
(Photo by Paul Wilson))

□ Reverse mathematics of rings
>> https://arxiv.org/pdf/2109.02037v1.pdf
Turning to a fine-grained analysis of four different definitions of Noetherian in the weak base system RCA0 + IΣ2.
The most obvious way is to construct a computable non-UFD in which every enumeration of a nonprincipal ideal computes ∅′. resp. a computable non-Σ1-PID in which every enumeration of a nonprincipal prime ideal computes ∅′.
an omega-dimensional vector space over Q w/ basis {xn : n ∈/ A}, the a′i are a linearly independent sequence in I. Let f(n) be the largest variable appearing in a′0,...,a′n+1. f(n) must be greater than the nth element of AC. f dominates μ∅′, and so a′0, a′1, . . . computes ∅′.

□ SUPERGNOVA: local genetic correlation analysis reveals heterogeneous etiologic sharing of complex traits
>> https://genomebiology.biomedcentral.com/articles/10.1186/s13059-021-02478-w
SUPERGNOVA is a principled framework for diverse types of genetic correlation analyses. And provides statistically rigorous & computationally efficient inference for both global / local genetic correlations and outperforms existing methods when applied to local genomic regions.
SUPERGNOVA resolves the statistical challenges by decorrelating local z scores with eigenvectors of the local LD matrix. SUPERGNOVA is equivalent to GNOVA which is a method that has been proven to achieve theoretical optimality compared to LDSC.

□ Atria: An Ultra-fast and Accurate Trimmer for Adapter and Quality Trimming
>> https://www.biorxiv.org/content/10.1101/2021.09.07.459340v1.full.pdf
The Atria algorithm can be used in a broad range of short-sequence matching applications, such as primer search and seed scanning before alignment.
Atria infers the insert DNA precisely by integrating both adapter information / reverse-complementary properties of pair-end reads within a decision tree. And finds possible overlapped regions with an ultra-fast designed byte-based matching algorithm - O(n) time with O(1) space.

□ Degrees of randomized computability: decomposition into atoms
>> https://arxiv.org/pdf/2109.04410v1.pdf
the structural properties of LV-degrees of the algebra of collections of sequences that are non-negligible in the sense that they can be computed by a probabilistic algorithm with positive probability.
the template for defining atoms of the algebra of LV-degrees and obtain the decomposition of the maximal LV-degree into a countable sequence of atoms and their non-zero complement – infinitely divisible LV-degree.
Constructing atoms defined by collections of hyperimmune sequences, moreover, a representation of LV-degree of the collection of all hyperimmune sequences will be obtained in the form of a union of an infinite sequence of atoms and an infinitely divisible element.

□ Robust haplotype-resolved assembly of diploid individuals without parental data
>> https://arxiv.org/pdf/2109.04785.pdf
a new algorithm that combines PacBio HiFi reads and Hi-C chromatin interaction data to produce a haplotype-resolved assembly without the sequencing of parents.
This algorithm directly operates on a HiFi assembly graph and tightly integrates Hi-C read mapping, phasing and assembly into one single executable program with no dependency to external tools.
Reduce the unitig bipartition to a graph max-cut problem and find a near optimal solution with a stochastic algorithm in the principle of simulated annealing. And also consider the topology of the assembly graph to reduce the chance of local optima.
The objective function takes a form similar to the Hamiltonian of Ising models and can be transformed to a graph maximum cut problem. It can be solved by a stochastic algorithm. After determining the phases, hifiasm spells contigs composed of unitigs in the same phase.

□ HyperEx: A Tool to Extract Hypervariable Regions from 16S rRNA Sequencing Data
>> https://www.biorxiv.org/content/10.1101/2021.09.03.455391v1.full.pdf
The Myers algorithm is a fast bit-vector algorithm for approximate string matching. It uses dynamic programming to rapidly match strings and has an application for pairwise sequence alignment given a distance.
HyperEx efficiently extracts V-regions from sequencing data based on primers sequences. HyperEx uses a slightly modified version of the Myers algorithm. HyperEx stands for HyperVariable Region Extractor.

