
□ Genomic language model: Deep learning of genomic contexts predicts protein co-regulation and function
>> https://www.biorxiv.org/content/10.1101/2023.04.07.536042v1
A genomic language model (gLM) learns contextualized protein embeddings that capture the genomic context as well as the protein sequence itself, and appears to encode biologically meaningful and functionally relevant information. gLM is learning co-regulated functional modules.
gLM is based on the transformer architecture. gLM is trained with the masked language modeling objective, with the hypothesis that its ability to attend to different parts of a multi-gene sequence will result in the learning of gene functional semantics and regulatory syntax.

□ GPN: DNA language models are powerful zero-shot predictors of genome-wide variant effects
>> https://www.biorxiv.org/content/10.1101/2022.08.22.504706v2
GPN’s internal representation of DNA sequences can distinguish genomic regions like introns, untranslated regions, and coding sequences. The confidence of GPN’s predictions can help reveal regulatory grammar.
GPN can be employed to calculate a pathogenicity or functionality score for any SNP in the genome using the log-likelihood ratio between the alternate and reference allele. GPN can learn from joint nucleotide distributions across all similar contexts appearing in the genome.
GPN uses the Hugging Face library to one-hot encode the masked DNA sequence and process it thru 25 convolutional blocks. Each block contains a dilated layer, feed-forward layer, intermediate residual connections, and layer normalization. The embedding is fixed at 512 dimensions.
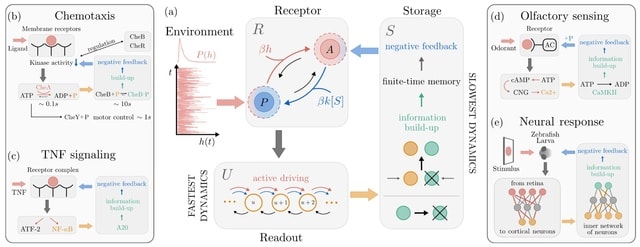
□ The architecture of information processing in biological systems
>> https://arxiv.org/abs/2301.12812
An archetypal model for sensing that starts from a thermodynamically consistent description. The combined effects of storage and negative feedback promote the emergence of a rich information dynamics shaped by adaptation and finite-time memory.
A chemical information reservoir for the system allows it to dynamically build up information on an external environment while reducing internal dissipation. Optimal sensing emerges from a dissipation-information trade-off, requires far-from-equilibrium in low-noise regimes.
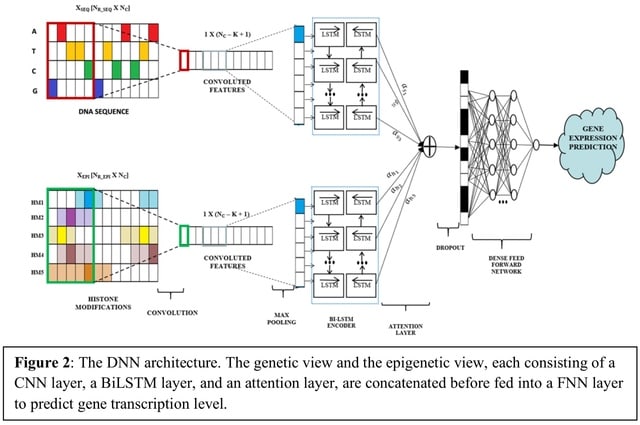
□ DeepCORE: An interpretable multi-view deep neural network model to detect co-operative regulatory elements
>> https://www.biorxiv.org/content/10.1101/2023.04.19.536807v1
DeepCORE uses a multi-view architecture to integrate genetic and epigenetic profiles in a DNN. It captures short-range and long-range interactions between REs through BiLSTM.
The learnt attention is a vector of length equal to the number of output nodes from the CNN layer containing importance score of each genomic region.
DeepCORE then joins the two views by concatenating the decoder outputs from each view and giving it to a fully connected feedforward neural network (FNN) to predict continuous gene transcription levels.
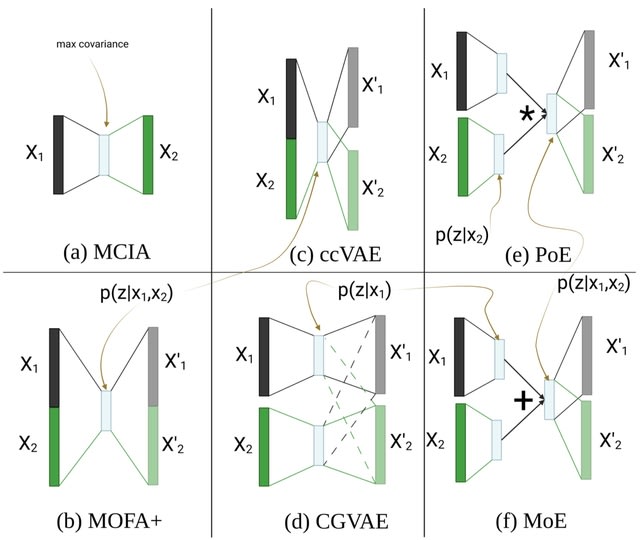
□ An in-depth comparison of linear and non-linear joint embedding methods for bulk and single-cell multi-omics
>> https://www.biorxiv.org/content/10.1101/2023.04.10.535672v1
Non-linear methods developed in other fields generally outperformed the linear and simple non-linear ones at imputing missing modalities. CGVAE and ccVAE did better than PoE and MoE on both bulk and single-cell data, while they typically underperformed in the other tasks.
ccVAE uses a single encoder for the concatenation of both modalities, which might be beneficial for generation coherence, as the latent space is directly and concurrently influenced by matched samples from all modalities.
