
□ Wasserstein Wormhole: Scalable Optimal Transport Distance with Transformers
>>
https://arxiv.org/abs/2404.09411
Wasserstein Wormhole, an algorithm that represents each point cloud as a single embedded point, such that the Euclidean distance in the embedding space matches the OT distance between point clouds. The problem solved by Wormhole is analogous to multidimensional scaling.
In Wormhole space, they compute Euclidean distance in O(d) time for an embedding space with dimension d, which acts as an approximate OT distance and enables Wasserstein-based analysis without expensive Sinkhorn iterations.
Wormhole minimizes the discrepancy between the embedding pairwise distances and the pairwise Wasserstein distances of the batch point clouds. The Wormhole decoder is a second transformer trained to reproduce the input point clouds from the embedding by minimizing the OT distance.

□ Symphony: Symmetry-Equivariant Point-Centered Spherical Harmonics for Molecule Generation
>>
https://arxiv.org/abs/2311.16199
Symphony, an autoregressive generative model that uses higher-degree equivariant features and spherical harmonic projections to build molecules while respecting the E(3) symmetries of molecular fragments.
Symphony builds molecules sequentially by predicting and sampling atom types and locations of new atoms based on conditional probability distributions informed by previously placed atoms.
Symphony stands out by using spherical harmonic projections to parameterize the distribution of new atom locations. This approach enables predictions to be made using features from a single 'focus' atom, which serves as the chosen origin for that step of the generation process.

□ Distributional Graphormer: Predicting equilibrium distributions for molecular systems with deep learning
>>
https://www.nature.com/articles/s42256-024-00837-3
Distributional Graphormer (DiG) can generalize across molecular systems and propose diverse structures that resemble observations. DiG draws inspiration from simulated annealing, which transforms a uniform distribution to a complex one through a simulated annealing process.
DiG enables independent sampling of the equilibrium distribution. The diffusion process can also be biased towards a desired property for inverse design and allows interpolation between structures that passes through high-probability regions.
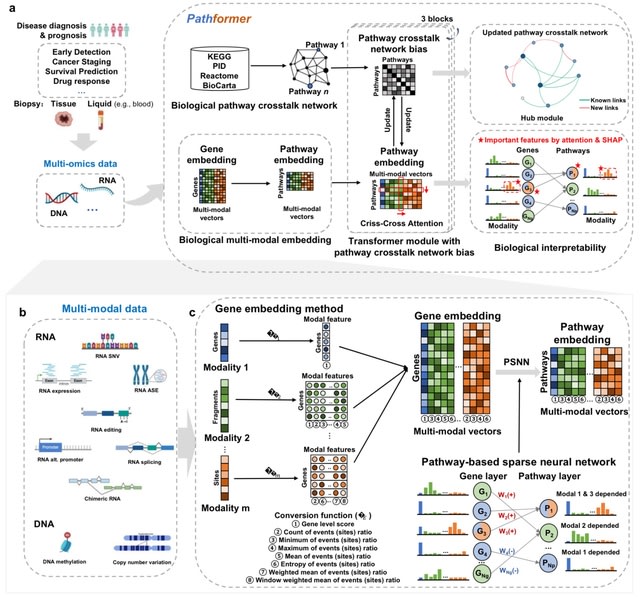
□ Pathformer: a biological pathway informed transformer for disease diagnosis and prognosis using multi-omics data
>>
https://academic.oup.com/bioinformatics/advance-article/doi/10.1093/bioinformatics/btae316/7671099
Pathformer transforms various modalities into distinct gene-level features using a series of statistical methods, such as the maximum value method, and connects these features into a novel compacted multi-modal vector for each gene.
Pathformer employs a sparse neural network based on the gene-to-pathway mapping to transform gene embedding into pathway embedding. Pathformer enhances the fusion of information b/n various modalities and pathways by combining pathway crosstalk networks with Transformer encoder.

