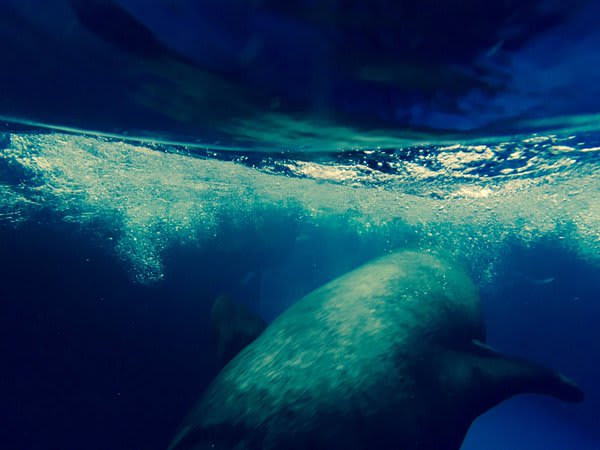
(iPhone 6s: camera)
□ 生物種という境界概念が無いのだとしたら、私たち他者や葦との異なりは、等しく空間に散逸する砂粒の模様の違いでしかない。そこに柵や水槽のようなレトリックもなく、文字通りの意味で、砂粒と私たちとを別つ理である。
□ 特有と共有は相容れないものではない。確かに自身の経験する思考や感情が、他者にとって既知であることを担保するものはない。然しながら記憶や発話が、対面の他者との表現上のレファレンスを書き換えているだけではないことは自明でありそうだ。私たちは差異を通じて同化しようとしているのである。
□ Private algebras in quantum information and infinite-dimensional complementarity:
>> http://scitation.aip.org/docserver/fulltext/aip/journal/jmp/57/1/1.4935399.pdf
This new framework is particularly amenable to the important class of linear bosonic channels, and preliminary investigations suggest a deeper connection between comple- mentarity and symplectic duality.

□ MaGuS: quality assessment and scaffolding of genome assemblies with Whole Genome Profiling™
>> http://bmcbioinformatics.biomedcentral.com/articles/10.1186/s12859-016-0969-x
Based on the QUAST NA50 and NA75, ranked the assemblies from the highest to lowest quality as BESST, OPERA-LG, SSPACE, SOAPdenovo2, and SGA. Existing scaffolder tools encounter issues when dealing with repeat-rich regions. The use of a map overcomes this problem if a contig or scaffold can be anchored onto the map. For large genomes, the sequencing depth of an MP library may result in low covered regions. Users of scaffolding programs often set a minimum cut-off for read pairs required to validate a link btwn contigs, to avoid assembly errors. The use of MaGuS is not restricted to WGP maps, other genome map types can be integrated after formatting.

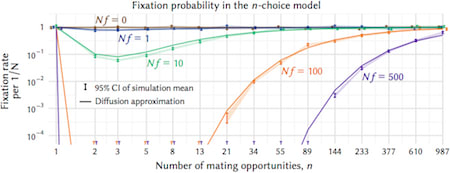
□ Assortative mating can impede or facilitate fixation of underdominant alleles
>> http://biorxiv.org/content/biorxiv/early/2016/03/03/042192.1.full.pdf
The n-choice model is a simple, mechanistic implementation of positive assortative mating that accounts for the fact that in reality rare genotypes are less likely to find preferred mates. The n-choice model fulfills the realistic condition that individuals can survey only a limited number of prospective mates.
□ Demographic inference under the coalescent in a spatial continuum
>> http://www.biorxiv.org/content/biorxiv/early/2016/03/02/042135.full.pdf
the spatial Λ-Fleming-Viot (ΛV) model is amenable to parameter inference under biologically realistic conditions. REX corresponds to either a single reproduction event accompanied by extinction of the parent w the offspring dispersing over long distances or a sum of multiple reproduction and extinction events, each reproduction accompanied by dispersal of the offspring over short distances. the average time between two successive REX in a given lineage is proportional to the generation time of the species under scrutiny.
□ HPG pore: an efficient and scalable framework for nanopore sequencing data
>> http://bmcbioinformatics.biomedcentral.com/articles/10.1186/s12859-016-0966-0
HPG Pore, the first scalable bioinformatic tool for exploring and analyzing nanopore sequencing data using the Hadoop framework. HPG Pore allows for virtually unlimited sequencing data scalability, efficient management of huge amounts of data. When HPG Pore runs in Hadoop mode it is faster than Poretools and poRe, despite an initial delay due to the preparation of the Hadoop nodes. as expected, the speed is even faster when more nodes are available, thus it outperforms the other two programs when running in local mode. The latency of the Hadoop framework causes the paradox that the stand alone version slightly slower than the counterpart running on one node.
