional relation between these two spaces.

□ Statistical learning for constrained functional parameters in infinite-dimensional models with applications in fair machine learning
>> https://arxiv.org/abs/2404.09847
A flexible framework for generating optimal prediction functions under a broad array of constraints. Learning a function-valued parameter of interest under the constraint that one or several pre-specified real-valued functional parameters equal zero or are otherwise bounded.
Characterizing the constrained functional parameter as the minimizer of a penalized risk criterion using a Lagrange multiplier formulation. It casts the constrained learning problem as an estimation problem for a constrained functional parameter in an infinite-dimensional model.
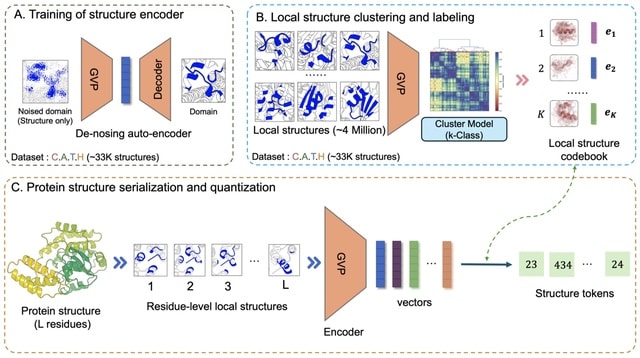
□ DeProt: A protein language model with quantizied structure and disentangled attention
>> https://www.biorxiv.org/content/10.1101/2024.04.15.589672v1
DeProt (Disentangled Protein sequence-structure model), a Transformer-based protein language model designed to incorporate protein sequences. DeProt can quantize protein structures to mitigate overfitting and is adeptly engineered to amalgamate sequence and structure tokens.

□ Nicheformer: a foundation model for single-cell and spatial omics
>> https://www.biorxiv.org/content/10.1101/2024.04.15.589472v1
Nicheformer is a transformer-based model pretrained on a large curated transcriptomics corpus of dissociated and spatially resolved single-cell assays containing more than 110 million cells, which they refer to as SpatialCorpus-110M.
Nicheformer uses a context length of 1,500 gene tokens serving as input for its transformer. The transformer block leverages 12 transformer encoder units 16,25 with 16 attention heads per layer and a feed-forward network size of 1,024 to generate a 512-dimensional embedding.


□ FCGR: Improved Python Package for DNA Sequence Encoding using Frequency Chaos Game Representation
>> https://www.biorxiv.org/content/10.1101/2024.04.14.589394v1
Frequency Chaos Game Representation (FCGR), an extended version of Chaos Game Representation (CGR), emerges as a robust strategy for DNA sequence encoding.
The core principle of the CGR algorithm involves mapping a one- dimensional sequence representation into a higher-dimensional space, typically in the two-dimensional spatial domain.
This package calculates FCGR using the actual frequency count of kmers, ensuring the accuracy of the resulting FCGR matrix. The accuracy of the FCGR matrix obtained from the R-based kaos package decreases significantly as the kmer length increases.


□ Long-read sequencing and optical mapping generates near T2T assemblies that resolves a centromeric translocation
>> https://www.nature.com/articles/s41598-024-59683-3
Constructing two sets of phased and non-phased de novo assemblies; (i) based on lrGS only and (ii) hybrid assemblies combining lrGS with optical mapping using lrGS reads with a median coverage of 34X.
Variant calling detected both structural variants (SVs) and small variants and the accuracy of the small variant calling was compared with those called with short-read genome sequencing (srGS).
The de novo and hybrid assemblies had high quality and contiguity with N50 of 62.85 Mb, enabling a near telomere to telomere assembly with less than a 100 contigs per haplotype. Notably, we successfully identified the centromeric breakpoint of the translocation.


