Emergence is the semantic analogy of "figure and ground" in the context of complex natural phenomena.
言語の構造性は「転がり続けるボール」ではなく、むしろ重力場そのものに近い。我々は断片的に言語化された島々を渡るだけだ。暗礁を見ずして陸続きと思い込んではならない。
論理ベースで意思決定することを「抽象的だ」「一貫性がない」と批判する場合は極めて慎重になるべきだ。行動原理の無謬性はしばしば縮小均衡を伴う為、不確定要素に対し論理的飛躍を試るのが仮説ドリヴンだが、この両者は背反しない。仮説と論理の対立は言語レベルにおける瑕疵であり、ドグマが在る必要はない。

□ SIS-seq, a molecular 'time machine', connects single cell fate with gene programs:
>> https://www.biorxiv.org/content/biorxiv/early/2018/08/29/403113.full.pdf
a clonal method SIS-seq, whereby single cells are allowed to divide, and progeny cells are assayed separately in SISter conditions; some for fate, others by RNA-seq. A time machine could conceivably allow one to first ascertain one feature of a given cell, then go back in time and re-test the same cell for the other feature, allowing cross-comparison of the two.
This is reminiscent of the challenge in quantum mechanics where a particle’s momentum and location cannot be simultaneously known (the popular interpretation of the ‘Heisenberg uncertainty principle’).
□ An accurate and rapid continuous wavelet Dynamic Time Warping algorithm for end-to-end mapping in ultra-long nanopore sequencing:
>> https://academic.oup.com/bioinformatics/article/34/17/i722/5093233
The warping path obtained from a coarser resolution is used to obtain a stable and narrow context-dependent boundary to constrain the warping path at a refiner resolution.
Continuous Wavelet Dynamic Time Warping:
the total runtime of Algorithm
TIME(DTW)+2·(TIME(cDTW)+TIME(ReMapIndex))+L·TIME(CWT)
, which gives the time- and memory-complexity of O(1/zN^2+2rN+LN)
, where z=(2^(L−1)k)^2 and r, L ≪ N.

□ State space reconstruction of spatially extended systems and of time delayed systems from the time series of a scalar variable:
>> https://aip.scitation.org/doi/full/10.1063/1.5023485
Time-delayed systems are infinite-dimensional systems because, in order to obtain a solution, one needs to specify as initial condition a function in the interval [−τ,0]. the high dimensional systems are one-dimensional spatially extended systems (1D SESs) and time delayed systems (TDSs). the state space reconstruction of these systems, from the time series of one scalar “observed” variable. In a space-time representation, these systems show similar phenomena (wave propagation, pattern formation, defects/dislocations, turbulence...). their dynamics can be reconstructed in a 3-dimensional pseudo-space, where the evolution is governed by the same polynomial potential.

□ VISION: Functional Interpretation of Single-Cell Similarity Maps:
>> https://www.biorxiv.org/content/biorxiv/early/2018/08/29/403055.full.pdf
VISION is designed to naturally operate inside of analysis pipelines, where it fits downstream of any method for manifold learning, clustering, or trajectory inference and provides functional interpretation of their output. Importantly, VISION also enables the exploration of the transcriptional effects of meta-data, including cell-level and sample-level. VISION operates directly on the manifold of cell-cell similarity and employs a flexible annotation approach that can operate either with or without preconceived stratification of the cells into groups or along a continuum.

