□ NEBULA: a fast negative binomial mixed model for differential expression and co-expression analyses of large-scale multi subject single-cell data
>>
https://www.biorxiv.org/content/10.1101/2020.09.24.311662v1.full.pdf
NEBULA, NEgative Binomial mixed model Using a Large-sample Approximation analytically solves the high-dimensional integral in the marginal likelihood instead of using the Laplace approximation.
NEBULA forcuses on the NBMM rather than a zero-inflated model because multiple recent studies show that a zero-inflated model might be redundant for unique molecular identifiers (UMI)-based single-cell data.
NEBULA decomposes the total overdispersion into subject-level (i.e., between-subject) and cell- level (i.e., within-subject) components using a random-effects term parametrized by 𝜎2 and the overdispersion parameter 𝜙 in the negative binomial distribution.

□ The Divider BMA algorithm: Reconstruction Algorithms for DNA-Storage Systems
>>
https://www.biorxiv.org/content/10.1101/2020.09.16.300186v1.full.pdf
the problem is referred by the deletion DNA reconstruction problem and the goal is to minimize the Levenshtein distance dL(x,x).
A DNA reconstruction algorithm is a mapping R : (Σ∗q )t → Σ∗q which receives t traces y1, . . . , yt as an input and produces x, an estimation of x. The goal in the DNA reconstruction problem is to minimize the edit distance de(x,x) between the original string and the algorithm’s estimation.
The Divider BMA algorithm look globally on the entire sequence of the traces and use dynamic programming algorithms, which are used for the shortest common supersequence and the longest common subsequence problems, in order to decode the original sequence.

□ iGDA: Detecting and phasing minor single-nucleotide variants from long-read sequencing data
>>
https://www.biorxiv.org/content/10.1101/2020.09.25.314252v1.full.pdf
iGDA (in vivo Genome Diversity Analyzer), uses a novel algorithm, Adaptive Nearest Neighbor clustering (ANN), which makes no assumption about number of haplotypes.
iGDA leverages the information of multiple loci without restricting the number of dependent loci, and uses a novel algorithm, Random Subspace Maximization (RSM), to overcome the issue of combinatorial explosion.

□ Time-course Deep Learning architecture: Deep learning of gene interactions from single cell time-course expression data
>>
https://www.biorxiv.org/content/10.1101/2020.09.21.306332v1.full.pdf
Determining the optimal dimension for the input NEPDF which should be a function of the number of time point. the current architecture is dependent on the number of time points and the same model cannot be applied to a different dataset, if the number of time points do not match.
Time-course Deep Learning architecture using a supervised computational framework to predict causality, infer interactions and assign function to genes. the models seem to focus on both phase delay between genes along the time axes, and dynamics among NEPDF in each time point.
□ wfmash: base-accurate DNA sequence alignments using Wavefront Alignment algorithm and mashmap2
>>
https://github.com/ekg/wfmash
wfmash, A DNA sequence read mapper based on mash distances and the wavefront alignment algorithm. It completes an alignment module in MashMap and extends it to enable multithreaded operation.
The PAF output format is harmonized and made equivalent to that in minimap2, and has been validated as input to seqwish. wfmash has been developed to accelerate the alignment step in variation graph induction in the seqwish / smoothxg.

□ GRGNN: Inductive Inference of Gene Regulatory Network Using Supervised and Semi-supervised Graph Neural Networks
>>
https://www.biorxiv.org/content/10.1101/2020.09.27.315382v1.full.pdf
Inspired by SIRENE, extending SEAL by formulating the GRN inference problem as a graph classification problem and propose an end-to-end framework gene regulatory graph neural network (GRGNN) to infer GRN.
GRGNN is a versatile framework that fits for many alternatives in each step. In its implementation, Pearson’s correlation coefficient and mutual information are used to calculate links as a noisy skeleton to guide the prediction on the feature vectors of gene expression.

