
□ Nebula: ultra-efficient mapping-free structural variant genotyper
>> https://academic.oup.com/nar/advance-article/doi/10.1093/nar/gkab025/6121467
Nebula is a two-stage approach and consists of a k-mer extraction phase and a genotyping phase. Nebula extracts a collection of k-mers that represent the input SVs. Nebula can count millions of k-mers in WGS reads at a rate of >500 000 reads per sec using a single processor core.
For a SV supported by multiple k-mers, the likelihood of each possible genotype g ∈ {0/0, 0/1, 1/1} can be calculated as L(g|k1,k2,k3,...) = p(k1,k2,k3,...|g)L(g|k1,k2,k3,...) = p(k1,k2,k3,...|g) where each ki represents a different k-mer.
Nebula only requires the SV coordinates. Genotype imputation algorithms can be incorporated into Nebula’s pipeline to improve the method’s accuracy and ability to genotype variants that are difficult to genotype using solely k-mers, e.g. SVs with breakpoints in repeat regions.

□ scETM: Learning interpretable cellular and gene signature embeddings from single-cell transcriptomic data
>> https://www.biorxiv.org/content/10.1101/2021.01.13.426593v1.full.pdf
scETM (single-cell Embedded Topic Model), a deep generative model that recapitulates known cell types by inferring the latent cell topic mixtures via a variational autoencoder. scETM is scalable to over 10^6 cells and enables effective knowledge transfer across datasets.
scETM models the cells-by-genes read-count matrix by factorizing it into a cells-by-topics matrix θ and a topics-by-genes matrix β, which is further decomposed into topics-by-embedding α and embedding-by-genes ρ matrices.
By the tri-factorization design, scETM can incorporate existing pathway information into gene embeddings during the model training to further improve interpretability, which is a salient feature compared to the related methods such as scVI-LD.

□ DeepSVP: Integration of genotype and phenotype for structural variant prioritization using deep learning
>> https://www.biorxiv.org/content/10.1101/2021.01.28.428557v1.full.pdf
DeepSVP significantly improves the success rate of finding causative variants. DeepSVP uses as input an annotated Variant Call Format (VCF) file of an individual and clinical phenotypes encoded using the Human Phenotype Ontology.
DeepSVP overcomes the limitation of missing phenotypes by incorporating information: mainly the functions of gene products, GE in individual cellt types, and anatomical sites of expression and systematically relating them to their phenotypic consequences through ontologies.

□ Multidimensional Boolean Patterns in Multi-omics Data
>> https://www.biorxiv.org/content/10.1101/2021.01.12.426358v1.full.pdf
a variety of mutual information-based methods are not suitable for estimating the strength of Boolean patterns because of the effects of the number of populated partitions and disbalance of the partitions’ population on the pattern's score.
Multidimensional patterns may not just be present but could dominate the landscape of multi-omics data, which is not surprising because complex interactions between components of biological systems are unlikely to be reduced to simple pairwise interactions.

□ Connectome: computation and visualization of cell-cell signaling topologies in single-cell systems data
>> https://www.biorxiv.org/content/10.1101/2021.01.21.427529v1.full.pdf
Connectome is a multi-purpose tool designed to create ligand-receptor mappings in single-cell data, to identify non-random patterns representing signal, and to provide biologically- informative visualizations of these patterns.
Mean-wise connectomics has the advantage of accommodating the zero-values intrinsic to single-cell data, while simplifying the system so that every cell parcellation is represented by a single, canonical node.
An edgeweight must be defined for each edge in the celltype-celltype connectomic dataset. Connectome, by default, calculates two distinct edgeweights, each of which captures biologically relevant information.

□ scAdapt: Virtual adversarial domain adaptation network for single cell RNA-seq data classification across platforms and species
>> https://www.biorxiv.org/content/10.1101/2021.01.18.427083v1.full.pdf
scAdapt used both the labeled source and unlabeled target data to train an enhanced classifier, and aligned the labeled source centroid and pseudo-labeled target centroid to generate a joint embedding.
scAdapt includes not only the adversary-based global distribution alignment, but also category-level alignment to preserve the discriminative structures of cell clusters in low dimensional feature (i.e., embedding) space.
At the embedding space, batch correction is achieved at global- and class-level: ADA loss is employed to perform global distribution alignment and semantic alignment loss minimizes the distance between the labeled source centroid and pseudo-labeled target centroid.