The architecture of CGVAE is identical to that of MoE and PoE with separate encoders per modality. MOFA+ has the advantage that it provides useful diagnostic messages about the input data as well as the learnt space.

□ Genotyping variants at population scale using DRAGEN gVCF Genotyper
>> https://www.illumina.com/science/genomics-research/articles/gVCF-Genotyper.html
DRAGEN gVCF Genotyper implements an iterative workflow to add new samples to an existing cohort. This workflow allows users to efficiently combine new batches of samples with existing batches without repeated processing.
DRAGEN gVCF Genotyper computes many variant metrics on the fly, among them allele counts. DRAGEN gVCF Genotyper relies on the gVCF input format, which contains both variant information, like a VCF, and a measure of confidence of a variant not existing at a given position.
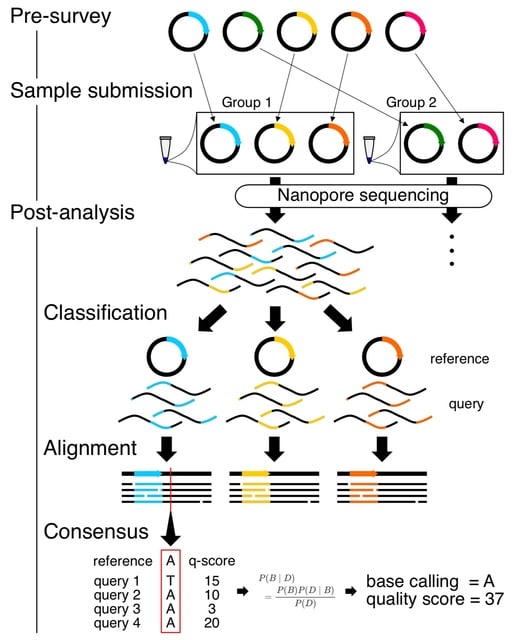
□ SAVEMONEY: Barcode-free multiplex plasmid sequencing using Bayesian analysis and nanopore sequencing
>> https://www.biorxiv.org/content/10.1101/2023.04.12.536413v1
SAVEMONEY (Simple Algorithm for Very Efficient Multiplexing of Oxford Nanopore Experiments for You) guides researchers to mix multiple plasmids and subsequently computationally de-mixes the resultant sequences.
SAVEMONEY involves submitting samples with multiple different plasmids mix and deconvolving the obtained sequencing results while maintaining the quality of the analysis. SAVEMONEY leverages plasmid maps, which are in most cases already made prior to plasmid construction.
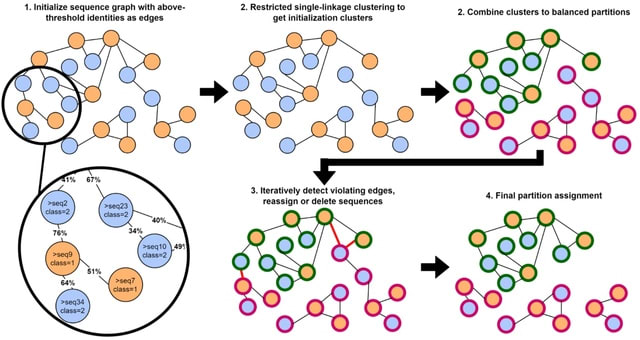
□ GraphPart: Homology partitioning for biological sequence analysis
>> https://www.biorxiv.org/content/10.1101/2023.04.14.536886v1
GraphPart, an algorithm for homology partitioning, where as many sequences as possible are kept in the dataset, but partitions are defined such that closely related sequences always end up in the same partition.
GraphPart operates on real-valued distance metrics. Sequence identities ranging from 0 to 1 are converted to distances as d(a,b) = 1-identity(a,b). The partitioning threshold undergoes the same conversion. GraphPart can accept any similarity metric and skip the alignment step.
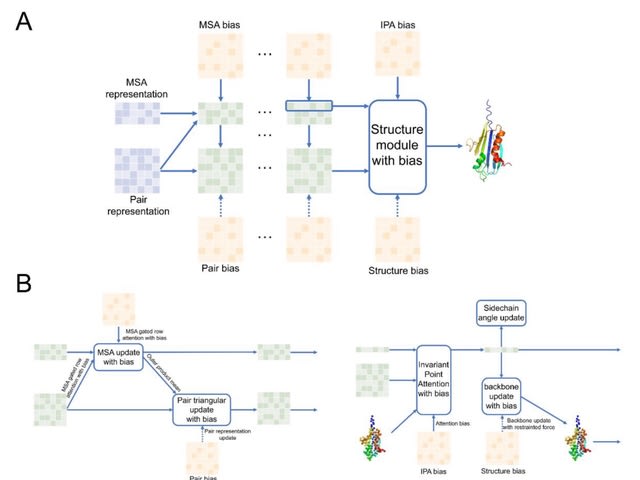
□ RASP / FAAST: Assisting and Accelerating NMR Assignment with Restrainted Structure Prediction
>> https://www.biorxiv.org/content/10.1101/2023.04.14.536890v1
RASP (Restraints Assisted Structure Predictor) is an architecture derived from AlphaFold evoformer and structure module, and it accepts abstract or experimental restraints, sparse or dense, to generate structures.
FAAST(iterative Folding Assisted peak ASsignmenT) is an iterative NMR NOESY peak assignment pipeline. Using chemical shift and NOE peak lists as input, FAAST assigns NOE peaks iteratively and generates a structure ensemble.

□ Emergent autonomous scientific research capabilities of large language models
>> https://arxiv.org/abs/2304.05332
An Intelligent Agent system that combines multiple large language models for autonomous design/planning/execution. The Agent's scientific research capabilities with 3 distinct examples, with the most complex being the successful performance of catalyzed cross-coupling reactions.