□ DNABERT: pre-trained Bidirectional Encoder Representations from Transformers model for DNA-language in genome
>>
https://www.biorxiv.org/content/10.1101/2020.09.17.301879v1.full.pdf
DNABERT adapts the idea of Bidirectional Encoder Representations from Transformers (BERT) model to DNA setting and developed a first-of-its-kind deep learning method in genomics.
DNABERT resolves the challenges by developing general and transferable understandings of DNA from the purely unlabeled human genome, and utilizing them to generically solve various sequence-related tasks in a “one-model-does-it-all” fashion.
DNABERT correctly captures the hidden syntax, and enables direct visualization of nucleotide-level importance and semantic relationship within input sequences for better interpretability and accurate identification of conserved sequence motifs and functional genetic variants.
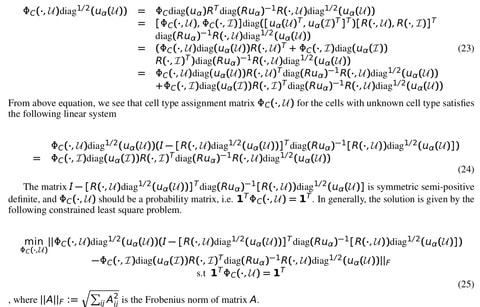
□ Dincta: Data INtegration and Cell Type Annotation of Single Cell Transcriptomes
>>
https://www.biorxiv.org/content/10.1101/2020.09.28.316901v1.full.pdf
Dincta can integrate the the data into a common low dimension embedding space such that cells with different cell types separate while cells from the different batches but in the same cell type cluster together.
The outer loop updates the unknown cell type indicator until it converges by checking the fitness b/w the cell / cluster assignments; the inner loop iterates b/w 2 complementary stages: maximum diversity clustering and inferring, and a mixture model based linear batch correction.
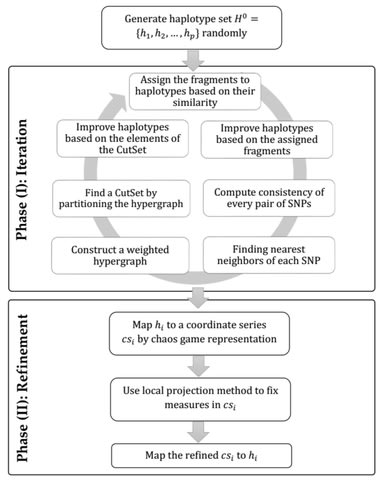
□ A chaotic viewpoint-based approach to solve haplotype assembly using hypergraph model
>>
https://www.biorxiv.org/content/10.1101/2020.09.29.318907v1.full.pdf
an iterative algorithm is proposed to reconstruct the haplotypes using the hypergraph model. Firstly, an iterative mechanism is applied due to the SNP matrix to construct the haplotype set, and the consistency between SNPs is modeled based on the hypergraph.
Each element of the finalized haplotype set is mapped to a line by chaos game representation, and a coordinate series is defined based on the position of mapped points.

□ MetaGraph: Indexing and Analysing Nucleotide Archives at Petabase-scale
>>
https://www.biorxiv.org/content/10.1101/2020.10.01.322164v1.full.pdf
The MetaGraph framework provides a wide range of compressed data structures for trans- forming very large sequencing archives into k-mer dictionaries, associating each k-mer with labels representing metadata associated with its originating sequences.
The data structures underlying MetaGraph are designed to balance the trade-off between the space taken by the index and the time needed for query operations. MetaGraph can directly generate the k-mer spectrum in memory.
□ CellPaths: Inference of multiple trajectories in single cell RNA-seq data from RNA velocity
>>
https://www.biorxiv.org/content/10.1101/2020.09.30.321125v1.full.pdf
CellPaths is able to find multiple high-resolution trajectories instead of one single trajectory from traditional trajectory inference methods, and the trajectory structure is no longer constrained to be of any specific topology.
CellPaths takes in the nascent and mature mRNA count matrix, and calculate RNA velocity using the dynamical model of scVelo.
CellPaths ameliorates the noise in the data by constructing meta-cells and perform regression model to smooth the calculated velocity. The use of meta-cells can also reduce the downstream calculation complexity.
CellPaths is first-order pseudotime reconstruction method to assign order of true cells in each meta-cell separately and merge the order together according to the meta-cell path order.