□ GeneQC: A New Machine Learning-Based Framework for Mapping Uncertainty Analysis in RNA-Seq Read Alignment and Gene Expression Estimation:
>> http://bmbl.sdstate.edu/GeneQC/home.html
The underlying algorithm is designed based on extracted genomic and transcriptomic features, which are then combined using elastic-net regularization and mixture model fitting to provide a clearer picture of mapping uncertainty for each gene. GeneQC also enables researchers to investigate continued re-alignment methods to determine more accurate gene expression estimates for those with low reliability.
□ Accurate error control in high dimensional association testing using conditional false discovery rates:
>> https://www.biorxiv.org/content/biorxiv/early/2018/09/12/414318.full.pdf
This new methods essentially involve computing an analogy of the p-value (broadly ‘the probability under H0P of an observed cFDR value being less than that observed’) and then using the Benjamini-Hochberg procedure. In alternative models of association such as the ‘universal pleiotropy hypothesis’, common in random-effects models, use of cFDR only changes the order in which associations are discovered, since all associations are eventually discovered as sample sizes tend to infinity.
□ Correcting the Mean-Variance Dependency for Differential Variability Testing Using Single-Cell RNA Sequencing Data
>> https://www.cell.com/cell-systems/fulltext/S2405-4712(18)30278-3
an analysis approach that extends the BASiCS statistical framework to derive a residual measure of variability that is not confounded by mean expression. This includes a robust procedure for quantifying technical noise in experiments where technical spike-in molecules are not available. This method provides biological insight into the dynamics of cell-to-cell expression variability, highlighting a synchronization of biosynthetic machinery components in immune cells upon activation.
□ NovoGraph: Genome graph construction from multiple long-read de novo assemblies:
>> https://f1000research.com/articles/7-1391/v1
As long-read sequencing becomes more cost-effective and enables de novo assembly for increasing numbers of whole genomes, a method for the direct construction of a genome graph from sets of assembled human genomes would be desirable. Such assembly-based genome graphs would encompass the wide spectrum of genetic variation accessible to long-read-based de novo assembly, including large structural variants and divergent haplotypes.

□ Long read club:
>> http://longreadclub.org
Long read club is launching … as part of a recently funded Wellcome Trust Technology Development Award.
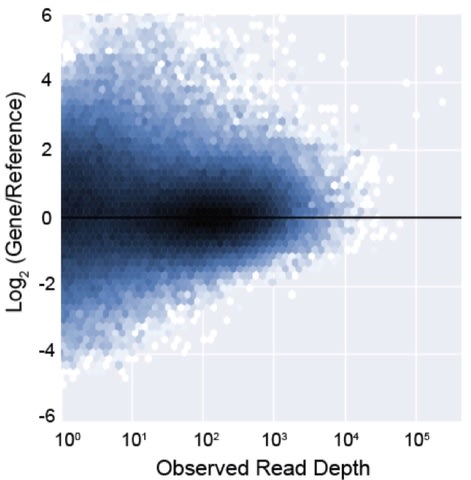
□ CNVkit-RNA: Copy number inference from RNA-Sequencing data:
>> https://www.biorxiv.org/content/biorxiv/early/2018/09/04/408534.full.pdf
CNVkit removes technical variation in gene expression associated with GC-content and transcript length. CNVkit assigns a weight, dictated by several variables, to each transcript with the net effect of preferentially inferring copy number from highly and stably expressed genes. In particular, this will be useful for single-cell RNA-sequencing studies because it is challenging to extract DNA and RNA from the same cell. Overall, CNVkit makes it possible to collect genotype- and phenotype- information from a single assay.

□ Scalable Nonlinear Programming Framework for Parameter Estimation in Dynamic Biological System Models:
>> https://www.biorxiv.org/content/biorxiv/early/2018/09/07/410688.full.pdf
a nonlinear programming (NLP) framework for solving estimation problems w/ embedded dynamic models. The framework is based on a direct transcription approach wherein the dynamic model is converted into a large set of algebraic equations by applying time-discretization techniques. The algebraic equations are then embedded directly as constraints in the optimization problem. The NLPs arising from time discretization are of high dimension (easily reaching hundreds of thousands to millions of variables and constraints) but are also sparse and structured.
□ QUBIC2: A novel biclustering algorithm for large-scale bulk RNA-sequencing and single-cell RNA-sequencing data analysis:
>> https://www.biorxiv.org/content/biorxiv/early/2018/09/07/409961.full.pdf
QUBIC2 uses a truncated model to handle the unreliable quantification of genes with low or moderate expression, adopted the mixture Gaussian distribution and an information-divergency objective function to capture shared transcriptional regulation signals among a set of genes. utilized a Core-Dual strategy to identify biclusters and optimize relevant parameters, and developed a size-based P-value framework to evaluate the statistical significances of all the identified biclusters.