The Agent calculates the required volumes of all reactants and writes the protocol. Subsequent GC-MS analysis of the reaction mixtures revealed the formation of the target products for both reactions. Agent corrects its own code based on the automatically generated outputs.

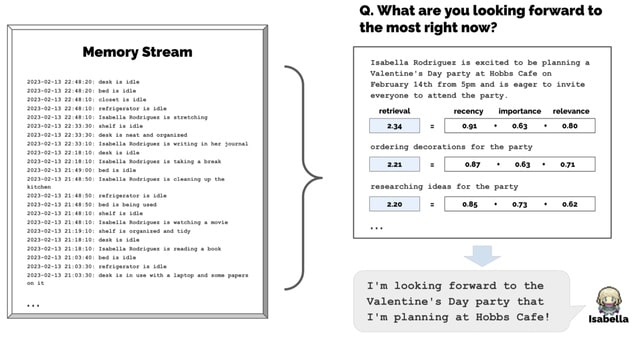
□ Generative Agents: Interactive Simulacra of Human Behavior
>> https://arxiv.org/abs/2304.03442
Generative agents wake up, cook breakfast, and head to work; artists paint, while authors write; they form opinions, notice each other, and initiate conversations; they remember and reflect on days past as they plan the next day.
An architecture that extends a large language model to store a complete record of the agent's experiences using natural language, synthesize those memories over time into higher-level reflections, and retrieve them dynamically to plan behavior.
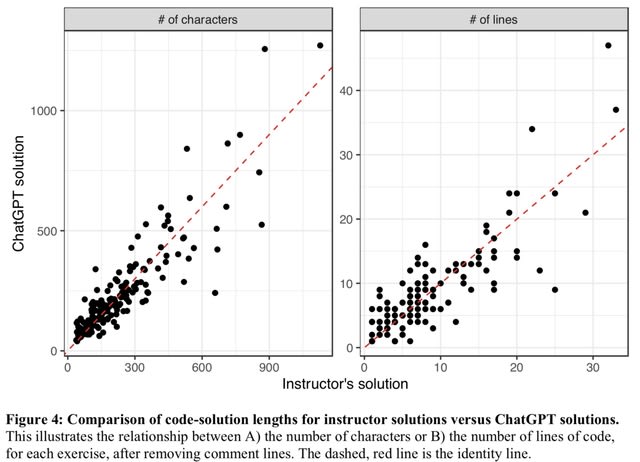
□ Many bioinformatics programming tasks can be automated with ChatGPT
>> https://arxiv.org/abs/2303.13528
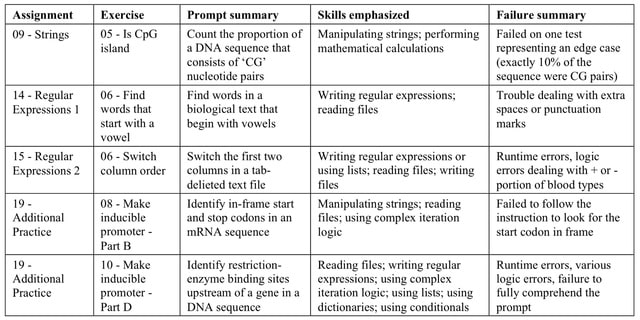
ChatGPT failed to solve 5 of the exercises within 10 attempts. This table summarizes characteristics of these exercises and provides a brief summary of complications that ChatGPT faced when attempting to solve them.
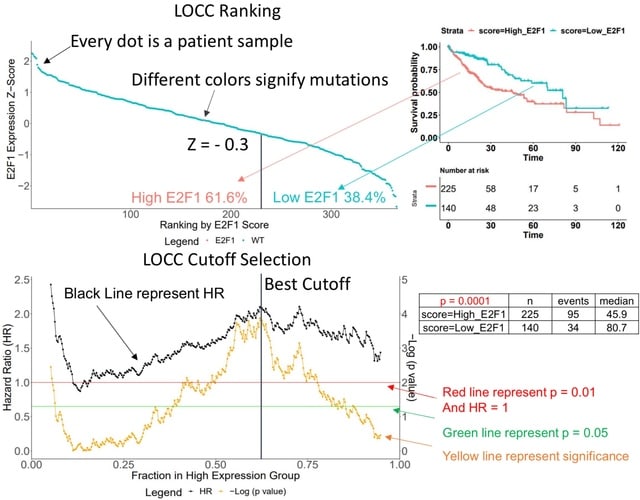
□ LOCC: a novel visualization and scoring of cutoffs for continuous variables
>> https://www.biorxiv.org/content/10.1101/2023.04.11.536461v1
Luo’s Optimization Categorization Curves (LOCC) helps visualize more information for better cutoff selection and understanding of the importance of the continuous variable against the measured outcome.
The LOCC score is made of three numeric components: a significance aspect, a range aspect, and an impact aspect. The higher the LOCC score, the more critical and predictive the expression is for prognosis.