□ RNAErnie: Multi-purpose RNA language modelling with motif-aware pretraining and type-guided fine-tuning
>>
https://www.nature.com/articles/s42256-024-00836-4
RNAErnie is built upon the Enhanced Representation through Knowledge Integration (ERNIE) framework and incorporates multilayer and multihead transformer blocks, each having a hidden state dimension of 768.
RNAErnie model consists of 12 transformer layers. In the motif-aware pretraining phase, RNAErnie is trained on a dataset of approximately 23 million sequences extracted from the RNAcentral database using self-supervised learning with motif-aware multilevel random masking.
RNAErnie first predicts the possible coarse-grained RNA types using output embeddings and then leverages the predicted types as auxiliary information for fine-tuning. RNAErnie leverages an RNAErnie basic block to predict the top-K most possible coarse-grained RNA types.

□ LucaOne: Generalized Biological Foundation Model with Unified Nucleic Acid and Protein Language
>>
https://www.biorxiv.org/content/10.1101/2024.05.10.592927v1
LucaOne possesses the capability to interpret biological signals and, as a foundation model, can be guided through input data prompts to perform a wide array of specialized tasks in biological computation.
LucaOne leverages a multifaceted computational training strategy that concurrently processes nucleic acids (DNA / RNA) and protein data from 169,861 species. LucaOne comprised 20 transformer-encoder blocks with an embedding dimension of 2560 and a total of 1.8 billion parameters.

□ BIMSA: Accelerating Long Sequence Alignment Using Processing-In-Memory
>>
https://www.biorxiv.org/content/10.1101/2024.05.10.593513v1
BIMSA (Bidirectional In-Memory Sequence Alignment), a PIM-optimized implementation of the state-of-the-art sequence alignment algorithm BiWFA (Bidirectional Wavefront Alignment), incorporating hardware-aware optimizations for a production-ready PIM architecture (UPMEM).
BIMSA follows a coarse-grain parallelization scheme, assigning one or more sequence pairs to each DPU thread. This parallelization scheme is the best fit when targeting the UPMEM platform, as it removes the need for thread synchronization or data sharing across compute units.

□ MrVI: Deep generative modeling of sample-level heterogeneity in single-cell genomics
>>
https://www.biorxiv.org/content/10.1101/2022.10.04.510898v2
MrVI (Multi-resolution Variational Inference) identifies sample groups without requiring a priori clustering of the cells. It allows for different sample groupings to be conferred by different subsets of cells that are detected automatically.
MrVI enables both DE and DA in an annotation-free manner and at high resolution while accounting for uncertainty and controlling for undesired covariates, such as the experimental batch.
MrVI provides a principled methodology for estimating the effects of sample-level covariates on gene expression at the level of an individual cell. MrVI leverages the optimization procedures incl. in sevi-tools, allowing it to scale to multi-sample studies with millions of cells.

□ DeChat: Repeat and haplotype aware error correction in nanopore sequencing reads
>>
https://www.biorxiv.org/content/10.1101/2024.05.09.593079v1
DeChat corrects sequencing errors in ONT R10 long reads in a manner that is aware of repeats, haplotypes or strains. DeChat combines the concepts of de Bruijn graphs (dBG) and variant-aware multiple sequence alignment via partial order alignment algorithm.
DeChat divides raw reads into small kmers and eliminates those with extremely low frequencies. Subsequently, it constructs a compacted de Bruijn graph (dBG). Each raw read is then aligned to the compacted dBG to identify the optimal alignment path.

□ CELLama: Foundation Model for Single Cell and Spatial Transcriptomics by Cell Embedding Leveraging Language Model Abilities
>>
https://www.biorxiv.org/content/10.1101/2024.05.08.593094v1
CELLama (Cell Embedding Leverage Language Model Abilities), a framework that leverage language model to transform cell data into 'sentences' that encapsulate gene expressions and metadata, enabling universal cellular data embedding for various analysis.
CELLama transforms scRNA-seq data into natural language sentences. CELLama can utilize pretrained models that cover general NLP processes for embedding, and it can also be fine-tuned using large-scale cellular data by generating sentences and their similarity metrics.

