
Two simple rules:1) Fill empty spaces2) Don't hit anything pic.twitter.com/5FjkjfRARx
— Adam Swaab (@adamswaab) October 30, 2021

□ MetaGraph: Lossless Indexing with Counting de Bruijn Graphs
>> https://www.biorxiv.org/content/10.1101/2021.11.09.467907v1.full.pdf
Together with the underlying graph, these annotations make up a data structure which we call a Counting de Bruijn graph. It can be used to represent quantitative information and, in particular, encode traces of the input sequences in de Bruijn graphs.
The concept of Counting de Bruijn graphs generalizing the notion of annotated (or colored) de Bruijn graphs. Counting de Bruijn graphs supplement each node-label relation with one or many attributes. Extended sequence-to-graph alignment algorithm introduced in MetaGraph.

□ Fast and Optimal Sequence-to-Graph Alignment Guided by Seeds
>> https://www.biorxiv.org/content/10.1101/2021.11.05.467453v1.full.pdf
An implementation of the seed heuristic as part of the AStarix aligner, that exploits information from the whole read to quickly align it to a general graphs reference, and guides the search by placing crumbs on nodes that lead towards optimal alignments even for long reads.
AStarix rephrases the task of alignment as a shortest-path problem in an alignment graph extended by a trie index, and solves it using the A⋆ algorithm instantiated with a problem- specific prefix heuristic.

□ scDeepHash: An automatic cell type annotation and cell retrieval method for large-scale scRNA-seq datasets using neural network-based hashing
>> https://www.biorxiv.org/content/10.1101/2021.11.08.467820v1.full.pdf
scDeepHash, a scalable scRNA- seq analytic tool that employs content-based deep hashing to index single-cell gene expressions. scDeepHash allows for fast and accurate automated cell-type annotation and similar-cell retrieval.
scDeepHash leverages the properties of Hadamard matrix for the cell anchor generation. And enforcing minimum information loss when quantizing continuous codes into discrete binary hash codes. scDeepHash formulates the two losses as Weighted Cell-Anchor Loss and Quantization Loss.

□ scGate: marker-based purification of cell types from heterogeneous single-cell RNA-seq datasets
>> https://www.biorxiv.org/content/10.1101/2021.11.08.467740v1.full.pdf
scGate purifies a cell population of interest using a set of markers organized in a hierarchical structure, akin to gating strategies employed in flow cytometry.
scGate automatically synchronizes its internal database of gating models. scGate takes as input a gene expression matrix or Seurat object and a “gating model” (GM), consisting of a set of marker genes that define the cell population of interest.

□ ENGRAM: Multiplex genomic recording of enhancer and signal transduction activity in mammalian cells
>> https://www.biorxiv.org/content/10.1101/2021.11.05.467434v1.full.pdf
ENGRAM (ENhancer-driven Genomic Recording of transcriptional Activity in Multiplex), an alternative paradigm in which the activity and dynamics of multiple transcriptional reporters are stably recorded to DNA.
ENGRAM is based on the prime editing-mediated insertion of signal- or enhancer-specific barcodes to a genomically encoded recording unit. this strategy can be used to concurrently genomically record the relative activity of at least hundreds of enhancers.

□ Block aligner: fast and flexible pairwise sequence alignment with SIMD-accelerated adaptive blocks
>> https://www.biorxiv.org/content/10.1101/2021.11.08.467651v1.full.pdf
Block aligner greedily shifts and grows a block of computed scores to span large gaps w/ the aligned sequences. This greedy approach is able to only compute a fraction of the DP matrix. Since differences b/n cells are small, this allows for maximum parallelism with SIMD vectors.
Block aligner uses the Smith-Waterman-Gotoh algorithm, along with its global variant, the Needleman-Wunsch algorithm. They are dynamic programming that computes the optimal alignment of two sequences in a O(|q||r|) matrix along with the transition directions (trace).

□ Sincast: a computational framework to predict cell identities in single cell transcriptomes using bulk atlases as references
>> https://www.biorxiv.org/content/10.1101/2021.11.07.467660v1.full.pdf
Sincast is a computational framework to query scRNA-seq data based on bulk reference atlases. Single cell data are transformed to be directly comparable to bulk data, either with pseudo-bulk aggregation or graph-based imputation to address sparse single cell expression profiles.
Sincast avoids batch effect correction, and cell identity is predicted along a continuum to highlight new cell states not found in the reference atlas. Sincast projects single cells into the correct biological niches in the expression space of the bulk reference atlas.