□ MVGCN: data integration through multi-view graph convolutional network for predicting links in biomedical bipartite networks
>> https://academic.oup.com/bioinformatics/advance-article-abstract/doi/10.1093/bioinformatics/btab651/6367769
MVGCN (Multi-View Graph Convolution Network) constructs a multi-view heterogeneous network (MVHN) by combining the similarity networks w/ the biomedical bipartite network, and performs a self-supervised learning strategy to obtain node attributes as initial embeddings.
MVGCN combines embeddings of multiple neighborhood information aggregation (NIA) layers in each view, and integrate multiple views to obtain the final node embeddings, which are then fed into a discriminator to predict the existence of links.
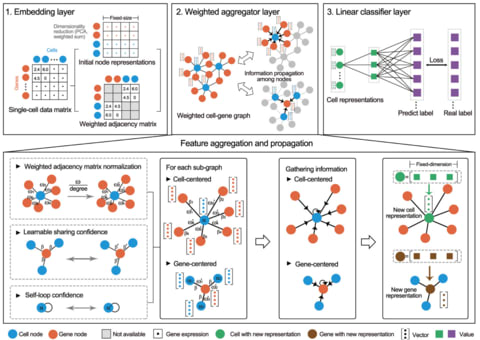
□ scDeepSort: a pre-trained cell-type annotation method for single-cell transcriptomics using deep learning with a weighted graph neural network
>> https://academic.oup.com/nar/advance-article/doi/10.1093/nar/gkab775/6368052
scDeepSort, using a state-of-the-art deep learning algorithm, i.e. a modified graph neural network (GNN) model. In brief, scDeepSort was constructed based on the weighted GNN framework and was then learned in two embedded high-quality scRNA-seq atlases.
The embedding layer stores the representation of graph nodes and is frozen during training. The weighted graph aggregator layer inductively learns graph structure information, generating linear separable feature space for cells.

□ Subspace Boosting: Randomized boosting with multivariable base-learners for high-dimensional variable selection and prediction
>> https://bmcbioinformatics.biomedcentral.com/articles/10.1186/s12859-021-04340-z
Subspace Boosting (SubBoost), base-learners can consist of several variables, allowing for multivariable updates in a single iteration. The ultimate selection of base-learners is based on information criteria leading to an automatic stopping of the algorithm.
Random Subspace Boosting (RSubBoost) additionally includes a random preselection of base-learners in each iteration, enabling the scalability to high-dimensional data.

□ CoDaCoRe: Learning Sparse Log-Ratios for High-Throughput Sequencing Data
>> https://academic.oup.com/bioinformatics/advance-article/doi/10.1093/bioinformatics/btab645/6366546
CoDaCoRe exploits a continuous relaxation. Combinatorial optimization over the set of log-ratios (equivalent to the set of pairs of disjoint subsets of the covariates), by continuous relaxation that can be optimized using gradient descent.
CoDaCoRe ensembles multiple regressors in a stage-wise additive fashion, where each successive balance is fitted on the residual from the current model. CoDaCoRe identifies a sequence of balances, in decreasing order of importance, each of which is sparse / interpretable.

□ scCTClust: Clustering single cell CITE-seq data with a canonical correlation based deep learning method
>> https://www.biorxiv.org/content/10.1101/2021.09.07.459236v1.full.pdf
scCTClust, a canonical correlation based deep learning method for clustering analysis over CITE-seq data. scCTClust imputes the characteristics of the high dimensional RNA part of data with a ZINB model-based autoencoder.
scCTClust can effectively utilize protein data to ameliorate clustering process. scCTClust occupied large memory space and is less stable than those methods without cca loss as SVD and matrix inversion are needed during the optimization of cca loss.

□ LongPhase: an ultra-fast chromosome-scale phasing algorithm for small and large variants
>> https://www.biorxiv.org/content/10.1101/2021.09.09.459623v1.full.pdf
LongPhase can simultaneously phase single nucleotide polymorphisms (SNPs) and SVs of a human genome in ~10-20 minutes, 10x faster than the state-of-the-art WhatsHap and Margin. LongPhase produces much larger phased blocks at almost chromosome level with only long reads N50=26Mbp.
in conjunction with Nanopore ultra-long reads, LongPhase can produce chromosome-level phasing without the need for additional trios, proximity ligation, and Strand-seq data.
the vertices become the initially-phased blocks, and the weights of the edges are the number of long reads spanning across SNPs/SVs in two adjacent blocks. These broken blocks are phased again by finding the longest pairs of disjoint paths in the graph.

