□ この世界には、事象と方法の二通りのパースペクティブしかない。この二つはコインの裏表でネガポジの関係である。依って概念や方法の開発は、それ自体で科学となる。しかしエビデンスの欠損は罪である。既存の構造を効率化できるものとして、意味はあるが価値はないものの選択圧は重要である。それが存在理由と言ってもいい。プロセスを踏んで結果を積み上げるだけではなく、磨耗し打ち消しあうことこそが本質なのだ。私たちは、傷跡だらけの化石に刻まれたテクストの中を泳ぐ存在である。
□ SF小説やスペースオペラは、我々が日常体験しうる感覚や概念を拡張し、それを違う時間軸や遠い惑星系の物語の流れに組み込もうとする意識活動ではあるが、その意味合い的な本質論はさておいて、あくまで「人間の主観が追体験しうる」という点で、舞台は違えど他のフィクションと大差ない。
あるいはジョイスの『Finnegans Wake』の手法を更に推し進めて、叙述の主体や対象が未知の概念で構築されてたらどうか。空間、駆動体、時系列、法則などが我々の宇宙とは別の、例えば球体を一度回転しただけでは、空間系が定常に戻らない世界。それは我々の日常と相似を為すかどうか。然しながら『叙述しうる』という点に於いて、意味論的にはレトリックとアナロジーの違いにしか成り得ないのかもしれない。では表現できるということは、表象するという行為はなんなのだろう。
私たちはアプリオリに他者との関係性を担う。存在自体が表現なのだから、発語の必要性自体はアポステリオリに決定する。叙述とは信号の転写であり、空間上の潜在的ポテンシャルと同義となる。記憶と表現は、滝が流れ落ちるように、或いは遡るように、相補的に転写され、共有されるグリッドを描くのだ。
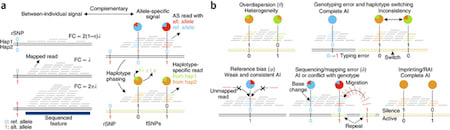
□ Fine-mapping cellular QTLs with RASQUAL and ATAC-seq:
>> http://www.nature.com/ng/journal/vaop/ncurrent/full/ng.3467.html
RASQUAL (Robust Allele-Specific Quantitation and Quality Control), a new statistical approach for association mapping that models genetic effects and accounts for biases in sequencing data using a single, probabilistic framework. For simplicity, RASQUAL assumes a single causal variant at each feature, although multiple causal variants can be tested for by conditioning on the lead SNP genotype. RASQUAL lead SNPs in the top 500 CTCF QTLs 2.5-fold more likely be “motif-disrupting” compared to simple linear regression.
□ MAST: a flexible statistical framework for assessing transcriptional changes in single-cell RNA sequencing data:
□ http://genomebiology.biomedcentral.com/articles/10.1186/s13059-015-0844-5
MAST is suitable for supervised analyses about differential expression of genes and gene modules, as well as unsupervised analyses of model residuals, to generate hypotheses regarding co-expression of genes. shrink the gene-specific variance estimates to a global estimate of the variance using an empirical Bayes method.
Let τ g 2 be the precision (1/variance) for Y g |Z g = 1 in gene g. We suppose τ g 2 ∼ Gamma(α, β),
find the joint likelihood (across genes), and integrate out the gene-specific inverse variances.
A gene module score for cell i is the average of the scores for the genes contained in the module, that is, ∑{j ∈ module} s ij /|module|.
MAST has greatest efficiency when the continuous (log)-expression is normally distributed, transformations (such as the Box-Cox) could also be applied if the non-zero continuous measurements are skewed.