□ RNA-Sieve: A likelihood-based deconvolution of bulk gene expression data using single-cell references
>>
https://www.biorxiv.org/content/10.1101/2020.10.01.322867v1.full.pdf
RNA-Sieve, a generative model and a likelihood-based inference method that uses asymptotic statistical theory and a novel optimization procedure to perform deconvolution of bulk RNA-seq data to produce accurate cell type proportion estimates.
The alternating optimization scheme is split into two components to better avoid sub-optimal local minima, with a final projection step handling flat extrema to avoid slow convergence.
RNA-Sieve algorithm can also perform joint deconvolutions, leveraging multiple samples to produce more reliable estimates while parallelizing much of the optimization.

□ GCAE: A deep learning framework for characterization of genotype data
>>
https://www.biorxiv.org/content/10.1101/2020.09.30.320994v1.full.pdf
GCAE - a Deep Learning framework denoted Genotype Convolutional Autoencoder for nonlinear dimensionality reduction of SNP data based on convolutional autoencoders.
The encoder transforms data to a lower-dimensional latent space through a series of convolutional, pooling and fully-connected layers.
The decoder reconstructs the input genotypes. The input consists of 3 layers: genotype data, a binary mask representing missing data, and a marker-specific trainable variable per SNP.
□ CrossICC: iterative consensus clustering of cross-platform gene expression data without adjusting batch effect
>>
https://academic.oup.com/bib/article-abstract/21/5/1818/5612157
CrossICC utilizes an iterative strategy to derive the optimal gene set and cluster number from consensus similarity matrix generated by consensus clustering.
CrossICC has the ability to automatically process arbitrary numbers of expression datasets, no matter which platform they came from.
CrossICC calculates correlation coefficient between samples and centroids of clusters to get a new feature vector of each samples. Based on this new matrix, samples were divided into new clusters.

□ Detox: Accurate determination of node and arc multiplicities in de bruijn graphs using conditional random fields
>>
https://bmcbioinformatics.biomedcentral.com/articles/10.1186/s12859-020-03740-x
Detox, Accurate determination of node and arc multiplicities in a de Bruijn graph using Conditional Random Fields.
Using the conservation of flow property, one might decide that a node or arc with a relatively poor coverage that falls in the zero-multiplicity interval, does have a multiplicity greater than zero because it provides an essential link in the graph.
a conditional random field (CRF) model to efficiently combine the coverage information within each node/arc individually with the information of surrounding nodes and arcs.
□ EnClaSC: a novel ensemble approach for accurate and robust cell-type classification of single-cell transcriptomes
>>
https://bmcbioinformatics.biomedcentral.com/articles/10.1186/s12859-020-03679-z
EnClaSC draws on the idea of ensemble learning in the feature selection, few-sample learning, neural network and joint prediction modules, respectively, and thus constitutes a novel ensemble approach for cell-type classification of single-cell transcriptomes.
EnClaSC superior to existing methods in the self-projection within a specific scRNA-seq dataset, the cell-type classification across different scRNA-seq datasets, various data dimensionality, and different data sparsity.

