
If áll time is etérnally présent
all time is únredéemable.

□ HyperHMM: Efficient inference of evolutionary and progressive dynamics on hypercubic transition graphs
>> https://academic.oup.com/bioinformatics/advance-article/doi/10.1093/bioinformatics/btac803/6895098
HyperHMM, an adapted Baum-Welch (expectation maximisation) algorithm for hypercubic inference with resampling to quantify uncertainty, and it allows orders-of-magnitude faster inference while making few practical sacrifices compared to previous hypercubic inference approaches.
The HyperHMM algorithm proceeds by iteratively estimating forward and backward probabilities of the different transitions observed in the dataset, given a current estimate of the hypercubic transition matrix.
Hypercubic inference learns the transition probabilities, finding the parameterisation most compatible with a set of emitted observations. It can be interpreted as a probability map of which feature is likely acquired at which stage, explicit pathways through the hypercube space.


□ Hypergraphs and centrality measures identifying key features in gene expression data
>> https://www.biorxiv.org/content/10.1101/2022.12.18.518108v1
The hypergraph modelling approach presented is designed to interrogate a data set, consisting of a structured collection of labelled multi-dimensional data records. Each data record is tested against a list of conditions of interest, giving a sequence of Boolean results.
The vertices of the hypergraph will correspond to the conditions and the hyperedges will correspond to the data records, with a hyperedge incident with a vertex if the discrete object satisfies the given condition.
The 2-multiplicity hyperedge, with distinct intersection pattern, forms a pendant vertex and strictly center around comparisons between the agravitropic and gravitropic phenotype.
Robust distance measures were obtained by representing hypergraphs in terms of s-line graphs. This definition of distance enabled the calculation of multiple centrality measures, with particular emphasis on betweenness and eigencentrality.

□ MIDAS: a deep generative model for mosaic integration and knowledge transfer of single-cell multimodal data
>> https://www.biorxiv.org/content/10.1101/2022.12.13.520262v1
MIDAS (the mosaic integration and knowledge transfer) simultaneously achieves dimensionality reduction, imputation, and batch correction of single-cell trimodal mosaic data by employing self-supervised modality alignment and information-theoretic latent disentanglement.
MIDAS uses self-supervised learning to align different modalities in latent space, and improving cross-modal inference. The scalable inference of MIDAS is achieved by the Stochastic Gradient Variational Bayes (SGVB), which enables “rectangular integration” and atlas construction.

□ HydRA: Deep-learning models for predicting RNA-binding capacity from protein interaction association context and protein sequence
>> https://www.biorxiv.org/content/10.1101/2022.12.23.521837v1
HydRA enables Occlusion Mapping to robustly detect known RNA-binding domains and to predict hundreds of uncharacterized RNA-binding domains. HydRA scores are highly correlated with the number of experimental studies that identify a given RBP as cross-linkable to RNA.
The HydRA algorithm applies an ensemble learning method that integrates convolutional neural network, Transformer and SVM in RBP prediction by utilizing both intermolecular protein context and sequence-level information.
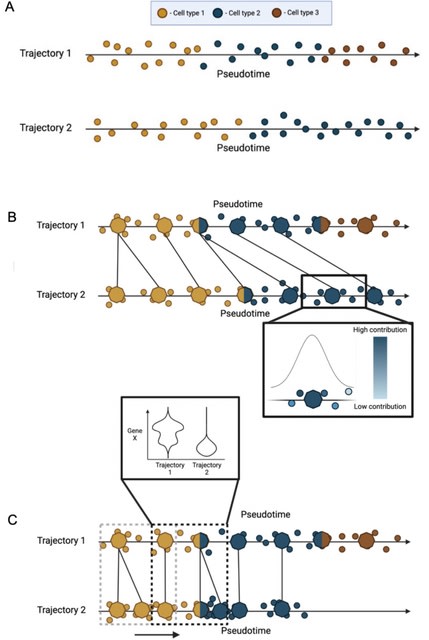

□ TrAGEDy: Trajectory Alignment of Gene Expression Dynamics
>> https://www.biorxiv.org/content/10.1101/2022.12.21.521424v1
TrAGEDy makes post-hoc changes to the alignment, allowing us to overcome the limitations of Dynamic Time Warping. TrAGEDy aligns the pseudotime of the interpolated points then the cells, and performes a sliding window comparison b/n cells at similar points in aligned pseudotime.
TrAGEDy finds the optimal path through the dissimilarity matrix of the interpolated points, which constitutes the shared process between the two trajectories. DTW, with alterations, is used to find the optimal path.
Another constraint of DTW is that all points must be matched to at least one other point; post-DTW pruned any matches that have high transcriptional dissimilarity, enabling processes which may have diverged in the middle of their respective trajectories.