(Distribution of starting position and lengths for i-tRFs. positions of the internal tRNA fragments, span & lengths.)
□ Dissecting tRNA-derived fragment complexities using personalized transcriptomes reveals novel fragment classes
>> http://www.impactjournals.com/oncotarget/index.php?journal=oncotarget&page=article&op=view&path[]=4695&path[]=10723
a novel category of tRNA fragments that significantly contribute to the differences we observe across tissues, genders, populations, & races. these fragments, referred to as i-tRFs, are abundant in human tissues, wholly internal to the respective tRNA, can straddle the anticodon. HITS-CLIP data analysis revealed that tRNA fragments are loaded on Argonaute in a cell-dependent manner, suggesting cell-dependent functional roles through the RNA interference pathway.
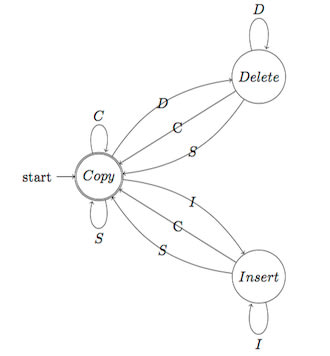
□ Streaming algorithms for identification of pathogens and antibiotic resistance from real-time MinION sequencing:
>> http://biorxiv.org/content/biorxiv/early/2015/12/09/019356.full.pdf
Schematic of a three-state probabilistic Finite State Machine: In order to assess an alignment of 2-sequences A & B, under a hypothesis specified by the parameters, the pFSM computes the cost to generate one sequence (say A) given the other (B). an information-theoretic measure where the cost of a transition is that of encoding the generated base, or in other words, the negative logarithm of the probability of the associated action (c = -log2(P(a)). In the E-step, the best alignment (lowest cost) is calculated by a dynamic programming algorithm. The frequencies of actions at each state are then used to re-estimate the probabilities in the M-step.
□ Quantitative flow analysis of swimming dynamics with coherent Lagrangian vortices:
>> http://scitation.aip.org/content/aip/journal/chaos/25/8/10.1063/1.4919784
investigating to what extent the momentum change of the swimmer can be attributed to that of the coherent structures in the swimmer's wake. the contribution of discrete coherent Lagrangian vortices to the momentum balance of two different modes of swimming propulsion, impulsive C-start escape, and steady anguilliform swimming. Coherent Lagrangian vortices are regions enclosed by outermost members of nested families of λ-lines. λ-lines are curves that uniformly stretch or shrink by a small amount over a predefined finite time interval. Outermost closed λ-lines define the boundaries of coherent fluid patches and, in particular, the boundaries of coherent vortices.
□ D-GEX: Gene expression inference with deep learning:
>> http://biorxiv.org/content/biorxiv/early/2015/12/15/034421.full.pdf
mean absolute error averaged across all genes, deep learning significantly outperforms linear regression with 15.33% relative improvement. A gene-wise comparative analysis shows that deep learning achieves lower error than linear regression in 99.97% of the target genes. the performance of D-GEX learned model on an independent RNA-Seq-based GTEx dataset, which consists of 2,921 expression profiles. Deep learning still outperforms linear regression with 6.57% relative improvement, and achieves lower error in 81.31% of the target genes.
cross platforms generalizability implies the great potential of D- GEX to be applied to the LINCS program where training and prediction were also done separately on the microarray data and the L1000 data. The uniform distribution of the output layer of D-GEX was set to be within a smaller range, [-1 × 10^-4, 1 × 10^-4] as it was adopted with the linear activation function. Learning rate was initialized to 5 × 10^-4 or 3 × 10^-4 depending on different architectures, and was decreased according to the training error on a subset of GEO-tr for monitoring the training process.

Different ANN models’ Cross-entropy scores for predicting FB generated targets on the validation set.
□ Automatic genome segmentation with HMM-ANN hybrid models:
>> http://biorxiv.org/content/early/2015/12/16/034579
By using the hybrid models, able to obtain much higher precisions while maintain similar recalls compared with the HMM-GMM boot model. The hybrid models based on the VT generated targets give the best performance while using a 2-hidden layer ANN does not improve the performance over a 1-hidden layer ANN.