□ Bifrost: highly parallel construction and indexing of colored and compacted de Bruijn graphs
>>
https://genomebiology.biomedcentral.com/articles/10.1186/s13059-020-02135-8
Bifrost, a parallel and memory-efficient algorithm enabling the direct construction of the compacted de Bruijn graph without producing the intermediate uncompacted graph.
Bifrost is competitive with the state-of-the-art de Bruijn graph construction method BCALM2 and the unitig indexing tool Blight with the advantage that Bifrost is dynamic.
□ Incomplete multi-view gene clustering with data regeneration using Shape Boltzmann Machine
>>
https://www.sciencedirect.com/science/article/abs/pii/S0010482520302985
a deep Boltzmann machine-based incomplete multi-view clustering framework for gene clustering. seeking to regenerate the data of the three NCBI datasets in the incomplete modalities using Shape Boltzmann Machines.
The overall performance of the proposed multi-view clustering technique has been evaluated using the Silhouette index and Davies–Bouldin index, the improvement attained by the proposed incomplete multi-view clustering is statistically significant.

□ MapGL: inferring evolutionary gain and loss of short genomic sequence features by phylogenetic maximum parsimony
>>
https://bmcbioinformatics.biomedcentral.com/articles/10.1186/s12859-020-03742-9
MapGL simplifies phylogenetic inference of the evolutionary history of short genomic sequence features by combining the necessary steps into a single piece of software with a simple set of inputs and outputs.
MapGL can reliably disambiguate the mechanisms underlying differential regulatory sequence content across a broad range of phylogenetic topologies and evolutionary distances. MapGL provides the necessary context to evaluate how sequence gain / loss to species-specific divergence.

□ Copy-scAT: An R package for detection of large-scale and focal copy number alterations in single-cell chromatin accessibility datasets
>>
https://www.biorxiv.org/content/10.1101/2020.09.21.305516v1.full.pdf
Copy-scAT (copy number inference using single-cell ATAC), an R package that uses a combination of Gaussian segmentation and changepoint analysis to identify large-scale gains and losses and regions of focal loss and amplification in individual cells.
Segmental losses are called in a similar fashion, by calculating a quantile for each bin on a chromosome, running changepoint analysis to identify regions w/ abnormally low average signal, and Gaussian decomposition of total signal in that region to identify distinct clusters.

□ Varlock: privacy preserving storage and dissemination of sequenced genomic data
>>
https://www.biorxiv.org/content/10.1101/2020.09.16.299594v1.full.pdf
The Varlock uses a set of population allele frequencies to mask personal alleles detected in genomic reads. Each detected allele is replaced by a randomly selected population allele concerning its frequency.
Varlock masks personal alleles within mapped reads while preserving valuable non-sensitive properties of sequenced DNA fragments. Varlock is reversible, allowing the user with access to masked personal alleles to unmask them within an arbitrary region of the associated genome.
□ GPU acceleration of Darwin read overlapper for de novo assembly of long DNA reads
>>
https://bmcbioinformatics.biomedcentral.com/articles/10.1186/s12859-020-03685-1
Darwin-GPU consists of two parts: D-SOFT and GACT, which represent typical seed-and-extend methods. D-SOFT (Diagonal-band based Seed Overlapping based Filtration Technique) filters the search space by counting non-overlapping bases in matching Kmers in a band of diagonals.
GACT (Genomic Alignment using Constant Tracebackmemory) can align reads of arbitrary length using constant memory for the compute-intensive step. a GPU implementation of Darwin which accelerates the Smith-Waterman alignment with traceback computation used in the GACT stage.
Darwin-GPU packs the sequences on the GPU and compute the Smith-Waterman alignment matrix by dividing the matrix into 8x8 submatrices. To further reduce the memory transactions, writing to the traceback matrix is coalesced.
□ MEIRLOP: improving score-based motif enrichment by incorporating sequence bias covariates
>>
https://bmcbioinformatics.biomedcentral.com/articles/10.1186/s12859-020-03739-4
MEIRLOP (Motif Enrichment In Ranked Lists of Peaks) uses logistic regression to model the probability of a regulatory region sequence containing a motif as a function of a regulatory region’s activity score.
MEIRLOP offers two-sided hypothesis testing, it enables researchers to investigate motifs enriched towards either extreme of such ratios in a single pass, instead of having to run a motif enrichment analysis tool twice to investigate both extremes.
□ Sarek: A portable workflow for whole-genome sequencing analysis of germline and somatic variants
>>
https://f1000research.com/articles/9-63
Sarek supports several reference genomes and can handle data from WGS, WES and gene panels, and is intended to be used both as a production workflow at core facilities and as a stand-alone tool for individual research groups.
Sarek provides annotated VCF files, CNV reports and quality metrics. Sarek builds on a philosophy of reasonably narrow, independent workflows, written in the domain-specific language Nextflow.
□ SeqRepo: A system for managing local collections biological sequences
>>
https://www.biorxiv.org/content/10.1101/2020.09.16.299495v1.full.pdf
SeqRepo permits the use of conventional identifiers and digests for accessing and retrieving sequences. a locally-maintained SeqRepo instance enables pipelines to transparently mix public and custom sequences, such as masked sequences or alternative assemblies for variant calling.
SeqRepo provides fast random access to sequence slices. a local SeqRepo sequence collection yields significant performance benefits of up to 1300-fold over remote sequence collections.
□ The qBED track: a novel genome browser visualization for point processes
>>
https://academic.oup.com/bioinformatics/advance-article-abstract/doi/10.1093/bioinformatics/btaa771/5907909
qBED is tab-delimited, plain text format for discrete genomic data, such as transposon insertions. qBED files can be used to visualize non-calling card datasets, such as CADD scores and GWAS/eQTL hits.
the qBED track on the WashU Epigenome Browser, a novel visualization that enables researchers to inspect calling card data in their genomic context.

