

□ SpaCeNet: Spatial Cellular Networks from omics data
>> https://www.biorxiv.org/content/10.1101/2022.09.01.506219v1
SpaCeNet analyzes patterns of correlation in spatial transcriptomics data by extending the concept of conditional independence to spatially distributed information, facilitating reconstruction of both the intracellular / intercellular interaction networks.
SpaCeNet is built on Gaussian Graphical Models (GGMs). SpaCeNet infers a joint density function describing spatially distributed, potentially high-dimensional molecular features. It uses a proximal gradient descent with Nesterov acceleration.

□ Ultima sequencing: Mostly natural sequencing-by-synthesis for scRNA-seq
>> https://www.nature.com/articles/s41587-022-01452-6
Mostly natural sequencing-by-synthesis (mnSBS) is a new sequencing chemistry that relies on a low fraction of labeled nucleotides, combining the efficiency of non-terminating chemistry w/ the throughput and scalability of optical endpoint scanning within an open fluidics system.
The results from mnSBS-based scRNA-seq are very similar to those using Illumina, with minor differences in results related to the position of reads relative to annotated gene boundaries, owing to single-end reads of Ultima being closer to gene ends than reads from Illumina.
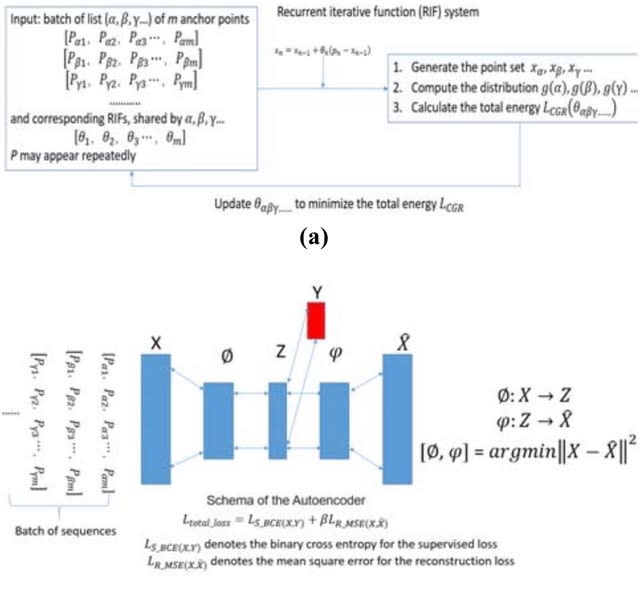
□ Sequence-based Optimized Chaos Game Representation and Deep Learning for Peptide/Protein Classification
>> https://www.biorxiv.org/content/10.1101/2022.09.10.507145v1
A novel energy function and enhanced the encoder quality by constructing a Supervised Autoencoders (SAE) neural network. The numerical Chaos Game Representation (CGR) and the SAE encoded representation and found that they are equivalent in the latent space.
The encoder φ can be used to encode the original sequences into new sets of points in the latent space. It can be used to measure the distance b/n different sequences through calculating the Jensen-Shannon Divergence, and compute the corresponding LCGR of the whole system.
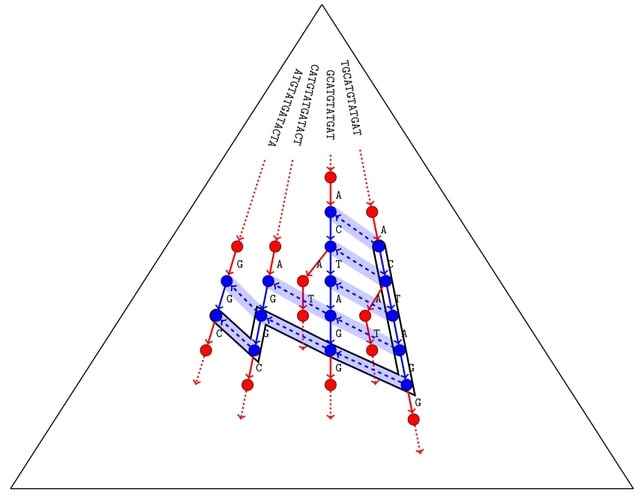
□ Genome assembly with variable order de Bruijn graphs
>> https://www.biorxiv.org/content/10.1101/2022.09.06.506758v1
The definition of voDBG resembles a generalized suffix trie. Both the nodes of the generalized suffix trie and the nodes of the voDBG correspond to all substrings occurring in the read set.
Thus the nodes of voDBG correspond one-to-one to the generalized suffix trie nodes, extension edges correspond one-to-one to the trie edges and contraction edges correspond one-to-one to the suffix links.
For the node centric definition of a DBG, the DBG edges of voDBG correspond to transitive edges composed of a contraction edge followed by an extension edge. The DBG edges of voDBG correspond to transitive edges composed of an extension edge followed by a contraction edge.
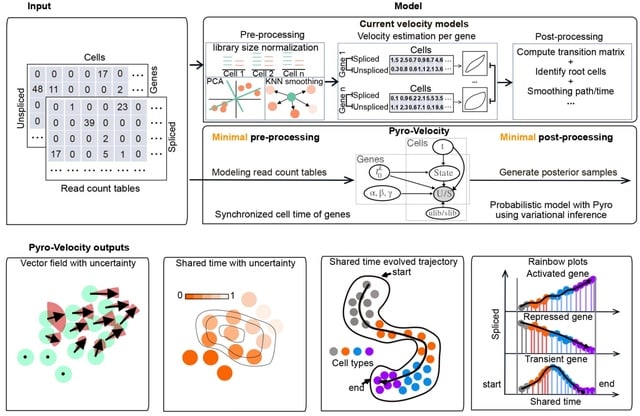
□ Pyro-Velocity: Probabilistic RNA Velocity inference from single-cell data
>> https://www.biorxiv.org/content/10.1101/2022.09.12.507691v1
Pyro-Velocity, a multivariate RNA Velocity model to estimate the cell future states. Pyro-Velocity models raw sequencing counts w/ the synchronized cell time across all expressed genes to provide quantifiable and improved information on cell fate choices and trajectory dynamics.
Pyro-Velocity recasts the velocity estimation problem into a latent variable posterior probability inference. The method is generative / fully Bayesian, w/ the different parameters considered as latent random variables. Central to the Pyro-Velocity model is a shared latent time.