□ The Ensembl Variant Effect Predictor: analysis, annotation & prioritization of genomic variants in non-coding region
>> http://biorxiv.org/content/biorxiv/early/2016/03/04/042374.full.pdf
The VEP incl PubMed identifiers for variants which have been cited, and also annotates those associated with a phenotype, disease or trait, using data from OMIM, Orphanet, the GWAS Catalog and other data sources.
□ Comparative evaluation of isoform-level gene expression estimation algorithms for RNA-seq and exon-array platforms
>> http://bib.oxfordjournals.org/content/early/2016/02/26/bib.bbw016.long
Multi-Mapping Bayesian Gene eXpression (MMBGX) programs achieved the best performance for RNA-seq and exon-array platforms. While eXpress achieved the highest correlation with the RT-qPCR and exon-array (MMBGX) results overall, RSEM was more highly correlated with MMBGX for changes in highly variable transcripts.
□ XGBoost: A Scalable Tree Boosting System: a sparsity-aware algorithm and weighted quantile sketch for tree learning.
>> http://arxiv.org/abs/1603.02754v1
XGBoost handles all sparsity patterns in a unified way. this method exploits the sparsity to make computation complexity linear to number of non-missing entries in the input. the cache-aware implementation of the exact greedy algorithm runs twice as fast as the naive version when the dataset is large. Scaling of XGBoost with different number of machines on criteo full 1.7 billion data with only four machines. Using more machines results in more file cache and makes the system run faster, causing the trend to be slightly super linear.
□ Topological phase transition of a fractal spin system: The relevance of the network complexity:
>> http://scitation.aip.org/content/aip/journal/adva/6/5/10.1063/1.4942826
the random links remove infrared divergences by breaking the inversion symmetry of a complex network. the dimensionality restrictions imposed by Mermin-Wagner theorem which assumes translational invariance, are completely removed. Particular attention is given to complex networks of small-world type. The phase a system adopts, in thermal equilibrium, is characterized by spontaneous symmetry breaking and by the ratio of the order parameter and the characteristic length of the system. time reversal inversion and discrete symmetries, neglecting higher order terms, the free energy Φ0(x, T) as Φ0(x, T) = A(T)|∇ψ|2 + B(T)|ψ|2,
□ A modified PATH algorithm rapidly generates transition states comparable to those found by other algorithms:
>> http://scitation.aip.org/docserver/fulltext/aca/journal/sdy/3/1/1.4941599.pdf
PATH defines the structures of equilibrium states using a linearized ANM potential. This approximation of the complex potential energy landscape works because most protein conformational changes are small displacements.
□ NanoSim: nanopore sequence read simulator based on statistical characterization:
>> http://biorxiv.org/content/biorxiv/early/2016/03/18/044545.full.pdf
The perfect flag of NanoSim can generate perfect reads with no errors, relying on the full-length distribution of aligned reads.
□ DeepNano: Deep Recurrent Neural Networks for Base Calling in MinION Nanopore Reads:
>> http://arxiv.org/pdf/1603.09195v1.pdf
DeepNano is a more accurate and efficent alternative to the HMM-based methods used in the Metrichor base caller by the device manufacturer. DeepNano does not require read overlaps, it processes reads individually and provides more precise base calls for downstream analysis. SGD is better at avoiding bad local optima in the initial phases of training, while L-BFGS seems to be faster during the final fine-tuning.
□ mattloose:
Very excited - rnn base caller running on R9....
□ Clive_G_Brown:
@mattloose for example, at 150-180mv. 200mv adds a 1-2%. Looking at 250 and 300mv. Note 2D tidied up since talk.
□ deepTarget: End-to-end Learning Framework for microRNA Target Prediction using Deep Recurrent Neural Networks
>> http://arxiv.org/pdf/1603.09123v1.pdf
The performance gap between the proposed method and existing alternatives is substantial (over 25% increase in F-measure), and deepTarget delivers a quantum leap in the long-standing challenge of robust miRNA target prediction.