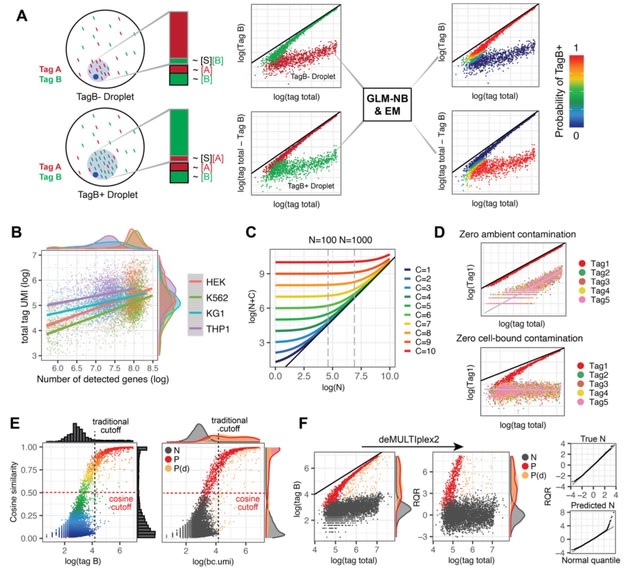
□ Demultiplex2: robust sample demultiplexing for scRNA-seq
>> https://www.biorxiv.org/content/10.1101/2023.04.11.536275v1
deMULTIplex2, a mechanism-guided classification algorithm for multiplexed scRNA-seq data that successfully recovers many more cells across a spectrum of challenging datasets compared to existing methods.
deMULTIplex2 is built on a statistical model of tag read counts derived from the physical mechanism of tag cross-contamination. Using GLM and expectation-maximization, deMULTIplex2 probabilistically infers the sample identity of each cell and classifies singlets w/ high accuracy.
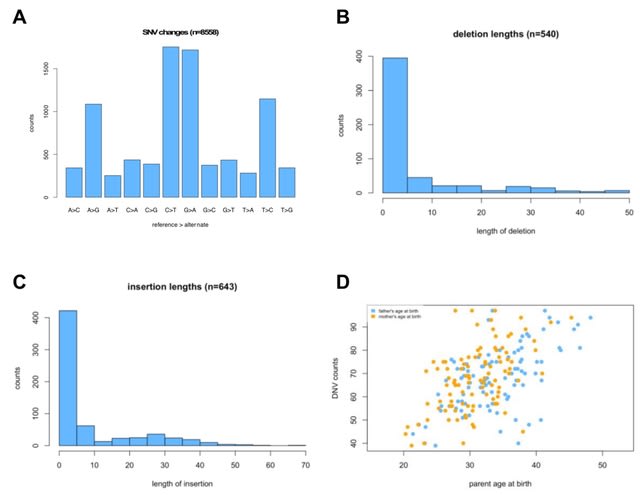
□ acorn: an R package for de novo variant analysis
>> https://www.biorxiv.org/content/10.1101/2023.04.11.536422v1
Acorn is an R package that works with de novo variants (DNVs) already called using a DNV caller. The toolkit is useful for extracting different types of DNVs and summarizing characteristics of the DNVs.
Acorn consists of several functions to analyze DNVs. readDNV reads in DNV data and turns it into an R object for use with other functions within acorn. Acorn fills a gap in genomic DNV analyses between the calling of DNVs and ultimate downstream statistical assessment.
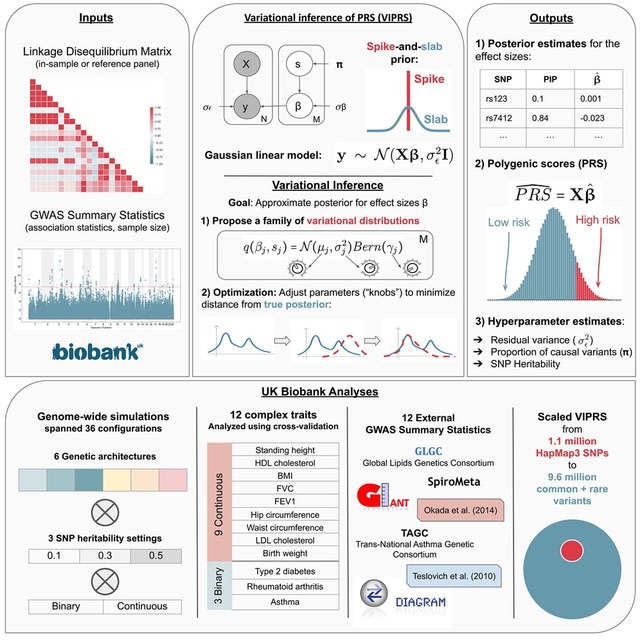
□ VIPRS: Fast and accurate Bayesian polygenic risk modeling with variational inference
>> https://www.cell.com/ajhg/fulltext/S0002-9297(23)00093-9
VIPRS, a Bayesian summary statistics-based PRS method that utilizes variational inference techniques to approximate the posterior distribution for the effect sizes.
VIPRS is consistently competitive w/ the state-of-the-art in prediction accuracy while being more than twice as fast as popular MCMC-based approaches. This performance advantage is robust across a variety of genetic architectures, SNP heritabilities, and independent GWAS cohorts.
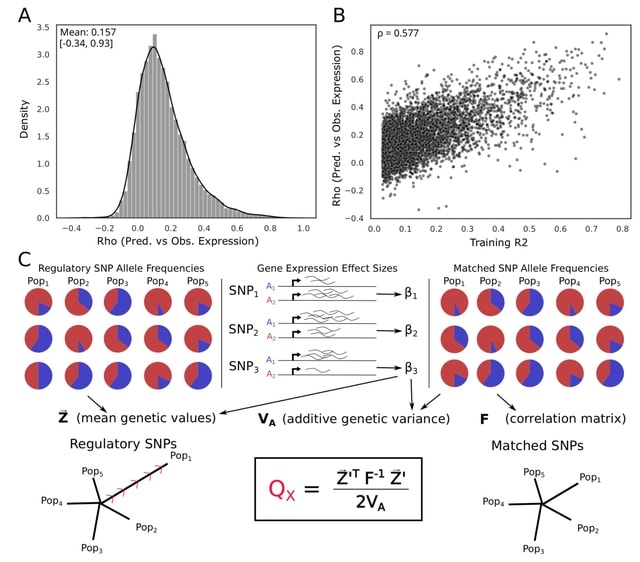
□ A gene-level test for directional selection on gene expression
>> https://academic.oup.com/genetics/advance-article/doi/10.1093/genetics/iyad060/7111744
Applying The QX test for polygenic selection to regulatory variants identified using Joint-tissue Imputation (JTI) models to test for population-specific selection on gene regulation in 26 human populations.