□ scHiMe: Predicting single-cell DNA methylation levels based on single-cell Hi-C data
>> https://www.biorxiv.org/content/10.1101/2022.09.13.507815v1
scHiMe is a computational tool for predicting the base-pair-specific methylation levels in the promoter regions genome-wide based on the single-cell Hi-C data and DNA nucleotide sequences using the graph transformer algorithm.
The true base-pair-specific DNA methylation values or target values for the 1000 base pairs in the target promoter were generated based on meta-cell. Node / Edge features were generated and input into the graph transformer network, which contained 5 blocks of graph transformer.
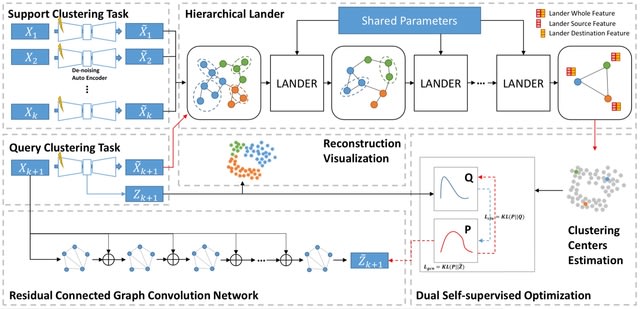
□ MeHi-SCC: A Meta-learning based Graph-Hierarchical Clustering Method for Single Cell RNA-Seq Data https://www.biorxiv.org/content/10.1101/2022.09.06.506784v1
MeHi-SCC features a whole-graph-tuning based hierarchical clustering section. LANDER, the separator, only learns how inter-cellular relationship helps cluster step by step toward ground truth, ignoring specific expression values.
Different from GNN with fixed adjacent matrix, LANDER updates both edge-connections and related node features. MeHi-SCC enables sub-cell-type detection.
Hierarchical LANDER divides cell graphs into sub-cell graphs and aggregate them into more detailed clusters for all cells until they cannot be divided into sub-graphs any more, and the cluster number is usually more than ground truth given by manual annotations from morphology.
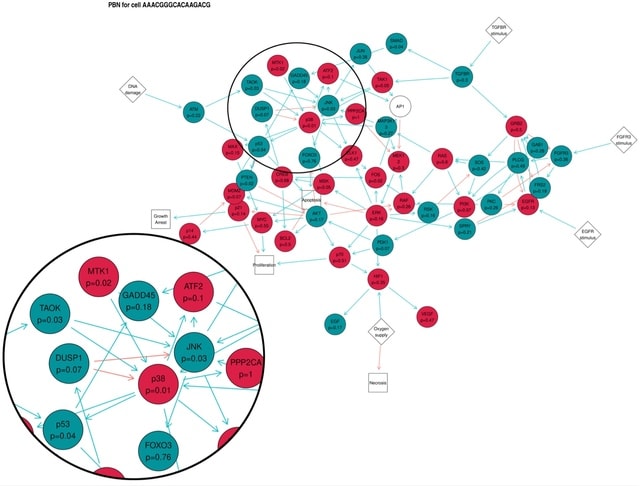
□ Ingres: from single-cell RNA-seq data to single-cell probabilistic Boolean networks
>> https://www.biorxiv.org/content/10.1101/2022.09.04.506528v1
Ingres provides another solution to this problem by representing different levels of activation/expression while still working with Boolean functions. Ingres uses VIPER algorithm to infer protein activity starting from a gene expression matrix and a list of regulons.
Ingres facilitates fitting models with cell-specific expression information without the need of inferring a new network for each cell or cluster.
Ingres runs the metaVIPER algorithm. Ingres provides several wrapper functions for relevant parts of BoolNet, which can be used to perform analyses on any PBN produced by Ingres, such as computing its attractors.