□ Spacemake: processing and analysis of large-scale spatial transcriptomics data
>> https://www.biorxiv.org/content/10.1101/2021.11.07.467598v1.full.pdf
Spacemake is designed to handle all major spatial transcriptomics datasets and can be readily configured to run on other technologies. It can process and analyze several samples in parallel, even if they stem from different experimental methods.
Spacemake enables reproducible data processing from raw data to automatically generated downstream analysis. Spacemake is built with a modular design and offers additional functionality such as sample merging, saturation analysis and analysis of long-reads as separate modules.

□ Weak SINDy for partial differential equations
>> https://www.sciencedirect.com/science/article/pii/S0021999121004204
a learning algorithm for the threshold in sequential-thresholding least-squares (STLS) that enables model identification from large libraries, and utilizing scale invariance at the continuum level to identify PDEs from poorly-scaled datasets.
WSINDy algorithm for identification of PDE systems using the weak form of the dynamics has a worst-case computational complexity of O(N^D+1log(N) for datasets with N points in each of D+1 dimensions.
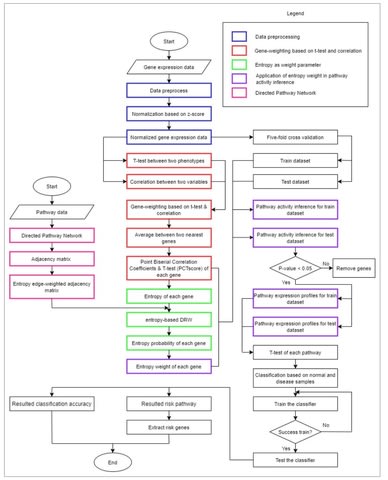
□ e-DRW: An Entropy-based Directed Random Walk for Pathway Activity Inference Using Topological Importance and Gene Interactions
>> https://www.biorxiv.org/content/10.1101/2021.11.05.467449v1.full.pdf
the entropy-based Directed Random Walk (e-DRW) method to quantify pathway activity using both gene interactions and information indicators based on the probability theory.
Moreover, the expression value of the member genes are inferred based on the t-test statistics scores and correlation coefficient values, whereas, the entropy weight method (EWM) calculates the activity score of each pathway.
The merged directed pathway network utilises e-DRW to evaluate the topological importance of each gene. An equation was proposed to assess the connectivity of nodes in the directed graph via probability values calculated from the Shannon entropy formula.

□ EntropyHub: An open-source toolkit for entropic time series analysis
>> https://journals.plos.org/plosone/article?id=10.1371/journal.pone.0259448
Numerous variants have since been derived from conditional entropy, and to a lesser extent Shannon’s entropy, to estimate the information content of time series data across various scientific domains, resulting in what has recently been termed “the entropy universe”.
EntropyHub (Ver. 0.1) provides an extensive range of more than forty functions for estimating cross-, multiscale, multiscale cross-, and bidimensional entropy, each incl. a number of keyword arguments that allows the user to specify multiple parameters in the entropy calculation.

□ QT-GILD: Quartet Based Gene Tree Imputation Using Deep Learning Improves Phylogenomic Analyses Despite Missing Data
>> https://www.biorxiv.org/content/10.1101/2021.11.03.467204v1.full.pdf
QT-GILD is an automated and specially tailored unsupervised deep learning technique, accompanied by cues from natural languageprocessing (NLP), which learns the quartet distribution in a given set of incomplete gene trees andgenerates a complete set of quartets accordingly.
QT-GILD obviates the need for a reference tree as well as accounts for gene tree estimation error. QT- GILD is a general-purpose approach which requires no explicit modeling of the reasons of gene tree heterogeneity or missing data, making it less vulnerable to model mis-specification.
QT-GILD measures the divergence between true quartet distributions and different sets of quartet distributions in estimated gene trees (e.g., complete, incomplete and imputed) in terms of the number of “dominant” quartets that differ between two quartet distributions.
QT-GILD tries to learn the overall quartet distribution guided by a self-supervised feedback loop and correct for gene tree estimation error, investigating its application beyond incomplete gene trees in order to improve estimated gene tree distributions would be an interesting direction to take.