□ scBSP: A fast and accurate tool for identifying spatially variable genes from spatial transcriptomic data
>>
https://www.biorxiv.org/content/10.1101/2024.05.06.592851v1
scBSP (single-cell big-small patch), a significantly enhanced version of BSP, to address computational challenges in the identification of SVGs from large-scale two/three-dimensional SRT data.
scBSP selects a set of neighboring spots within a certain distance to capture the regional means and filters the SVGs using the velocity of changes in the variances of local means with different granularities.

□ EpiTrace: Tracking single-cell evolution using clock-like chromatin accessibility loci
>>
https://www.nature.com/articles/s41587-024-02241-z
EpiTrace counts the fraction of opened clock-like loci from scATAC-seq data to perform lineage tracing. The measurement was performed using a hidden Markov model -mediated diffusion-smoothing approach, borrowing information from similar single cells to reduce noise.
The EpiTrace algorithm simply leverages the fact that heterogeneity of given reference ClockDML reduces during cell replication and then uses such information as an intermediate tool variable to infer cell age.

□ SYNY: a pipeline to investigate and visualize collinearity between genomes
>>
https://www.biorxiv.org/content/10.1101/2024.05.09.593317v1
Collinear segments, also known as syntenic blocks, can be inferred from sequence alignments and/or from the identification of genes arrayed in the same order and relative orientations between investigated genomes.
SYNY investigates gene collinearity (synteny) between genomes by reconstructing clusters from conserved pairs of protein-coding genes identified from DIAMOND homology searches. It also infers collinearity from pairwise genome alignments with minimap2.

□ seismic: Disentangling associations between complex traits and cell types
>>
https://www.biorxiv.org/content/10.1101/2024.05.04.592534v1
seismic, a framework that enables robust and efficient discovery of cell type-trait associations and provides the first method to simultaneously identify the specific genes and biological processes driving each association.
seismic eliminates the need to select arbitrary thresholds to characterize trait or cell-type association. seismic calculates the statistical significance of a cell type-trait association using a regression-based framework with the gene specificity scores and MAGMA z-scores.
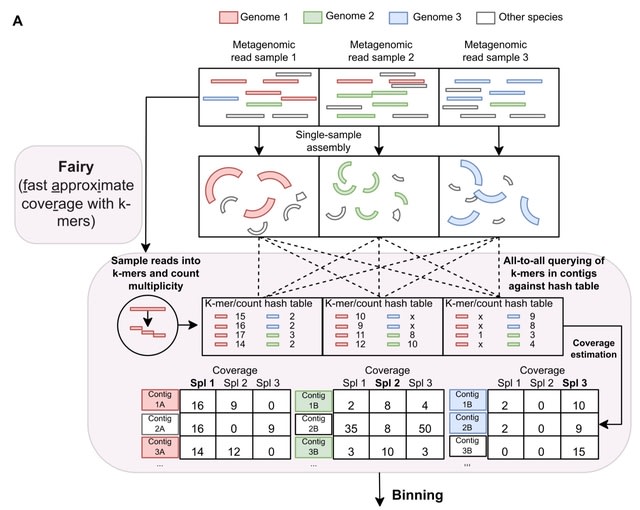
□ Fairy: fast approximate coverage for multi-sample metagenomic binning
>>
https://www.biorxiv.org/content/10.1101/2024.04.23.590803v1
fairy, a much faster, k-mer-based alignment-free method of computing multi-sample coverage for metagenomic binning. fairy is built on top of their metagenomic profiler sylph, but fairy is specifically adapted for metage-nomic binning of contigs.
Fairy indexes (or sketches) the reads into subsampled k-mer-to-count hash tables. K-mers from contigs are then queried against the hash tables to estimate coverage. Finally, fairy's output is used for binning and is compatible with several binners (e.g. MetaBAT2, MaxBin2).