□ HexSE: Simulating evolution in overlapping reading frames
>> https://www.biorxiv.org/content/10.1101/2022.09.09.453067v1
HexSE is a Python module designed to simulate sequence evolution along a phylogeny while considering the coding context the nucleotides. The ultimate porpuse of HexSE is to account for multiple selection preasures on Overlapping Reading Frames.
HexSE uses the Gillespie algorithm to simulate mutations along branches of the phylogenetic tree in order to create a nucleotide alignment. Traversing the event probability tree from the root to a tip resolves the shared characteristics for a subset of substitution events.
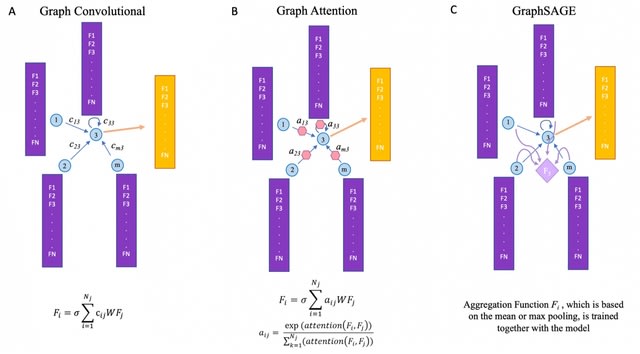
□ DeepZ: Graph Neural Networks for Z-DNA prediction in Genomes
>> https://www.biorxiv.org/content/10.1101/2022.08.23.504929v1
There is potential for improvement of GNN architecture by incorporating long-range interactions b/n DNA nodes into the graph representation, by using different weighing schemes that capture the correlation b/n features of adjacent nodes and the use of L1 metrics.
DeepZ approach with GNN deep learning model instead of RNN. GraphZ is based on three major types of graph neural network modes – two types of Graph Convolutional Networks, two types of Graph Attention Networks and inductive representation learning network GraphSAGE.

□ Scelestial: Fast and accurate single-cell lineage tree inference based on a Steiner tree approximation algorithm
>> https://journals.plos.org/ploscompbiol/article?id=10.1371/journal.pcbi.1009100
Scelestial, a method for lineage tree reconstruction from single-cell data. In this representation the phylogeny inference problem could be considered as a geometric Steiner tree problem, in which weight of edges are calculated as the Euclidean distances between the points.
Scelestial’s input is a set of genome sequences given as a matrix of point mutations, which may contain missing values. Scelestial iteratively improves the inferred tree by considering all subsets of samples of a size up to a constant parameter and all the potential phylogenies.

□ Sequence to graph alignment using gap-sensitive co-linear chaining
>> https://www.biorxiv.org/content/10.1101/2022.08.29.505691v1
A novel co-linear chaining problem formulations for sequence-to-DAG alignment that penalize gaps. It is designed gap cost functions such that they enable us to adapt the sparse dynamic programming framework, and solve the chaining problem optimally in O(KN log KN) time.
This algorithm for Problems 1a-1c uses a brute-force approach that evaluates all O(N2) pairs of anchors, and uses Dijkstra’s algorithm with a Fibonacci heap for shortest-path calculations. Problems 1a, 1b and 1c can be solved optimally in O(N2(|V|log|V|+|E|)) time.

□ CANTATA - prediction of missing links in Boolean networks using genetic programming
>> https://academic.oup.com/bioinformatics/advance-article/doi/10.1093/bioinformatics/btac623/6696209
The CANTATA algorithm optimizes network models towards a certain behaviour based on a multi-objective genetic programming approach. CANTATA allows for perturbed network conditions with knocked-out or overexpressed compounds.
CANTATA is elaborated to guide an evolutionary transformation process, yielding network models that resemble the initial model drafts closely while matching the observed dynamic behaviour. The algorithm ensures minimal interventions by relying on symbolic representation.