□ Si-C: method to infer biologically valid super-resolution intact genome structure from single-cell Hi-C data
>>
https://www.biorxiv.org/content/10.1101/2020.09.19.304923v1.full.pdf
Single-Cell Chromosome Conformation Calculator (Si-C) within the Bayesian theory framework and applied this approach to reconstruct intact genome 3D structures from the single-cell Hi-C data. Si-C adopts the steepest gradient descent algorithm to maximize the conditional probability.
Si-C directly describes the single-cell Hi-C contact restraints using an inverted-S shaped probability function of the distance between the contacted locus pair, instead of translating the binary contact into an estimated distance.
□ MBG: Minimizer-based Sparse de Bruijn Graph Construction
>>
https://www.biorxiv.org/content/10.1101/2020.09.18.303156v1.full.pdf
MBG - Minimizer based sparse de Bruijn Graph constructor. Homopolymer compress input sequences, winnow minimizers from hpc-compressed sequences, connect minimizers with an edge if they are adjacent in a read, unitigify.
MBG can construct graphs with arbitrarily high k-mer sizes. Transitive edges caused by sequencing errors are cleaned. Non-branching paths of the graph are then condensed into unitigs. MBG can run orders of magnitude faster than tools for building dense de Bruijn graphs.
□ GREEN-DB: a framework for the annotation and prioritization of non-coding regulatory variants in whole-genome sequencing
>>
https://www.biorxiv.org/content/10.1101/2020.09.17.301960v1.full.pdf
GREEN-DB (Genomic Regulatory Elements ENcyclopedia Database) integrates a collection of ~2.4M regulatory elements, additional functional elements (TFBS, DNase peaks, ultra-conserved non-coding elements (UCNE), and super-enhancers), and 7 non-coding impact prediction scores.
GREEN-VARAN (Genomic Regulatory Elements ENcyclopedia VARiant ANnotation) brings together in a single annotation framework information, non-coding impact prediction scores and population AF annotations, creating a system suitable for systematic WGS variants annotation.