□ Learning in cortical networks through error back-propagation:
>> http://biorxiv.org/content/early/2015/12/28/035451
the predictive coding model corresponding to back-propagation assumes that output samples are generated from a probabilistic model with multiple layers of random variables, but most of the noise is added only at the level of output samples (i.e. Σ(0) >> Σ(l>0))
□ 10X Genomics Founders Prevail in Arbitration Dispute With Bio-Rad:
>> https://www.genomeweb.com/business-news/10x-genomics-founders-prevail-arbitration-dispute-bio-rad
RainDance Technologies sued the company in the US District Court for the District of Delaware accusing 10X of infringing six of its patents.
□ SPECtre: a spectral coherence-based classifier of actively translated transcripts from ribosome profiling seq data:
>> http://biorxiv.org/content/early/2015/12/17/034777
the median SPECtre score was calculated over 30 nucleotide overlapping windows, with 3 nucleotides stepped to the next window in order to capture the canonical reading frame. Full Transcript Spectral Coherence, FLOSS, and ORFscore will follow the same generalized output format as that of SPECtre.
□ Bayesian molecular clock dating of species divergences in the genomics era:
>> http://www.nature.com/nrg/journal/vaop/ncurrent/abs/nrg.2015.8.html
Bayesian clock dating methodology and the explosive accumulation of genetic sequence data, molecular clock dating has found widespread, from tracking virus pandemics and the macroevolutionary process of speciation and extinction to estimating a timescale for life on Earth.
□ DanQ: a hybrid convolutional and recurrent deep neural network for quantifying the function of DNA sequences:
>> http://biorxiv.org/content/early/2015/12/20/032821
DanQ is a hybrid framework that combines CNNs and BLSTMs, the framework uses the same features and data as the DeepSEA framework. Whereas the convolution step of the DeepSEA contains 3 convolution layers and 2 max pooling layers in alternating order to learn motifs, the convolution step of the DanQ model is much simpler and contains one convolution layer and one max pooling layer to learn motifs.
a recurrent layer after the max pooling layer is that motifs can follow a regulatory “grammar” governed by physical constraints that dictate the in vivo spatial arrangements and frequencies of combinations of motifs. DanQ-JASPAR contains 1024 convolution kernels, took 30 epochs to fully train, and each epoch of training takes approximately 12 hours.

□ Fischer decomposition for polynomials on superspace:
>> http://scitation.aip.org/content/aip/journal/jmp/56/11/10.1063/1.4935362
the Fischer decomposition of polynomials on Rm|2n into spherical harmonics is obtained unless M ∈ - 2N0. For a given superdimension M, we define the set of exceptional indices by IM = 0̸ if M ∉ - 2N0, and IM={k∈N0|2-M/2≤k≤2-M} if M∈-2N0.

□ Prediction of Synergism from Chemical-Genetic Interactions by Machine Learning:
>> http://www.cell.com/cell-systems/abstract/S2405-4712(15)00217-3
a software toolset to predict chemical synergies, collectively called the Second Order Naive Bayesian and Random Forest (SONAR) suite. a network-based algorithm that integrates chemical-genetic data with genetic interactions to predict the target genes interactions, proteins for each compound and concomitantly synergistic chemical chemical interactions.

Synergy Prediction Based on Chemical-Genetic and Genetic Interactions: Synergy Prediction Based on Chemical-Genetic and Genetic Interactions: Sentinel strains sensitive to cryptagen c represent first order connections s. Second order connections t are inferred from genetic interactions of sentinel strains and ranked by interactions with sentinel strains in s. ROCs for the single property Exy (AUC = 0.64) and for synergy scores based on SONARGNR parameters (AUC = 0.87).

