
“When the theorem is proved from the right axioms, the axioms can be proved from the theorem.”
—Harvey Friedman [Fri74]
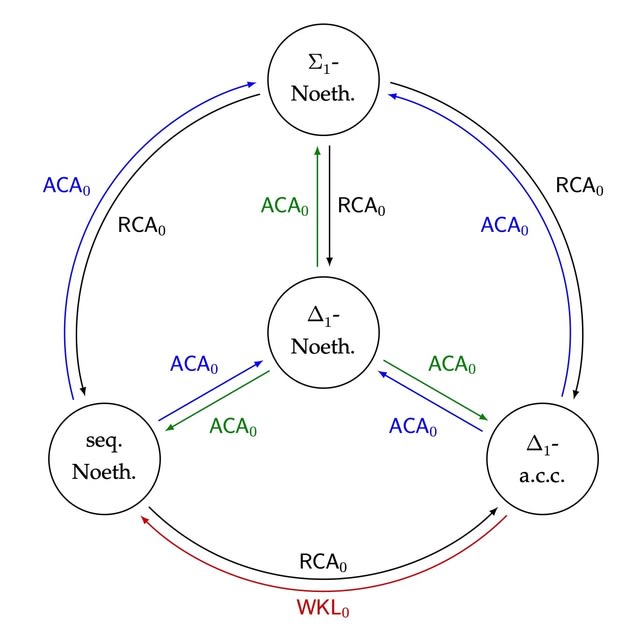
□ Reverse mathematics of rings
>> https://arxiv.org/pdf/2109.02037v1.pdf
Turning to a fine-grained analysis of four different definitions of Noetherian in the weak base system RCA0 + IΣ2.
The most obvious way is to construct a computable non-UFD in which every enumeration of a nonprincipal ideal computes ∅′. resp. a computable non-Σ1-PID in which every enumeration of a nonprincipal prime ideal computes ∅′.
an omega-dimensional vector space over Q w/ basis {xn : n ∈/ A}, the a′i are a linearly independent sequence in I. Let f(n) be the largest variable appearing in a′0,...,a′n+1. f(n) must be greater than the nth element of AC. f dominates μ∅′, and so a′0, a′1, . . . computes ∅′.
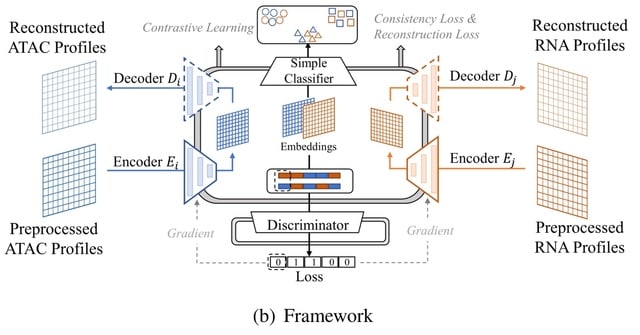
□ Con-AAE: Contrastive Cycle Adversarial Autoencoders for Single-cell Multi-omics Alignment and Integration
>> https://www.biorxiv.org/content/10.1101/2021.12.12.472268v1.full.pdf
Contrastive Cycle adversarial Autoencoders (Con-AAE) can efficiently map the above data with high sparsity and noise from different spaces to a low-dimensional manifold in a unified space, making the downstream alignment and integration straightforward.
Con-AAE uses two autoencoders to map the two modal data into two low-dimensional manifolds, forcing the two spaces as unified as possible with the adversarial loss and latent cycle-consistency loss.

□ SpaceX: Gene Co-expression Network Estimation for Spatial Transcriptomics
>> https://www.biorxiv.org/content/10.1101/2021.12.24.474059v1.full.pdf
SpaceX (spatially dependent gene co-expression network) employs a Bayesian model to infer spatially varying co-expression networks via incorporation of spatial information in determining network topology.
SpaceX uses an over-dispersed spatial Poisson model coupled with a high-dimensional factor model to infer the shared and cluster specific co-expression networks. The probabilistic model is able to quantify the uncertainty and based on a coherent dimension reduction.
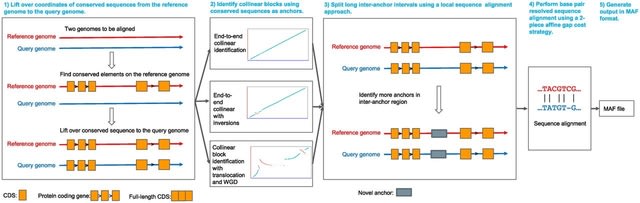
□ AnchorWave: Sensitive alignment of genomes with high sequence diversity, extensive structural polymorphism, and whole-genome duplication
>> https://www.pnas.org/content/119/1/e2113075119
AnchorWave - Anchored Wavefront alignment implements a genome duplication informed longest path algorithm to identify collinear regions and performs base pair–resolved, end-to-end alignment for collinear blocks using an efficient two-piece affine gap cost strategy.
AnchorWave improves the alignment under a number of scenarios: genomes w/ high similarity, large genomes w/ high transposable element activity, genomes w/ many inversions, and alignments b/n species w/ deeper evolutionary divergence / different whole-genome duplication histories.

□ Grandline: Network-guided supervised learning on gene expression using a graph convolutional neural network
>> https://www.biorxiv.org/content/10.1101/2021.12.27.474240v1.full.pdf
Grandline transforms PPI into a spectral domain enables convolution of neighbouring genes and pinpointing high-impact subnetworks, which allow better interpretability of deep learning models.