□ SCING: Single Cell INtegrative Gene regulatory network inference elucidates robust, interpretable gene regulatory networks
>> https://www.biorxiv.org/content/10.1101/2022.09.07.506959v1
SCING, a gradient boosting and mutual information based approach for identifying robust GRNs from scRNAseq, snRNAseq, and spatial transcriptomics data.
SCING GRNs reveal unique disease subnetwork modeling capabilities, have intrinsic capacity to correct for batch effects, retrieve disease relevant genes and pathways.
SCING uses a random walk framework to determine the increase in performance of a GRN to model disease subnetworks versus a random GRN with similar node attributes. And it utilizes the leiden graph partitioning algorithm to identify GRN subnetworks.
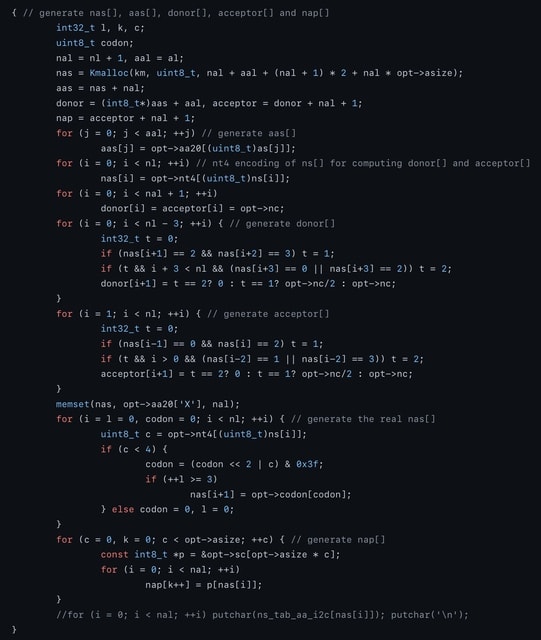
□ nasw: Dynamic programming for aa-to-nt alignment with affine gap, splicing and frameshift
>> https://github.com/lh3/nasw
The DP involves 6 states and 20 transitions, similar to the GeneWise model. Different from GeneWise, nasw explicitly implements the DP recursion with SSE2 or NEON intrinsics and is tens of times faster.
nasw supports global alignment and left or right extension. In the extension mode, only extension ends and alignment score are computed. Users need to call the function again to get CIGAR.

□ miniprot: a new mapper for aligning proteins to genomes with splicing and frameshift.
>> https://github.com/lh3/miniprot
Miniprot aligns a protein sequence against a genome with affine gap penalty, splicing and frameshift. It is primarily intended for annotating protein-coding genes in a new species using known genes from other species.
Miniprot is not optimized for mapping distant homologs because distant homologs are less informative to gene annotations. Miniprot outputs alignment in the protein PAF format. miniprot uses more CIGAR operators to encode introns and frameshifts.
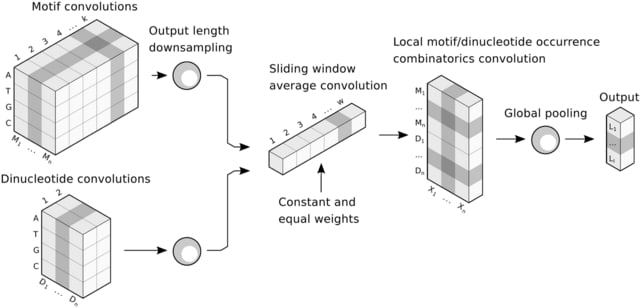