□ Gramtools enables multiscale variation analysis with genome graphs
>> https://genomebiology.biomedcentral.com/articles/10.1186/s13059-021-02474-0
a framework for identifying and genotyping multiscale variation in genome graphs and show its successful implementation in gramtools. Multiscale variation analysis goes hand in hand with the gradual extension of reference genomes beyond their linear coordinates.
gramtools genotypes a nested DAG in which variant sites have been defined. Sites are genotyped independently, choosing the maximum-likelihood allele under a coverage model that draws on ideas from kallisto, incl. per-base coverage information / equivalence class counts for reads.

□ PRINCESS: comprehensive detection of haplotype resolved SNVs, SVs, and methylation
>> https://genomebiology.biomedcentral.com/articles/10.1186/s13059-021-02486-w
PRINCESS achieves high accuracy and long phasing even on low coverage datasets and can resolve repetitive, complex medical relevant genes that often escape detection.
PRINCESS extends the principle of phasing variants to structural variations and also includes modules to phase methylation data. PRINCESS includes code to enable the haplotype assessment of the methylation calls which provides a comprehensive foundation for maximal analysis.

□ Hobotnica: exploring molecular signature quality
>> https://www.biorxiv.org/content/10.1101/2021.09.12.459931v1.full.pdf
A signature based on a predefined Molecular Features Set (MFS), which is designed to distinguish biological conditions or phenotypes from each other — is one of major concepts of bioinformatics and precision medicine.
Hobotnica is designed to quantitatively evaluate Molecular Feature Set’s quality by their ability for data stratification from their inter-sample Distance Matrix, and to assess the statistical significance.

□ MetaTrass: High-quality Metagenomic Taxonomic Read Assembly of Single-Species based on co-barcoding sequencing data and references
>> https://www.biorxiv.org/content/10.1101/2021.09.13.459686v1.full.pdf
MetaTrass, a reference- guided assembling pipeline, which exploited both the public microbe reference genomes and long-range co-barcoding information, to assemble high-quality draft genomes from metagenomic co-barcoding reads.
The refined read sets of each species were independently assembled by Supernova. Several long fragments from different species genomes shared the same barcode in real stLFR libraries, thus involving some false positive reads from non-target species in the assembly process.

□ paraSBOLv: a foundation for standard-compliant genetic design visualization tools
>>
paraSBOLv that enables access to the full suite of Synthetic Biology Open Language Visual (SBOLv) glyphs through the use of machine-readable parametric glyph definitions.
sbolv-kaleidoscope, is a dynamic generative art tool that can create unique moving artworks consisting solely of customized parametric SBOLv glyphs.

□ Scallop2 enables accurate assembly of multiple-end RNA-seq data
>> https://www.biorxiv.org/content/10.1101/2021.09.03.458862v1.full.pdf
Scallop2 proposes a new algorithm that infers a single (long) path in the underlying splice graph that connects all individual ends in a read group.
Scallop2 then employs a “phase-preserving” graph-decomposition algorithm to decompose the splice graph into paths (i.e., transcripts) while all the inferred long paths are fully preserved. A resulting s-t path that contains any false vertex will be classified as transcript fragment.

□ CoNSEPT: Deciphering enhancer sequence using thermodynamics-based models and convolutional neural networks
>> https://academic.oup.com/nar/advance-article/doi/10.1093/nar/gkab765/6368530
CoNSEPT learns to predict expression from sequence with almost no pre-determined notion of cis-regulatory grammar such as activation, repression or pair-wise interactions between sites.
The scanning module computes the complementary sequence (negative strand) of the input enhancer and converts both strands into a one-hot encoded representation by replacing each nucleotide (A, C, G or T) with a 4-dimensional vector.