□ Causal K-Means Clustering
>>
https://arxiv.org/abs/2405.03083
Causal k-Means Clustering harnesses the widely-used k-means clustering algorithm to uncover the unknown subgroup structure. Their problem differs significantly from the conventional clustering setup since the variables to be clustered are unknown counterfactual functions.
They present a plug-in estimator which is simple and readily implementable using off-the-shelf algorithms, and study its rate of convergence.
They also develop a new bias-corrected estimator based on nonparametric efficiency theory and double machine learning, and show that this estimator achieves fast root-n rates and asymptotic normality in large nonparametric models.

□ GoT–ChA: Mapping genotypes to chromatin accessibility profiles in single cells
>>
https://www.nature.com/articles/s41586-024-07388-y
GoT–ChA (genotyping of targeted loci with single-cell chromatin accessibility) links genotypes to chromatin accessibility at single-cell resolution across thousands of cells within a single assay.
Integration of mitochondrial genome profiling and cell-surface protein expression measurement allowed expansion of genotyping onto DOGMA-seq through imputation, enabling single-cell capture of genotypes, chromatin accessibility, RNA expression and cell-surface protein expression.

□ stDyer enables spatial domain clustering with dynamic graph embedding
>>
https://www.biorxiv.org/content/10.1101/2024.05.08.593252v1
stDyer employs a Gaussian Mixture Variational AutoEncoder (GMVAE) with graph attention networks (GAT) and graph embedding in the latent space. stDyer enables deep representation learning and clustering from Gaussian Mixture Models (GMMs) simultaneously.
stDyer also introduces dynamic graphs to involve more edges to a KNN spatial graph. Dynamic graphs can increase the likelihood that units at the domain boundaries establish connections with others belonging to the same spatial domain.
stDyer introduces mini-batch neighbor sampling to enable its application to large-scale datasets. stDyer is the first method that could enable multi-GPU training for spatial domain clustering.

□ xLSTM: Extended Long Short-Term Memory
>>
https://arxiv.org/abs/2405.04517
Enhancing LSTM to xLSTM by exponential gating with memory mixing and a new memory structure. xLSTM models perform favorably on language modeling when compared to state-of-the-art methods like Transformers and State Space Models.
XLSTM is based on a matrix memory. Lack of parallelizability due to memory mixing, i.e., the hidden-hidden connections between hidden states from one time step to the next, which enforce sequential processing.
An XLSTM architecture is constructed by residually stacking building blocks. An xLSTM block should non-linearly summarize the past in a high-dimensional space. Separating histories is the prerequisite to correctly predict the next sequence element such as the next token.

□ COEXIST: Coordinated single-cell integration of serial multiplexed tissue images
>>
https://www.biorxiv.org/content/10.1101/2024.05.05.592573v1
COEXIST, a novel algorithm that synergistically combines shared molecular profiles with spatial information to seamlessly integrate serial sections at the single-cell level.
COEXIST not only elevates MTI platform validation but also overcomes the constraints of MTI's panel size and the limitation of full nuclei on a single slide, capturing more intact nuclei in consecutive sections and enabling deeper profiling of cell lineages and functional states.

□ Streamlining remote nanopore data access with slow5curl
>>
https://academic.oup.com/gigascience/article/doi/10.1093/gigascience/giae016/7644676
Slow5curl uses an index to quickly fetch specific reads from a large dataset in SLOW5/BLOW5 format and highly parallelized data access requests to maximize download speeds.
The initiative is inspired by the SAM/BAM alignment data format and its many associated utilities, such as the remote client feature in samtools/htslib, which slow5curl emulates for nanopore signal data.

□ MerCat2: a versatile k-mer counter and diversity estimator for database-independent property analysis obtained from omics database
>>
https://academic.oup.com/bioinformaticsadvances/advance-article/doi/10.1093/bioadv/vbae061/7657691
MerCat2 (“Mer - Catenate2") computes k-mer frequency counting to any length k on assembled contigs as nucleotide fasta, raw reads or trimmed (e.g., fastq), and translated protein-coding open reading frames (ORFs) as a protein fasta.
MerCat2 has two analysis modes utilizing nucleotide or protein files. In nucleotide mode, outputs include %G+C and %A+T content, contig assembly statistics, and raw/trim read quality reports are a provided output. For protein mode, nucleotide files (can be translated into ORFs.