□ Single Cell Atlas: a single-cell multi-omics human cell encyclopedia
>> https://genomebiology.biomedcentral.com/articles/10.1186/s13059-024-03246-2
Single Cell Atlas (SCA), a single-cell multi-omics map of human tissues, through a comprehensive characterization of molecular phenotypic variations across 125 healthy adult and fetal tissues and eight omics, incl. five single-cell (sc) omics modalities.
Single Cell Atlas includes 67,674,775 cells from scRNA-Seq, 1,607,924 cells from scATAC-Seq, 526,559 clonotypes from scImmune profiling, and 330,912 cells from multimodal scImmune profiling with scRNA-Seq, 95,021,025 cells from CyTOF, and 334,287,430 cells from flow cytometry.

□ spVC for the detection and interpretation of spatial gene expression variation
>> https://genomebiology.biomedcentral.com/articles/10.1186/s13059-024-03245-3
spVC integrates constant and spatially varying effects of cell/spot-level covariates, enabling a comprehensive exploration of how spatial locations and other covariates collectively contribute to gene expression variability.
spVC serves as a versatile tool for investigating diverse biological questions. Second, spVC offers statistical inference tools for each of the constant or spatially varying coefficient, providing a statistically principled approach to selecting different types of SVGs.
spVC can estimate the expected effect of spatial locations and other covariates on GE in the designated spatial domain. This additional layer of information facilitates the interpretation of identified SVGs, enhancing the ability to understand their functional implications.

□ CATD: a reproducible pipeline for selecting cell-type deconvolution methods across tissues
>> https://academic.oup.com/bioinformaticsadvances/advance-article/doi/10.1093/bioadv/vbae048/7634289
The critical assessment of transcriptomic deconvolution (CATD) pipeline encompasses functionalities for generating references and pseudo-bulks and running implemented deconvolution methods.
In the CATD pipeline , each scRNA-seq dataset is split in half into a training dataset, used as a 'reference input' for deconvolution, and a testing dataset that is utilized to generate pseudo-bulk mixtures to be deconvolved afterwards.

□ GradHC: Highly Reliable Gradual Hash-based Clustering for DNA Storage Systems
>> https://academic.oup.com/bioinformatics/advance-article/doi/10.1093/bioinformatics/btae274/7655853
Gradual Hash-based clustering (GradHC), a novel clustering approach for DNA storage systems. The primary strength of GradHC lies in its capability to cluster with excellent accuracy various types of designs, incl. varying strand lengths, cluster sizes, and different error ranges.
Given an input design (with potential similarity among different DNA strands), one can randomly choose a seed and use it to generate pseudo-random DNA strands matching the original design's length and input set size.
Each input strand is then XORed with its corresponding pseudo-random DNA strand, ensuring a high likelihood that the new strands are far from each other (in terms of edit distance) and do not contain repeated substrings across different input strands.
To retrieve the original data, pseudo-random strands are regenerated using the original seed and XORed with the received information. The scheme's redundancy is log(seed) = O(1), as only extra bits are needed for the seed value.

□ Binette: a fast and accurate bin refinement tool to construct high quality Metagenome Assembled Genomes.
>> https://www.biorxiv.org/content/10.1101/2024.04.20.585171v1
Binette is a Python reimplementation of the bin refinement module used in metaWRAP. It takes as input sets of bins generated by various binning tools. Using these input bin sets, Binette constructs new hybrid bins using basic set operations.
Specifically, a bin can be defined as a set of contigs, and when two or more bins share at least one contig, Binette generates new bins based on their intersection, difference, and union.

□ Mora: abundance aware metagenomic read re-assignment for disentangling similar strains
>> https://bmcbioinformatics.biomedcentral.com/articles/10.1186/s12859-024-05768-9
Mora, a tool that allows for sensitive yet efficient metagenomic read re-assignment and abundance calculation at the strain level for both long and short reads.
Given an alignment in SAM or BAM format and a set of reference strains, Mora calculates the abundance of each reference strain present in the sample and re-assigns the reads to the correct reference strain in a way such that abundance estimates are preserved.