□ EFMlrs: a Python package for elementary flux mode enumeration via lexicographic reverse search
>> https://bmcbioinformatics.biomedcentral.com/articles/10.1186/s12859-021-04417-9
Recently, Avis et al. developed mplrs—a parallel version of the lexicographic reverse search (lrs) algorithm, which, in principle, enables an EFM analysis on high-performance computing environments.
EFMlrs uses COBRApy to process metabolic models from sbml files, performs loss-free compressions of the stoichiometric matrix. the enumeration of EFM/Vs in metabolic networks is a vertex enumeration problem in convex polyhedra.

□ Hofmann-Mislove theorem for approach spaces
>> https://arxiv.org/pdf/2111.02665v1.pdf
The Hofmann-Mislove theorem says that compact saturated sets of a sober topological space correspond bijectively to open filters of its open set lattice. They concerns an analogy of this result for approach spaces.
It is shown that for a sober approach space, the inhabited and saturated compact functions correspond bijectively to the proper open [0,∞]-filters of the metric space of its upper regular functions, which is an analogy of the Hofmann-Mislove theorem for approach spaces.

□ Classification of pre-Jordan Algebras and Rota-Baxter Operators on Jordan Algebras in Low Dimensions
>> https://arxiv.org/pdf/2111.02035v1.pdf
The equations involving structural constants of Jordan algebras are “cubic”. it is difficult to give all solutions of these equations as well as give the corresponding classification in the sense of isomorphism, even more for pre-Jordan algebras since they involve two identities.
Classifying complex pre-Jordan algebras and give Rota-Baxter operators (of weight zero) on complex Jordan algebras in dimensions ≤ 3.

□ Oriented and unitary equivariant bordism of surfaces
>> https://arxiv.org/pdf/2111.02693v1.pdf
an alternative proof of the fact that surfaces with free actions (of groups of odd order in the oriented case) which in-duce non-trivial elements in the Bogomolov multiplier of the group cannot equivariantly bound.
Surfaces without 0-dimensional fixed points: Let us then denote by ΩG2 the subgroup of ΩG2 generated by manifolds without isolated fixed points, and whose underlying Euler characteristic is zero in the unitary case.

□ Hausdorff dimension of sets with restricted, slowly growing partial quotients
>> https://arxiv.org/pdf/2111.02694v1.pdf
the set of irrational numbers in (0, 1) whose partial quotients an tend to infinity is of Hausdorff dimension 1/2. a precise asymptotics of the Hausdorff dimension of this set as q → ∞ using the thermo-dynamic formalism.
for an arbitrary B and an arbitrary f with values in [min B, ∞) and tending to infinity, the set of irrational numbers in (0, 1) such that
an ∈B, an ≤f(n) for all n∈N, and an → ∞ asn→∞ is of Hausdorff dimension τ(B)/2, where τ(B) is the exponent of convergence of B.
Constructing a sequence of Bernoulli measures with non-uniform weights, supported on finitely many 1-cylinders indexed by elements of B and having dimensions (the Kolmogorov-Sinai entropy divided by the Lyapunov exponent) not much smaller than τ(B)/2.

□ HATTUSHA: Multiplex Embedding of Biological Networks Using Topological Similarity of Different Layers
>> https://www.biorxiv.org/content/10.1101/2021.11.05.467392v1.full.pdf
HATTUSHA formulates an optimization problem that accounts for inner-network smoothness, intra-network smoothness, and topological similarity of networks to compute diffusion states for each network using Gromov-Wasserteins discrepancy.
HATTUSHA integrates the resulting diffusion states and apply dimensionality reduction - singular value decomposition after log-transformation to compute node embeddings.

□ IEPWRMkmer: An Information-Entropy Position-Weighted K-Mer Relative Measure for Whole Genome Phylogeny Reconstruction
>> https://www.frontiersin.org/articles/10.3389/fgene.2021.766496/full
Using the Shannon entropy of the feature matrix to determine the optimal value of K. And can obtain an N×4K feature matrix for a dataset with N genomes. The optimal K is the value at which score(K) reaches its maximum.
IEPWRMkmer, An Information-Entropy Position-Weighted K-Mer Relative Measure, a new alignment-free method which combines the position-weighted measure of k-mers and the information entropy of frequency of k-mers to obtain phylogenetic information for sequence comparison.