The gamma-corrected approach was uniformly more powerful than the permutation approach. Indeed, while the gamma-corrected test approaches a power of 1.0 under regimes with stronger selection, the effect-permuted version never reached that.
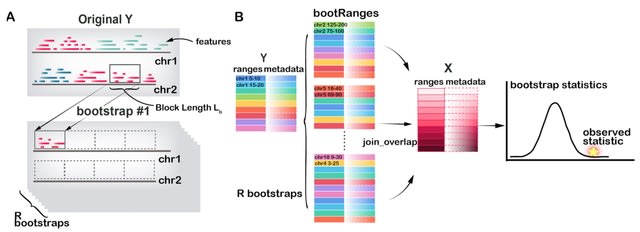
□ bootRanges: Flexible generation of null sets of genomic ranges for hypothesis testing
>> https://academic.oup.com/bioinformatics/advance-article/doi/10.1093/bioinformatics/btad190/7115835
bootRanges provides fast functions for generation of block bootstrapped genomic ranges representing the null hypothesis in enrichment analysis. bootRanges offers greater flexibility for computing various test statistics leveraging other Bioconductor packages.
Shuffling/permutation schemes may result in overly narrow test statistic null distributions and over-estimation of statistical significance, while creating new range sets w/ a block bootstrap preserves local genomic correlation structure and generates reliable null distributions.
189,900-bases sequenced:1,658 bases inserted,937 bases deleted,248 SNVs,4,825 CpG methylations.One read.What you're missing matters... find out how nanopore sequencing provides the most comprehensive view of variants in the cancer genome: https://t.co/wheVdgMbmq #AACR23 pic.twitter.com/fULOtSX4tb
— Oxford Nanopore (@nanopore) April 13, 2023
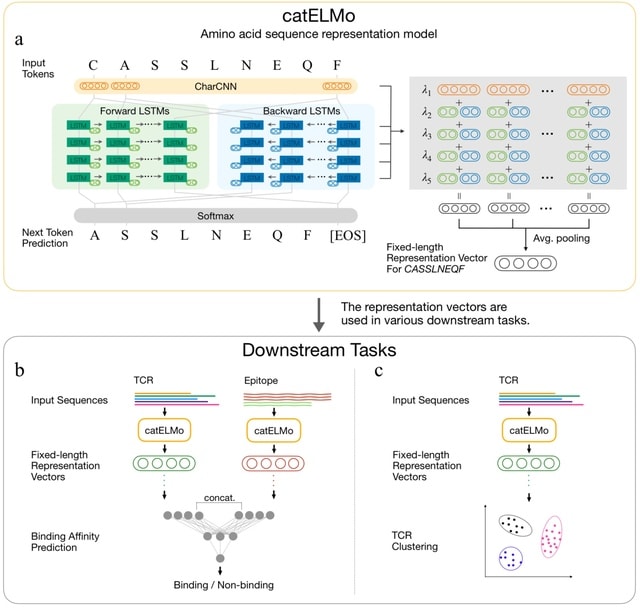
□ catELMo: Context-Aware Amino Acid Embedding Advances Analysis of TCR-Epitope Interactions
>> https://www.biorxiv.org/content/10.1101/2023.04.12.536635v1
catELMo, whose architecture is adapted from ELMo (Embeddings from Language Models), a bi-directional context-aware language model. catELMo consists of a charCNN layer and four bidirectional LSTM layers followed by a softmax activation.
catELMo is trained on more than four million TCR sequences collected from ImmunoSEQ in an unsupervised manner, by contextualizing amino acid inputs and predicting the next amino acid token.
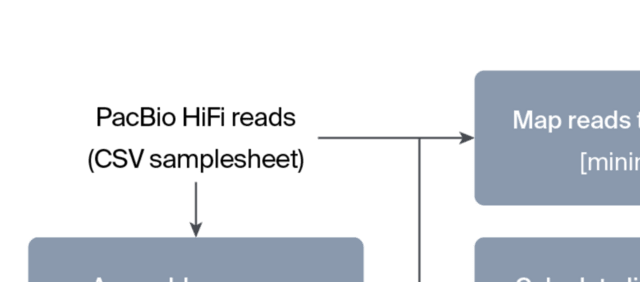
□ Streamlining PacBio HiFi assembly and QC with the hifi2genome workflow
>> https://research.arcadiascience.com/pub/resource-hifi2genome
hifi2genome assembles PacBio HiFi reads from a single organism and produce quality control statistics for the resulting assembly. The product of this pipeline is an assembly, mapped reads, and interactive visualizations reported with MultiQC.
hifi2genome uses Flye to assemble PacBio HiFi reads into contigs, followed by parallel processing steps for generating QC statistics. These steps include assembly QC stats with QUAST, lineage-specific QC stats with BUSCO, and mapping stats using SAMtools and minimap2.