□ Gnocis: An integrated system for interactive and reproducible analysis and modelling of cis-regulatory elements in Python 3
>> https://journals.plos.org/plosone/article?id=10.1371/journal.pone.0274338
Deep-MOCCA has a layer of longer convolutions, and in order to model dinucleotides, a layer of 2bp convolutions. These two convolutional layers are concatenated. The 5-spectrum SVM achieves the highest sensitivity to independent PREs, but also the lowest precision.
Gnocis is a system for the interactive and reproducible analysis and modelling of CRE DNA sequences. Gnocis employs Cython and a variety of techniques in order to optimally implement the glue necessary in order to apply machine learning for CRE analysis and prediction.
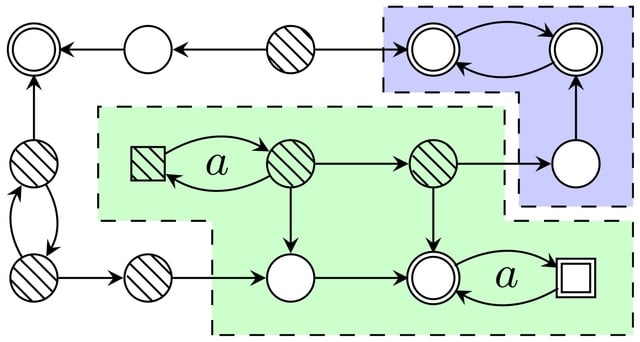
□ AEON.py: Python Library for Attractor Analysis in Asynchronous Boolean Networks
>> https://academic.oup.com/bioinformatics/advance-article-abstract/doi/10.1093/bioinformatics/btac624/6697883
AEON.py combines a known symbolic detection algorithm (adapted to better handle partially specified BNs) with a more advanced reduction method guided by the fire-ability of transitions in the Boolean network.
AEON.py allows solving attractor detection and source-target control problems on large, non-trivial networks. Furthermore, these problems can be addressed even in networks with logical parameters or partially unknown dynamics.
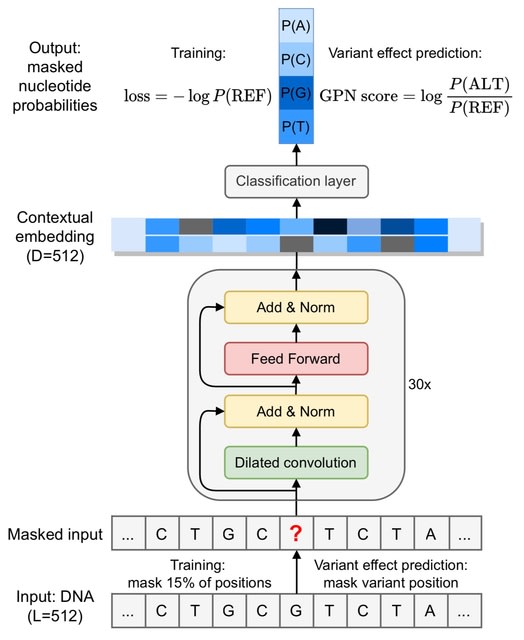
□ GPN: DNA language models are powerful zero-shot predictors of non-coding variant effects
>> https://www.biorxiv.org/content/10.1101/2022.08.22.504706v1
GPN (Genomic Pre-trained Network) learns variant effects in non-coding DNA using unsupervised pre-training on genomic DNA sequence alone. GPN is also able to learn gene structure and DNA motifs without any supervision.
GPN outperforms the DeepSEA model trained on functional genomics data. GPN’s internal representation of DNA sequences is able to accurately distinguish genomic regions such as introns, untranslated regions and coding sequences.