□ ACE: adaptive cluster expansion for maximum entropy graphical model inference:
>> http://biorxiv.org/content/biorxiv/early/2016/03/18/044677.full.pdf
ACE avoids overfitting by constructing a sparse network of interactions sufficient to reproduce the observed correlation data within the statistical error expected due to finite sampling. an extension of the adaptive cluster expansion (ACE) method, originally devised for binary (Ising) variables, to more general (Potts) variables taking multiple categorical values. When convergence of the ACE algorithm is slow, we combine it with a Boltzmann Machine Learning algorithm (BML). Refinement with Boltzmann Machine Learning, adapted the RPROP algorithm for neural network learning to the case of Potts models.
□ EMPIAR: Supporting the bioimaging revolution: Commentary in @naturemethods
>> http://www.ebi.ac.uk/about/news/press-releases/supporting-bioimaging-revolution
EMPIAR is part of a larger project at PDBe called “MOL2CELL”, that aims to integrate 3D structural information on different length scales.
□ Robust normalization protocols for multiplexed fluorescence bioimage analysis:
>> http://biodatamining.biomedcentral.com/articles/10.1186/s13040-016-0088-2
the proposed method produces higher between class Kullback-Leibler (KL) divergence and lower within class on a distribution of phenotypes. the combination of No clipping + bilateral filtering + linear scaling which produces the best results.


□ Modeling multi-particle complexes in equilibrium/non-equilibrium stochastic chemical system
>> http://biorxiv.org/content/biorxiv/early/2016/03/23/045435.full.pdf
The concept of occlusion is essential for any rule-based description is realized by using a Fock space constructed from hard core bosons. Using this composite Fock space dramatically simplifies the Hamiltonian of the 0-Dimentional polymer system. one readily verifies that gallery G and Hamiltonian properly define a zero-dimensional monomeric particles in the grand canonical ensemble.
□ An integral formula adapted to different boundary conditions for arbitrarily high-dimensional nonlinear KG equations
>> http://scitation.aip.org/docserver/fulltext/aip/journal/jmp/57/2/1.4940050.pdf
The operators have a complete system of orthogonal eigenfunctions in the complex Hilbert space L2(Ω). Because of the isomorphism between L2 and l2, the operator ∆ on L2(Ω) induces a corresponding operator on l2. the Laplacian-valued functions de ned on D(∆) depending on different boundary conditions are bounded operators with respect to the norm.
□ An algebraic approach to parameter optimization in biomolecular bistable systems:
>> http://biorxiv.org/content/biorxiv/early/2016/03/24/045518.full.pdf
Algebraic equilibrium conditions are also bistability conditions in a class of bistable networks. Theorem 3: Consider a linear positive system x ̇ = Ax, whose characteristic polynomial is an F-polynomial. The index of a regular equilibrium point x ̄ is the sign of the determinant of -J(x ̄):
ind(x ̄) = sign[det(-J(x ̄))]
□ Div-Seq: A single nucleus RNA-Seq method reveals dynamics of rare adult newborn neurons in the CNS:
>> http://biorxiv.org/content/biorxiv/early/2016/03/27/045989.full.pdf
Div-Seq, which combines Nuc-Seq, a scalable single nucleus RNA-Seq method, with EdU-mediated labeling of proliferating cells. Div-Seq provides a unique opportunity to profile the transcriptional program underlying neuronal maturation. Div-Seq’s ability to clearly identify and characterize rare cells in the spinal cord shows its significantly improved sensitivity.
□ RQBoost: Developing Quantum Annealer Driven Data Discovery to Quantum Machine Learning:
>> http://arxiv.org/pdf/1603.07980v1.pdf
explore the performance of RQBoost in the space of NLP and seizure prediction and find QA-enabled ML using QBoost and RQBoost. RQBoost are sensitive to initial feature space partitions when the number of features is larger than the number of possible QUBO variables. RQBoost could be used as an ensembler where the base learners are traditional machine learning algorithms.
□ Markov substitute processes : a new model for linguistics and beyond:
>> http://arxiv.org/abs/1603.07850v1
define one-dimensional Markov substitute processes and shown that they are an extension of one-dimensional Markov random fields. generalize the notion of a multi-dimensional Markov random field by proposing a definition for multi-dimensional Markov substitute processes. the state space of the model is D+= ∞j=1 Dj the set of all sentences of finite non-zero length. the empty string ε of zero length, D∗={ε}∪D+
□ Hybridization of Expectation-Maximization and K-Means Algorithms for Better Clustering Performance:
>> http://arxiv.org/pdf/1603.07879v1.pdf
K-Means is formally equivalent to EM as K-Means is a limiting case of fitting data by a mixture of k Gaussians with identical, isotropic covariance matrices (Σ = σ2I), when the soft assignments of data points to mixture components are hardened to allocate each data point solely to the most likely component. A random space is isotropic if its covariance function depends on distance alone.