□ Rail-dbGaP: a protocol and tool for analyzing protected genomic data in a commercial cloud:
>> http://biorxiv.org/content/early/2015/12/24/035287
Rail-dbGaP, a protocol for analyzing dbGaP data in the cloud using Elastic MapReduce (EMR), an AWS service. Rail-RNA v0.2 spliced aligner for RNA-seq data, demonstrate by running on 9,662 samples from the dbGaP-protected GTEx consortium dataset. In the Rail-dbGaP protocol, an administrator grants the user only those privileges required to run Hadoop programs on EMR clusters. The administrator uses multi-factor authentication & constrains the user to set up a password satisfying NIH’s requirements in the document.
□ Line Cross Analysis approach to estimating the composite genetic effects contributing to variation among generation:
>> http://www.ncbi.nlm.nih.gov/pubmed/26704183
Software for Analysis of Genetic Architecture (SAGA), which comprehensively assesses the potential model space, quantifies model selection uncertainty, and uses model weighted averaging to accurately estimate composite genetic effects.
□ scDD: A statistical approach for identifying differential distributions in single-cell RNA-seq experiments:
>> http://biorxiv.org/content/early/2015/12/29/035501
scDD is the first statistical method to detect differences in scRNA-seq experiments that explicitly accounts for potential multi-modality. scDD calculates a Bayes Factor for the evidence that the data arises from two independent condition-specific models, (differential distributions (DD)) versus one overall model that ignores condition (equivalent distributions).
□ Canu 1.0: the Celera Assembler designed for high-noise single-molecule sequencing (PacBio RSII or MinION)
>> https://github.com/marbl/canu/releases
Canu using the MinHash Alignment Process (MHAP) for overlapping noisy, long reads using probabilistic, locality-sensitive hashing. Canu supports Sun/Open/Univa Grid Engine (SGE), Portable Batch System (PBS), Platform Computing’s Load Sharing Facility (LSF) and the SLURM.
□ Biological screens from linear codes: Reed-Solomon codes, and more generally linear codes.
>> http://biorxiv.org/content/early/2015/12/25/035352
a pooling design based on Reed-Solomon (RS) error correcting codes that dramatically reduces the number of pools compared to the pooling designs widely used in biological experiments. In coding theory, a code is a set of vectors that differ pairwise in a large number of places. RS codes are linear codes, based on polynomials over finite fields.

□ Regmex: Motif analysis in ranked lists of sequences
>> http://biorxiv.org/content/early/2016/01/05/035956
Regmex uses regular expressions to define motifs & embedded Markov models to calculate exact probabilities for motif observations. A central point in the way Regmex calculates a motif RCP is to calculate SSPs for observing the motif the observed number of times (nobs) Briefly, from a deterministic finite state automaton (DFA) associated with the RE motif, identify a sequence specific transition probability matrix (TPM) which is used to build an embedded TPM (eTPM) specific for n(obs). Regmex can accurately evaluate rank correlation significance for arbitrary combined sets of simple motifs.

□ Circlator: automated circularization of genome assemblies using long sequencing reads:
>> http://www.genomebiology.com/2015/16/1/294

□ Mining genetic variation in any species with GEMINI:
>> https://speakerdeck.com/arq5x/mining-genetic-variation-in-any-species-with-gemini
Improve speed for WGS datasets, use GQT: 500x faster than bcftools and 1800x faster for PCA than Google Genomics. 10 million ExAC variants annotated with 34 annotations from 11 distinct files in ~30 minutes. current gemini takes at least 40X longer to load the same number of variants.