□ Nyströmformer: A Nystöm-based Algorithm for Approximating Self-Attention
>> https://www.ncbi.nlm.nih.gov/pmc/articles/PMC8570649/
Nyströmformer – a model that exhibits favorable scalability as a function of sequence length. Nyströmformer is based on adapting the Nyström method to approximate standard self-attention with O(n) complexity.
NyströmFormer algorithm makes use of landmark (or Nyström) points to reconstruct the softmax matrix in self-attention, thereby avoiding computing the n × n softmax matrix. The scalability of Nyströmformer enables application to longer sequences with thousands of tokens.

□ AWGAN: A Powerful Batch Correction Model for scRNA-seq Data
>> https://www.biorxiv.org/content/10.1101/2021.11.08.467781v1.full.pdf
AWGAN, a new deep learning framework based on Wasserstein Generative Adversarial Network (WGAN) combined with an attention mechanism to reduce the differences among batches.
AWGAN can remove the batch effect in different datasets and preserve the biological variation. AWGAN adopts a strategy of confrontation training to improve the ability of the two models and finally achieve the Nash equilibrium.
□ Optimizing weighted gene co-expression network analysis with a multi-threaded calculation of the topological overlap matrix
>> https://www.degruyter.com/document/doi/10.1515/sagmb-2021-0025/html
The WGCNA R software package uses an Adjacency Matrix to store a network, next calculates the Topological Overlap Matrix (TOM), and then identifies the modules (sub-networks), where each module is assumed to be associated with a certain biological function.
the single-threaded algorithm of the TOM has been changed into a multi-threaded algorithm (the default values of WGCNA). In the multi-threaded algorithm, Rcpp was used to make R call a C++ function, and then C++ used OpenMP to calculate TOM from the Adjacency Matrix.

□ Nature Reviews Genetics RT
Functional genomics data: privacy risk assessment and technological mitigation
>> https://www.nature.com/articles/s41576-021-00428-7
>> https://twitter.com/naturerevgenet/status/1458732446560800769?s=21
This Perspective highlights privacy issues related to the sharing of functional genomics data, including genotype and phenotype information leakage from different functional genomics data types and their summarization steps.

□ DeepKG: An End-to-End Deep Learning-Based Workflow for Biomedical Knowledge Graph Extraction, Optimization and Applications
>> https://academic.oup.com/bioinformatics/advance-article-abstract/doi/10.1093/bioinformatics/btab767/6425667
To improve the performance of DeepKG, a cascaded hybrid information extraction framework (CHIEF) is developed for training model of 3-tuple extraction, and a novel AutoML-based knowledge representation algorithm (AutoTransX) is proposed for knowledge representation and inference.
For link prediction in knowledge graph learning (KGL), the core problem is to learn the relations between 3-tuples, where a triplet includes the embedding vectors of two entities (head and tail) and one relation.
AutoTransX is a data-driven method to address this issue, which automatically combines several candidate operations of 3-tuples in traditional methods to represent the relations in biomedical KGL accurately.
CHIEF is a cascaded hybrid information extraction framework, which extracts relational 3-tuples as a whole and learns both entities and relations through a joint encoder. a fine-tuned deep bidirectional Transformer (BERT) has been utilized to capture the contextual information.
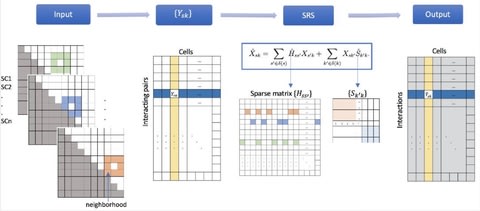
□ scHiCSRS: A Self-Representation Smoothing Method with Gaussian Mixture Model for Imputing single-cell Hi-C Data
>> https://www.biorxiv.org/content/10.1101/2021.11.09.467824v1.full.pdf
scHiCSRS, a self-representation smoothing method that improves the data quality, and a Gaussian mixture model that identifies structural zeros among observed zeros.
scHiCSRS takes spatial dependencies of scHi-C 2D data structure into consideration while also borrows information from similar single cells. scHiCSRS was motivated by scTSSR that recovers scRNA data using a two-sided sparse self-representation method.
□ From shallow to deep: exploiting feature-based classifiers for domain adaptation in semantic segmentation
>> https://www.biorxiv.org/content/10.1101/2021.11.09.467925v1.full.pdf
a Convolutional Neural Networks can be trained to correct the errors of the Random Forest in the source domain and then be applied to correct such errors in the target domain without retraining, as the domain shift b/n the RF predictions is much smaller than between the raw data.
This method can be classified as source-free domain adaption, but the additional feature-based learning step allows us to avoid training set estimation or reconstruction.
a new Random Forest from a few brushstroke labels and simply apply the pre-trained Prediction Enhancer (PE) network to improve the probability maps.