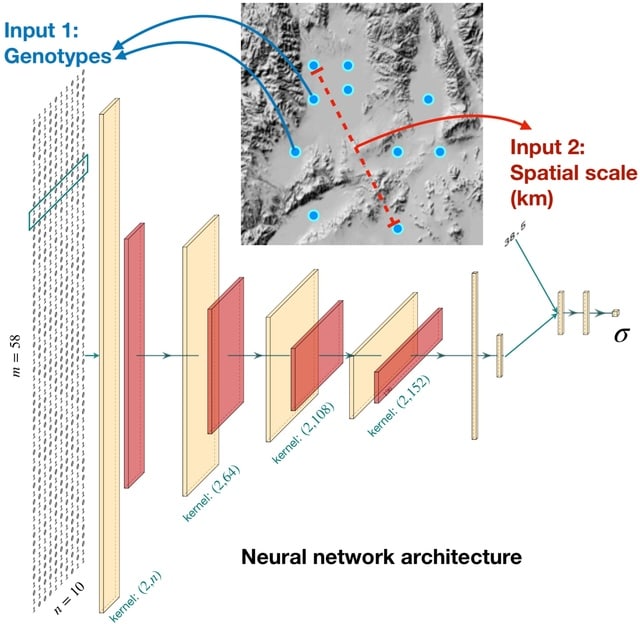
□ disperseNN: Dispersal inference from population genetic variation using a convolutional neural network
>> https://academic.oup.com/genetics/advance-article/doi/10.1093/genetics/iyad068/7117621
disperseNN uses forward in time spatial genetic simulationsto train a deep neural network to infer the mean, per-generation dispersal distance from a single population sample of single nucleotide polymorphism (SNP) genotypes, e.g., whole genome data or RADseq data.
disperseNN predicts σ from full-spatial test data after simulations w/ 100 generations. Using successive layers of data compression, through convolution / pooling, to coerce disperseNN to look at the genotypes at different scales and learn the extent of linkage disequilibrium.
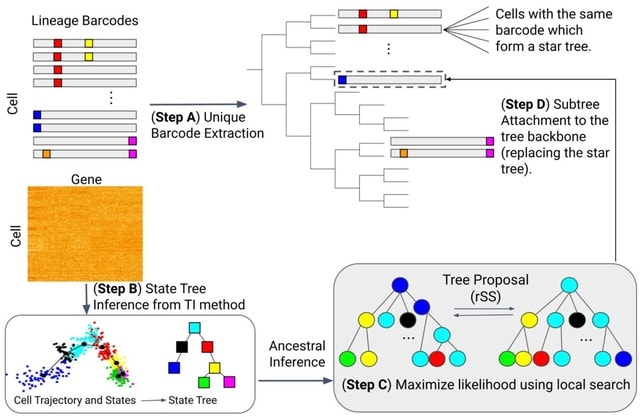
□ LinRace: single cell lineage reconstruction using paired lineage barcode and gene expression data
>> https://www.biorxiv.org/content/10.1101/2023.04.12.536601v1
LinRace (Lineage Reconstruction w/ asymmetric cell division model), that integrates the lineage barcode and gene expression data using the asymmetric cell division model and infers cell lineage under a framework combining Neighbor Joining and maximum-likelihood heuristics.
LinRace outputs more accurate cell division trees than existing methods for lineage reconstruction. Moreover, LinRace can output the cell states (cell types) of ancestral cells, which is rarely performed with existing lineage reconstruction methods.
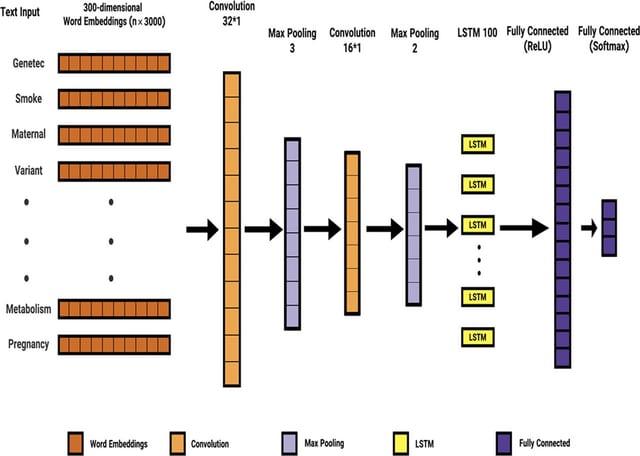
□ Automatic extraction of ranked SNP-phenotype associations from text using a BERT-LSTM-based method
>> https://bmcbioinformatics.biomedcentral.com/articles/10.1186/s12859-023-05236-w
Transforms do not embed positional information as they do in recurrent models; however, they still embody positional information in modeling sentence order. Early stopping is a regularization technique to prevent over fitting when learning something iteratively.
Although the used linguist features could be employed to implement a superior association extraction method outperforming the kernel-based counterparts, the used BERT-CNN-LSTM-based methods exhibited the best performance.
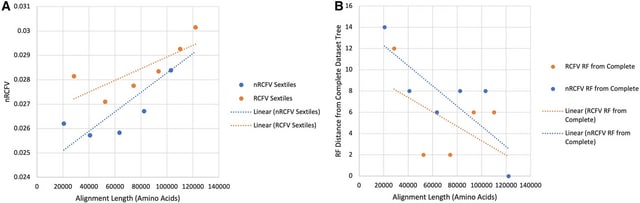
□ nRCFV: a new, dataset-size-independent metric to quantify compositional heterogeneity in nucleotide and amino acid datasets
>> https://bmcbioinformatics.biomedcentral.com/articles/10.1186/s12859-023-05270-8
nRCFV, a truly normalised Relative Compositional Frequency Variation value. This new metrics add a normalisation constant to each of the different RCFV values (total, character-specific, taxon-specific) to mitigate the effect of increasing taxa number and sequence length.
