(Art by Rui Huang)Vertical versionBlend file on patreon #b3d pic.twitter.com/tKJmQn1JYl
— Rui Huang (@RuiHuang_art) May 24, 2024

□ SSGATE: Single-cell multi-omics and spatial multi-omics data integration via dual-path graph attention auto-encoder
>> https://www.biorxiv.org/content/10.1101/2024.06.03.597266v1
SSGATE, a single-cell multi-omics and spatial multi-omics data integration method based on dual-path GATE. SSGATE constructs neighborhood graphs based on expression data and spatial information respectively, which is the key to its ability to process both single-cell and spatially resolved data.
In SSGATE architecture, the encoder consists of 2 graph attention layers. The attention mechanism is active in the first layer but inactive in the second. The decoder adopts a symmetrical structure w/ the encoder. The ReLU / Tanh functions are used for nonlinear transformation.


□ D3 - DNA Discrete Diffusion: Designing DNA With Tunable Regulatory Activity Using Discrete Diffusion
>> https://www.biorxiv.org/content/10.1101/2024.05.23.595630v1
DNA Discrete Diffusion (D3), a generative framework for conditionally sampling regulatory sequences with targeted functional activity levels. D3 can accept a conditioning signal, a scalar or vector, alongside the data as input to the score network.
D3 generates DNA sequences that better capture the diversity of cis-regulatory grammar. D3 employs a similar method with a different function for Bregman divergence.

□ scFoundation: Large-scale foundation model on single-cell transcriptomics
>> https://www.nature.com/articles/s41592-024-02305-7
scFoundation, a large-scale model that models 19,264 genes with 100 million parameters, pre-trained on over 50 million scRNA-seq data. It uses xTrimoGene, a scalable transformer-based model that incl. an embedding module and an asymmetric encoder-decoder structure.
scFoundation converts continuous gene expression scalars into learnable high-dimensional vectors. A read-depth-aware pre-training task enables scFoundation not only to model the gene co-expression patterns within a cell but also to link the cells w/ different read depths.

□ PSALM: Protein Sequence Domain Annotation using Language Models
>> https://www.biorxiv.org/content/10.1101/2024.06.04.596712v1
PSALM, a method to predict domains across a protein sequence at the residue-level. PSALM extends the abilities of self-supervised pLMs trained on hundreds of millions of protein sequences to protein sequence annotation with just a few hundred thousand annotated sequences.
PSALM provides residue-level annotations and probabilities at both the clan and family level, enhancing interpretability despite possible model uncertainty. The PSALM clan and family models are trained to minimize cross-entropy loss.

□ POLAR-seq: Combinatorial Design Testing in Genomes
>> https://www.biorxiv.org/content/10.1101/2024.06.06.597521v1
POLAR-seq (Pool of Long Amplified Reads sequencing) takes genomic DNA isolated from library pools and uses long range PCR to amplify target genomic regions.
The pool of long amplicons is then directly read by nanopore sequencing with full length reads then used to identify the gene content and structural variation of individual genotypes.
POLAR-seq allows rapid identification of structural rearrangements: duplications, deletions, inversions, and translocations. Genotypes are revealed by annotating each read with Liftoff, allowing the arrangement and content of the DNA parts in the synthetic region.

□ π-TransDSI: A protein sequence-based deep transfer learning framework for identifying human proteome-wide deubiquitinase-substrate interactions
>> https://www.nature.com/articles/s41467-024-48446-3
π-TransDSI is based on TransDSI architecture, which is a novel, sequence-based ab initio method that leverages explainable graph neural networks and transfer learning for deubiquitinase-substrate interaction (DSI) prediction.
TransDSI transfers intrinsic biological properties to predict the catalytic function of DUBs. TransDSI features an explainable module, allowing for accurate predictions of DSIs and the identification of sequence features that suggest associations between DUBs and substrates.

□ ULTRA: ULTRA-Effective Labeling of Repetitive Genomic Sequence
>> https://www.biorxiv.org/content/10.1101/2024.06.03.597269v1
ULTRA (ULTRA Locates Tandemly Repetitive Areas) models tandem repeats using a hidden Markov model. ULTRA's HMM uses a single state to represent non-repetitive sequence, and a collection of repetitive states that each model different repetitive periodicities.
ULTRA can annotate tandem repeats inside genomic sequence. It is able to find repeats of any length and of any period. ULTRA's implementation of Viterbi replaces emission probabilities with the ratio of model emission probability relative to the background frequency of letters.