□ Beyond comparisons of means: understanding changes in gene expression at single-cell level:
>> http://biorxiv.org/content/early/2016/01/05/035949
□ BASiCS: Bayesian Analysis of Single-Cell Sequencing Data:
>> https://github.com/catavallejos/BASiCS
a statistical approach for the comparison of expression patterns between P pre-specified populations of cells. It builds upon BASiCS, a Bayesian model for the analysis of scRNA-seq data. the examples described here focus on the detection of genes whose absolute LFC in biological cell-to-cell heterogeneity between populations, p and p′ exceeds a minimum tolerance threshold ω0, i.e. when | log(δip/δip′ )| > ω0, for a given ω0 ≥ 0. using the decision rule | log(μip/μip′ )| > τ0, where τ0 ≥ 0 is an a priori chosen threshold for LFC in over- all expression.
□ Illumina's New sequencer Miniseq perspective
>> http://omicsomics.blogspot.jp/2016/01/miniseq.html
>> http://www.illumina.com/systems/miniseq.html?linkId=20273579
MiniSeq. $50k, 4-24 hours, up to 2x150 bp, 2-7.5 Gbp.
□ 10X Genomics to Unveil New Single-Cell Genetic Analysis Product:
>> http://www.businesswire.com/news/home/20160111005369/en/10X-Genomics-Unveil-Single-Cell-Genetic-Analysis-Product
10X’s new single-cell product will enable detailed gene-expression profiling on a cell-by-cell basis. The instrument runtime is just 10 minutes and costs one dollar or less per cell. Researchers can produce 40,000 barcoded single-cell cDNA libraries in a single run.
□ Parallel single-cell profiling:
>> http://www.ebi.ac.uk/about/news/press-releases/parallel-single-cell-profiling
a method for parallel single-cell genome-wide methylome and transcriptome sequencing that allows for the discovery of associations between transcriptional and epigenetic variation.
□ SF小説やスペースオペラは、我々が日常体験しうる感覚や概念を拡張し、それを違う時間軸や遠い惑星系の物語の流れに組み込もうとする意識活動ではあるが、その意味合い的な本質論はさておいて、あくまで「人間の主観が追体験しうる」という点で、舞台は違えど他のフィクションと大差ない。
あるいはジョイスの『Finnegans Wake』の手法を更に推し進めて、叙述の主体や対象が未知の概念で構築されてたらどうか。空間、駆動体、時系列、法則などが我々の宇宙とは別の、例えば球体を一度回転しただけでは、空間系が定常に戻らない世界。それは我々の日常と相似を為すかどうか。然しながら『叙述しうる』という点に於いて、意味論的にはレトリックとアナロジーの違いにしか成り得ないのかもしれない。では表現できるということは、表象するという行為はなんなのだろう。
私たちはアプリオリに他者との関係性を担う。存在自体が表現なのだから、発語の必要性自体はアポステリオリに決定する。叙述とは信号の転写であり、空間上の潜在的ポテンシャルと同義となる。記憶と表現は、滝が流れ落ちるように、或いは遡るように、相補的に転写され、共有されるグリッドを描くのだ。
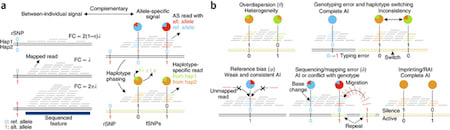
□ Fine-mapping cellular QTLs with RASQUAL and ATAC-seq:
>> http://www.nature.com/ng/journal/vaop/ncurrent/full/ng.3467.html
RASQUAL (Robust Allele-Specific Quantitation and Quality Control), a new statistical approach for association mapping that models genetic effects and accounts for biases in sequencing data using a single, probabilistic framework. For simplicity, RASQUAL assumes a single causal variant at each feature, although multiple causal variants can be tested for by conditioning on the lead SNP genotype. RASQUAL lead SNPs in the top 500 CTCF QTLs 2.5-fold more likely be “motif-disrupting” compared to simple linear regression.
□ MAST: a flexible statistical framework for assessing transcriptional changes in single-cell RNA sequencing data:
□ http://genomebiology.biomedcentral.com/articles/10.1186/s13059-015-0844-5
MAST is suitable for supervised analyses about differential expression of genes and gene modules, as well as unsupervised analyses of model residuals, to generate hypotheses regarding co-expression of genes. shrink the gene-specific variance estimates to a global estimate of the variance using an empirical Bayes method.
Let τ g 2 be the precision (1/variance) for Y g |Z g = 1 in gene g. We suppose τ g 2 ∼ Gamma(α, β),
find the joint likelihood (across genes), and integrate out the gene-specific inverse variances.
A gene module score for cell i is the average of the scores for the genes contained in the module, that is, ∑{j ∈ module} s ij /|module|.
MAST has greatest efficiency when the continuous (log)-expression is normally distributed, transformations (such as the Box-Cox) could also be applied if the non-zero continuous measurements are skewed.