Grandline integrates PPI network by considering the network as an undirected graph and gene expression values as node signals. Similar to a standard conventional neural network models, the model consists of multiple blocks for convolution and pooling layer.
Grandline could identify subnetworks that are important for the phenotype prediction using Grad-CAM technique. Grandline defines a spectral graph convolution on the Fourier domain and then defined a convolutional filter based on Chebychev polynomial.
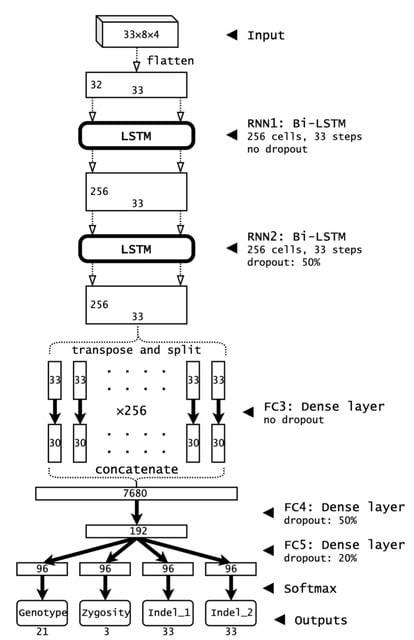
□ Clair3: Symphonizing pileup and full-alignment for deep learning-based long-read variant calling
>> https://www.biorxiv.org/content/10.1101/2021.12.29.474431v1.full.pdf
Clair3 is the 3rd generation of Clair and Clairvoyante. the Clair3 method is not restricted to a certain sequencing technology. It should work particularly well in terms of both runtime and performance on noisy data.
Clair3 integrates both pileup model and full-alignment model for variant calling. While a pileup model determines the result of a majority of variant candidates, candidates with uncertain results are further processed with a more intensive haplotype-resolved full-alignment model.

□ scGET: Predicting Cell Fate Transition During Early Embryonic Development by Single-cell Graph Entropy
>> https://www.sciencedirect.com/science/article/pii/S1672022921002539
scGET accurately predicts all the impending cell fate transitions. scGET provides a new way to analyze the scRNA-seq data and helps to track the dynamics of biological systems from the perspectives of network entropy.
The Single-Cell Graph Entropy (SGE) value quantitatively characterizes the stability and criticality of gene regulatory networks among cell populations and thus can be employed to detect the critical signal of cell fate or lineage commitment at the single-cell level.
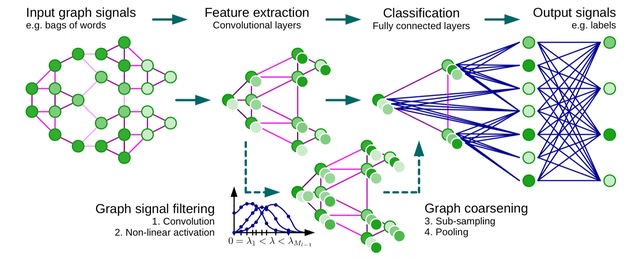
□ GLRP: Stability of feature selection utilizing Graph Convolutional Neural Network and Layer-wise Relevance Propagation
>> https://www.biorxiv.org/content/10.1101/2021.12.26.474194v1.full.pdf
a graph convolutional layer of GCNN as a Keras layer so that the SHAP (SHapley Additive exPlanation) explanation method could be also applied to a Keras version of a GCNN model.
GCNN+LRP shows the highest stability among other feature selection methods including GCNN+SHAP. a GLRP subnetwork of an individual patient is on average substantially more connected (and interpretable) than a GCNN+SHAP subnetwork, which consists mainly of single vertices.
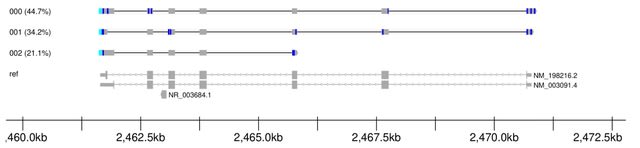
□ isoformant: A visual toolkit for reference-free long-read isoform analysis at single-read resolution
>> https://www.biorxiv.org/content/10.1101/2021.12.17.457386v1.full.pdf
isoformant, an alternative approach that derives isoforms by generating consensus sequences from long reads clustered on k-mer density without the requirement for a reference genome or prior annotations.
isoformant was developed based on the concept that an individual long-read isoform can be uniquely identified by its constituent k-mer composition. For an appropriate length k, each unique read in a mixture can be represented by a correspondingly unique k-mer frequency vector.