□ Cell-Graph Compass: Modeling Single Cells with Graph Structure Foundation Model
>> https://www.biorxiv.org/content/10.1101/2024.06.04.597354v1
Cell-Graph Compass (CGC), a graph-based, knowledge-guided foundational model with large scale single-cell sequencing data. CGC conceptualizes each cell as a graph, with nodes representing the genes it contains and edges denoting the relationships between them.
CGC utilizes gene tokens as node features and constructs edges based on transcription factor-target gene Interactions, gene co-expression relationships, and genes' positional relationship on chromosome, with the GNN module to synthesize and vectorize these features.
CGC is pre-trained on fifty million human single-cell sequencing data from ScCompass-h50M. CGC employs a Graph Neural Network architecture. It utilizes the message-passing mechanisms along with self-attention mechanisms to jointly learn the embedding representations of all genes.
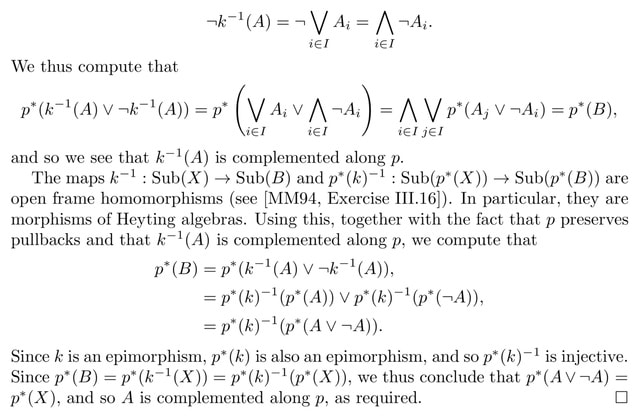
□ Existentially closed models and locally zero-dimensional toposes
>> https://arxiv.org/abs/2406.02788
The definition of locally zero-dimensional topos requires a choice of a generating set of objects, but like they have seen for s.e.c. geometric morphisms, there is a canonical choice if the topos is coherent.
Evidently, a topos is locally zero-dimensional if and only if there is a generating set of locally zero-dimensional objects, because each locally zero-dimensional object is covered by zero-dimensional objects.

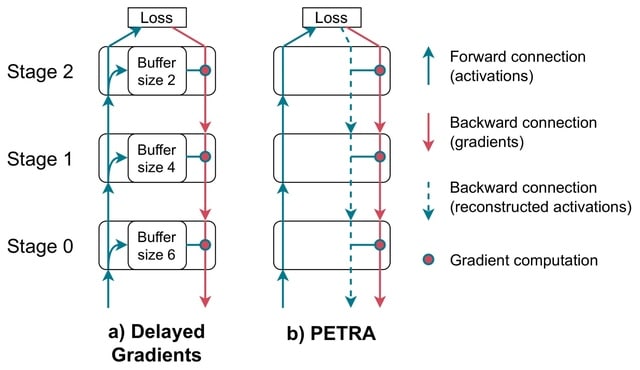
□ PETRA: Parallel End-to-end Training with Reversible Architectures
>> https://arxiv.org/abs/2406.02052
PETRA (Parallel End-to-End Training with Reversible Architectures), a novel method designed to parallelize gradient computations within reversible architectures. PETRA leverages a delayed, approximate inversion of activations during the backward pass.
By avoiding weight stashing and reversing the output into the input during the backward phase, PETRA fully decouples the forward and backward phases in all reversible stages, with no memory overhead, compared to standard delayed gradient approaches.

□ ProTrek: Navigating the Protein Universe through Tri-Modal Contrastive Learning
>> https://www.biorxiv.org/content/10.1101/2024.05.30.596740v1
ProTrek, a tri-modal protein language model, enables contrastive learning of protein sequence, structure, and function (SSF). ProTrek employs a pre-trained ESM encoder for its AA sequence language model and a pre-trained BERT encoder.
This tri-modal alignment training enables Pro-Trek to tightly associate SSE by bringing genuine sample pairs (sequence-structure, sequence-function, and structure-function) closer together while pushing negative samples farther apart in the latent space.
ProTrek employs global alignment via cross-modal contrastive learning. ProTrek significantly outperforms all sequence alignment tools and even surpasses Foldseek in terms of the number of correct hits.