M-Step: Compute means μj and covariance matrices Σj for j = 1, ..., k, based on the results of K-Means step.
Wj = nj/N for j = 1, ..., k.
E-Step: For each given data vector Xi (i = 1, 2, ..., N), the cluster probability P(Cj|Xi) for j = 1, ..., k
Max{P(Cj | Xi ); j = 1,...,k}.
K-Means Step: Assign each data vector Xi to the closest cluster with mean, μj using Euclidean distance
□ PhredEM: A Phred-Score-Informed Genotype-Calling Approach for Next-Generation Sequencing Studies:
>> http://biorxiv.org/content/biorxiv/early/2016/03/29/046136.full.pdf
PhredEM uses the Expectation-Maximization algorithm to obtain consistent estimates of genotype frequencies & logistic regression parameters. a simple and computationally efficient screening algorithm to identify monomorphic loci. Nominally, the phred score is defined as
Q = -10 log10 Pr(observed allele ≠ true allele),
□ Nanocall: An Open Source Basecaller for Oxford Nanopore Sequencing Data:
>> http://biorxiv.org/content/early/2016/03/28/046086
Nanocall uses some simple heuristics for splitting the sequence of events (current levels) into strands, it models the events using a hidden Markov model where the states are the kmers being sequenced, optionally scales the pore model emissions using several rounds of Expectation Maximization based on posteriors computed w/ Forward-Backward, it produces basecalls by running Viterbi.
□ Classification-based Financial Markets Prediction using Deep Neural Networks:
>> http://arxiv.org/pdf/1603.08604v1.pdf
Gaussian random numbers are generated from transforming the uniform random numbers with an inverse Gaussian cumulative distribution function with zero mean and standard deviation of 0.01. the subset of the training set used for each epoch is defined as
De := {xnk ∈ Dtrain | nk ∈ U(1,Ntrain),k := 1,...,Nepoch}
If cross entropy(e) ≤ cross entropy(e-1) then γ ← γ/2
□ Feather: fast, interoperable binary data frame storage:
>> https://github.com/wesm/feather
Language agnostic: Feather files are the same whether written by Python or R code. Other languages can read and write Feather files
arr = np.random.randn(10000000) # 10% nulls
arr[::10] = np.nan
df = pd.DataFrame({'column_{0}'.format(i): arr for i in range(10)})
In [9]: %time df = feather.read_dataframe('test.feather')
CPU times: user 316 ms, sys: 944 ms, total: 1.26 s
Wall time: 1.26 s

□ DiagrammeR: Generate graph diagrams using text in a Markdown-like syntax.
>> http://rich-iannone.github.io/DiagrammeR/
DiagrammeR directly edit mermaid (.mmd) or Graphviz (.gv) files inside RStudio with syntax coloring. Preview easily. Scalable Vector Graphics, Output looks great as SVG. Diagram elements such as nodes and edges won't lose visual clarity.
□ pomegranate: a package for graphical models and Bayesian statistics for Python, implemented in Python
>> http://pomegranate.readthedocs.org/en/latest/index.html
pomegranate is flexible enough to allow nesting of components to form models such as general mixture model hidden Markov models (GMM-HMMs) or Naive Bayes comparing a hidden Markov model to a Markov chain. Yet Another Hidden Markov Model library module implements graph-based interface. forward-backward, Baum-Welch and Viterbi algorithms.
□ BayesDB: Data science is a communication problem: Strata + Hadoop World conf in San Jose, March 28-31, 2016.
>> https://www.oreilly.com/ideas/bayesdb-data-science-is-a-communication-problem
no graphical environment can overcome the fundamental information asymmetry and complexity inherent in modeling data. The Bayesian Query Language (BQL) provides three key verbs that encompass the full range of inference questions: SIMULATE, INFER, ESTIMATE. Bayesian Query Language enables users to generate answers to a broad class of "what-if?" scenarios, contingencies and hypotheticals. The Meta-modeling Language (MML) enables machine assisted modeling for populations based on samples and domain insight.