□ XCVATR: detection and characterization of variant impact on the Embeddings of single -cell and bulk RNA-sequencing samples
>> https://bmcgenomics.biomedcentral.com/articles/10.1186/s12864-022-09004-7
XCVATR performs a multiscale analysis of the distance matrices to identify variant clumps. XCVATR performs a scale selection to tune the analysis to the cell–cell distance metric. XCVATR identifies Nν cells that are closest to it, and defining the close neighborhood of each cell.
XCAVTR builds a matrix and computes the estimated alternative AF. XCVATR performs a cell-centered analysis, wherein it does not aim to model the whole embedding space, but rather focuses on the cells. XCVATR identifies the medians of the minimum and maximum radii over all cells.

□ LuxHMM: DNA methylation analysis with genome segmentation via Hidden Markov Model
>> https://www.biorxiv.org/content/10.1101/2022.12.20.521327v1
LuxHMM, a probabilistic method that uses hidden Markov model (HMM) to segment the genome into regions and a Bayesian regression model, which allows handling of multiple covariates, to infer differential methylation of regions.
LuxHMM determines hypo- and hypermethylated regions. LuxHMM enables to describe the underlying biochemistry in bisulfite sequencing and model inference is done using either automatic differentiation variational inference for genome-scale analysis or Hamiltonian Monte Carlo.

□ Asteroid: a new algorithm to infer species trees from gene trees under high proportions of missing data
>> https://academic.oup.com/bioinformatics/advance-article/doi/10.1093/bioinformatics/btac832/6964379
Asteroid, a novel algorithm that infers an unrooted species tree from a set of unrooted gene trees. Asteroid is substantially more accurate than ASTRAL and ASTRID for very high proportions of missing data.
Asteroid is parallelized, and can take as input multi-furcating gene trees. Asteroid computes for input gene tree a distance matrix based on the gene internode distance. It computes a species tree from this set of distance matrices under the minimum balanced evolution principle.

□ Liam tackles complex multimodal single-cell data integration challenges
>> https://www.biorxiv.org/content/10.1101/2022.12.21.521399v1
Liam (leveraging information across modalities) is a model for the simultane- ous horizontal / vertical integration of paired multimodal single-cell data. Liam learns a joint low-dimensional representation of two concurrently measured modalities.
Liam integrates the accounts for complex batch effects using CVAE / AVAE and can be optimized using replicate information. Liam employs a logistic-normal distribution for the latent cell variable, making the latent factor loadings interpretable as probabilities.

□ scTensor detects many-to-many cell-cell interactions from single cell RNA-sequencing data
>> https://www.biorxiv.org/content/10.1101/2022.12.07.519225v1
scTensor, a novel method for extracting representative triadic relationships incl. ligand / receptor expression, and related L-R pairs. scTensor detects hypergraphs that cannot be detected using conventional CCI detection, especially when they incl. many-to-many relationships.
scTensor constructs the CCI-tensor, decomposes the tensor by the NTD-2 algorithm. scTensor estimates the NTD-2 ranks for each matricized CCI-tensor. Because NMF is performed in each matricized CCI-tensor, each rank of NMF are estimated based on the residual sum of squares.

□ NPGREAT: assembly of human subtelomere regions with the use of ultralong nanopore reads and linked-reads
>> https://bmcbioinformatics.biomedcentral.com/articles/10.1186/s12859-022-05081-3
NanoPore Guided REgional Assembly Tool (NPGREAT) combines Linked-Read data with mapped ultralong nanopore reads spanning subtelomeric segmental duplications to potentially overcome these difficulties.
Linked-Read sets of DNA sequences identified by matches with 1-copy subtelomere sequence adjacent to segmental duplications are assembled and extended into the segmental duplication regions using Regional Extension of Assemblies using Linked-Reads (REXTAL).
REXTAL contig alignment with the cognate nanopore read sequence is monitored and alignment discrepancies above a given threshold. Mapped telomere-containing ultralong nanopore reads are used to provide contiguity and correct orientation for matching REXTAL sequence.