□ High-Quality Genomes of Nanopore Sequencing by Homologous Polishing
>>
https://www.biorxiv.org/content/10.1101/2020.09.19.304949v1.full.pdf
Homopolish, a novel polishing tool based on a support-vector machine trained from homologous sequences extracted from closely- related genomes. the results indicate that Homopolish outperforms state-of-the-art Medaka and HELEN.
Although deep neural network is theoretically suitable for learning non-trivial features, Homopolish provides a set of manually-inspected features capable of capturing Nanopore systematic errors that may be directly used by other model developer.

□ MetaFusion: A high-confidence metacaller for filtering and prioritizing RNA-seq gene fusion candidates
>>
https://www.biorxiv.org/content/10.1101/2020.09.17.302307v1.full.pdf
MetaFusion is a flexible meta-calling tool that amalgamates the outputs from any number of fusion callers. Results from individual callers are converted into Common Fusion Format, a new file type that standardizes outputs from callers.
Calls are then annotated, merged using graph clustering, filtered and ranked to provide a final output of high confidence candidates. MetaFusion outperformed individual callers with respect to recall and precision on real and simulated datasets, achieving up to 100% precision.

□ MarkerCapsule: Explainable Single Cell Typing using Capsule Networks
>>
https://www.biorxiv.org/content/10.1101/2020.09.22.307512v1.full.pdf
MarkerCapsule reflects most advanced progress in deep learning, automatizes the annotation step, enables to coherently integrate heterogeneous data, and supports a human-mind-friendly interpretation, by relating marker genes with the fundamental units of capsule networks.
MarkerCapsule is based on non-negative matrix factorization and variational autoencoders that support the coherent integration of data from additional experimental resources, such as sc-ATAC-seq, sc-CITE- seq, or sc-bisulfite sequencing, and which is generally applicable.
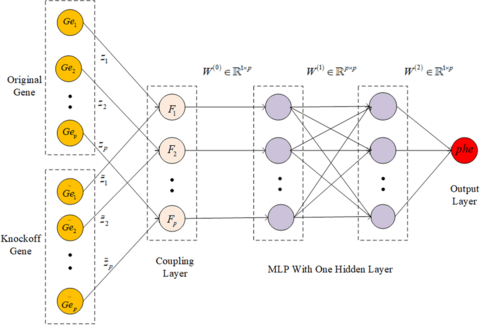
□ An embedded gene selection method using knockoffs optimizing neural network
>>
https://bmcbioinformatics.biomedcentral.com/articles/10.1186/s12859-020-03717-w
By constructing the knockoff feature genes of the original feature genes, this method not only make each feature gene to compete with each other, but also make each feature gene compete with its knockoff feature gene.
Knockoffs-NN can deal with the complex relationships between genes and phenotypes, and then mine candidate genes affecting specific phenotypic traits. Knockoffs-NN is suitable to process the complex non-linear data with independently identically distribution.

□ DeepHE: Accurately predicting human essential genes based on deep learning
>>
https://journals.plos.org/ploscompbiol/article?id=10.1371/journal.pcbi.1008229
DeepHE integrates two types of features, sequence features extracted from DNA sequence and protein sequence and features learned from PPI network, as its input.
DeepHE is based on the multilayer perceptron structure. All the hidden layers utilize the rectified linear unit (ReLU) activation function. A ReLU is simply defined as f(x) = max(0, x), which turns negative values to zero and grows linearly for positive values.
□ MMAP: A Cloud Computing Platform for Mining the Maximum Accuracy of Predicting Phenotypes from Genotypes
>>
https://academic.oup.com/bioinformatics/advance-article/doi/10.1093/bioinformatics/btaa824/5909989
Mining the Maximum Accuracy of Predicting phenotypes from genotypes (MMAP) is a knowledge-based cloud computing platform that continuously gains knowledge over time during application.
MMAP currently implements eight GS methods include gBLUP, compressed BLUP, SUPER BLUP, Bayes A, Bayes B, Bayes C, Bayes Cpi, and Bayesian LASSO. The mining system consists of an existing database and an interactive and dynamic evaluation (IDE) across GS methods and datasets.