(Distribution of starting position and lengths for i-tRFs. positions of the internal tRNA fragments, span & lengths.)
□ Dissecting tRNA-derived fragment complexities using personalized transcriptomes reveals novel fragment classes
>> http://www.impactjournals.com/oncotarget/index.php?journal=oncotarget&page=article&op=view&path[]=4695&path[]=10723
a novel category of tRNA fragments that significantly contribute to the differences we observe across tissues, genders, populations, & races. these fragments, referred to as i-tRFs, are abundant in human tissues, wholly internal to the respective tRNA, can straddle the anticodon. HITS-CLIP data analysis revealed that tRNA fragments are loaded on Argonaute in a cell-dependent manner, suggesting cell-dependent functional roles through the RNA interference pathway.
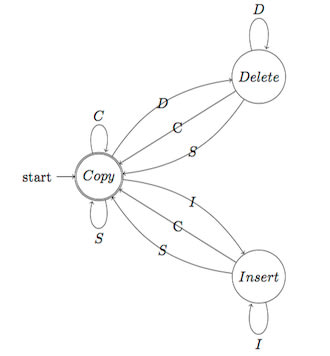
□ Streaming algorithms for identification of pathogens and antibiotic resistance from real-time MinION sequencing:
>> http://biorxiv.org/content/biorxiv/early/2015/12/09/019356.full.pdf
Schematic of a three-state probabilistic Finite State Machine: In order to assess an alignment of 2-sequences A & B, under a hypothesis specified by the parameters, the pFSM computes the cost to generate one sequence (say A) given the other (B). an information-theoretic measure where the cost of a transition is that of encoding the generated base, or in other words, the negative logarithm of the probability of the associated action (c = -log2(P(a)). In the E-step, the best alignment (lowest cost) is calculated by a dynamic programming algorithm. The frequencies of actions at each state are then used to re-estimate the probabilities in the M-step.
□ Quantitative flow analysis of swimming dynamics with coherent Lagrangian vortices:
>> http://scitation.aip.org/content/aip/journal/chaos/25/8/10.1063/1.4919784
investigating to what extent the momentum change of the swimmer can be attributed to that of the coherent structures in the swimmer's wake. the contribution of discrete coherent Lagrangian vortices to the momentum balance of two different modes of swimming propulsion, impulsive C-start escape, and steady anguilliform swimming. Coherent Lagrangian vortices are regions enclosed by outermost members of nested families of λ-lines. λ-lines are curves that uniformly stretch or shrink by a small amount over a predefined finite time interval. Outermost closed λ-lines define the boundaries of coherent fluid patches and, in particular, the boundaries of coherent vortices.
□ D-GEX: Gene expression inference with deep learning:
>> http://biorxiv.org/content/biorxiv/early/2015/12/15/034421.full.pdf
mean absolute error averaged across all genes, deep learning significantly outperforms linear regression with 15.33% relative improvement. A gene-wise comparative analysis shows that deep learning achieves lower error than linear regression in 99.97% of the target genes. the performance of D-GEX learned model on an independent RNA-Seq-based GTEx dataset, which consists of 2,921 expression profiles. Deep learning still outperforms linear regression with 6.57% relative improvement, and achieves lower error in 81.31% of the target genes.
cross platforms generalizability implies the great potential of D- GEX to be applied to the LINCS program where training and prediction were also done separately on the microarray data and the L1000 data. The uniform distribution of the output layer of D-GEX was set to be within a smaller range, [-1 × 10^-4, 1 × 10^-4] as it was adopted with the linear activation function. Learning rate was initialized to 5 × 10^-4 or 3 × 10^-4 depending on different architectures, and was decreased according to the training error on a subset of GEO-tr for monitoring the training process.

Different ANN models’ Cross-entropy scores for predicting FB generated targets on the validation set.
□ Automatic genome segmentation with HMM-ANN hybrid models:
>> http://biorxiv.org/content/early/2015/12/16/034579
By using the hybrid models, able to obtain much higher precisions while maintain similar recalls compared with the HMM-GMM boot model. The hybrid models based on the VT generated targets give the best performance while using a 2-hidden layer ANN does not improve the performance over a 1-hidden layer ANN.

