

□ Numbat: Haplotype-enhanced inference of somatic copy number profiles from single-cell transcriptomes
>> https://www.biorxiv.org/content/10.1101/2022.02.07.479314v1.full.pdf
Numbat integrates expression/allele/haplotype information derived from population-based phasing to comprehensively characterize the CNV landscape. a Hidden Markov model integrates expression deviation and haplotype imbalance signals to detect CNVs in cell population pseudobulks.
Numbat employs an iterative approach to reconstruct the subclonal phylogeny and single-cell copy number profile. Numbat identifies distinct subclonal lineages that harbor haplotype-specific alterations. It does not require sample-matched DNA data or a priori genotyping.

□ ClonoCluster: a method for using clonal origin to inform transcriptome clustering
>> https://www.biorxiv.org/content/10.1101/2022.02.11.480077v1.full.pdf
ClonoCluster, a computational method that combines both clone and transcriptome information to create hybrid clusters that weight both kinds of data with a tunable parameter - Warp Factor.
Warp Factor incorporates clonality information into the dimensionality reduction step prior to the commonly-used UMAP algorithm for visualizing high dimensional datasets. Individual clone clusters formed distinct spatial clusters in UMAP space.

□ Optimal Evaluation of Symmetry-Adapted n-Correlations Via Recursive Contraction of Sparse Symmetric Tensors
>> https://arxiv.org/pdf/2202.04140v1.pdf
A comprehensive analysis of an algorithm for evaluating high-dimensional polynomials. The key bottleneck is the contraction of a high-dimensional symmetric and sparse tensor with a specific sparsity pattern that is directly related to the symmetries imposed on the polynomial.
The key step is to understand the insertion of so-called “auxiliary nodes” into this graph which represent intermediate computational steps. An explicit construction of a recursive evaluation strategy and show that it is optimal in the limit of infinite polynomial degree.

□ n-Best Kernel Approximation in Reproducing Kernel Hilbert Spaces
>> https://arxiv.org/pdf/2201.07228v1.pdf
By making a seminal use of the maximum modulus principle of holomorphic functions they prove existence of n-best kernel approximation for a wide class of reproducing kernel Hilbert spaces of holomorphic functions in the unit disc.
A clever and concise proof for the existence of the n-best kernel approximation for a large class of reproducing kernel Hilbert spaces, that is in particular strictly larger than that of the weighted Bergman spaces, enclosing all the weighted Hardy spaces.

□ LSCON: Fast and accurate gene regulatory network inference by normalized least squares regression
>> https://academic.oup.com/bioinformatics/advance-article/doi/10.1093/bioinformatics/btac103/6530276
LSCON (Least Squares Cut-Off with Normalization) extends the LSCO algorithm by regularization to avoid hyper-connected genes and thereby reduce false positives.
LSCON performed similarly to the LASSO algorithm in correctness, while outperforming LSCO, RidgeCO, and Genie3 on data with infinitesimal fold change values. LSCON was found to be about 1000 times faster than Genie3.

□ polishCLR: a Nextflow workflow for polishing PacBio CLR genome assemblies
>> https://www.biorxiv.org/content/10.1101/2022.02.10.480011v1.full.pdf
polishCLR, a reproducible Nextflow workflow that implements best practices for polishing assemblies made from Continuous Long Reads (CLR) data.
PolishCLR provides re-entry points throughout several key processes including identifying duplicate haplotypes in purge_dups, allowing a break for scaffolding if data are available, and throughout multiple rounds of polishing and evaluation with Arrow and FreeBayes.

□ NEN: Single-cell RNA sequencing data analysis based on non-uniform ε- neighborhood network
>> https://academic.oup.com/bioinformatics/advance-article-abstract/doi/10.1093/bioinformatics/btac114/6533440
non-uniform ε - neighborhood network (NEN) combines the advantages of both k-nearest neighbors (KNN) and ε - neighborhood (EN) to represent the manifold that data points reside in gene space.
Then from such a network, NEN uses its layout, its community and further its shortest path to achieve the purpose of scRNA-seq data visualization, clustering and trajectory inference.