□ SC3s: efficient scaling of single cell consensus clustering to millions of cells
>> https://bmcbioinformatics.biomedcentral.com/articles/10.1186/s12859-022-05085-z
SC3s takes as input the gene-by-cell expression matrix, after preprocessing and dimensionality reduction via PCA using Scanpy commands. SC3s attempts to combine the results of multiple clustering runs, where the number of principal components is changed.
All this information is then encoded into a binary matrix, which can be efficiently used to produce the final k cell clusters. The key difference from the original SC3 is that for each d, the cells are first grouped into microclusters which can be reused for multiple values of K.
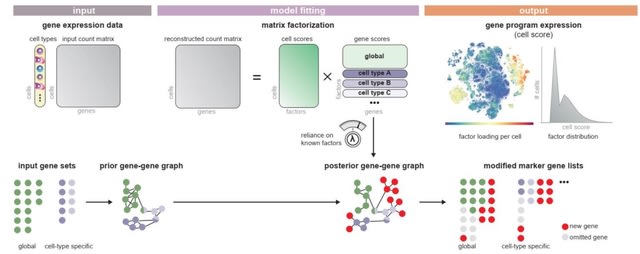
□ Spectra: Supervised discovery of interpretable gene programs from single-cell data
>> https://www.biorxiv.org/content/10.1101/2022.12.20.521311v1
Spectra overcomes the dominance of cell-type signals by modeling cell-type-specific programs, and can characterize interpretable cell states along a continuum.
Spectra retrieves gene programs from scRNA-seq data using biological priors. As input, Spectra receives a gene expression count matrix with cell type labels for each cell, as well as pre-defined gene sets, which it converts to a gene-gene graph.
The algorithm fits a factor analysis using a loss function that optimizes reconstruction of the count matrix and guides factors to support the input gene-gene graph. As output, Spectra provides factor loadings and gene programs corresponding to cell types and cellular processes.

□ DEAPLOG: A method for differential expression analysis and pseudo- temporal locating and ordering of genes in single-cell transcriptomic data
>> https://www.biorxiv.org/content/10.1101/2022.12.21.521359v1
DEAPLOG, a method for differential expression analysis and pseudo-temporal locating and ordering of genes in sc-transcriptomic data. DEAPLOG infers pseudo-time / embedding coordinates of genes, therefore is useful in identifying regulators in trajectory of cell fate decision.
DEAPLOG identifies a large number of statistically significant DEGs. DEAPLOG defines the point with the maximum curvature on the fitting curve of a gene expression as threshold. DEAPLOG combines polynomial fitting and hypergeometric distribution.

□ SCellBOW: Latent representation of single-cell transcriptomes enables algebraic operations on cellular phenotypes
>> https://www.biorxiv.org/content/10.1101/2022.12.28.522060v1
SCellBOW uses Doc2vec, which is a bag- of-words model, and therefore is independent of any strict ordering of genes. The SCellBOW algorithm provides a latent representation of single-cells in a manner that captures the 'semantics' associated with cellular phenotypes.
SCellBOW learned neuronal weights are transferable. These representations, aka embeddings, allow algebraic operations such as +/-. SCellBOW-based vector representation of cellular transcriptomes preserves their phenotypic relationships in a vector space.

□ SEISM: Neural Networks beyond explainability: Selective inference for sequence motifs
>> https://www.biorxiv.org/content/10.1101/2022.12.23.521748v1
SEISM, a selective inference procedure to test the association b/n the extracted features and the predicted phenotype. SEISM uses a one-layer convolutional network is formally equivalent to selecting motifs maximizing some association score.
SEISM partitions the space of motifs to quantize the selection. The selection event is the set of phenotype vectors. SEISM uses 50, 000 replicates under the conditional null hypothesis using the hypersphere direction sampler, after 10, 000 burn-in iterations.

□ mapquik: Efficient low-divergence mapping of long reads in minimizer space
>> https://www.biorxiv.org/content/10.1101/2022.12.23.521809v1
mapquik, which instead of using a single minimizer as a seed to a genome (e.g. minimap2), builds accurate longer seeds by anchoring alignments through matches of k consecutively-sampled minimizers (k-min-mers).
mapquik borrows from natural language processing, where the tokens of the k-mers are the minimizers instead of base-pair letters. mapquik application of minimizer-space computation is entirely distinct from genome assembly, as no de Bruijn graph is constructed.
Indexing the long minimizer-space seeds (k-min-mers) that occur uniquely in the genome is sufficient for mapping. mapquik devises a provably O(n) time pseudo-chaining algorithm, which improves upon the subsequent best O(nlogn) runtime of all other known colinear chaining.

□ ASTER: accurately estimating the number of cell types in single-cell chromatin accessibility data
>> https://academic.oup.com/bioinformatics/advance-article/doi/10.1093/bioinformatics/btac842/6961187
ASTER, an ensemble learning-based tool for accurately estimating the number of cell types in scCAS data. ASTER performs estimation based on the Davies-Bouldin index.
ASTER calculates the mean silhouette coefficient of all cells based on Louvain and Leiden clustering. It provides the maximum coefficient is thus adopted as the optimal number of clusters.

