
(Created with Midjourney v6.0 ALPHA)

□ nanoranger: long-read sequencing-based genotyping of single cell RNA profiles
>> https://www.nature.com/articles/s41467-023-44137-7
nanoranger, a versatile workflow that enables the amplification, long-read sequencing, and processing of targets of interest using the ONT platform such that a wide range of natural barcodes, including somatic and mtDNA mutations, fusion genes and isoforms can be detected.
nanoranger originates from single cell cDNA libraries that are whole-transcriptome amplified “intermediate libraries”. After extraction of subreads, cell barcodes are identified and TCR information is processed or transcripts are genome-aligned for downstream genotyping.

□ Comprehensive and accurate genome analysis at scale using DRAGEN accelerated algorithms
>> https://www.biorxiv.org/content/10.1101/2024.01.02.573821v1
A new developments of Dynamic Read Analysis for GENomics (DRAGEN) and its optimization in SNV and indel calling as well as its ability to detect the entire landscape of variations - CNV, SV, repeat expansions, specialized methodologies for certain regions.
The accuracy of DRAGEN is boosted by the first multigenome (graph) implementation that scales and enables the detection of variant types beyond just SNV. The DRAGEN Iterative gVCF Genotyper (IGG) can efficiently aggregate hundreds of thousands to millions of gVCFs.

□ CellHint: Automatic cell-type harmonization and integration across Human Cell Atlas datasets
>> https://www.cell.com/cell/fulltext/S0092-8674(23)01312-0
CellHint, a predictive clustering tree (PCT)-based tool to efficiently align multiple datasets by assessing their cell-cell similarities and harmonizing cell annotations.
CellHint defines semantic relationships among cell types and captures their underlying biological hierarchies, which are further leveraged to guide the downstream data integration at different levels of annotation granularity.
CellHint derives a global distance matrix representing the inferred dissimilarities between all cells and cell types. CellHint is able to produce batch-insensitive dissimilarity measures, enabling a robust cross-dataset meta-analysis.
CellHint defines two levels of novelties for cell types: unmatched cell types (“NONE”), which cannot align with any cell type from the other datasets, and unharmonized cell types (“UNRESOLVED”), which fail to integrate into the harmonization graph after the final iteration.

□ TRGT: Characterization and visualization of tandem repeats at genome scale
>> https://www.nature.com/articles/s41587-023-02057-3
Tandem Repeat Genotyping Tool (TRGT) determines the consensus sequences and methylation levels of specified TRs from PacBio HiFi sequencing data. It reports reads that support each repeat allele. These reads can be subsequently visualized with a companion TR visualization tool.
Assessing 937,122 TRs, TRGT showed a Mendelian concordance of 98.38%, allowing a single repeat unit difference. TRGT detected all expansions while also identifying methylation signals and mosaicism and providing finer repeat length resolution.

□ EnhancerTracker: Comparing cell-type-specific enhancer activity of DNA sequence triplets via an ensemble of deep convolutional neural networks
>> https://www.biorxiv.org/content/10.1101/2023.12.23.573198v1
EnhancerTracker utilizes an ensemble of deep artificial neural networks; particularly depthwise separable convolutional networks in measuring an enhancer-enhancer similarity metric.
Enhancer Tracker is trained to classify triplets of sequences that have similar enhancer activities versus triplets of sequences that have dissimilar enhancer activities. EnhancerTracker can compare sequences in a triplet regardless of where they are active.
A separable-convolutional layer learns patterns in each sequence separately. Similar triplets are given a label of 1 and dissimilar triplets are given a label of 0. The classifier takes three sequences — represented as a three-channel tensor.
EnhancerTracker consists of a masking layer followed by four blocks of layers, each of which includes a separable-convolutional layer, a batch-normalization layer, and a max-pooling layer. The output layer of the classifier is a dense layer with sigmoid activation function.

□ Rewriting regulatory DNA to dissect and reprogram gene expression
>> https://www.biorxiv.org/content/10.1101/2023.12.20.572268v1
An experimental method to measure the quantitative effects of hundreds of designed edits to endogenous regulatory DNA directly on gene expression.
This method combines pooled prime editing-in which we introduce many programmed insertions or deletions into a population of cells—with RNA fluorescence in situ hybridization (RNA FISH) and flow sorting (Variant-FlowFISH), to directly measure effects on gene expression.
A mathematical approach (Variant-EFFECTS: Variant-Estimation For Flow-sorting Effects in CRISPR Tiling Screens) is developed to estimate the quantitative effect of each edit based on these frequency measurements, considering editing efficiency and cell ploidy.
Variant-EFFECTS infers the effects of edits on gene expression by adjusting their maximum likelihood estimation procedure to account for a distribution of genotypes.

□ BulkLMM: Real-time genome scans for multiple quantitative traits using linear mixed models
>> https://www.biorxiv.org/content/10.1101/2023.12.20.572698v1
BulkLMM uses vectorized, multi-threaded operations and regularization to improve optimization, and numerical approximations to speed up the computations using the Julia language.
Bulkscan-Null-Grid, makes additional relaxation on the accuracy required for the results by estimating the heritability of each trait approximately on a grid of finite candidate values
Bulkscan-Alt-Grid, combines the ideas of the grid-search approach for estimating the heritability and the matrix multiplication approach for efficiently computing LOD scores.