□ Anc2vec: embedding gene ontology terms by preserving ancestors relationships
>> https://academic.oup.com/bib/advance-article-abstract/doi/10.1093/bib/bbac003/6523148
Significant performance improvements have been observed when the vector representations are used on diverse downstream tasks, such as the measurement of semantic similarity. However, existing embeddings of GO terms still fail to capture crucial structural features.
anc2vec, a novel protocol based on neural networks for constructing vector representations of Gene Ontology. These embeddings are built to preserve three structural features: the ontological uniqueness of terms, their ancestor relationships and the sub-ontology to which they belong.

□ scvi-tools: A Python library for probabilistic analysis of single-cell omics data
>> https://www.nature.com/articles/s41587-021-01206-w
scvi-tools offers standardized access to methods for many single-cell data analysis, such as integration of scVI / scArches, annotation of CellAssign / scANVI, deconvolution of bulk spatial transcriptomics (Stereoscope), doublet detection(Solo) and multi-modal analysis (totalVI).
Those elements are organized into a class that inherits from the abstract class BaseModuleClass. scvi-tools offers a set of building blocks. It can be used for efficient model development through Stereoscope, which demonstrates a substantial reduction in code complexity.

□ MegaGate: A toxin-less gateway molecular cloning tool
>> https://star-protocols.cell.com/protocols/1120
MegaGate is an enabling technology for use in cDNA screening and cell engineering for mammalian systems. MegaGate eliminates the ccdb toxin used in Gateway recombinase cloning and instead utilizes mega- nuclease-mediated digestion to eliminate background vectors during cloning.
MegaDestination vectors can optionally feature unique DNA barcodes that can be captured through gDNA sequencing. if a plasmid does not contain a gene of interest, it retains the meganuclease recognition cassette which is digested by the meganucleases in the MegaGate reaction mix.

□ Syllable-PBWT for space-efficient haplotype long-match query
>> https://www.biorxiv.org/content/10.1101/2022.01.31.478234v1.full.pdf
Syllable-PBWT, a space- efficient variation of the positional Burrows-Wheeler transform which divides every haplotype into syllables, builds the PBWT positional prefix arrays on the compressed syllabic panel, and leverages the polynomial rolling hash function.
The Syllable-Query algorithm finds long matches between a query haplotype and the panel. Syllable-Query is significantly faster than the full memory algorithm. After reading in the query haplotype in O(N ) time, these sequences require O(nβ log M ) time to compute.

□ mcPBWT: Space-efficient Multi-column PBWT Scanning Algorithm for Composite Haplotype Matching
>> https://www.biorxiv.org/content/10.1101/2022.02.02.478879v1.full.pdf
mcPBWT (multi-column PBWT) uses multiple synchronized runs of PBWT at different variant sites providing a “look-ahead” information of matches at those variant sites. Such “look-ahead” information allows us to analyze multiple contiguous matching pairs in a single pass.
Triangulating the genealogical relationship among individuals carrying these matching segments. double-PBWT finds two matching pairs’ combinations representative of phasing error while triple-PBWT finds three matching pairs’ combinations representative of gene-conversion tract.

□ gwfa: Proof-of-concept implementation of GWFA for sequence-to-graph alignment
>> https://github.com/lh3/gwfa
GWFA (Graph WaveFront Alignment) is an algorithm to align a sequence against a sequence graph. It adapts the WFA algorithm for graphs. A proof-of-concept implementation of GWFA that computes the edit distance between a graph and a sequence without backtracing.
GWFA algorithm assumes the start of the sequence to be aligned with the start of the first segment in the graph and requires the query sequence to be fully aligned. GWFA is optimized for graphs consisting of long segments.

□ Dynamo: Mapping transcriptomic vector fields of single cells
>> https://www.cell.com/cell/fulltext/S0092-8674(21)01577-4
Dynamo infers absolute RNA velocity, reconstructs continuous vector fields that predict cell fates, employs differential geometry to extract underlying regulations, and ultimately predicts optimal reprogramming paths.
Dynamo calculates RNA acceleration, curvature, divergence and RNA Jacobian. Dynamo uses Least Action Paths (LAPs) and in silico perturbation. Dynamo makes it possible to use single-cell data to directly explore governing regulatory mechanisms and even recover kinetic parameters.