□ NanoSNP: A progressive and haplotype-aware SNP caller on low coverage Nanopore sequencing data
>> https://academic.oup.com/bioinformatics/advance-article/doi/10.1093/bioinformatics/btac824/6957086
NanoSNP, a novel deep learning-based SNP calling method to identify the SNP sites (excluding short indels) based on low-coverage Nanopore sequencing reads. NanoSNP utilizes the naive pileup feature to predict a subset of SNP sites with a Bi-LSTM network.
NanoSNP has the highest precision score and second highest recall and F1 score on each dataset compared to Clair, Clair3, Pepper-DeepVariant, and NanoCaller. And NanoSNP extracts the features from both the alignment before WhatsHap phasing and the phased alignment.

□ SpaGFT is a graph Fourier transform for tissue module identification from spatially resolved transcriptomics
>> https://www.biorxiv.org/content/10.1101/2022.12.10.519929v1
SpaGFT transforms complex gene expression patterns into simple, but informative signals, leading to the accurate identification of spatially variable genes (SVGs) at a fast computational speed.
SpaGFT generates a novel representation of GE and the corresponding spot graph topology in a Fourier space, which enables TM identification and enhances SVG prediction. The low-frequency SVG FM signals are selected as features to identify SVG clusters using Louvain clustering.

□ EnDecon: cell type deconvolution of spatially resolved transcriptomics data via ensemble learning
>> https://academic.oup.com/bioinformatics/advance-article/doi/10.1093/bioinformatics/btac825/6957087
EnDecon obtains the ensemble result by alternatively updating the ensemble result as a weighted median of the base deconvolution results and the weights of base results based on their distance from the ensemble result.
EnDecon correctly locates cell type to the specific spatial regions, which are consistent with the gene expression patterns of the corresponding cell type marker genes. Furthermore, cell types enriched regions are in line with those of located regions.

□ STREAM: Enhancer-driven gene regulatory networks inference from single-cell RNA-seq and ATAC-seq data
>> https://www.biorxiv.org/content/10.1101/2022.12.15.520582v1
STREAM (Single-cell enhancer regulaTory netwoRk inference from gene Expression And ChroMatin accessibility), a computational framework to infer eGRNs from jointly profiled scRNA-seq and scATAC-seq data.
STREAM combines the Steiner forest problem (SFP) model and submodular optimization, respectively, to discover the enhancer-gene relations and TF-enhancer-gene relations in a global optimization manner. STREAM formulates the eGRN inference by detecting a set of hybrid biclusters.
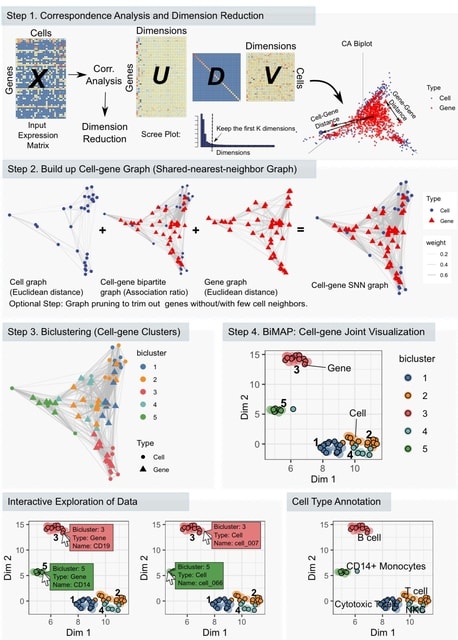
□ CAbiNet: Joint visualization of cells and genes based on a gene-cell graph
>> https://www.biorxiv.org/content/10.1101/2022.12.20.521232v1
“Correspondence Analysis based Biclustering on Networks” (CAbiNet) to produce a joint visualization and co-clustering of cells and genes in a planar embedding. CAbiNet employs CA to build a graph in which the nodes are comprised of both cells and genes.
Then a clustering algorithm determines the cell-gene clusters from the graph. Finally, the cells, genes and the clustering results are visualized in a 2D-embedding (biMAP). Cells and genes from the same cluster are colored identically in the biMAP.

□ scPROTEIN: A Versatile Deep Graph Contrastive Learning Framework for Single-cell Proteomics Embedding
>> https://www.biorxiv.org/content/10.1101/2022.12.14.520366v1
scPROTEIN, a novel versatile framework composed of peptide uncertainty estimation based on a multi-task heteroscedastic regression model and cell embedding learning based on graph contrastive learning designed for single-cell proteomic data analysis.
sPROTEIN can construct cell graph based on spatial proximity. scPROTEIN contains four major components: Data augmentation, GCN-based graph encoder, Node-level graph contrastive learning and Alternated topology-attribute denoising module.