□ Comparative Genome Viewer: whole-genome eukaryotic alignments
>>
https://journals.plos.org/plosbiology/article?id=10.1371/journal.pbio.3002405
Comparative Genome Viewer (CGV), a new visualization tool for analysis of whole-genome assembly-assembly alignments. CGV visualizes pairwise same-species and cross-species alignments provided by NCBI.
The main view of CGV takes the “stacked linear browser” approach—chromosomes from 2 assemblies are laid out horizontally with colored bands connecting regions of sequence alignment.
These sequence-based alignments can be used to analyze gene synteny conservation but can also expose similarities in regions outside known genes, e.g., ultraconserved regions that may be involved in gene regulation.

□ DiSMVC: a multi-view graph collaborative learning framework for measuring disease similarity
>>
https://academic.oup.com/bioinformatics/advance-article/doi/10.1093/bioinformatics/btae306/7666859
DiSMVC is a supervised graph collaborative framework incl. two major modules. The former one is cross-view graph contrastive learning module, aiming to enrich disease representation by considering their underlying molecular mechanism from both genetic and transcriptional views.
while the latter module is association pattern joint learning, which can capture deep association patterns by incorporating phenotypically interpretable multimorbidities in a supervised manner.
DiSMVC can identify molecularly interpretable similar diseases, and the synergies gained from DiSMVC contributed to its superior performance in measuring disease similarity.

□ scDAPP: a comprehensive single-cell transcriptomics analysis pipeline optimized for cross-group comparison
>>
https://www.biorxiv.org/content/10.1101/2024.05.06.592708v1
scDAPP (single-cell Differential Analysis and Processing Pipeline) implements critical options for using replicates to generate pseudobulk data automatically, which are more appropriate for cross-group comparisons, for both gene expression and cell composition analysis.
scDAPP uses DoubletFinder to predict doublets for removal from further analysis. DoubletFinder hyperparameters such as the homotypic doublet rate are automatically estimated for each sample using the number of cells and the empirical multiplet rate provided by 10X Genomics.

□ Direct transposition of native DNA for sensitive multimodal single-molecule sequencing
>>
https://www.nature.com/articles/s41588-024-01748-0
SAMOSA by tagmentation (SAMOSA-Tag), which adds a concurrent channel for mapping chromatin structure. In SAMOSA-Tag, nuclei were methylated using the non-specific EcoGII m6dAase and tagmented in situ with hairpin-loaded transposomes.
DNA was purified, gap-repaired and sequenced, resulting in molecules where the ends resulted from Tn5 transposition, the m6dA marks represented fiber accessibility and computationally defined unmethylated ‘footprints’ captured protein–DNA interactions.