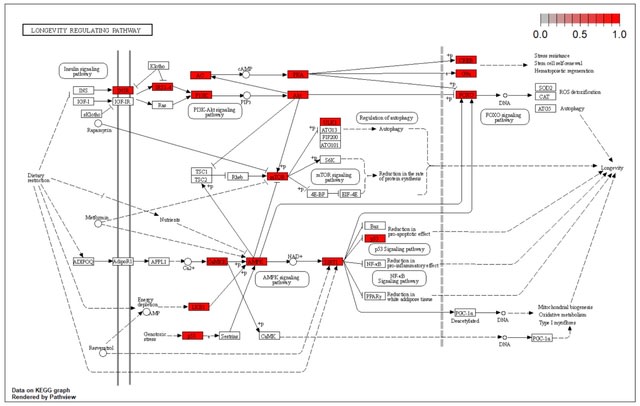
□ Wearable-ome meets epigenome: A novel approach to measuring biological age with wearable devices.
>> https://www.biorxiv.org/content/10.1101/2023.04.11.536462v1
Aging is a dynamic process and simply utilizing chronological age as a predictor of All-Cause Mortality and disease onset is insufficient. Instead, measuring the organismal state of function, biological age, may provide greater insight.

□ PhenoCellPy: A Python package for biological cell behavior modeling
>> https://www.biorxiv.org/content/10.1101/2023.04.12.535625v1
PhenoCellPy defines Python classes for the Cell Volume (which it subdivides between the cytoplasm and nucleus) and its evolution, the state of the cell and the behaviors the cell displays in each state (called the Phase), and the sequence of behaviors (called the Phenotype).
PhenoCellPy’s can extend existing modeling frameworks as an embedded model. It integrates with the frameworks by defining the cell states (phases), signaling when a state change occurs, if division occurs, and by receiving information from the framework.
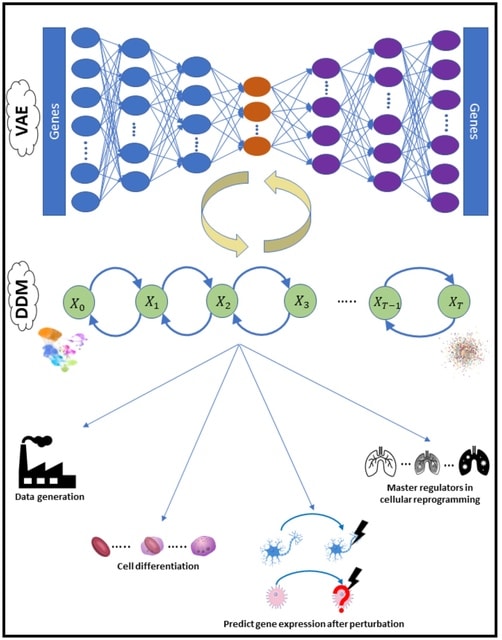
□ scVAEDer: The Power of Two: integrating deep diffusion models and variational autoencoders for single-cell transcriptomics analysis
>> https://www.biorxiv.org/content/10.1101/2023.04.13.536789v1
scVAEDer, a scalable deep-learning model that combines the power of variational autoencoders and deep diffusion models to learn a meaningful representation which can capture both global semantics and local variations in the data.
scVAEDer combes the strengths of VAEs and Denoising Diffusion Models (DDMs). It incorporates both VAE and DDM priors to more precisely capture the distribution of latent encodings in the data. By using vector arithmetic in the DDM space scVAEDer outperforms SOTA methods.
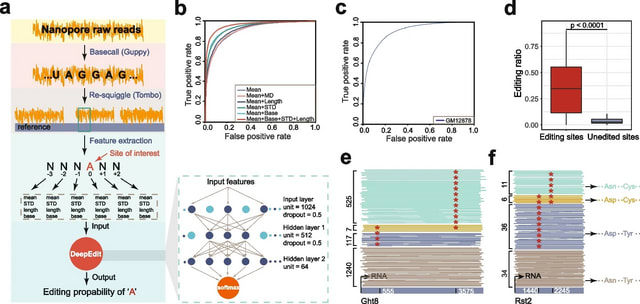
□ DeepEdit: single-molecule detection and phasing of A-to-I RNA editing events using nanopore direct RNA sequencing
>> https://genomebiology.biomedcentral.com/articles/10.1186/s13059-023-02921-0
DeepEdit can identify A-to-I editing events on single nanopore reads and determine the phasing information on transcripts through nanopore direct RNA sequencing.
DeepEdit is a fully connected neural network model which takes advantage of the raw electrical signal features flanking the editing sites. A total of 40,823 I-type reads from FY-ADAR2 and randomly chosen 47,757 HFF1 reads were used as the positive and negative controls.

□ GBC: a parallel toolkit based on highly addressable byte-encoding blocks for extremely large-scale genotypes of species
>> https://genomebiology.biomedcentral.com/articles/10.1186/s13059-023-02906-z
Genotype Block Compressor (GBC) manages genotypes in Genotype Block (GTB). GTB is a unified data structure to store large-scale genotypes into many highly addressable byte-encoding compression blocks. Then, multiple advanced algorithms were developed for efficient compression.
The AMDO (approximate minimum discrepancy ordering) algorithm is applied on the variant level to sort the variants with similar genotype distributions for improving the compression ratio. The ZSTD algorithm is then adopted to compress the sorted data in each block.