□ Transformation of alignment files improves performance of variant callers for long-read RNA sequencing data
>> https://www.biorxiv.org/content/10.1101/2022.02.08.479579v1.full.pdf
DeepVariant, Clair3 and NanoCaller use a deep learning approach in which variants are detected by analysis of read-alignment images; Clair3 uses a pileup model to call most variants, and a more computationally-intensive full-alignment model to handle more complex variants.
Clair3-mix and SNCTR+flagCorrection+DeepVariant are among the best-performing pipelines to call indels, the former having higher recall and the latter higher precision.

□ SeCNV: Resolving single-cell copy number profiling for large datasets
>> https://www.biorxiv.org/content/10.1101/2022.02.09.479672v1.full.pdf
SeCNV, a novel method that leverages structural entropy, to profile the copy numbers. SeCNV adopts a local Gaussian kernel to construct a matrix, depth congruent map, capturing the similarities between any two bins along the genome.
SeCNV partitions the genome into segments by minimizing the structural entropy from the depth congruent map. With the partition, SeCNV estimates the copy numbers within each segment for cells.

□ WAFNRLTG: A Novel Model for Predicting LncRNA Target Genes Based on Weighted Average Fusion Network Representation Learning Method
>> https://www.frontiersin.org/articles/10.3389/fcell.2021.820342/full
WAFNRLTG constructs a heterogeneous network, which integrated two similar networks and three interaction networks. Next, the network representation learning method was utilized to gain the representation vectors of lncRNA and mRNA nodes.
The representation vectors of lncRNAs and the representation vectors of mRNAs were merged to form the lncRNA-gene pairs, and XGBoost classifier was built based on the merged representations of lncRNA-miRNA pairs.

□ CELESTA: Identification of cell types in multiplexed in situ images by combining protein expression and spatial information
>> https://www.biorxiv.org/content/10.1101/2022.02.02.478888v1.full.pdf
CELESTA (CELl typE identification with SpaTiAl information) incorporates both cell’s protein expression profile and its spatial information, with minimal to no user-dependence, to produce relatively fast cell type assignments.
CELESTA defines an energy function using the Potts model to leverage cell type information on its spatially N-nearest neighboring cells in a probabilistic manner. CELESTA represents each index cell as a node in an undirected graph with each edge connecting its spatially N-NN.
CELESTA associates each node with a hidden state, where the hidden state is the cell type to be inferred, and assumes that the joint distribution of the hidden states satisfy discrete Markov Random Field.

□ Vivarium: an interface and engine for integrative multiscale modeling in computational biology
>> https://academic.oup.com/bioinformatics/advance-article-abstract/doi/10.1093/bioinformatics/btac049/6522109
Vivarium can apply to any type of dynamic model – ordinary differential equations (ODEs), stochastic processes, Boolean networks, spatial models, and more – and allows users to plug these models together in integrative, multiscale representations.
Vivarium's modular interface makes individual simulation tools into modules that can be wired together in composite multi-scale models, parallelized across multiple CPUs, and run with Vivarium's discrete-event simulation engine.

□ RaPID-Query for Fast Identity by Descent Search and Genealogical Analysis
>> https://www.biorxiv.org/content/10.1101/2022.02.03.478907v1.full.pdf
RaPID-Query (Random Projection-based Identical-by-descent Detection Query) method identifies IBD segments between a query haplotype and a panel of haplotypes. RaPID-Query locates IBD segments quickly with a given cutoff length while allowing mismatched sites in IBD segments.
RaPID-Query uses x-PBWT-Query, an extended PBWT query algorithm, by single sweep long match query algorithm. It eliminates the redundant steps of evaluating the divergence values of the haplotypes if they are already in the set-maximal match block.

□ Dysgu: efficient structural variant calling using short or long reads
>> https://academic.oup.com/nar/advance-article/doi/10.1093/nar/gkac039/6517943
dysg can rapidly call SVs from PE or LR data, across all size categories. Conceptually, dysgu identifies SVs from alignment cigar information as well as discordant and split-read mappings.
Dysgu employs a fast consensus sequence algorithm, inspired by the positional de Brujin graph, followed by remapping of anomalous sequences to discover additional small SVs.