□ CAREx: context-aware read extension of paired-end sequencing data
>>
https://bmcbioinformatics.biomedcentral.com/articles/10.1186/s12859-024-05802-w
CAREx—a new read extension algorithm for Illumina PE data based on indel-free multiple-sequence-alignment (MSA). The key idea is to build MSAs of reads sequenced from the same genomic region.
CAREx gains efficiency by applying a variant of minhashing to quickly find a set of candidate reads which are similar to a query read with high probability and aligning with fast bit-parallel algorithms.
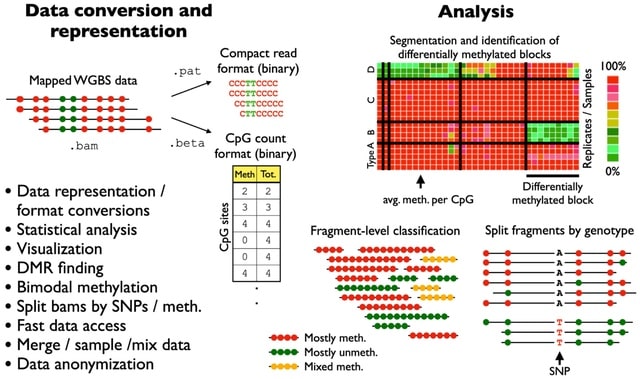
□ wgbstools: A computational suite for DNA methylation sequencing data representation, visualization, and analysis
>>
https://www.biorxiv.org/content/10.1101/2024.05.08.593132v1
wgbstools is an extensive computational suite tailored for bisulfite sequencing data. It allows fast access and ultra-compact data representation, as well as machine learning and statistical analysis, and visualizations, from fragment-level to locus-specific representations.
wgbstools converts data from standard formats (e.g., bam, bed) into tailored compact yet useful and intuitive formats (pat, beta). These can be visualized in terminal, or analyzed in different ways - subsample, merge, slice, mix, segment and more.

□ fastCCLasso: a fast and efficient algorithm for estimating correlation matrix from compositional data
>>
https://academic.oup.com/bioinformatics/advance-article/doi/10.1093/bioinformatics/btae314/7668443
FastCCLasso solves a penalized weighted least squares problem with the sparse assumption of the covariance matrix. Instead of the alternating direction method of multipliers, fastCCLasso introduces an auxiliary vector and provides a simple updating scheme in each iteration.
FastCCLasso only involves the calculation of multiplications between matrices and vectors and avoids the eigenvalue decomposition and multiplications of large dense matrices in CCLasso. The computational complexity of fastCCLasso is O(p2) per iteration.

□ SCIPAC: quantitative estimation of cell-phenotype associations
>>
https://genomebiology.biomedcentral.com/articles/10.1186/s13059-024-03263-1
SCIPAC enables quantitative estimation of the strength of association between each cell in a scRNA-seq data and a phenotype, with the help of bulk RNA-seq data with phenotype information. SCIPAC enables the estimation of association between cells and an ordinal phenotype.
SCIPAC identifies cells in single-cell data that are associated with a given phenotype. This phenotype can be binary, ordinal, continuous, or survival. The association strength and its p-value between a cell cluster and the phenotype are given to all cells in the cluster.

□ Bayesian modelling of time series data (BayModTS) - a FAIR workflow to process sparse and highly variable data
>>
https://academic.oup.com/bioinformatics/advance-article/doi/10.1093/bioinformatics/btae312/7671098
BayModTS, a FAIR workflow for processing time series data that incorporates process knowledge. BayModTS is designed for sparse data with low temporal resolution, a small number of replicates and high variability between replicates.
BayModTS is based on a simulation model, representing the underlying data generation process. This simulation model can be an Ordinary Differential Equation (ODE), a time-parameterised function, or any other dynamic modelling approach.
BayModTS infers the dynamics of time series data via Retarded Transient Functions. BayModTS uses Markov Chain Monte Carlo (MCMC) sampling. Parameter ensembles are simulated from the posterior distribution to transfer the uncertainty from the parameter to the data space.

□ Giraffe: a tool for comprehensive processing and visualization of multiple long-read sequencing data
>>
https://www.biorxiv.org/content/10.1101/2024.05.10.593289v1
Giraffe stands out by offering features that allow for the assessment of read quality, sequencing bias, and genomic regional methylation proportions of DNA reads and direct RNA sequencing reads.

□ RESHAPE: A resampling-based approach to share reference panels
>>
https://www.nature.com/articles/s43588-024-00630-7
RESHAPE (Recombine and Share Haplotypes), a method that enables the generation of a synthetic haplotype reference panel by simulating hypothetical descendants of reference panel samples after a user-defined number of meiosis.
This data transformation helps to protect against re-identification threats and preserves data attributes, such as linkage disequilibrium patterns and, to some degree, identity-by-descent sharing, allowing for genotype imputation.