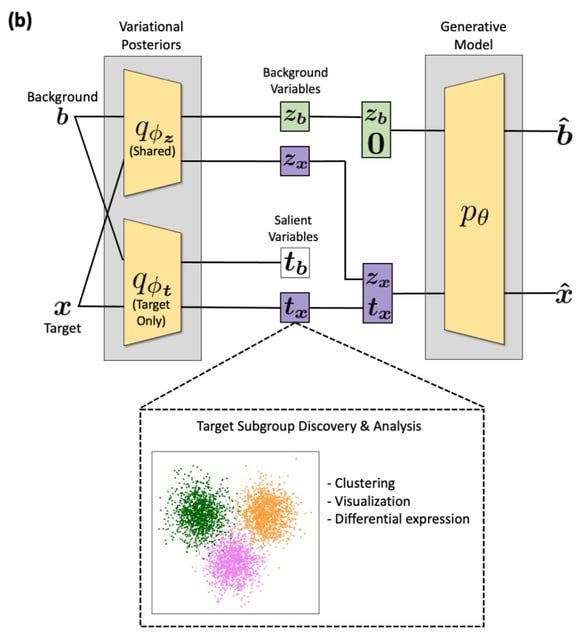
□ contrastiveVI: Isolating salient variations of interest in single-cell transcriptomic data with contrastiveVI
>> https://www.biorxiv.org/content/10.1101/2021.12.21.473757v1.full.pdf
contrastiveVI learns latent representations that recover known subgroups of target data points better than previous methods and finds differentially expressed genes that agree with known ground truths.
contrastiveVI encodes each cell as the parameters of a distribution in a low-dimensional latent space. Only target data points are given salient latent variable values; background data points are instead assigned a zero vector for these variables to represent their absence.
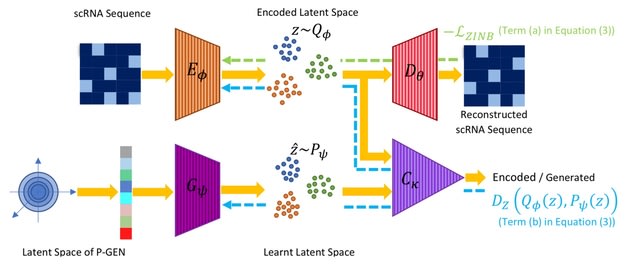
□ scRAE: Deterministic Regularized Autoencoders with Flexible Priors for Clustering Single-cell Gene Expression Data
>> https://arxiv.org/pdf/2107.07709.pdf
There is a bias-variance trade-off with the imposition of any prior on the latent space in the finite data regime.
scRAE is a generative AE for single-cell RNA sequencing data, which can potentially operate at different points of the bias-variance curve.
scRAE consists of deterministic AE with a flexibly learnable prior generator network, which is jointly trained with the AE. This facilitates scRAE to trade-off better between the bias and variance in the latent space.
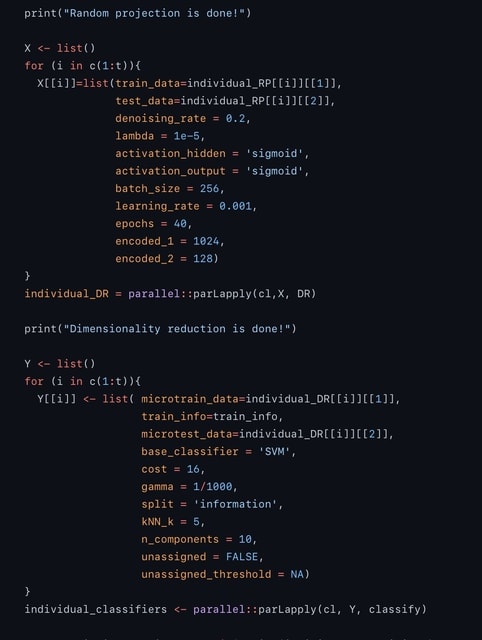
□ scIAE: an integrative autoencoder-based ensemble classification framework for single-cell RNA-seq data
>> https://academic.oup.com/bib/advance-article/doi/10.1093/bib/bbab508/6463428
scIAE, an integrative autoencoder-based ensemble classification framework, to firstly perform multiple random projections and apply integrative and devisable autoencoders (integrating stacked, denoising and sparse autoencoders) to obtain compressed representations.
Then base classifiers are built on the lower-dimensional representations and the predictions from all base models are integrated. The comparison of scIAE and common feature extraction methods shows that scIAE is effective and robust, independent of the choice of dimension, which is beneficial to subsequent cell classification.
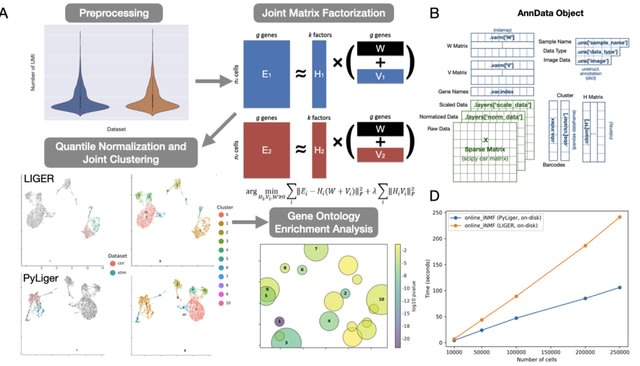
□ PyLiger: Scalable single-cell multi-omic data integration in Python
>> https://www.biorxiv.org/content/10.1101/2021.12.24.474131v1.full.pdf
LIGER is a widely-used R package for single-cell multi-omic data integration. However, many users prefer to analyze their single-cell datasets in Python, which offers an attractive syntax and highly- optimized scientific computing libraries for increased efficiency.
PyLiger offers faster performance than the previous R implementation (2-5× speedup), interoperability with AnnData format, flexible on-disk or in-memory analysis capability, and new functionality for gene ontology enrichment analysis.