□ LinkedSV: Detection of mosaic structural variants from linked-read exome and genome sequencing data:
>> https://www.biorxiv.org/content/biorxiv/early/2018/09/06/409789.full.pdf
LinkedSV, a novel SV detection algorithm which combines two types of evidence. Simulation and real data analysis demonstrated that LinkedSV outperforms several existing tools including Longranger, GROC-SVs and NAIBR.
□ hsegHMM: Hidden Markov Model-based Allele-specific Copy Number Alteration Analysis Accounting for Hypersegmentation:
>> https://www.biorxiv.org/content/biorxiv/early/2018/09/06/410845.full.pdf
hsegHMM provides statistical inference of copy number profiles by using an ecient E-M algorithm procedure. hsegHMM handles hypersegmentation effectively with a t-distribution as a part of the emission probability distribution structure and a carefully defined state space. The estimation of global parameters are robust to the distribution of logR and to an expanded genotype state space. This is in contrast to the allele specific genotype status that does appear to be sensitive to the distribution of logR and to the specification of an appropriate state space of genotypes, and hence to hypersegmentation.

□ MAPS: model-based analysis of long-range chromatin interactions from PLAC-seq and HiChIP experiments:
>> https://www.biorxiv.org/content/biorxiv/early/2018/09/08/411835.full.pdf
MAPS adopts a zero-truncated Poisson regression framework to explicitly remove systematic biases in the PLAC-seq and HiChIP datasets, and then uses the normalized chromatin contact frequencies to identify significant chromatin interactions anchored at genomic regions bound.
□ Interpretable Convolution Methods for Learning Genomic Sequence Motifs:
>> https://www.biorxiv.org/content/biorxiv/early/2018/09/08/411934.full.pdf
a schema to learn the sequence motifs such that interpretation of the convolutional filters is not reliant upon test set observations & the weights comprising the filter are directly interpretable as information IGMs/PWMs, both of which may be easily visualized as sequence logos.
□ CNV Detection Power Calculator: Coverage-based detection of copy number alterations in mixed samples using DNA sequencing data: a theoretical framework for evaluating statistical power:
>> https://www.biorxiv.org/content/biorxiv/early/2018/09/10/413690.full.pdf
a systematically evaluated the factors affecting statistical power to detect CNAs from sequencing read depth data. In a basic Poisson-sampling model the overall signal for a stepwise change of DNA ploidy can be expressed as a t score, and it depends on N by |N − 2|/ √N , and is affected by CNA length L, read length l and sequencing depth D by √DL/l.
□ Cardelino: Integrating whole exomes and single-cell transcriptomes to reveal phenotypic impact of somatic variants:
>> https://www.biorxiv.org/content/biorxiv/early/2018/09/10/413047.full.pdf
A clonal tree is reconstructed based on DNA-sequencing (e.g. deep exome sequencing) data, followed by probabilistic assignment of single-cell transcriptome profiles to reconstructed clones from variants detected in scRNA-seq reads. Cardelino reveals evidence of non-neutral clonal evolution in eight of 32 lines and observed a trend that indicates an increase in the number of differentially expressed genes between clones for lines with stronger evidence for non-neutral dynamics.