□ LIBRA: Machine Translation between paired Single Cell Multi Omics Data
>> https://www.biorxiv.org/content/10.1101/2021.01.27.428400v1.full.pdf
LIBRA, an encoder-decoder architecture using AutoEncoders (AE). LIBRA encodes one omic and decodes the other omics to and from a reduced space.
the Preserved Pairwise Jacard Index (PPJI), a non-symmetric distance metric aimed to investigate the added value (finer granularity) of clustering B (multi-omic) in relation to cluster A.
LIBRA consists of two NN; the first NN is designed similarly to an Autoencoder, its input / output correspond to two different paired multi-modal datasets. This identifies a Shared Latent Space for two data-types. The second NN generates a mapping to the shared projected space.

□ DYNAMITE: a phylogenetic tool for identification of dynamic transmission epicenters
>> https://www.biorxiv.org/content/10.1101/2021.01.21.427647v1.full.pdf
DYNAMITE (DYNAMic Identification of Transmission Epicenters), a cluster identification algorithm based on a branch-wise (rather than traditional clade-wise) search for cluster criteria, allowing partial clades to be recognized as clusters.
DYNAMITE’s branch-wise approach enables the identification of clusters for which the branch length distribution within the clade is highly skewed as a result of dynamic transmission patterns.
□ ALGA: Genome-scale de novo assembly
>> https://academic.oup.com/bioinformatics/advance-article/doi/10.1093/bioinformatics/btab005/6104855
ALGA (ALgorithm for Genome Assembly) is a genome-scale de novo sequence assembler based on the overlap graph strategy. The method accepts at the input reads from the next generation DNA sequencing, paired or not.
In ALGA, the level of similarity is set to 95% measured in vertices of compared uncompressed paths. The similarity is determined with taking into consideration also vertices corresponding to reverse complementary versions of reads.
ALGA can be used without setting any parameter. The parameters are adjusted internally by ALGA on the basis of input data. Only one optional parameter is left, the maximum allowed error rate in overlaps of reads, with its default value 0.

□ Scalpel: Information-based Dimensionality Reduction for Rare Cell Type Discovery
>> https://www.biorxiv.org/content/10.1101/2021.01.19.427303v1.full.pdf
Scalpel leverages mathematical information theory to create featurizations which accurately reflect the true diversity of transcriptomic data. Scalpel’s information-theoretic paradigm forms a foundation for further innovations in feature extraction in single-cell analysis.
Scalpel’s information scores are similar in principle to Inverse Document Frequency, a normalization approach widely used in text processing and in some single-cell applications, whereby each feature is weighted by the logarithm of its inverse frequency.
□ Nebulosa: Recover single cell gene expression signals by kernel density estimation
>> https://academic.oup.com/bioinformatics/advance-article-abstract/doi/10.1093/bioinformatics/btab003/6103785
Nebulosa aims to recover the signal from dropped-out features by incorporating the similarity between cells allowing a “convolution” of the cell features.
Nebulosa makes use of weighted kernel density estimation methods to represent the expression of gene features from cell neighnours. Besides counts and normalised gene expression, It is possible to visualise metadata variables, and feature information from other assays.
□ S3: High-content single-cell combinatorial indexing
>> https://www.biorxiv.org/content/10.1101/2021.01.11.425995v1.full.pdf
a novel adaptor-switching strategy, ‘s3’, capable of producing one-to-two order-of-magnitude improvements in usable reads obtained per cell for chromatin accessibility (s3-ATAC), whole genome sequencing (s3-WGS), and whole genome plus chromatin conformation (s3-GCC).
S3, Symmetrical Strand Sci uses single-adapter transposition to incorporate the forward primer sequence, the Tn5 mosaic end sequence and a reaction-specific DNA barcode. This format permits the use of a DNA index sequence embedded within the transposase adaptor complex.

□ MichiGAN: Sampling from Disentangled Representations of Single-Cell Data Using Generative Adversarial Networks
>> https://www.biorxiv.org/content/10.1101/2021.01.15.426872v1.full.pdf
The MichiGAN network provides an alternative to the current disentanglement learning literature, which focuses on learning disentangled representations through improved VAE-based or GAN-based methods, but rarely by combining them.
MichiGAN does not need to learn its own codes, and thus the discriminator can focus exclusively on enforcing the relationship between code and data.
MichiGAN’s ability to sample from a disentangled representation allows predicting unseen combinations of latent variables using latent space arithmetic.
the entropy of the latent embeddings for the held-out data and the latent values predicted by latent space arithmetic by calculating ∆H = H{τF ake(Z), g(X)} − H{τReal(Z), g(X)}, where τF ake is calculated by latent space arithmetic and τReal is calculated using the encoder.