□ Dynamic Suffix Array with Polylogarithmic Queries and Updates
>> https://arxiv.org/pdf/2201.01285.pdf
the first data structure that supports both suffix array queries and text updates in O(polylog n) time, achieving O(log4 n) and O(log3+o(1) n) time.
Complement the structure by a hardness result: unless the Online Matrix-Vector Multiplication (OMv) Conjecture fails, no data structure with O(polylog n)-time suffix array queries can support the “copy-paste” operation in O(n1−ε) time for any ε > 0.
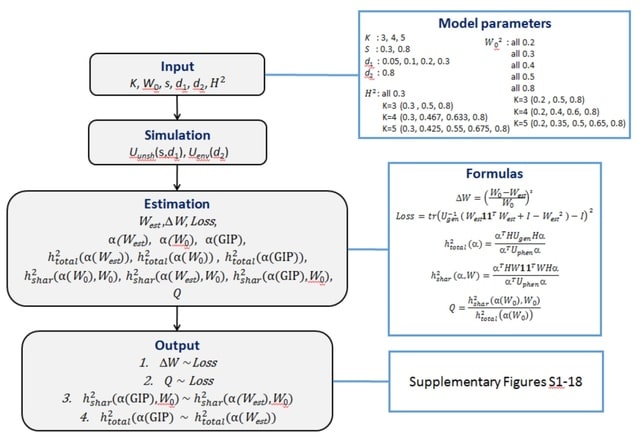
□ SHAHER: A novel framework for analysis of the shared genetic background of correlated traits
>> https://www.biorxiv.org/content/10.1101/2021.12.13.472525v1.full.pdf
SHAHER is versatile and applicable to summary statistics from GWASs with arbitrary sample sizes and sample overlaps, allows incorporation of different GWAS models (Cox, linear and logistic) and is computationally fast.
SHAHER is based on the construction of a linear combination of traits by maximizing the proportion of its genetic variance explained by the shared genetic factors. SHAHER requires only full GWAS summary statistics and matrices of genetic and phenotypic correlations.
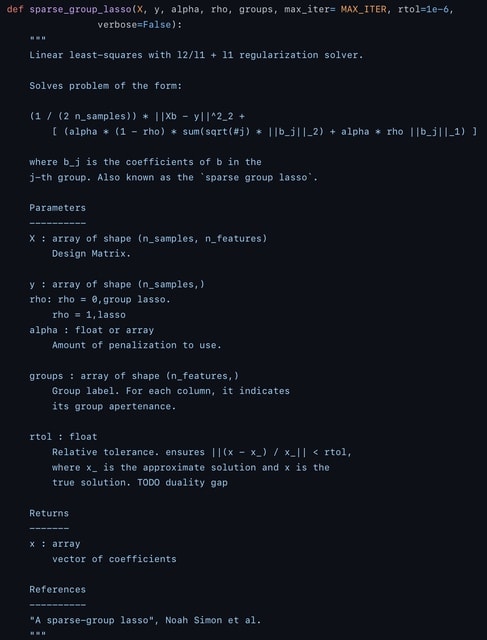
□ Stacked-SGL: Overcoming the inadaptability of sparse group lasso for data with various group structures by stacking
>> https://academic.oup.com/bioinformatics/advance-article/doi/10.1093/bioinformatics/btab848/6462433
Sparse group lasso has a mixing parameter representing the ratio of lasso to group lasso, thus providing a compromise between selecting a subset of sparse feature groups and introducing sparsity within each group.
Stacked SGL satisfies the criteria of prediction, stability and selection based on sparse group lasso penalty by stacking. stacked SGL weakens feature selection, because it selects a feature if and only if the meta learner selects the base learner that selects that feature.
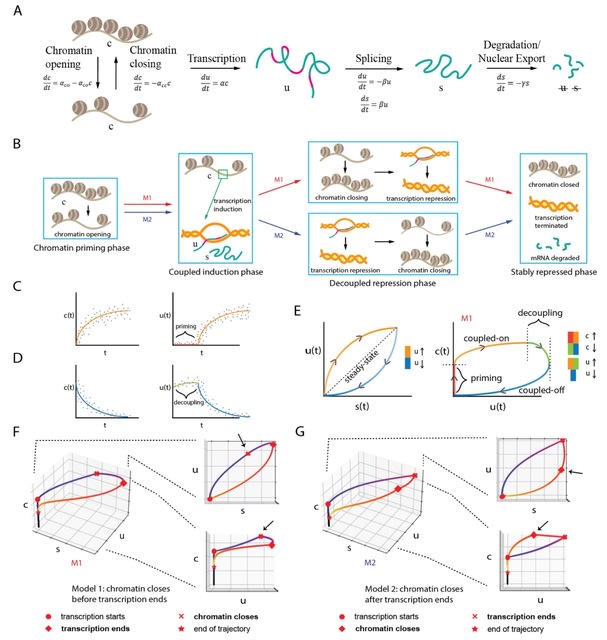
□ MultiVelo: Single-cell multi-omic velocity infers dynamic and decoupled gene regulation
>> https://www.biorxiv.org/content/10.1101/2021.12.13.472472v1.full.pdf
MultiVelo uses a probabilistic latent variable model to estimate the switch time and rate parameters of gene regulation, providing a quantitative summary of the temporal relationship between epigenomic and transcriptomic changes.
MultiVelo accurately recovers cell lineages and quantifies the length of priming and decoupling intervals in which chromatin accessibility and gene expression are temporarily out of sync.