□ The ‘un-shrunk’ partial correlation in Gaussian graphical models
>> https://bmcbioinformatics.biomedcentral.com/articles/10.1186/s12859-021-04313-2
The application of GGMs to reconstruct regulatory networks is commonly performed using shrinkage to overcome the ‘high-dimensional problem’. Besides it advantages, the shrinkage introduces a non-linear bias in the partial correlations.
an improvement for the ‘shrunk’ partial correlations inferred w/ the LW-shrinkage. the effect of the shrinkage value on the shrunk partial correlation. this effect is non-linear, the magnitudes / order of the estimated partial correlations change w/ varying the shrinkage value.
□ SNPxE: SNP-environment interaction pattern identifier
>> https://bmcbioinformatics.biomedcentral.com/articles/10.1186/s12859-021-04326-x
SNPxE evaluates 27 interaction patterns for an ordinal environment factor and 18 patterns for a categorical environment factor. For detecting SNP-environment interactions, SNPxE considers three major components: model structure, SNP’s inheritance mode, and risk direction.
For SNPxE, the outcome can be a binary or continuous variable. For a continuous outcome, the linear-based SNPxE based on linear regression will be used. For a binary outcome, the logistic-based SNPxE based on logistic regression will be applied.
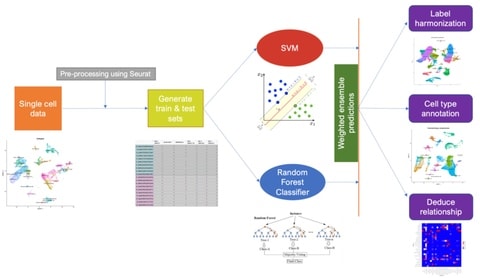
□ ELeFHAnt: A supervised machine learning approach for label harmonization and annotation of single cell RNA-seq data
>> https://www.biorxiv.org/content/10.1101/2021.09.07.459342v1.full.pdf
Ensemble Learning for Harmonization and Annotation of Single Cells (ELeFHAnt) provides an easy to use R package to annotate clusters of single cells, harmonize labels across single cell datasets to generate a unified atlas and infer relationship among celltypes b/n 2 datasets.
ELeFHAnt provides users with the flexibility of choosing a single machine learning based classifier or letting ELeFHAnt automatically use the power of randomForest and SVM to make predictions. It has 3 functions: CelltypeAnnotation, LabelHarmonization, and DeduceRelationship.

□ AMGT-TS: An integrated strategy for target SSR genotyping with toleration of nucleotide variations in the SSRs and flanking regions
>> https://bmcbioinformatics.biomedcentral.com/articles/10.1186/s12859-021-04351-w
AMGT-TS, a new integrated strategy named the accurate microsatellite genotyping tool based on targeted sequencing. It can achieve accurate Simple Sequence Repeat genotyping based on targeted sequencing, and it can tolerate SNV in the SSRs and flanking regions.
BMA, a broad matching algorithm that can quickly and accurately achieve SSR typing for ultradeep coverage and high-throughput analysis of loci with SNVs compatibility and grouping of typed reads for further in-depth information mining.

□ VC@Scale: Scalable and high-performance variant calling on cluster environments
>> https://academic.oup.com/gigascience/article/10/9/giab057/6366276
VC@Scale, a scalable, parallel implementation of NGS data pre-processing and variant-calling workflows. Its design tightly integrates most pre-processing workflow stages, using Spark built-in functions to sort reads by coordinates and mark duplicates efficiently.
VC@Scale detects the same number of indel true-positive and false-negative results and slightly fewer false-positive results compared to the baseline. The load-balanced BAM file output is used in Deep-Variant, making variant calling more efficient on a compute cluster.

□ STRIDE: accurately decomposing and integrating spatial transcriptomics using single-cell RNA sequencing
>> https://www.biorxiv.org/content/10.1101/2021.09.08.459458v1.full.pdf
Spatial TRanscrIptomics DEconvolution by topic modeling (STRIDE), is a computational method to decompose cell types from spatial mixtures by leveraging topic profiles trained from single-cell transcriptomics.
STRIDE could deconvolve the cell-type compositions of spatial transcriptomics based on latent topics. And improves the identification of spatial localized genes and domains.

□ scBasset: Sequence-based modeling of single cell ATAC-seq using convolutional neural networks
>> https://www.biorxiv.org/content/10.1101/2021.09.08.459495v1.full.pdf
scBasset is trained to predict individual cell accessibility from the DNA sequence underlying ATAC peaks, learning a vector embedding to represent the single cells in the process.
The linear transfor mation matrix comprises a vector representation of each task, which specifies how to make use of each of the sequence embedding latent variables to predict cell-specific accessibility.
Clustering the model’s cell embeddings achieves greater alignment with ground-truth cell type labels. scBasset takes as input a 1344 bp DNA sequence from each peak’s center and one-hot encodes it as a 4×1344 matrix.