□ The SAMBA tool uses long reads to improve the contiguity of genome assemblies
>> https://journals.plos.org/ploscompbiol/article?id=10.1371/journal.pcbi.1009860
Several previously developed tools also allow for scaffolding with long reads. These include AHA, which is part of the SMRT software analysis suite, SSPACE-LongRead, and LINKS however none of these tools utilize the consensus of the long reads to fill gaps in the scaffolds.
SAMBA (Scaffolding Assemblies with Multiple Big Alignments) is designed to scaffold and gap-fill genome assemblies with long-read data, resulting in substantially greater contiguity. SAMBA fills in the sequence for all spanned gaps in the scaffolds, yielding much longer contigs.
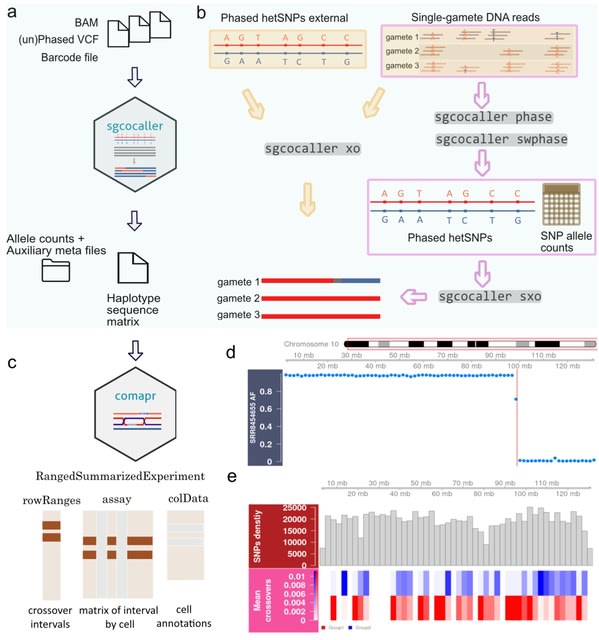
□ sgcocaller and comapr: personalised haplotype assembly and comparative crossover map analysis using single gametes
>> https://www.biorxiv.org/content/10.1101/2022.02.10.479822v1.full.pdf
an efficient software toolset using modern programming languages for the common tasks of haplotyping haploid gamete genomes and calling crossovers (sgcocaller), and constructing and visualising individualised crossover landscapes (comapr) from single gametes.
sgcocaller xo implements a two-state Hidden Markov Model and adopts binomial distributions for modelling the emission probabilities of the observed allele read counts. the Viterbi algorithm is applied to infer the most probable hidden state sequence for the list of hetSNPs.

□ UINMF performs mosaic integration of single-cell multi-omic datasets using nonnegative matrix factorization
>> https://www.nature.com/articles/s41467-022-28431-4
UINMF can integrate data types such as scRNA-seq and snATAC-seq using both gene-centric features and intergenic information. UINMF fully utilizes the available data when estimating metagenes and matrix factors, significantly improving sensitivity for resolving cellular distinctions.
UINMF can integrate targeted spatial transcriptomic data with simultaneous single-cell RNA and chromatin accessibility measurements using both unshared epigenomic information and unshared genes.
The UINMF optimization algorithm has a reduced computational complexity per iteration compared to iNMF algorithm on a dataset of the same size. UINMF, as well as iNMF, requires random initializations, and is nondeterministic in nature.

□ SmMIP-tools: a computational toolset for processing and analysis of single-molecule molecular inversion probes derived data
>>
Single-molecule molecular inversion probes (SmMIPs) - tools is specifically tailored to address the high error rates associated with amplicon-based sequencing and support the implementation of cost-effective molecular inversion probes-based NGS.
By linking each sequence read to its probe-of-origin, AmMIP-tools can identify and filter error-prone reads, such as chimeric reads or those derived from self-annealing probes, that are uniquely associated with smMIP-based sequencing.

□ INTEGRATE: Model-based multi-omics data integration to characterize multi-level metabolic regulation
>> https://journals.plos.org/ploscompbiol/article?id=10.1371/journal.pcbi.1009337
INTEGRATE, a computational pipeline that integrates metabolomics and transcriptomics data, using constraint-based stoichiometric metabolic models as a scaffold.
INTEGRATE takes as input a generic metabolic network model, including GPRs, cross-sectional transcriptomics data, cross-sectional intracellular metabolomics data and steady-state extracellular fluxes data.