□ SCsnvcna: Integrating SNVs and CNAs on a phylogenetic tree from single-cell DNA sequencing data
>> https://www.biorxiv.org/content/10.1101/2022.08.26.505465v1
SCARLET requires that the SNVs and CNAs are detected from the same sets of cells, which is technically challenging due to the sequencing errors or the low sequencing coverage associated with a particular WGA procedure.
SCsnvcna is a Bayesian probabilistic model that utilizes both the genotype constraints on the tree and the cellular prevalence to search the solution that has the highest joint probability. SCsnvcna aims at placing SNVs on a CNA tree whereas the sets of cells rendering independent.

□ IndepthPathway: an integrated tool for in-depth pathway enrichment analysis based on bulk and single cell sequencing data
>> https://www.biorxiv.org/content/10.1101/2022.08.28.505179v1
WCSEA algorithm took a broader approach for assessing the functional relations of pathway gene sets to differentially expressed genes, and leverage the cumulative signature of molecular concepts characteristic of the highly differentially expressed genes.
“IndepthPathway” for deep pathway enrichment analysis from bulk and single cell sequencing data that took a broader approach for assessing gene set relations and leverage the universal concept signature of the target gene list to tolerate the high noise and low gene coverage.
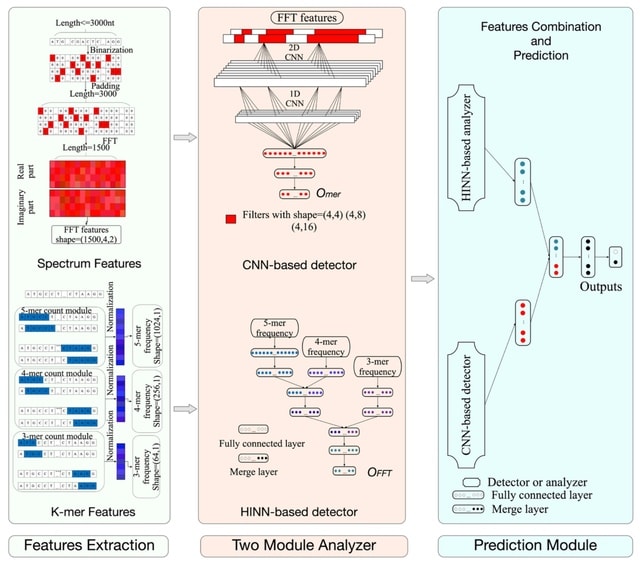
□ LncDLSM: Identification of Long Non-coding RNAs with Deep Learning-based Sequence Model
>> https://www.biorxiv.org/content/10.1101/2022.09.02.506180v1
The whole lncDLSM consists of two parts, the first part is based on hierarchical input neural networks, called HINN-based analyzer, which is designed to extract the advanced features of the k-mer frequency features.
Another part is a CNN-based detector, which is designed to extract the advanced features of the spectrum features. Then it merges these high-level features using another neural network-based prediction module to identify lncRNAs finally.
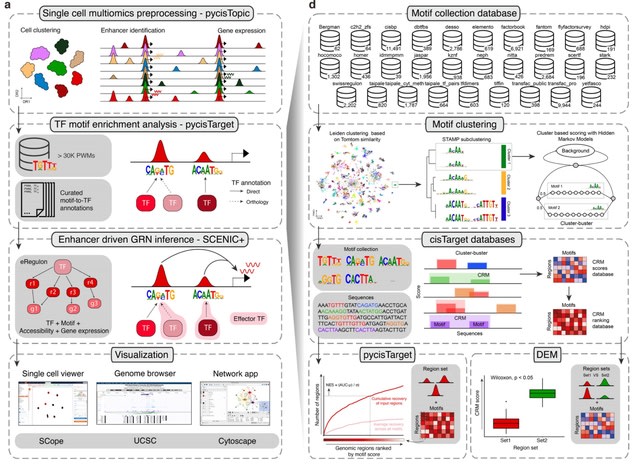
□ SCENIC+: single-cell multiomic inference of enhancers and gene regulatory networks
>> https://www.biorxiv.org/content/10.1101/2022.08.19.504505v1.full.pdf
SCENIC+ predicts genomic enhancers along w/ candidate upstream TF and links these enhancers to candidate target genes. Specific TFs for each cell type or cell state are predicted based on the concordance of TF binding site accessibility, TF expression, and target gene expression.
SCENIC+ combines the gene expression values, the denoised region accessibility, and the cistromes to predict TF-region-gene triplets. Region-to-gene and TF-to-gene relationships are inferred using Pearson correlation and Gradient Boosting Machines.
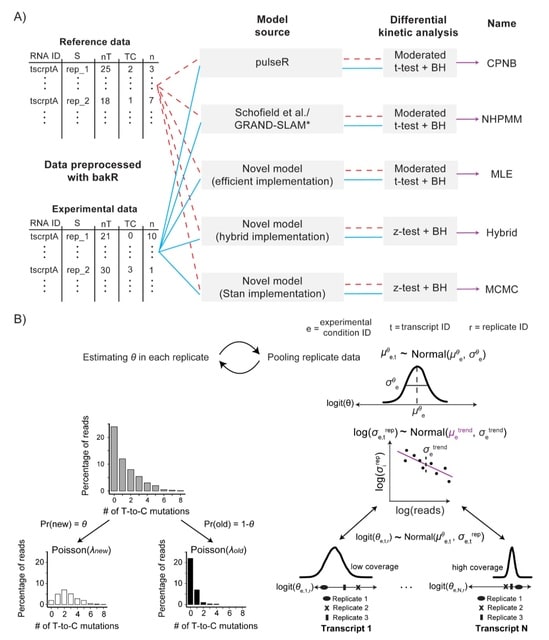
□ Differential kinetic analysis using nucleotide recoding RNA-seq and bakR
>> https://www.biorxiv.org/content/10.1101/2022.09.02.505697v1
bakR (Bayesian analysis of the kinetics of RNA) relies on Bayesian hierarchical modeling of nucleotide recoding RNA-seq (NR-seq) data to increase statistical power by sharing information across transcripts.
bakR includes three distinct computational implementations of the Bayesian hierarchical mixture model (MLE / Hybrid / MCMC). Partial pooling across fraction new and variance estimates in a given replicate is performed to make use of the high-throughput nature of NR-seq datasets.