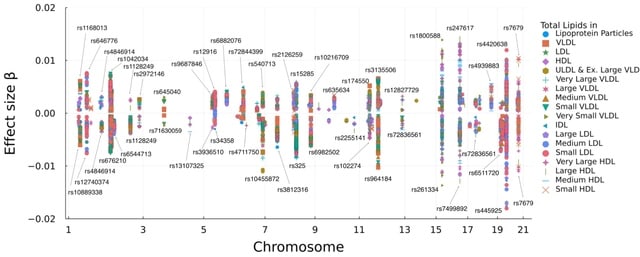
□ Multivariate Genome-wide Association Analysis by Iterative Hard Thresholding
>> https://academic.oup.com/bioinformatics/advance-article/doi/10.1093/bioinformatics/btad193/7126408
Multivariate IHT for analyzing multiple correlated traits. In simulation studies, multivariate IHT exhibits similar true positive rates, significantly lower false positive rates, and better overall speed than linear mixed models and canonical correlation analysis.
In IHT the most computationally intensive operations are the matrix-vector and matrix- matrix multiplications required in computing gradients. To accelerate these operations, SIMD (single instruction, multiple data) is employed for vectorization and tiling.

□ moslin: Mapping lineage-traced cells across time points
>> https://www.biorxiv.org/content/10.1101/2023.04.14.536867v1
moslin, a Fused Gromov-Wasserstein-based model to couple matching cellular profiles across time points. moslin leverages both intra-individual lineage relations and inter-individual gene expression similarity.
moslin uses lineage information at two or more time points and to include the effects of cellular growth and stochastic cell sampling. The algorithm combines gene expression with lineage information at all time points to reconstruct precise differentiation trajectories.
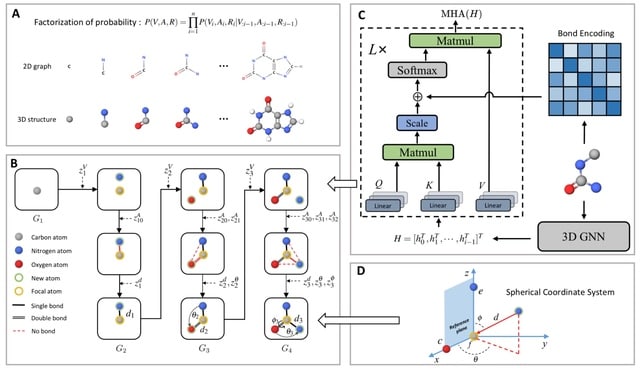
□ MolCode: An Equivariant Generative Framework for Molecular Graph-Structure Co-Design
>> https://www.biorxiv.org/content/10.1101/2023.04.13.536803v1
MolCode, a roto-translation equivariant generative framework for Molecular graph-structure Co-design. In MolCode, 3D geometric information empowers the molecular 2D graph generation, which in turn helps guide the prediction of molecular 3D structure.
MolCode not only consistently generates valid and diverse molecular graphs/structures with desirable properties, but also generate drug-like molecules with high affinity to target proteins, which demonstrates MolCode’s potential applications in material design and drug discovery.
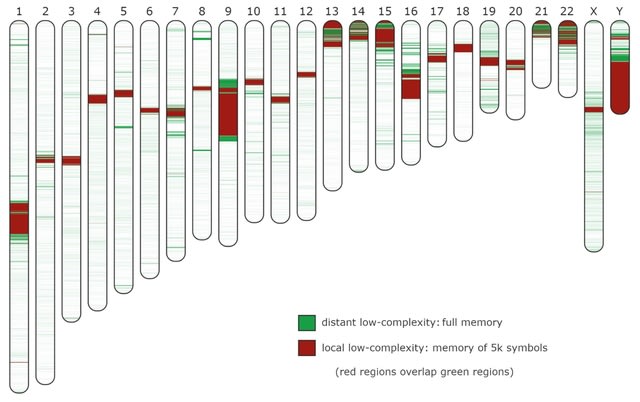
□ AlcoR: alignment-free simulation, mapping, and visualization of low-complexity regions in biological data
>> https://www.biorxiv.org/content/10.1101/2023.04.17.537157v1
AlcoR addresses the challenge of automatically modeling and distinguishing LCRs. AlcoR enables the use of models with different memories, providing the ability to distinguish local from distant low-complexity patterns.
AlcoR is reference- and alignment-free, providing additional methodologies for testing, incl. a highly-flexible simulation method for generating biological sequences with different complexity levels, sequence masking, and a automatic computation of the LCR maps into ideogram.
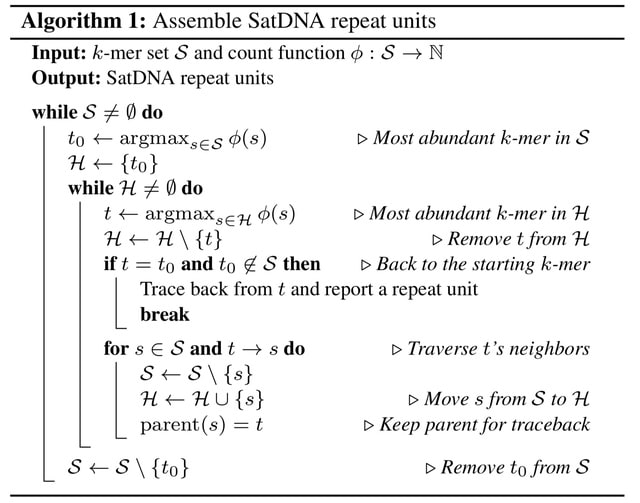
□ De novo reconstruction of satellite repeat units from sequence data
>> https://arxiv.org/abs/2304.09729
Satellite Repeat Finder (SRF), a de novo assembler for reconstructing SatDNA repeat units and can identify most known HORs and SatDNA in well-studied species without prior knowledge on monomer sequences or repeat structures.
SRF uses a greedy algorithm to assemble SatDNA repeat units, but may miss the lower abundance and higher diversity unit when sharing long similar sequences. SRF may reconstruct repeat units similar in sequence. The similar repeat units may be mapped the same genomic locus.