□ Genion: an accurate tool to detect gene fusion from long transcriptomics reads
>> https://bmcgenomics.biomedcentral.com/articles/10.1186/s12864-022-08339-5
Genion is an accurate gene fusion discovery tool that uses a combination of dynamic programming and statistical filtering. Genion accurately identifies the gene fusions and its clustering accuracy for detecting fusion reads is better than LongGF.
From the mapping of transcriptomic long reads to a reference genome, Genion first identifies chains of exons. Reads with chains that contain exons from several genes provide an initial set of reads supporting potential gene fusions.
Genion clusters the reads that indicate potential gene fusions to define fusion candidates using a statistical method based on the analysis of background expression patterns for the involved genes and on the co-occurrence of the fusion candidates in other potential fusion events.

□ uniPort: a unified computational framework for single-cell data integration with optimal transport
>> https://www.biorxiv.org/content/10.1101/2022.02.14.480323v1.full.pdf
uniPort, a unified single-cell data integration framework which combines coupled-VAE and Minibatch Unbalanced Optimal Transport. It leverages both highly variable common and dataset-specific genes for integration and is scalable to large-scale and partially overlapping datasets.
uniPort can further construct a reference atlas for online prediction across datasets. Meanwhile, uniPort provides a flexible label transfer framework to deconvolute spatial heterogeneous data using optimal transport space, instead of embedding latent space.

□ scGAC: a graph attentional architecture for clustering single-cell RNA-seq data
>> https://academic.oup.com/bioinformatics/advance-article-abstract/doi/10.1093/bioinformatics/btac099/6530275
scGAC (single-cell Graph Attentional Clustering), for scRNA-seq data. scGAC firstly constructs a cell graph and refines it by network denoising. scGAC adopts a self-optimizing method to obtain the cell clusters.
scGAC learns clustering-friendly representation of cells through a graph attentional autoencoder, which propagates information across cells with different weights and captures latent relationship among cells.

□ scITD: Tensor decomposition reveals coordinated multicellular patterns of transcriptional variation that distinguish and stratify disease individuals
>> https://www.biorxiv.org/content/10.1101/2022.02.16.480703v1.full.pdf
A joint decomposition would more naturally describe scenarios where different cell types respond specifically to the same external signals. It would also improve the ability to infer dependencies between transcriptional programs across cell types.
Single-cell Interpretable Tensor Decomposition (scITD) extracts “multicellular GE patterns” that vary acrossdifferent biological samples. The multicellular patterns inferred by scITD can be linked with various clinical annotations, technical batch effects, and other metadata.

□ AIscEA: Unsupervised Integration of Single-cell Gene Expression and Chromatin Accessibility via Their Biological Consistency
>> https://www.biorxiv.org/content/10.1101/2022.02.17.480279v1.full.pdf
AIscEA first defines a ranked similarity score to quantify the biological consistency between cell types across measurements. AIscEA then uses the ranked similarity score and a novel permutation test to identify the cell-type alignment across measurements.
AIscEA further utilizes graph alignment to align the cells across measurements. the graph alignment method uses the symmetric k nearest neighbor graph to characterize the low-dimensional manifold.

□ EMOGEA: ERROR MODELLED GENE EXPRESSION ANALYSIS PROVIDES A SUPERIOR OVERVIEW OF TIME COURSE RNA-SEQ MEASUREMENTS AND LOW COUNT GENE EXPRESSION
>> https://www.biorxiv.org/content/10.1101/2022.02.18.481000v1.full.pdf
EMOGEA, a principled framework for analyzing RNA-seq data that incorporates measurement uncertainty in the analysis, while introducing a special formulation for modelling data that are acquired as a function of time or other continuous variable.
EMOGEA yields gene expression profiles that represent groups of genes with similar modulations in their expression during embryogenesis. EMOGEA profiles highlight with clarity how the expression of different genes is modulated over time with a tractable biological interpretation.

□ scAnnotate: an automated cell type annotation tool for single-cell RNA-sequencing data
>> https://www.biorxiv.org/content/10.1101/2022.02.19.481159v1.full.pdf
scAnnotate uses a marginal mixture model to describe both the dropout proportion and the non-dropout expression level distribution of a gene.
A marginal model based ensemble learning approach is developed to avoid having to specify and estimate a high-dimensional joint distribution for all genes.
To address the curse of high dimensionality, they use every gene to make a classifier and consider it as a ‘weak’ learner, and then use a combiner function to ensemble ‘weak’ learners built from all genes into a single ‘strong’ learner for making the final decision.