□ Vex-seq: high-throughput identification of the impact of genetic variation on pre-mRNA splicing efficiency:
>> https://genomebiology.biomedcentral.com/articles/10.1186/s13059-018-1437-x
Vex-seq (Variant exon sequencing), a massively parallel approach to test the impact on pre-mRNA splicing of 2059 human genetic variants spanning 110 alternative exons, yields data that reinforce known mechanisms of pre-mRNA splicing. The impacts of changes on maximum entropy of 3′-5′ splice sites: Vex-seq still observe intronic variants changing splicing that are outside of the conventionally effects of changes. The variants that do affect branchpoint probability didn't show any significant correlation w/ ΔΨ.

□ iDEG: A single-subject method for assessing gene differential expression from two transcriptomes of an individual:
>> https://www.biorxiv.org/content/biorxiv/early/2018/09/11/405332.full.pdf
iDEG “borrows” information across genes of similar baseline expression levels and applies a variance- stabilizing transformation to estimate a gene’s distribution. iDEG provides predictions that are robust to fold-change inflation and to constricting data assumptions for identifying individualized DEGs in absence of biological replicates.
□ Multiple-kernel learning for genomic data mining and prediction:
>> https://www.biorxiv.org/content/biorxiv/early/2018/09/13/415950.full.pdf
Multiple-kernel learning (MKL) is better suited for integration of multiple high throughout data sources than SVM, which is limited to a single kernel for the entire analysis, regardless of the number of features and number of data sources.
□ GraphDDP: a graph-embedding approach to detect differentiation pathways in single-cell-data using prior class knowledge:
>> https://www.nature.com/articles/s41467-018-05988-7
GraphDDP starts from a user-defined cluster assignment and then uses a force-based graph layout approach on two types of carefully constructed edges: one emphasizing cluster membership, the other, based on density gradients, emphasizing differentiation trajectories. compared to pseudotime-based methods like TSCAN & Monocle2, as well as k-nearest neighbor approaches like SPRING. GraphDDP shows excellent performance in recovering actual biology for a well-characterized ground-truth dataset superior to established methods.
□ Proteus: an R package for downstream analysis of MaxQuant output:
>> https://www.biorxiv.org/content/biorxiv/early/2018/09/14/416511.full.pdf
Proteus performs differential expression analysis with the well-established tool limma, which offers robust treatment of missing data, frequently encountered in label-free mass-spectrometry experiments. performing differential expression on the simulated data using Perseus and Proteus. In Perseus they imported simulated data from a file, log2-transformed, applied default imputation and used a 2-sample t-test. In Proteus, log2-transformed the data and used limma for DE.
□ Dante: genotyping of known complex and expanded short tandem repeats:
>> https://academic.oup.com/bioinformatics/advance-article-abstract/doi/10.1093/bioinformatics/bty791/5092927
Dante is an alignment-free algorithm for genotyping STR alleles at user-specified known loci based on sequence reads originating from STR loci of interest. The method accounts for natural deviations from the expected sequence, such as variation in the repeat count, sequencing errors, ambiguous bases, and complex loci containing several different motifs.
□ SLiM 3: Forward genetic simulations beyond the Wright–Fisher model:
>> https://www.biorxiv.org/content/biorxiv/early/2018/09/15/418657.full.pdf
SLiM 3 supports a new non-Wright–Fisher or “nonWF” model type that provides much greater flexibility in how key processes, that allowing the explicit linking of evolutionary dynamics with ecological patterns and processes. SLiM 3 adds support for continuous space, incl spatial interactions and maps of environmental variables, which can define landscape characteristics that influence model dynamics. a spatial interaction engine that can efficiently calculate interaction strengths btwn individuals.
□ Hamiltonian Descent Methods:
>> https://arxiv.org/pdf/1809.05042.pdf
From an applied perspective, first-order methods are playing an increasingly important role in the era of large datasets and high-dimensional non-convex problems. this methods are discretizations of conformal Hamiltonian dynamics, which generalize the classical momentum method to model the motion of a particle with non-standard kinetic energy exposed to a dissipative force and the gradient field of the function of interest.