□ IGEGRNS: Inferring gene regulatory networks from single-cell transcriptomics based on graph embedding
>> https://academic.oup.com/bioinformatics/advance-article/doi/10.1093/bioinformatics/btae291/7684950
IGEGRNS infers gene regulatory networks from scRNA-seq data through graph embedding. IGEGRNS converts the GRNs inference into a linkage prediction problem, determining whether there are regulatory edges between transcription factors and target genes.
IGEGRNS formulates gene-gene relationships, and learns low-dimensional embeddings of gene pairs using GraphSAGE. It aggregates neighborhood nodes to generate low-dimensional embedding. Meanwhile, Top-k pooling filters the top k nodes with the highest influence on the whole graph.
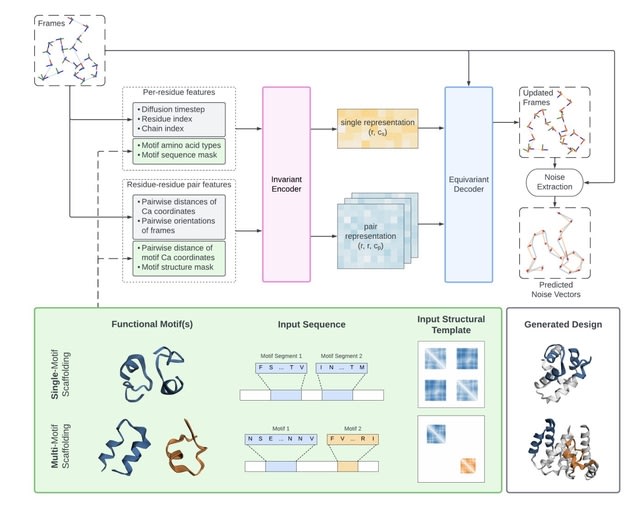
□ Genie2: massive data augmentation and model scaling for improved protein structure generation with (conditional) diffusion.
>> https://arxiv.org/abs/2405.15489
Genie 2 surpasses RFDiffusion on motif scaffolding tasks, both in the number of solved problems and the diversity of designs. Genie 2 can propose complex designs incorporating multiple functional motifs, a challenge unaddressed by existing protein diffusion models.
Genie 2 consists of an SE(3)-invariant encoder that transforms input features into single residue and pair residue-residue representations, and an SE(3)-equivariant decoder that updates frames based on single representations, pair representations, and input reference frames.


□ Bayesian Occam's Razor to Optimize Models for Complex Systems
>> https://www.biorxiv.org/content/10.1101/2024.05.28.594654v1
A method for optimizing models for complex systems by (i) minimizing model uncertainty; (ii) maximizing model consistency; and (iii) minimizing model complexity, following the Bayesian Occam's razor rationale.
Leveraging the Bayesian formalism, we establish definitive rules and propose quantitative assessments for the probability propagation from input models to the metamodel.


□ INSTINCT: Multi-sample integration of spatial chromatin accessibility sequencing data via stochastic domain translation
>> https://www.biorxiv.org/content/10.1101/2024.05.26.595944v1
INSTINCT, a method for multi-sample INtegration of Spatial chromaTIN accessibility sequencing data via stochastiC domain Translation. INSTINCT can efficiently handle the high dimensionality of spATAC-seq data and eliminate the complex noise and batch effects of samples.
INSTINCT trains a variant of graph attention autoencoder to integrate spatial information and epigenetic profiles, implements a stochastic domain translation procedure to facilitate batch correction, and obtains low-dimensional representations of spots in a shared latent space.

□ Genesis: A Modular Protein Language Modelling Approach to Immunogenicity Prediction
>> https://www.biorxiv.org/content/10.1101/2024.05.22.595296v1
Genesis a modular immunogenicity prediction protein language model based on the transformer architecture. Genesis comprises a pMHC sub-module, trained sequentially on multiple pMHC prediction tasks.
Genesis provides the input embeddings for an immunogenicity prediction head model to perform p.MHC-only immunogenicity prediction. Genesis is trained in an iterative manner and uses cross-validation in some optimization.