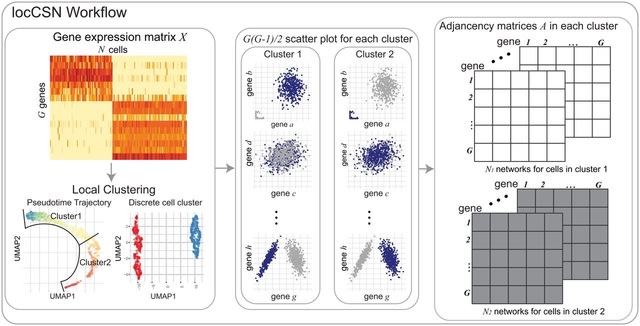
□ LocCSN: Constructing local cell-specific networks from single-cell data
>> https://www.pnas.org/content/118/51/e2113178118
locCSN, that estimates cell-specific networks (CSNs) for each cell, preserving information about cellular heterogeneity that is lost with other approaches.
LocCSN is based on a nonparametric investigation of the joint distribution of gene expression; hence it can readily detect nonlinear correlations, and it is more robust to distributional challenges.
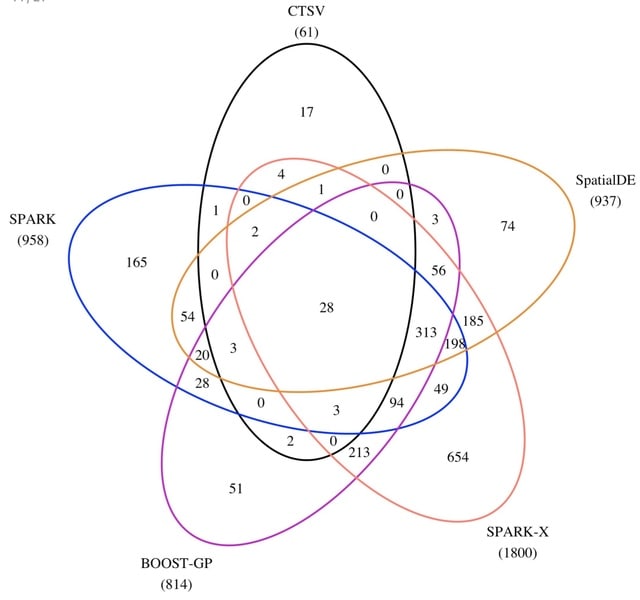
□ CTSV: Identification of Cell-Type-Specific Spatially Variable Genes Accounting for Excess Zeros
>> https://www.biorxiv.org/content/10.1101/2021.12.27.474316v1.full.pdf
CTSV can achieve more power than SPARK-X in detecting cell-type-specific SV genes and also outperforms other methods at the aggregated level.
CTSV directly models spatial raw count data and considers zero-inflation as well as overdispersion using a zero-inflated negative binomial distribution. It then incorporates cell-type proportions and spatial effect functions in the zero-inflated negative binomial regression framework.
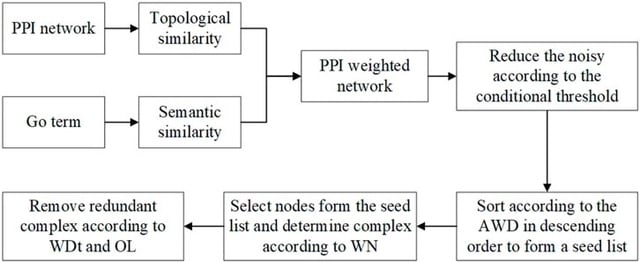
□ TSSN: A New Method for Recognizing Protein Complexes Based on Protein Interaction Networks and GO Terms
>> https://www.frontiersin.org/articles/10.3389/fgene.2021.792265/full
Topology and Semantic Similarity Network (TSSN) can filter the noise of PPI data. TSSN uses a new algorithm, called Neighbor Nodes of Proteins (NNP), for recognizing protein complexes by considering their topology information.
TSSN computes the edge aggregation coefficient as the topology characteristics of N, makes use of the GO annotation as the biological characteristics of N, and then constructs a weighted network. NNP identifies protein complexes based on this weighted network.
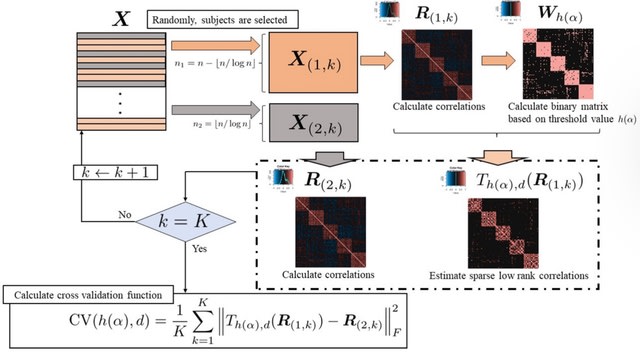
□ Thresholding Approach for Low-Rank Correlation Matrix based on MM algorithm
>> https://www.biorxiv.org/content/10.1101/2021.12.28.474401v1.full.pdf
Low-rank approximation is a very useful approach for interpreting the features of a correlation matrix; however, a low-rank approximation may result in estimation far from zero even if the corresponding original value was far from zero.