□ Latent Schrödinger Bridge Diffusion Model for Generative Learning
>> https://arxiv.org/abs/2404.13309
A novel latent diffusion model rooted in the Schrödinger bridge. An SDE, defined over the time interval [0,1] is formulated to effectuate the transformation of the convolution distribution into the encoder target distribution within the latent space.
The model employs the Euler–Maruyama (EM) approach to discretize the SDE corresponding to the estimated score, thereby obtaining the desired samples by implementing the early stopping technique and the trained decoder.

□ OmicNavigator: open-source software for the exploration, visualization, and archival of omic studies
>> https://bmcbioinformatics.biomedcentral.com/articles/10.1186/s12859-024-05743-4
The OmicNavigator R package contains web application code, R functions for data deposition and retrieval, and a dedicated study container for the storage of measurements (e.g. RNAseq read counts), statistical analyses, metadata, and custom plotting functions.
Within OmicNavigator, a barcode plot is produced upon clicking a p-value within the enrichment results table. The interactive barcode, box and feature plot is produced using test result information from each feature within the selected term-test combination.

□ Variational Bayesian surrogate modelling with application to robust design optimisation
>> https://arxiv.org/abs/2404.14857
The non-Gaussian posterior is approximated by a simpler trial density with free variational parameters. They employed the stochastic gradient method to compute the variational parameters and other statistical model parameters by minimising the Kullback-Leibler (KL) divergence.
The proposed Reduced Dimension Variational Gaussian Process (RDVGP) surrogate is applied to illustrative and robust structural optimization problems where the cost functions depend on a weighted sum of the mean and standard deviation of model outputs.

□ ExpOmics: a comprehensive web platform empowering biologists with robust multi-omics data analysis capabilities
>> https://www.biorxiv.org/content/10.1101/2024.04.23.588859v1
ExpOmics offers robust multi-omics data analysis capabilities for exploring gene, mRNA/IncRNA, miRNA, circRNA, piNA, and protein expression data, covering various aspects of differential expression, co-expression, WGCNA, feature selection, and functional enrichment analysis.

□ OMIC: Orthogonal multimodality integration and clustering in single-cell data
>> https://bmcbioinformatics.biomedcentral.com/articles/10.1186/s12859-024-05773-y
OMIC (Orthogonal Multimodality Integration and Clustering) excels at modeling the relationships among multiple variables, facilitating scalable computation, and preserving accuracy in cell clustering compared to existing methods.

□ Mapping semantic space: Exploring the higher-order structure of word meaning
>> https://www.sciencedirect.com/science/article/pii/S0010027724000805
Multiple representation accounts of conceptual knowledge have emphasized the crucial importance of properties derived from multiple sources, such as social experience, and it is not clear how these fit together into a single conceptual space.
Exploring the organization of the semantic space underpinning concepts of all concreteness levels in a data-driven fashion in order to uncover latent factors among its multiple dimensions, and reveal where socialness fits within this space.

□ BTR: a bioinformatics tool recommendation system
>> https://academic.oup.com/bioinformatics/advance-article/doi/10.1093/bioinformatics/btae275/7658303
Bioinformatics Tool Recommendation system (BTR), a deep learning model designed to recommend suitable tools for a given workflow-in-progress. BTR represents the workflow as a directed graph, with a variant of the system constrained to employ linear sequence representations.
The methods of BTR are adapted for the tool recommendation problem based on the architecture of Session-based Recommendation with Graph Neural Networks (SR-GNN). BTR correctly outputs FeatureCounts as the highest-ranked tool from 1250+ choices.

□ Spherical Phenotype Clustering
>> https://www.biorxiv.org/content/10.1101/2024.04.19.590313v1
A non-parametric variant of contrastive learning incorporating the metadata. To use well metadata inside a contrastive setup, they pursue a scheme where the wells are represented as non-parametric class vectors.
This method optimizes the model with a contrastive loss adapted to compare images with the non-parametric well representations. The well representations are improved with a simple update rule. An approach of this type can be effective with over a million non-parametric vectors.
※コメント投稿者のブログIDはブログ作成者のみに通知されます