□ Attending to Topological Spaces: The Cellular Transformer
>> https://arxiv.org/abs/2405.14094
The Cellular Transformer (CT) generalizes the graph-based transformer to process higher-order relations within cell complexes. By augmenting the transformer with topological awareness through cellular attention, CT is inherently capable of exploiting complex patterns.
CT uses cell complex positional encodings and formulates self-attention / cross-attention in topological terms. Cochain spaces are used to process data supported over a cell complex. The k-cochains can be represented by means of eigenvector bases of corresponding Hodge Laplacian.

□ CodonBERT: a BERT-based architecture tailored for codon optimization using the cross-attention mechanism
>> https://academic.oup.com/bioinformatics/advance-article/doi/10.1093/bioinformatics/btae330/7681883
CodonBERT, an LLM which extends the BERT model and applies it to the language of mRNAs. CodonBERT uses a multi-head attention transformer architecture framework. The pre-trained model can also be generalized to a diverse set of supervised learning tasks.
CodonBERT takes the coding region as input using codons as tokens, and outputs an embedding that provides contextual codon representations. CodonBERT constructs the input embedding by concatenating codon, position, and segment embeddings.

□ Circular single-stranded DNA as a programmable vector for gene regulation in cell-free protein expression systems
>> https://www.nature.com/articles/s41467-024-49021-6
A programmable vector - circular single-stranded DNA (CssDNA) for gene expression in CFE systems. CssDNA can provide another route for gene regulation.
CssDNA can not only be engineered for gene regulation via the different pathways of sense CssDNA and antisense CssDNA, but also be constructed into several gene regulatory logic gates in CFE systems.

□ scG2P: Genotype-to-phenotype mapping of somatic clonal mosaicism via single-cell co-capture of DNA mutations and mRNA transcripts
>> https://www.biorxiv.org/content/10.1101/2024.05.22.595241v1
scG2P, a single-cell approach for the highly multiplexed capture of multiple recurrently mutated regions in driver genes to decipher mosaicism in solid tissue, while elucidating cell states with an mRNA readout.
scG2P can jointly capture genotype and phenotype at high accuracy. scG2P provides a novel platform to interrogate clonal diversification and the resulting cellular differentiation biases at the throughput necessary to address human clonal complexity.

□ scRNAkinetics: Inferring Single-Cell RNA Kinetics from Various Biological Priors
>> https://www.biorxiv.org/content/10.1101/2024.05.21.595179v1
scRNAkinetics leverages the pseudo-time trajectory derived from multiple biological priors combined with a specific RNA dynamic model to accurately infer the RNA kinetics for scRNA-seq datasets.
scRNAkinetics assumes each cell and its neighborhood have the same kinetic parameters and fit the kinetic parameters by forcing the earliest cell evolve into later cells on the pseudo-time axis.

□ GigaPath: A whole-slide foundation model for digital pathology from real-world data
>> https://www.nature.com/articles/s41586-024-07441-w
GigaPath, a novel vision transformer architecture for pretraining gigapixel pathology slides. To scale GigaPath for slide-level learning with tens of thousands of image tiles, GigaPath adapts the newly developed LongNet method to digital pathology.
Prov-GigaPath, a whole-slide pathology foundation model pretrained on 1.3 billion 256 × 256 pathology image tiles in 171,189 whole slides. Prov-GigaPath uses DINOv2 for tile-level pretraining. Prov-GigaPath generates contextualized embeddings.

□ POASTA: Fast and exact gap-affine partial order alignment
>> https://www.biorxiv.org/content/10.1101/2024.05.23.595521v1
POASTA's algorithm is based on an alignment graph, enabling the use of common graph traversal algorithms such as the A* algorithm to compute alignments. POASTA enables the construction of megabase-length POA graphs.
POASTA accelerates alignment using the A* algorithm, a depth-first search component, greedily aligning exact matches b/n the query and the graph; and a method to detect and prune alignment states that are not part of the optimal solution, informed by the POA graph topology.