Estimating a sparse low-rank correlation matrix based on threshold values combined with cross-validation. the MM algorithm was used to estimate the sparse low-rank correlation matrix, and a grid search was performed to select the threshold values related to sparse estimation.

□ Pairs and Pairix: a file format and a tool for efficient storage and retrieval for Hi-C read pairs
>> https://academic.oup.com/bioinformatics/advance-article-abstract/doi/10.1093/bioinformatics/btab870/6493233
Pairs, a block-compressed text file format for storing paired genomic coordinates from Hi-C data, and Pairix, is a stand-alone C program that was written on top of tabix as a tool for the 4DN-standard pairs file format describing Hi-C data.
However, Pairix can be used as a generic tool for indexing and querying any bgzipped text file containing genomic coordinates, for either 2D- or 1D- indexing and querying.
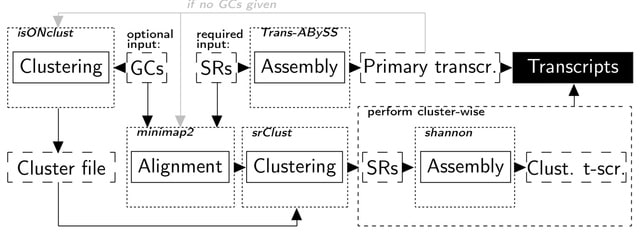
□ ClusTrast: a short read de novo transcript isoform assembler guided by clustered contigs
>> https://www.biorxiv.org/content/10.1101/2022.01.02.473666v1.full.pdf
ClusTrast, the de novo transcript isoform assembler which clusters a set of guiding contigs by similarity, aligns short reads to the guiding contigs, and assembles each clustered set of short reads individually.
ClusTrast combines two assembly methods: Trans-ABySS and Shannon, and incorporates a novel approach to clustering and cluster-wise assembly of short reads. The final step of ClusTrast is to merge the cluster-wise assemblies with the primary assembly by concatenation.

□ TIPars: Robust expansion of phylogeny for fast-growing genome sequence data
>> https://www.biorxiv.org/content/10.1101/2021.12.30.474610v1.full.pdf
TIPars, an algorithm which inserts sequences into a reference phylogeny based on parsimony criterion with the aids of a full multiple sequence alignment of taxa and pre-computed ancestral sequences.
TIPars searches the position for insertion by calculating the triplet-based minimal substitution score for the query sequence on all branches. TIPars showed promising taxa placement and insertion accuracy in the phylogenies with homogenous and divergent sequences.
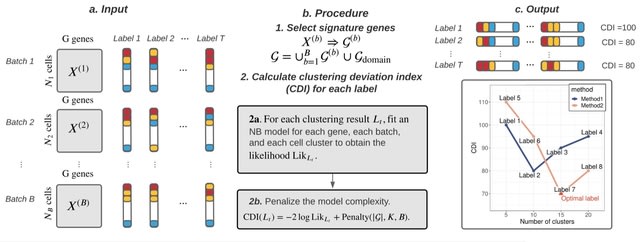
□ Clustering Deviation Index (CDI): A robust and accurate unsupervised measure for evaluating scRNA-seq data clustering
>> https://www.biorxiv.org/content/10.1101/2022.01.03.474840v1.full.pdf
Clustering Deviation Index (CDI) that measures the deviation of any clustering label set from the observed single-cell data. CDI is an unsupervised evaluation index whose calculation does not rely on the actual unobserved label set.
CDI calculates the negative penalized maximum log-likelihood of the selected feature genes based on the candidate label set. CDI also informs the optimal tuning parameters for any given clustering method and the correct number of cluster components.
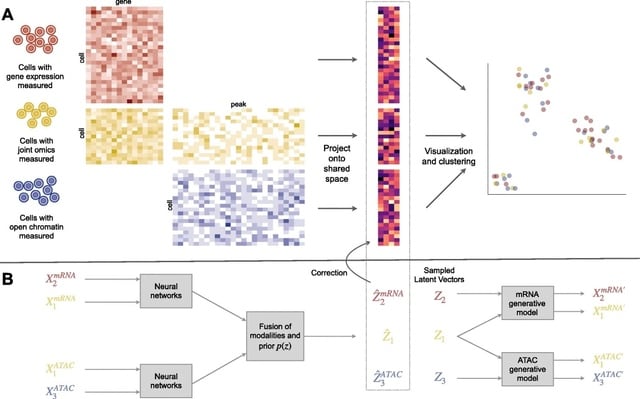
□ Cobolt: integrative analysis of multimodal single-cell sequencing data
>> https://genomebiology.biomedcentral.com/articles/10.1186/s13059-021-02556-z
Cobolt, a novel method that not only allows for analyzing the data from joint-modality platforms, but provides a coherent framework for the integration of multiple datasets measured on different modalities.
Cobolt’s generative model for a single modality i starts by assuming that the counts measured on a cell are the mixture of the counts from different latent categories.
Cobolt estimates this joint representation via a novel application of Multimodal Variational Autoencoder (MVAE) to a hierarchical generative model. Cobolt results in an estimate of the latent variable for each cell, which is a vector in a K-dimensional space.