□ Categorical Syntax and Consequence Relations
>> https://arxiv.org/pdf/2109.04291.pdf
The syntax of logic over algebraic signature is quite simple, since it does not involve variable binding. The syntax of such logics can then be studied from a universal algebra point of view, using the language of monads and algebras of monads.
a consequence relation can be defined on various sets, depending on the style of the inference system. An asymmetric consequence relation is usually considered as a binary relation ⊢ ⊆ PFml × Fml. A symmetric consequence relation is a binary relation ⊢ ⊆ PFml × PFml.
the syntactic monad F that F-algebras are the same as Σ-algebras, it is well-known that P-algebras are exactly suplattices, i.e. an equivalence — isomorphism, actually — of categories.
□ Chris Mason RT
>> https://twitter.com/mason_lab/status/1436773940169478149?s=20
Our lab was just selected by NASA
for a project, "Spatiotemporal Mapping of the Impact of Spaceflight on the Heart and Brain," where we will create extensive spatial omics data on tissues before and after spaceflight. Thx!
NASA Selects 10 Space Biology Research Projects that will Enable Organisms to Thrive in Deep Space
https://science.nasa.gov/science-news/biological-physical/nasa%3Dselects-10-space-biology-research-projects-that-will-enable-organisms-to-thrive-in-deep-space
□ Nanopore Sequencing Firm iNanoBio Says Fast Sensor Will Result in Speedy Platform
>> https://www.genomeweb.com/sequencing/nanopore-sequencing-firm-inanobio-says-fast-sensor-will-result-speedy-platform

□ MinoTour, real-time monitoring and analysis for Nanopore Sequencers.
>> https://www.biorxiv.org/content/10.1101/2021.09.10.459783v1.full.pdf
minoTour is a web-based real-time laboratory information management system (LIMS) for Oxford Nanopore Technology (ONT) sequencers built using the Django framework.
minoTour can monitor the activity of a sequencer in real time independent of analysing basecalled files providing a breakdown and analysis of live sequencing metrics via integration with ONT’s minKNOW API and parsing of sequence files as they are generated.
□ Entropy coding in Oodle Data: Huffman coding
>> https://fgiesen.wordpress.com/2021/08/30/entropy-coding-in-oodle-data-huffman-coding/
struct HuffTableEntry {
uint8_t len; // length of code in bits
uint8_t sym; // symbol value
};
while (!done) {
bitbuf.refill();
// can decode up to 25 bits without refills!
// Decode first symbol
{
intptr_t index = bitbuf.peek(11);
HuffTableEntry e = table[index];
bitbuf.consume(e.len);
output.append(e.sym);
}
□ Benchmarking tools for DNA repeat identification in diverse genomes
>> https://www.biorxiv.org/content/10.1101/2021.09.10.459798v1.full.pdf
Understanding the diversity of repeat sequences and structures, various tools and algorithms have been developed to locate the repetitive patterns in the genome.
Phobos can be marked as the best tool for perfect minisatellites and imperfect repeat identification and has performed many times better than TRF both in repeat detection and execution time.

□ Analytic Pearson residuals for normalization of single-cell RNA-seq UMI data
>> https://genomebiology.biomedcentral.com/articles/10.1186/s13059-021-02451-7
a more parsimonious model produces stable estimates even without smoothing and is equivalent to a special case of GLM-PCA.
the estimates of per-gene overdispersion parameter θg in the original paper exhibit substantial and systematic bias. the Bayesian procedure shrinks their expression towards zero whereas our approach yields large Pearson residuals.

□ Random Forest Factorization Reveals Latent Structure in Single Cell RNA Sequencing Data
>> https://www.biorxiv.org/content/10.1101/2021.09.13.460168v1.full.pdf
A Random Forest Regressor takes a very similar approach by iteratively partitioning the dataset and then treating each partition as an entirely new problem.
However RFRs are generally regarded as somewhat opaque tools that don’t provide interpretable information on the structure of the underlying data.
to construct a node, the raw data matrix is bootstrapped by sample with replacement (eg rows are randomly selected and copied into a new matrix), then the sample bootstrap matrix columns are divided into inputs and outputs without replacement.
The input and output columns are then bootstrapped with replacement producing the node input and node output matrices of a specified dimension.

□ The value of genotype-specific reference for transcriptome analyses
>> https://www.biorxiv.org/content/10.1101/2021.09.14.460213v1.full.pdf
Mapping barley cultivar Barke RNA-seq reads to the Barke genome and to the cultivar Morex genome (common barley genome reference) to construct a genotype specific Reference Transcript Dataset (sRTD) and a common Reference Transcript Datasets (cRTD).
The proportions of sequence overlap were evaluated with precision (TP/(TP+FP)) and recall (TP/(TP+FN)), and their weighted mean F1 score (2×(Recall × Precision) / (Recall + Precision)) which took both recall and precision into account.