□ MNMST: topology of cell networks leverages identification of spatial domains from spatial transcriptomics data
>> https://genomebiology.biomedcentral.com/articles/10.1186/s13059-024-03272-0
MNMST constructs cell spatial network by exploiting indirect relations among cells and learns cell expression network by using self-representation learning (SRL) with local preservation constraint.
MNMST jointly factorizes cell multi-layer networks with non-negative matrix factorization by projecting cells into a common subspace. It automatically learns cell expression networks by utilizing SRL with local preservation constraint by exploiting augmented expression profiles.

□ BioIB: Identifying maximally informative signal-aware representations of single-cell data using the Information Bottleneck
>> https://www.biorxiv.org/content/10.1101/2024.05.22.595292v1
biolB, a single-cell tailored method based on the IB algorithm, providing a compressed, signal-informative representation of single-cell data. The compressed representation is given by metagenes, which are clustered probabilistic mapping of genes.
The probabilistic construction preserves gene-level biological interpretability, allowing characterization of each metagene. biolB generates a hierarchy of these metagenes, reflecting the inherent data structure relative to the signal of interest.
The biolB hierarchy facilitates the interpretation of metagenes, elucidating their significance in distinguishing between biological labels and illustrating their interrelations with both one another and the underlying cellular populations.

□ MMDPGP: Bayesian model-based method for clustering gene expression time series with multiple replicates
>> https://www.biorxiv.org/content/10.1101/2024.05.23.595463v1
In the context of clustering, a Dirichlet process (DP) is used to generate priors for a Dirichlet process mixture model (DPMM) which is a mixture model that accounts for a theoretically infinite number of mixture components.
MMDPGP (Multiple Models Gaussian process Dirichlet process), a Bayesian model-based method for clustering transcriptomics time series data with multiple replicates. This technique is based on sampling Gaussian processes within an infinite mixture model from a Dirichlet process.
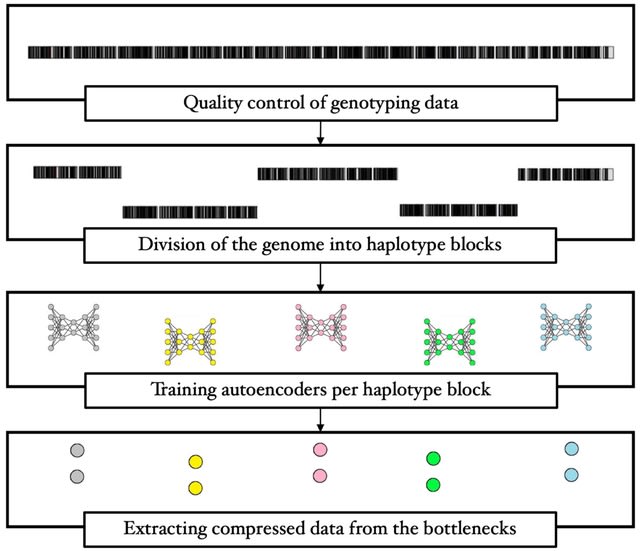
□ Computing linkage disequilibrium aware genome embeddings using autoencoders
>> https://academic.oup.com/bioinformatics/advance-article/doi/10.1093/bioinformatics/btae326/7679649
A method to compress single nucleotide polymorphism (SNP) data, while leveraging the linkage disequilibrium (LD) structure and preserving potential epistasis. They provide an adjustable autoencoder design to accommodate diverse blocks and bypass extensive hyperparameter tuning.
This method involves clustering correlated SNPs into haplotype blocks and training per-block autoencoders to learn a compressed representation of the block's genetic content.