□ Learning in cortical networks through error back-propagation:
>> http://biorxiv.org/content/early/2015/12/28/035451
the predictive coding model corresponding to back-propagation assumes that output samples are generated from a probabilistic model with multiple layers of random variables, but most of the noise is added only at the level of output samples (i.e. Σ(0) >> Σ(l>0))
□ 10X Genomics Founders Prevail in Arbitration Dispute With Bio-Rad:
>> https://www.genomeweb.com/business-news/10x-genomics-founders-prevail-arbitration-dispute-bio-rad
RainDance Technologies sued the company in the US District Court for the District of Delaware accusing 10X of infringing six of its patents.
□ SPECtre: a spectral coherence-based classifier of actively translated transcripts from ribosome profiling seq data:
>> http://biorxiv.org/content/early/2015/12/17/034777
the median SPECtre score was calculated over 30 nucleotide overlapping windows, with 3 nucleotides stepped to the next window in order to capture the canonical reading frame. Full Transcript Spectral Coherence, FLOSS, and ORFscore will follow the same generalized output format as that of SPECtre.
□ Bayesian molecular clock dating of species divergences in the genomics era:
>> http://www.nature.com/nrg/journal/vaop/ncurrent/abs/nrg.2015.8.html
Bayesian clock dating methodology and the explosive accumulation of genetic sequence data, molecular clock dating has found widespread, from tracking virus pandemics and the macroevolutionary process of speciation and extinction to estimating a timescale for life on Earth.
□ DanQ: a hybrid convolutional and recurrent deep neural network for quantifying the function of DNA sequences:
>> http://biorxiv.org/content/early/2015/12/20/032821
DanQ is a hybrid framework that combines CNNs and BLSTMs, the framework uses the same features and data as the DeepSEA framework. Whereas the convolution step of the DeepSEA contains 3 convolution layers and 2 max pooling layers in alternating order to learn motifs, the convolution step of the DanQ model is much simpler and contains one convolution layer and one max pooling layer to learn motifs.
a recurrent layer after the max pooling layer is that motifs can follow a regulatory “grammar” governed by physical constraints that dictate the in vivo spatial arrangements and frequencies of combinations of motifs. DanQ-JASPAR contains 1024 convolution kernels, took 30 epochs to fully train, and each epoch of training takes approximately 12 hours.

□ Fischer decomposition for polynomials on superspace:
>> http://scitation.aip.org/content/aip/journal/jmp/56/11/10.1063/1.4935362
the Fischer decomposition of polynomials on Rm|2n into spherical harmonics is obtained unless M ∈ - 2N0. For a given superdimension M, we define the set of exceptional indices by IM = 0̸ if M ∉ - 2N0, and IM={k∈N0|2-M/2≤k≤2-M} if M∈-2N0.

□ Prediction of Synergism from Chemical-Genetic Interactions by Machine Learning:
>> http://www.cell.com/cell-systems/abstract/S2405-4712(15)00217-3
a software toolset to predict chemical synergies, collectively called the Second Order Naive Bayesian and Random Forest (SONAR) suite. a network-based algorithm that integrates chemical-genetic data with genetic interactions to predict the target genes interactions, proteins for each compound and concomitantly synergistic chemical chemical interactions.

Synergy Prediction Based on Chemical-Genetic and Genetic Interactions: Synergy Prediction Based on Chemical-Genetic and Genetic Interactions: Sentinel strains sensitive to cryptagen c represent first order connections s. Second order connections t are inferred from genetic interactions of sentinel strains and ranked by interactions with sentinel strains in s. ROCs for the single property Exy (AUC = 0.64) and for synergy scores based on SONARGNR parameters (AUC = 0.87).

□ Rail-dbGaP: a protocol and tool for analyzing protected genomic data in a commercial cloud:
>> http://biorxiv.org/content/early/2015/12/24/035287
Rail-dbGaP, a protocol for analyzing dbGaP data in the cloud using Elastic MapReduce (EMR), an AWS service. Rail-RNA v0.2 spliced aligner for RNA-seq data, demonstrate by running on 9,662 samples from the dbGaP-protected GTEx consortium dataset. In the Rail-dbGaP protocol, an administrator grants the user only those privileges required to run Hadoop programs on EMR clusters. The administrator uses multi-factor authentication & constrains the user to set up a password satisfying NIH’s requirements in the document.
□ Line Cross Analysis approach to estimating the composite genetic effects contributing to variation among generation:
>> http://www.ncbi.nlm.nih.gov/pubmed/26704183
Software for Analysis of Genetic Architecture (SAGA), which comprehensively assesses the potential model space, quantifies model selection uncertainty, and uses model weighted averaging to accurately estimate composite genetic effects.
□ scDD: A statistical approach for identifying differential distributions in single-cell RNA-seq experiments:
>> http://biorxiv.org/content/early/2015/12/29/035501
scDD is the first statistical method to detect differences in scRNA-seq experiments that explicitly accounts for potential multi-modality. scDD calculates a Bayes Factor for the evidence that the data arises from two independent condition-specific models, (differential distributions (DD)) versus one overall model that ignores condition (equivalent distributions).
□ Canu 1.0: the Celera Assembler designed for high-noise single-molecule sequencing (PacBio RSII or MinION)
>> https://github.com/marbl/canu/releases
Canu using the MinHash Alignment Process (MHAP) for overlapping noisy, long reads using probabilistic, locality-sensitive hashing. Canu supports Sun/Open/Univa Grid Engine (SGE), Portable Batch System (PBS), Platform Computing’s Load Sharing Facility (LSF) and the SLURM.
□ Biological screens from linear codes: Reed-Solomon codes, and more generally linear codes.
>> http://biorxiv.org/content/early/2015/12/25/035352
a pooling design based on Reed-Solomon (RS) error correcting codes that dramatically reduces the number of pools compared to the pooling designs widely used in biological experiments. In coding theory, a code is a set of vectors that differ pairwise in a large number of places. RS codes are linear codes, based on polynomials over finite fields.