□ SiGra: Single-cell spatial elucidation through image-augmented graph transformer
>> https://www.biorxiv.org/content/10.1101/2022.08.18.504464v1.full.pdf
SiGra deciphers spatial domains and enhance spatial signals simultaneously. SiGra is one of the first method to utilize multi-modalities including multi-channel images of cell morphology and functions to address technology limitations and achieve augmented spatial profiles.
In SiGra, the multi-modal information from images and original transcriptomics are summarized at single-cell level, with the information from neighboring cells selectively captured by the attention mechanism.

□ BWA-MEM2-LISA: https://github.com/bwa-mem2/bwa-mem2/tree/bwa-mem2-lisa
bwa-mem2-lisa is an accelerated version of bwa-mem2. Accelerating the seeding phase of bwa-mem2 using: 1. LISA (Learned-Indexes for Sequence Analysis) and 2. binary interval tree.
BWA-MEM2-LISA accelerated seeding kernels achieve up to 4.5x speedup compared to the seeding phase. The ert branch of bwa-mem2 repository contains codebase of Enuerated Radix Tree based acceleration.
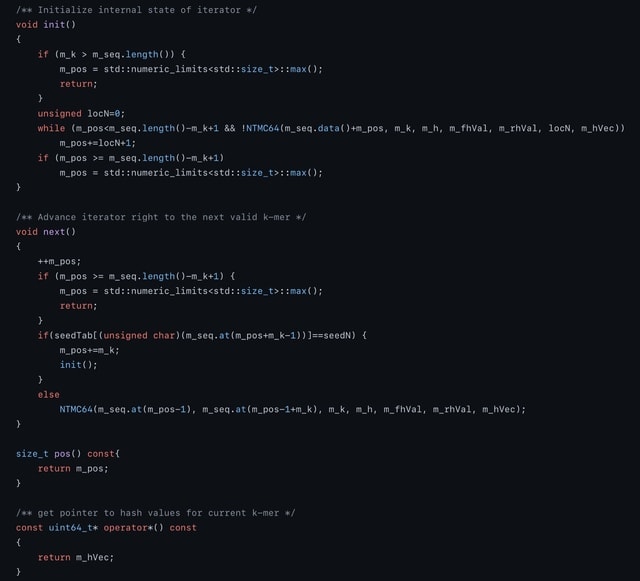
□ ntHash2: recursive spaced seed hashing for nucleotide sequences
>> https://academic.oup.com/bioinformatics/advance-article/doi/10.1093/bioinformatics/btac564/6674501
ntHash2 is up to 2.1x faster at hashing various spaced seeds than the previous version and 3.8x faster than conventional hashing algorithms with naïve adaptation.
ntHash2 performs reverse-complement hashing w/o requiring extra iterations by swapping the corresponding indices in the blocks. Reducing the collision rate for longer k-mer lengths and improved the uniformity of the hash distribution by modifying the canonical hashing mechanism.
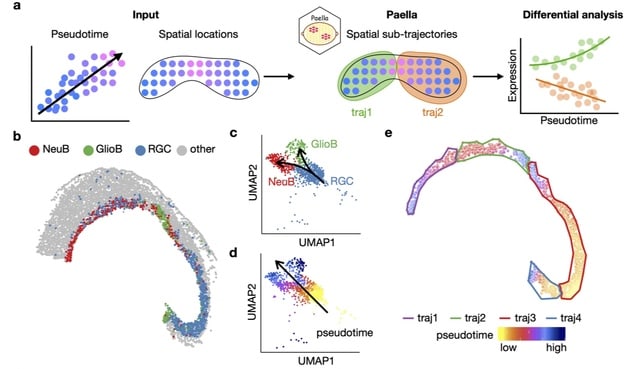
□ Paella: Decomposing spatial heterogeneity of cell trajectories
>> https://www.biorxiv.org/content/10.1101/2022.09.05.506682v1
Paella requires as input the spatial locations of cells or spatial spots and the cell trajectory information. Paella then identifies a parsimonious set of spatially continuous sub-trajectories where each sub-trajectory represents a unidirectional process of cell progression.
Paella constructs an undirected Delaunay network. Paella converts the undirected network into two directed networks by comparing the pseudotime values of the two nodes connected by an edge, and identifies with three modes all node sets where nodes in each set are reachable.
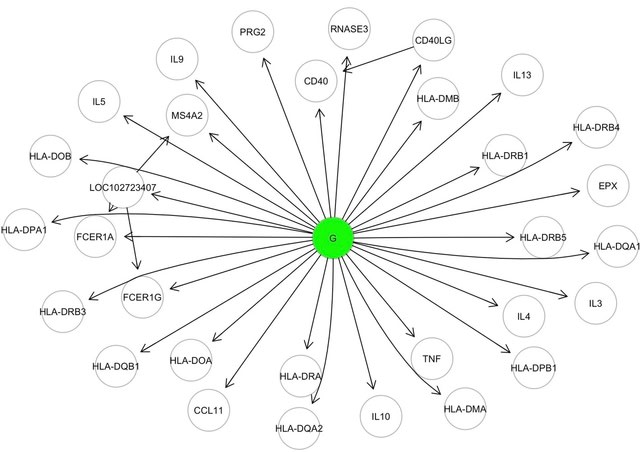
□ SEMgsa: topology-based pathway enrichment analysis with structural equation models
>> https://bmcbioinformatics.biomedcentral.com/articles/10.1186/s12859-022-04884-8
SEMgsa() represent a topological based and self-contained hypotesis method, in line with NetGSA, DEGraph and topologyGSA. SEMgsa() accepts as input directed and/or undirected networks that define pathway interconnectedness.

□ SCIΦN: Single-cell mutation calling and phylogenetic tree reconstruction with loss and recurrence
>> https://academic.oup.com/bioinformatics/advance-article/doi/10.1093/bioinformatics/btac577/6674502
SCIPhIN considers the full read and variant counts for each cell at each genomic position to better distinguish mutations from sequencing and amplification noise. SCIPhIN allows for mutation loss and parallele mutations, relaxing the infinite sites assumption.

□ New algorithms for accurate and efficient de-novo genome assembly from long DNA sequencing reads
>> https://www.biorxiv.org/content/10.1101/2022.08.30.505891v1
A new hashing scheme for minimizers to efficiently identify overlaps and build OLC graphs. The implemented algorithm to build an overlap graph and a layout.
The graph construction is similar to that of the Best Overlap Graph, having two vertices for each read representing the start (5’-end) and the end (3’-end) of the read.
Edge features are combined based on their likelihood, replacing edge filtering by edge prioritization. This approach eliminates the need of hard filtering decisions and makes the algorithm adaptable to genomic regions with different repeat structures.