□ Establishing a conceptual framework for holistic cell states and state transitions
>> https://www.cell.com/cell/fulltext/S0092-8674(24)00461-6
Defining a stable holistic cell state and state transitions via a conceptual visualization of a dynamic, spring-connected tetrahedron. The bi-directional feedback is represented by springs connecting each pair of observables
All of the combinations of all of the observables across the four categories that can actually exist as a holistic cell state manifold of observables within the very high-dimensional space of all theoretical observables.
This manifold is largest if all possible cell states, including abnormal or pathological, are considered and most constrained within the controlled environment of a developing multicellular organism.
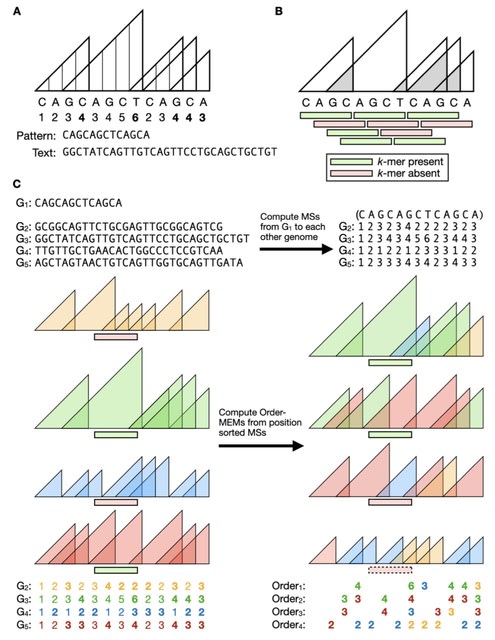
□ MEMO: MEM-based pangenome indexing for k-mer queries
>> https://www.biorxiv.org/content/10.1101/2024.05.20.595044v1
MEMO (Maximal Exact Match Ordered), a pangenome indexing method based on maximal exact matches (MEMs) between sequences. A single MEMO index can handle arbitrary-length queries over pangenomic windows.
If the pangenome consists of N genome sequences, a k-mer membership query returns a length-N vector of true/ false values indicating the presence/ absence of the k-mer in each genome.

□ scCDC: a computational method for gene-specific contamination detection and correction in single-cell and single-nucleus RNA-seq data
>> https://genomebiology.biomedcentral.com/articles/10.1186/s13059-024-03284-w
scCDC (single-cell Contamination Detection and Correction), which first detects the “contamination-causing genes,” which encode the most abundant ambient RNAs, and then only corrects these genes’ measured expression levels.
scCDC improved the accuracy of identifying cell-type marker genes and constructing gene co-expression networks. scCDC excelled in robustness and decontamination accuracy for correcting highly contaminating genes, while it avoids over-correction for lowly/non-contaminating genes.

□ iResNetDM: Interpretable deep learning approach for four types of DNA modification prediction
>> https://www.biorxiv.org/content/10.1101/2024.05.19.594892v1
iResNetDM, which, to the best of our knowledge, is the first deep learning model designed to predict specific types of DNA modifications rather than merely detecting the presence of modifications.
iResNetDM integrates a Residual Network with a self-attention mechanism. The incorporation of ResNet blocks facilitates the extraction of local features. iResNetDM exhibits significant enhancements in performance, achieving high accuracy across all DNA modification types.

□ GCRTcall: a Transformer based basecaller for nanopore RNA sequencing enhanced by gated convolution and relative position embedding via joint loss training
>> https://www.biorxiv.org/content/10.1101/2024.06.03.597255v1
GCRTcall, a novel approach integrating Transformer architecture with gated convolutional networks and relative positional encoding for RNA sequencing signal decoding.
GCRTcall is trained using a joint loss approach and is enhanced with gated depthwise separable convolution and relative position embeddings. GCRTcall incorporates additional forward and backward Transformer decoders at the top, utilizing the joint loss for improved convergence.
GCRTcall combines relative positional embedding with a multi-head self-attention mechanism. They integrate depthwise separable convolutions based on gate mechanisms to process the outputs of attention layers, it enhances the model’s ability to capture local sequence dependencies.

□ DICE: Fast and Accurate Distance-Based Reconstruction of Single-Cell Copy Number Phylogenies
>> https://www.biorxiv.org/content/10.1101/2024.06.03.597037v1
DICE-bar (Distance-based Inference of Copy-number Evolution using breakpoint-root distance) is a "Copy Number Alteration aware" approach that utilizes breakpoints between adjacent copy number bins to estimate the number of CNA events.
DICE-star (Distance-based Inference of Copy-number Evolution using standard-root distance) utilizes a simple penalized Manhattan distance between the copy number profiles themselves. Both methods use the Minimum Evolution criterion to reconstruct the final cell lineage tree.