□ Quantum-Si
>> https://ir.quantum-si.com/news-releases/news-release-details/quantum-si-announces-commercial-availability-platinumtm-worlds/
Introducing the world’s 1st next-generation single-molecule protein sequencing platform — #Platinum™. Learn more about this simple-to-use system and its low price point, unique design, and advanced capabilities here: ir.quantum-si.com/news-releases/… $QSI #ProteinSequencing #Biotech #NGS
"by monitoring for amino-acid specific patterns in fluorescent probe behavior. This means that a single probe can be used for the robust identification of multiple distinct amino acids, including those containing post translational modifications."

□ Dissecting Complexity: The Hidden Impact of Application Parameters on Bioinformatics Research
>> https://www.biorxiv.org/content/10.1101/2022.12.20.521257v1
SOMATA, a methodology to facilitate systematic exploration of the vast choice of configuration options, and apply it to three different tools on a range of scientific inquires.
SOMATA involves Selecting tools and data, identifying Objective metrics, Modeling the parameter space, choosing a sample design Approach, Testing, and Analyzing. A single parameter — MaxO — was varied since that is intuitively related to growth, the output objective of interest.

□ DRfold: Integrating end-to-end learning with deep geometrical potentials for ab initio RNA structure prediction
>> https://www.biorxiv.org/content/10.1101/2022.12.30.522296v1
DRfold predicts RNA tertiary structures by simultaneous learning of local frame rotations and geometric restraints from experimentally solved RNA structures, where the learned knowledge is converted into a hybrid energy potential to guide subsequent RNA structure constructions.
The core of the DRfold pipeline is the introduction of two types of complementary potentials, i.e., FAPE potential and geometry potentials, from two separate transformer networks.
The former models directly predict the rotation matrix and the translation vector for the frames representing each nucleotide, forming an end-to-end learning strategy for RNA structure.

□ A Boolean Algebra for Genetic Variants
>> https://academic.oup.com/bioinformatics/advance-article/doi/10.1093/bioinformatics/btad001/6967432
A comprehensive set of Boolean relations: equivalence, containment, overlap and disjoint, that partitions the domain of binary variant relations. Using these relations, additional variants of interest, i.e., variants with a specific relation to the queried variant can be identified.
The relations can be computed efficiently using a novel algorithm that computes all minimal alignments. Filtering on the maximal influence interval allows for calculating the relations for all pairs of variants for an entire gene.

□ RGT: a toolbox for the integrative analysis of high throughput regulatory genomics data
>> https://www.biorxiv.org/content/10.1101/2022.12.31.522372v1
RGT provides three core classes to handle the genomic regions and signals. Each genomic region is represented by GenomicRegion class and multiple regions are represented by GenomicRegionSet class. The genomic signals are represented CoverageSet class.
The several tools are developed, namely, HINT for analysis of ATAC/DNase-seq; RGT-viz for finding associations b/n chromatin experiments; TDF for DNA/RNA triplex domain finder; THOR for differential peak calling; Motif analysis for transcription factor binding sites matching.

□ MuLan-Methyl: Multiple Transformer-based Language Models for Accurate DNA Methylation Prediction
>> https://www.biorxiv.org/content/10.1101/2023.01.04.522704v1
The output of MuLan-Methyl is based on the average of the prediction probabilities obtained by transformer-based language models, namely BERT, DistilBERT, ALBERT, XLNet and ELECTRA. Each of the five language models is trained according to the “pre-train / fine-tune” paradigm.

□ ACIDES: In-silico monitoring of directed evolution convergence to unveil best performing variants with credibility score
>> https://www.biorxiv.org/content/10.1101/2023.01.03.522172v1
ACIDES (Accurate Confidence Intervals to rank Directed Evolution Scores), a combination of statistical inference and in-silico simulations to reliably estimate the selectivity of individual variants and its statistical error using the data from all available rounds.
ACIDES realizes a 50- to 70-fold improvement over the Poisson model in the predictive ability of the NGS sampling noise. ACIDES uses simulations to quantify a Rank Robustness (RR), a measure of the quality of the selection convergence.

□ ElasticBLAST: Accelerating Sequence Search via Cloud Computing
>> https://www.biorxiv.org/content/10.1101/2023.01.04.522777v1
One of the ElasticBLAST parameters that is critical to its performance is the batch length, which specifies the number of bases or residues per query batch. ElasticBLAST automatically selects an appropriate instance type for a search, based on database metadata and the BLAST program.