□ PrismExp: Predicting Human Gene Function by Partitioning Massive RNA-seq Co-expression Data
>> https://www.biorxiv.org/content/10.1101/2021.01.20.427528v1.full.pdf
While some gene expression resources are well organized into individual tissues, these resources only cover a fraction of all human tissues and cell types. More diverse datasets such as ARCHS4 lack accurate tissue classification of individual samples.
Partitioning RNA-seq data Into Segments for Massive co-EXpression-based gene function Predictions (PrismExp), generates a high dimensional feature space. The generated feature space automatically encodes tissue specific information via vertical partitioning of the data matrix.
□ satuRn: Scalable Analysis of differential Transcript Usage for bulk and single-cell RNA-sequencing applications
>> https://www.biorxiv.org/content/10.1101/2021.01.14.426636v1.full.pdf
satuRn can deal with realistic proportions of zero counts, and provides direct inference on the biologically relevant transcript level. In brief, satuRn adopts a quasi-binomial (QB) generalized linear model (GLM) framework.
satuRn requires a matrix of transcript-level expression counts, which may be obtained either through pseudo-alignment using kallisto. satuRn can extract biologically relevant information from a large scRNA-seq dataset that would have remained obscured in a canonical DGE analysis.

□ CONSENT: Scalable long read self-correction and assembly polishing with multiple sequence alignment
>> https://www.nature.com/articles/s41598-020-80757-5
CONSENT (Scalable long read self-correction and assembly polishing w/ multiple sequence alignment) is a self-correction method. It computes overlaps b/n the long reads, in order to define an alignment pile (a set of overlapping reads used for correction) for each read.
CONSENT using a method based on partial order graphs. And uses an efficient segmentation strategy based on k-mer chaining. This segmentation strategy thus allows to compute scalable multiple sequence alignments. it allows CONSENT to efficiently scale to ONT ultra-long reads.

□ VeloSim: Simulating single cell gene-expression and RNA velocity
>> https://www.biorxiv.org/content/10.1101/2021.01.11.426277v1.full.pdf
VeloSim is able to simulate the whole dynamics of mRNA molecule generation, produces unspliced mRNA count matrix, spliced mRNA count matrix and RNA velocity at the same time.
VeloSim outputs the assignment of cells to each trajectory lineage, and the pseudotime of each cell. VeloSim uses the two-state kinetic model, And allows to provide any trajectory structure that is made of basic elements of “cycle” and “linear”.
□ The variant call format provides efficient and robust storage of GWAS summary statistics
>> https://genomebiology.biomedcentral.com/articles/10.1186/s13059-020-02248-0
A limitation of the current summary statistics formats, including GWAS-VCF, is the lack of a widely adopted and stable representation of sequence variants that can be used as a universal unique identifier for the said variants.
Adapting the variant call format to store GWAS summary statistics (GWAS-VCF) and developed a set of requirements for a suitable universal format in downstream analyses.

□ SquiggleNet: Real-Time, Direct Classification of Nanopore Signals
>> https://www.biorxiv.org/content/10.1101/2021.01.15.426907v1.full.pdf
SquiggleNet employs a convolutional architecture, using residual blocks modified from ResNet to perform one-dimensional (time-domain) convolution over squiggles.
SquiggleNet operates faster than the DNA passes through the pore, allowing real-time classification and read ejection. the classifier achieves significantly higher accuracy than base calling followed by sequence alignment.
□ MegaR: an interactive R package for rapid sample classification and phenotype prediction using metagenome profiles and machine learning
>> https://bmcbioinformatics.biomedcentral.com/articles/10.1186/s12859-020-03933-4
The MegaR employs taxonomic profiles from either whole metagenome sequencing or 16S rRNA sequencing data to develop machine learning models and classify the samples into two or more categories.
MegaR provides an error rate for each prediction model generated that can be found under the Error Rate tab. The error rate of prediction on a test set is a better estimate of model accuracy, which can be estimated using a confusion matrix.