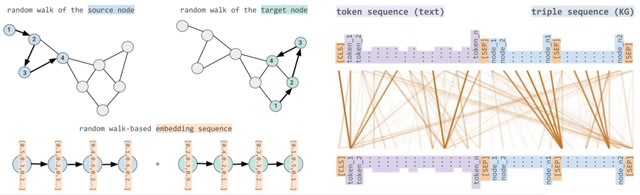
□ STonKGs: A Sophisticated Transformer Trained on Biomedical Text and Knowledge Graphs
>> https://academic.oup.com/bioinformatics/advance-article/doi/10.1093/bioinformatics/btac001/6497782
In order to exploit the information contained in KGs through machine learning algorithms, numerous KG embedding models have been developed to encode the entities and relations of KGs in a higher dimensional vector space while attempting to retain their structural properties.
STonKGs uses combined input sequences of structured information from KGs and unstructured text data from biomedical literature assembled by Integrated Network and Dynamical Reasoning Assembler (INDRA) to learn joint representations in a shared embedding space.

□ am: Implementation of a practical Markov chain Monte Carlo sampling algorithm in PyBioNetFit
>> https://academic.oup.com/bioinformatics/advance-article-abstract/doi/10.1093/bioinformatics/btac004/6497784
the implementation of a practical MCMC method in the open-source software package PyBioNetFit (PyBNF), which is designed to support parameterization of mathematical models for biological systems.
am, the new MCMC method that incorporates an adaptive move proposal distribution. Sampling can be initiated at a specified location in parameter space and with a multivariate Gaussian proposal distribution defined initially by a specified covariance matrix.
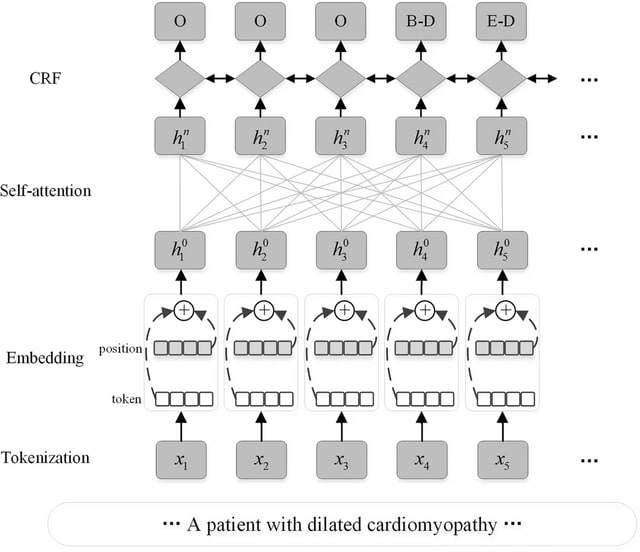
□ Hierarchical shared transfer learning for biomedical named entity recognition
>> https://bmcbioinformatics.biomedcentral.com/articles/10.1186/s12859-021-04551-4
the hierarchical shared transfer learning, which combines multi-task learning and fine-tuning, and realizes the multi-level information fusion between the underlying entity features and the upper data features.
The model uses XLNet based on Self-Attention PLM to replace BERT as encoder, avoiding the problem of input noise from autoencoding language model. When fine-tuning the BioNER task, it decodes the output of the XLNet model with Conditional Random Field decoder.
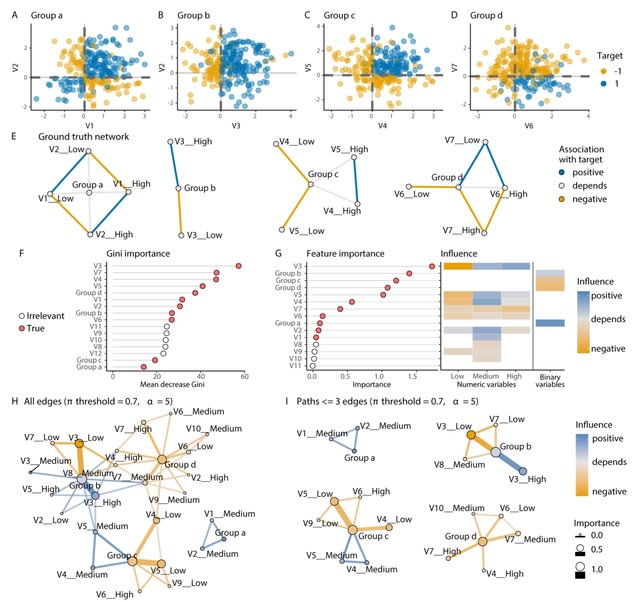
□ endoR: Interpreting tree ensemble machine learning models
>> https://www.biorxiv.org/content/10.1101/2022.01.03.474763v1.full.pdf
endoR simplifies the fitted model into a decision ensemble from which it then extracts information on the importance of individual features and their pairwise interactions and also visualizes these data as an interpretable network.
endoR infers true associations with comparable accuracy than other commonly used approaches while easing and enhancing model interpretation. Adjustable regularization and bootstrapping help reduce the complexity and ensure that only essential parts of the model are retained.