□ Regmex: Motif analysis in ranked lists of sequences
>> http://biorxiv.org/content/early/2016/01/05/035956
Regmex uses regular expressions to define motifs & embedded Markov models to calculate exact probabilities for motif observations. A central point in the way Regmex calculates a motif RCP is to calculate SSPs for observing the motif the observed number of times (nobs) Briefly, from a deterministic finite state automaton (DFA) associated with the RE motif, identify a sequence specific transition probability matrix (TPM) which is used to build an embedded TPM (eTPM) specific for n(obs). Regmex can accurately evaluate rank correlation significance for arbitrary combined sets of simple motifs.

□ Circlator: automated circularization of genome assemblies using long sequencing reads:
>> http://www.genomebiology.com/2015/16/1/294

□ Mining genetic variation in any species with GEMINI:
>> https://speakerdeck.com/arq5x/mining-genetic-variation-in-any-species-with-gemini
Improve speed for WGS datasets, use GQT: 500x faster than bcftools and 1800x faster for PCA than Google Genomics. 10 million ExAC variants annotated with 34 annotations from 11 distinct files in ~30 minutes. current gemini takes at least 40X longer to load the same number of variants.

□ Beyond comparisons of means: understanding changes in gene expression at single-cell level:
>> http://biorxiv.org/content/early/2016/01/05/035949
□ BASiCS: Bayesian Analysis of Single-Cell Sequencing Data:
>> https://github.com/catavallejos/BASiCS
a statistical approach for the comparison of expression patterns between P pre-specified populations of cells. It builds upon BASiCS, a Bayesian model for the analysis of scRNA-seq data. the examples described here focus on the detection of genes whose absolute LFC in biological cell-to-cell heterogeneity between populations, p and p′ exceeds a minimum tolerance threshold ω0, i.e. when | log(δip/δip′ )| > ω0, for a given ω0 ≥ 0. using the decision rule | log(μip/μip′ )| > τ0, where τ0 ≥ 0 is an a priori chosen threshold for LFC in over- all expression.
□ Illumina's New sequencer Miniseq perspective
>> http://omicsomics.blogspot.jp/2016/01/miniseq.html
>> http://www.illumina.com/systems/miniseq.html?linkId=20273579
MiniSeq. $50k, 4-24 hours, up to 2x150 bp, 2-7.5 Gbp.
□ 10X Genomics to Unveil New Single-Cell Genetic Analysis Product:
>> http://www.businesswire.com/news/home/20160111005369/en/10X-Genomics-Unveil-Single-Cell-Genetic-Analysis-Product
10X’s new single-cell product will enable detailed gene-expression profiling on a cell-by-cell basis. The instrument runtime is just 10 minutes and costs one dollar or less per cell. Researchers can produce 40,000 barcoded single-cell cDNA libraries in a single run.
□ Parallel single-cell profiling:
>> http://www.ebi.ac.uk/about/news/press-releases/parallel-single-cell-profiling
a method for parallel single-cell genome-wide methylome and transcriptome sequencing that allows for the discovery of associations between transcriptional and epigenetic variation.