□ UniverSC: a flexible cross-platform single-cell data processing pipeline
>> https://www.biorxiv.org/content/10.1101/2021.01.19.427209v1.full.pdf
UniverSC, a universal single-cell processing tool that supports any UMI-based platform. Its command-line tool enables consistent and comprehensive integration, comparison, and evaluation across data generated from a wide range of platforms.
UniverSC assumes Read 1 of the FASTQ to contain the cell barcode and UMI and Read 2 to contain the transcript sequences which will be mapped to the reference, as is common in 3’ scRNA-seq protocols.
□ Identification of haploinsufficient genes from epigenomic data using deep forest
>> https://academic.oup.com/bib/advance-article-abstract/doi/10.1093/bib/bbaa393/6102676
The multiscale scanning is proposed to extract local contextual representations from input features under Linear Discriminant Analysis. the cascade forest structure is applied to obtain the concatenated features directly by integrating decision-tree-based forests.
to exploit the complex dependency structure among haploinsufficient genes, the LightGBM library is embedded into HaForest to reveal the highly expressive features.
□ 2-kupl: mapping-free variant detection from DNA-seq data of matched samples
>> https://www.biorxiv.org/content/10.1101/2021.01.17.427048v1.full.pdf
2-kupl extracts case-specific k-mers and the matching counterpart k-mers corresponding to a putative mutant and reference sequences and merges them into contigs.
the number of k-mers considered from unaltered regions and non-specific variants is drastically reduced compared with DBG-based methods. 2-kupl outputs the contig harboring the variation and the corresponding putative reference without the variation for each event.
□ scBUC-seq: Highly accurate barcode and UMI error correction using dual nucleotide dimer blocks allows direct single-cell nanopore transcriptome sequencing
>> https://www.biorxiv.org/content/10.1101/2021.01.18.427145v1.full.pdf
scBUC-seq, a novel approach termed single-cell Barcode UMI Correction sequencing can be applied to correct either short-read or long-read sequencing, thereby allowing users to recover more reads per cell and permits direct single-cell Nanopore sequencing for the first time.
scBUC-seq uses direct Nanopore sequencing, which circumvents the need for additional short-read alignment data. And can be used to error-correct both short-read and long-read data, thereby recovering sequencing data that would otherwise be lost due to barcode misassignment.
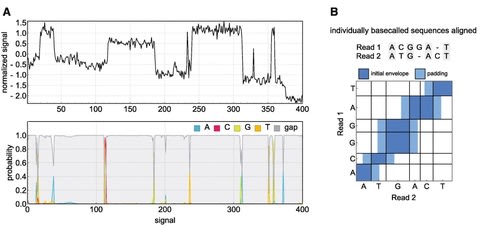
□ PoreOver: Pair consensus decoding improves accuracy of neural network basecallers for nanopore sequencing
> https://genomebiology.biomedcentral.com/articles/10.1186/s13059-020-02255-1
PoreOver is a basecalling tool for the Oxford Nanopore sequencing platform and is primarily intended for the task of consensus decoding raw basecaller probabilities for higher accuracy 1D2 sequencing.
PoreOver includes a standalone RNN basecaller (PoreOverNet) that can be used to generate these probabilities, though the highest consensus accuracy is achieved in combination with Bonito, one of ONT's research basecallers.
The pairwise dynamic programming approach could be extended to multiple reads, although the curse of dimensionality (a full dynamic programming alignment of N reads takes O(T^N) steps) would necessitate additional heuristics to narrow down the search space.

□ Freddie: Annotation-independent Detection and Discovery of Transcriptomic Alternative Splicing Isoforms
>> https://www.biorxiv.org/content/10.1101/2021.01.20.427493v1.full.pdf
Freddie, a multi-stage novel computational method aimed at detecting isoforms using LR sequencing without relying on isoform annotation data. The design of each stage in Freddie is motivated by the specific challenges of annotation-free isoform detection from noisy LRs.
Freddie achieves accuracy on par with FLAIR despite not using any annotations and outperforms StringTie2 in accuracy. Furthermore, Freddie’s accuracy outpaces FLAIR’s when FLAIR is provided with partial annotations.

□ SNF-NN: computational method to predict drug-disease interactions using similarity network fusion and neural networks
>> https://bmcbioinformatics.biomedcentral.com/articles/10.1186/s12859-020-03950-3
SNF-NN integrates similarity measures, similarity selection, Similarity Network Fusion (SNF), and Neural Network (NN) and performs a non-linear analysis that improves the drug-disease interaction prediction accuracy.
SNF-NN achieves remarkable performance in stratified 10-fold cross-validation with AUC-ROC ranging from 0.879 to 0.931 and AUC-PR from 0.856 to 0.903.

□ DEPP: Deep Learning Enables Extending Species Trees using Single Genes
>> https://www.biorxiv.org/content/10.1101/2021.01.22.427808v1.full.pdf
Deep-learning Enabled Phylogenetic Placement (DEPP) framework does not rely on pre-specified models of sequence evolution or gene tree discordance; instead, it uses highly parameterized DNNs to learn both aspects from the data.
The distance-based LSPP problem provides a clean mathematical formulation. DEPP learns a neural network to embed sequences in a high dimensional Euclidean space, such that pairwise distances in the new space correspond to the square root of tree distances.