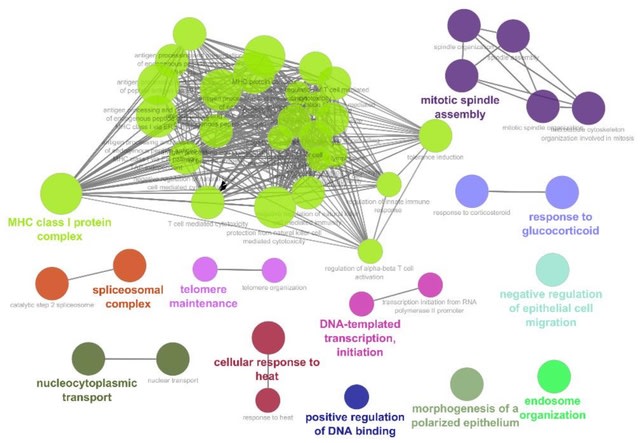
□ Nm-Nano: Predicting 2′-O-methylation (Nm) Sites in Nanopore RNA Sequencing Data
>> https://www.biorxiv.org/content/10.1101/2022.01.03.473214v1.full.pdf
Nm-Nano framework integrates two supervised machine learning models for predicting Nm sites in Nanopore sequencing data, namely Xgboost and Random Forest (RF).
Each model is trained with set of features that are extracted from the raw signal generated by the Oxford Nanopore MinION device, as well as the corresponding basecalled k-mer resulting from inferring the RNA sequence reads from the generated Nanopore signals.
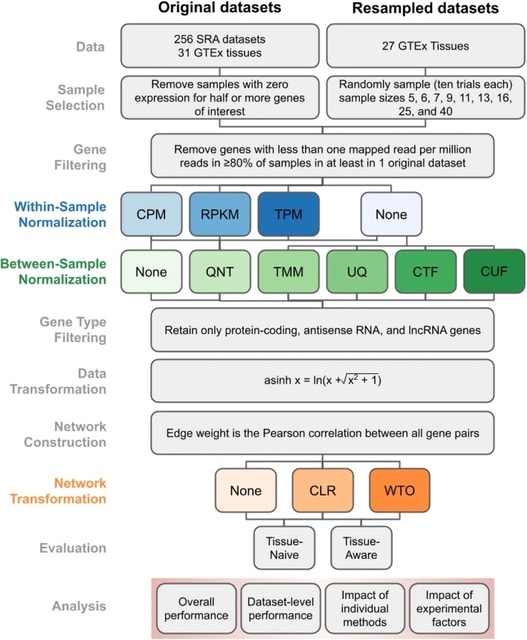
□ Robust normalization and transformation techniques for constructing gene coexpression networks from RNA-seq data
>> https://genomebiology.biomedcentral.com/articles/10.1186/s13059-021-02568-9
a comprehensive benchmarking and analysis of 36 different workflows, each with a unique set of normalization and network transformation methods, for constructing coexpression networks from RNA-seq datasets.
Between-sample normalization has the biggest impact, with counts adjusted by size factors producing networks that most accurately recapitulate known tissue-naive and tissue-aware gene functional relationships.
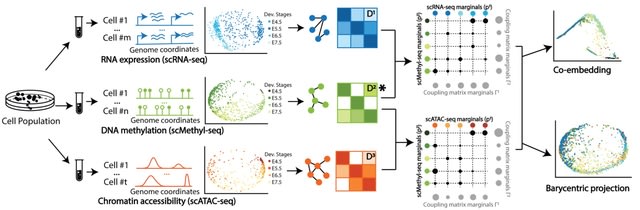
□ SCOT: Single-Cell Multiomics Integration
>> https://www.liebertpub.com/doi/full/10.1089/cmb.2021.0477
Single-cell alignment using optimal transport (SCOT) is an unsupervised algorithm that addresses this limitation by using optimal transport to align single-cell multiomics data.
the Gromov-Wasserstein distance in the algorithm can guide SCOT's hyperparameter tuning in a fully unsupervised setting when no orthogonal alignment information is available.
SCOT finds a probabilistic coupling matrix that minimizes the discrepancy between the intra-domain distance matrices. Finally, it uses the coupling matrix to project one single-cell data set onto another through barycentric projection.
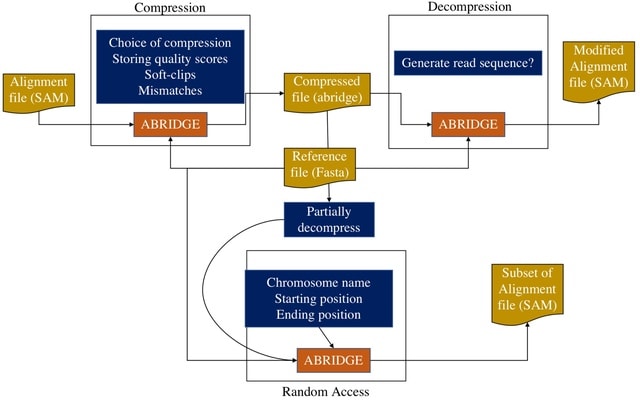
□ ABRIDGE: An ultra-compression software for SAM alignment files
>> https://www.biorxiv.org/content/10.1101/2022.01.04.474935v1.full.pdf
ABRIDGE, an ultra-compressor for SAM files offering users both lossless and lossy compression options. This reference-based file compressor achieves the best compression ratio among all compression software ensuring lower space demand and faster file transmission.
ABRIDGE accepts a single SAM file as input and returns a compressed file that occupies less space than its BAM or CRAM counterpart. ABRIDGE compresses alignments after retaining only non-redundant information.
ABRIDGE accumulates all reads that are mapped onto the same nucleotide on a reference. ABRIDGE modifies the traditional CIGAR string to store soft-clips, mismatches, insertions, deletions, and quality scores thereby removing the need to store the MD string.