□ KMer-Node2Vec: Learning Vector Representations of K-mers from the K-meGraph
>> https://www.biorxiv.org/content/10.1101/2022.08.30.505832v1
KMer-Node2Vec, a graph-based DNA embedding algorithm, which converts the large DNA corpus into a k-mer co-occurrence graph, then takes the k-mer sequence samples from this graph by randomly traveling and finally trains the k-mer embedding on this sampling corpus.
KMer-Node2Vec uses an effective sampling strategy to generate the k-mer sequences, and the Skip-Gram algorithm is used to calculate the k-mer embedding on k-mer sequences. The KMer-Node2Vec’s time complex is O(|N | + nl + nllog(|V |)) and space complexity is O(m|V |+nl+d|V |).
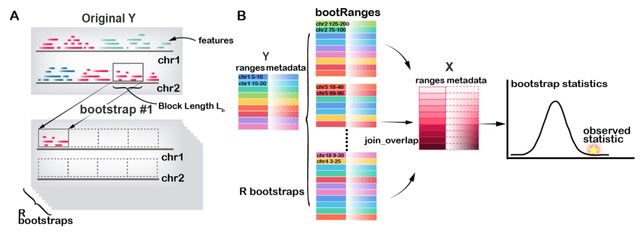
□ bootRanges: Flexible generation of null sets of genomic ranges for hypothesis testing
>> https://www.biorxiv.org/content/10.1101/2022.09.02.506382v1
bootRanges software, with efficient vectorized code for performing block boot-strap sampling of genomic ranges. bootRanges is part of a modular analysis workflow, where bootstrapped ranges can be analyzed at block or genome scale using tidy analysis with plyranges.
bootRanges offers a simple “unsegmented” block bootstrap as well as a “segmented” block bootstrap: since the distribution of ranges in the genome exhibits multi-scale structure, It follows the logic of Bickel et al. and performs block bootstrapping within segments of the genome.
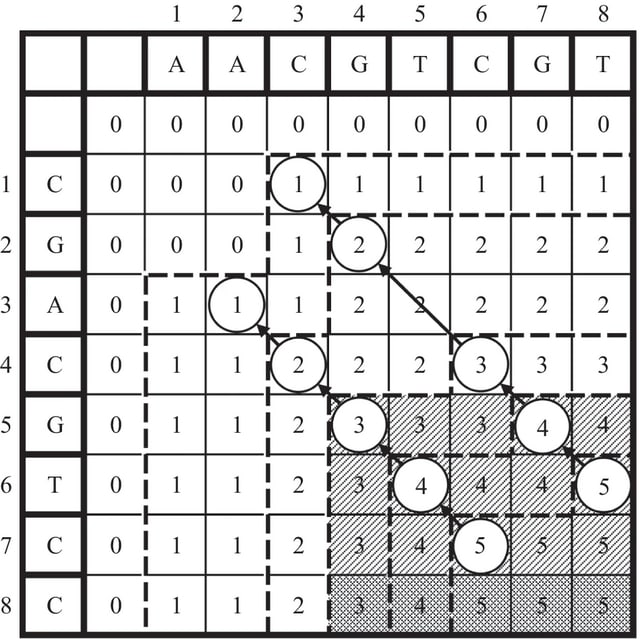
□ A fast and efficient path elimination algorithm for large-scale multiple common longest sequence problems
>> https://bmcbioinformatics.biomedcentral.com/articles/10.1186/s12859-022-04906-5
A mini Directed Acyclic Graph (mini-DAG) model and a novel Path Elimination Algorithm are proposed to address large-scale MLCS issues efficiently. mini-DAG employs the branch and bound approach to reduce paths during DAG construction, resulting in a very mini DAG.
Before obtaining the final MLCS, if we can judge that the currently calculated match point is not the point that constitutes the MLCS, then the path through this point will not be the longest; these are called the non-point and non-optimal paths.
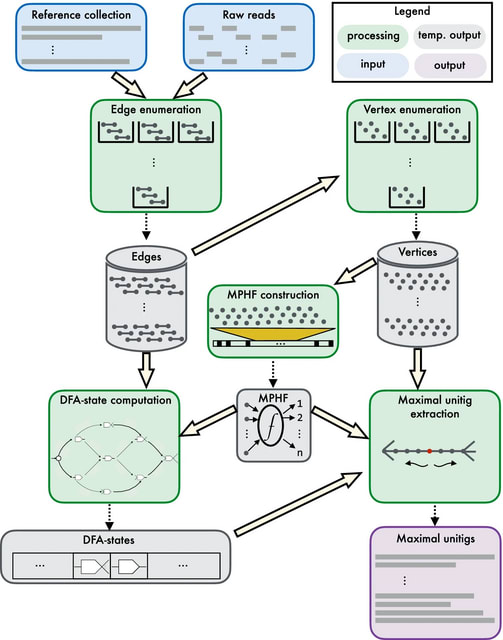
□ Cuttlefish 2: Scalable, ultra-fast, and low-memory construction of compacted de Bruijn graphs
>> https://genomebiology.biomedcentral.com/articles/10.1186/s13059-022-02743-6
CUTTLEFISH 2 can seamlessly extract such maximal path covers by simply constraining the algorithm to operate on some specific subgraph(s) of the original graph. The edges ((k+1)-mers) are enumerated from the input, and optionally filtered based on the user-defined threshold.