□ FIVEx: an interactive multi-tissue eQTL browser
>> https://www.biorxiv.org/content/10.1101/2021.01.22.426874v1.full.pdf
FIVEx (Functional Interpretation and Visualization of Expression), an eQTL-focused web application that leverages the widely used tools LocusZoom and LD server.
FIVEx visualizes the genomic landscape of cis-eQTLs across multiple tissues, focusing on a variant, gene, or genomic region. FIVEx is designed to aid the interpretation of the regulatory functions of genetic variants by providing answers to functionally relevant questions.
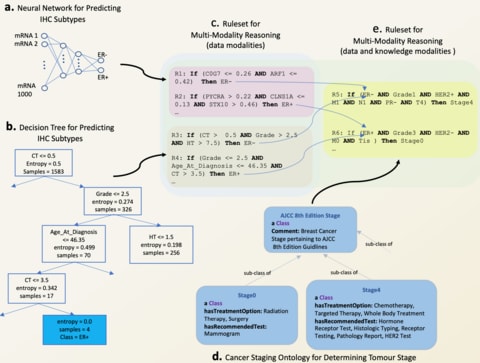
□ REM: An Integrative Rule Extraction Methodology for Explainable Data Analysis in Healthcare
>> https://www.biorxiv.org/content/10.1101/2021.01.22.427799v1.full.pdf
REM functionalities also allow direct incorporation of knowledge into data-driven reasoning by catering for rule ranking based on the expertise of clinicians/physicians.
REM embodies a set of functionalities that can be used for revealing the connections between various data modalities (cross-modality reasoning) and integrating the modalities for multi-modality reasoning, despite being modelled using a combination of DNNs and tree-based.
□ GECO: gene expression clustering optimization app for non-linear data visualization of patterns
>> https://bmcbioinformatics.biomedcentral.com/articles/10.1186/s12859-020-03951-2
GECO (Gene Expression Clustering Optimization), a minimalistic GUI app that utilizes non-linear reduction techniques to visualize expression trends in biological data matrices (such as bulk RNA-seq, single cell RNA-seq, or proteomics).
GECO has a system for automatic data cleaning to ensure that the data loaded into the dimensionality reduction algorithms are properly formatted. GECO provides simple options to remove these confounding entries.
□ LSTrAP-Kingdom: an automated pipeline to generate annotated gene expression atlases for kingdoms of life
>> https://www.biorxiv.org/content/10.1101/2021.01.23.427930v1.full.pdf
the Large-Scale Transcriptomic Analysis Pipeline in Kingdom of Life (LSTrAP-Kingdom) pipeline generates quality-controlled, annotated gene expression matrices that rival the manually curated gene expression data in identifying functionally-related genes.
LSTrAP-Kingdom can be annotated with a simple natural language processing pipeline that leverages organ ontology information. the coexpression networks obtained by our pipeline perform as well as networks constructed from manually assembled matrices.
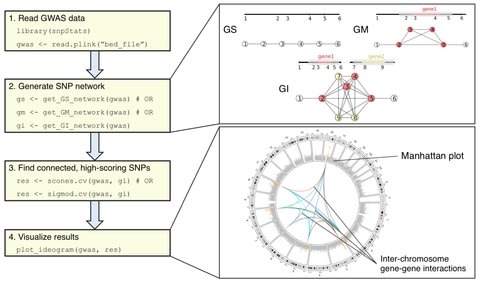
□ martini: an R package for genome-wide association studies using SNP networks
>> https://www.biorxiv.org/content/10.1101/2021.01.25.428047v1.full.pdf
Martini implements two network-guided biomarker discovery algorithms based on graph cuts that can handle such large networks: SConES and SigMod.
Both algorithms use parameters that control the relative importance of the SNPs’ association scores, the number of SNPs selected, and their interconnection.

□ GLUER: integrative analysis of single-cell omics and imaging data by deep neural network
>> https://www.biorxiv.org/content/10.1101/2021.01.25.427845v1.full.pdf
GLUER combines joint nonnegative matrix factorization, mutual nearest neighbor algorithm, and deep neural network to integrate data of different modalities. co-embedded data is then computed by combining the reference factor loading matrix and query factor loading matrices.

□ SMILE: Mutual Information Learning for Integration of Single Cell Omics Data
>> https://www.biorxiv.org/content/10.1101/2021.01.28.428619v1.full.pdf
A one-layer MLP generating a 32-dimension vector will produce rectified linear unit (ReLU) activated output, and the other will produce probabilities of pseudo cell-types with SoftMax activation. NCE was applied on the 32-dimension output and pseudo probabilities.