□ MEP: Improving Neural Networks for Genotype-Phenotype Prediction Using Published Summary Statistics
>> https://www.biorxiv.org/content/10.1101/2021.11.09.467937v1.full.pdf
main effect prior (MEP), a new regularization method for making use of GWAS summary statistics from external datasets. The main effect prior is generally applica- ble for machine learning algorithms, such as neural networks and linear regression.
a tractable solution by accessing the summary statistics from another large study. Since the main effects of SNPs have already been captured by GWAS summary statistics on the large external dataset in MEP(external), using MEP is especially beneficial for high-dimensional data.

□ Combining dictionary- and rule-based approximate entity linking with tuned BioBERT
>> https://www.biorxiv.org/content/10.1101/2021.11.09.467905v1.full.pdf
a two-stage approach as follows usage of fine-tuned BioBERT for identification of chemical entities semantic approximate search in MeSH and PubChem databases for entity linking.
This mainly affects the new entities that are not part of the base vocabulary of BERT’s WordPiece tokenizer, resulting into multiple splitting of sub-tokens.
□ REDigest: a Python GUI for In-Silico Restriction Digestion Analysis of Genes or Complete Genome Sequences
>> https://www.biorxiv.org/content/10.1101/2021.11.09.467873v1.full.pdf
REDigest is a fast, user-interactive and customizable software program which can perform in- silico restriction digestion analysis on a multifasta gene or a complete genome sequence file.
REDigest can process Fasta and Genbank format files as input and can write output file for sequence information in Fasta or Genbank format. Validation of the restriction fragment or the terminal restriction fragment size and taxonomy against a database.
□ A2Sign: Agnostic algorithms for signatures — a universal method for identifying molecular signatures from transcriptomic datasets prior to cell-type deconvolution
>> https://academic.oup.com/bioinformatics/advance-article-abstract/doi/10.1093/bioinformatics/btab773/6426077
A2Sign is a global framework that can be applied to uncover molecular signatures for cell type deconvolution in arbitrary tissues using bulk transcriptome data.
A2Sign: Agnostic Algorithms for Signatures, based on a non-negative tensor factorization strategy that allows us to identify cell type-specific molecular signatures, greatly reduce collinearities, and also account for inter-individual variability.

□ Scalable inference of transcriptional kinetic parameters from MS2 time series data
>> https://academic.oup.com/bioinformatics/advance-article-abstract/doi/10.1093/bioinformatics/btab765/6426074
scalable implementation of the cpHMM for fast inference of promoter activity and transcriptional kinetic parameters. This new method can model genes of arbitrary length through the use of a time-adaptive truncated compound state space.
The truncated state space provides a good approximation to the full state space by retaining the most likely set of states at each time during the forward pass of the algorithm.

□ bollito: a flexible pipeline for comprehensive single-cell RNA-seq analyses
>> https://academic.oup.com/bioinformatics/advance-article-abstract/doi/10.1093/bioinformatics/btab758/6426066
bollito is an automated, flexible and parallelizable computational pipeline for the comprehensive analysis of single-cell RNA-seq data. bollito performs both basic and advanced tasks in single-cell analysis integrating over 30 state-of-the-art tools.
bollito is built using the Snakemake workflow management system includes quality control, read alignment, dimensionality reduction, clustering, cell-marker detection, differential expression, functional analysis, trajectory inference and RNA velocity.
□ MatrixQCvis: shiny-based interactive data quality exploration for omics data
>> https://academic.oup.com/bioinformatics/advance-article/doi/10.1093/bioinformatics/btab748/6426067
In high-throughput quantitative omics experiments, after initial processing, the data are typically presented as a matrix of numbers (feature IDs × samples).
Efficient and standardized data-quality metrics calculation and visualization are key to track the within-experiment quality of these rectangular data types and to guarantee for high-quality data sets and subsequent biological question-driven inference.
MatrixQCvis, which provides interactive visualization of data quality metrics at the per-sample and per-feature level using R’s shiny framework. It provides efficient and standardized ways to analyze data quality of quantitative omics data types that come in a matrix-like format.