□ scDMV: A Zero-one Inflated Beta Mixture Model for DNA Methylation Variability with scBS-Seq Data
>> https://academic.oup.com/bioinformatics/advance-article/doi/10.1093/bioinformatics/btad772/7492658
scDMV is a statistical method applied to single-cell bisulfite sequencing data(scBS-seq data) to detect differentially methylated regions of DNA.
scDMV is based on a 0-1 inflated beta binomial distribution model, using the Wald test to calculate p-values for each region in scBS-seq data to identify differentially methylated regions.

□ GeNNius: An ultrafast drug-target interaction inference method based on graph neural networks
>> https://academic.oup.com/bioinformatics/advance-article/doi/10.1093/bioinformatics/btad774/7491592
GeNNius (Graph Embedding Neural Network Interaction Uncovering System), a novel DTI prediction method, built upon SAGEConv layers followed by a neural network (NN)-based classifier.
GeNNius reveals that the GNN encoder maintains biological information after the graph convolutions while diffusing this information through nodes, eventually distinguishing protein families in the node embeddings.

□ SOHPIE: Statistical Approach via Pseudo-Value Information and Estimation for Differential Network Analysis of Microbiome Data
>> https://academic.oup.com/bioinformatics/advance-article/doi/10.1093/bioinformatics/btad766/7491589
SOHPIE implements a suite of functions facilitating differential network analysis of finding differentially connected (DC) taxa between two heterogeneous groups.
The key features are the ability to appropriately to test for differential connectivity of a co-abundance network and also to adjust for covariates by introducing a pseudo-value regression framework.
The Jackknife-generated pseudo response values for regression reflect the influence of the i-th sample on the centrality of each taxon. The regression model describes the "effect" of the main factor (binary group variable) Z and covariates X on the quantified influences.
Thus, DC between two groups is described and quantified by the regression coefficient on Z, in terms of how much the grouping affect the influences on the centrality, adjusting for other covariates.

□ Coracle: A Machine Learning Framework to Identify Bacteria Associated with Continuous Variables
>> https://academic.oup.com/bioinformatics/advance-article/doi/10.1093/bioinformatics/btad749/7484655
Coracle is an Artificial Intelligence (Al) framework that uses an ensemble approach of prominent feature selection methods and machine learning (ML) models to identify associations between bacterial communities and continuous variables.
Coracle can identify bacterial taxa that are predictive of phenotypic trait or environmental condition performance, and thus provide a means to align host biology or the prevailing environment with microbiome assemblage.
Coracle is not restricted to microbial community data matrices but can process other types of high-dimensional data, such as gene expression matrices, in association with a continuous variable. Importantly, Coracle can only account for association and not for causation.

□ FlowAtlas.jl: an interactive tool bridging FlowJo with computational tools in Julia
>> https://www.biorxiv.org/content/10.1101/2023.12.21.572741v1
FlowAtlas, an open source, fully graphical, interactive high-dimensional data exploration tool. FlowAtlas links the familiar Flow Jo workflow with a high-performance machine learning framework enabling rapid computation of millions of high-dimensional events.
FlowAtlas parses user-defined individual channel transformation settings from FlowJo as well as channel, gate and sample group names, ensuring optimal embedding geometry. The resulting embedding is highly interactive, offering zooming to explore deeper cluster structures.

□ SCRIPro: Single-cell and spatial multiomic inference of gene regulatory networks
>> https://www.biorxiv.org/content/10.1101/2023.12.21.572934v1
SCRIPro first employs density clustering using a high coverage SuperCell strategy. While for spatial data, SCRIPro combines gene expression and cell spatial similarity information to a latent low-dimension embeddings via a graph attention auto-encoder.
SCRIPro conducts in silico deletion analyses, utilizing matched scATAC-seq or reconstructed chromatin landscapes from public chromatin accessibility data, to assess the regulatory significance of TRs by RP model in each SuperCell.
SCRIPro combines TR expression and TR to generate TR-centered GRNs at the SuperCell resolution. The output of SCRIPro can be applied for TR target clustering, temporal GRN trajectory and spatial GRN trajectory.

□ OmniClustifyXMBD: Uncover putative cell states within multiple single-cell omics datasets
>> https://www.biorxiv.org/content/10.1101/2023.12.22.573159v1
OmniClustifyXMBD combines adaptive signal isolation with deep variational Gaussian-mixture clus-tering. This involves iterative process aimed at estimating and attenuating residual variations linked to distinct factors in the remaining data.
OmniClustify XMBD is meticulously designed to isolate the multifaceted influences stemming from diverse factors acting upon individual cells. Once these influences are effectively isolated, the remaining gene expression signals encapsulate the inherent cell states.
The second component is strategically engineered to execute the clustering of cells predicted on these refined gene expression signals. Notably, these components are seamlessly interwoven within the framework of deep random-effects modeling.

□ CellularPotts.jl: Simulating Multiscale Cellular Models in Julia
>> https://academic.oup.com/bioinformatics/advance-article/doi/10.1093/bioinformatics/btad773/7491591
CellularPotts.jl is a Julia package designed to simulate behaviors observed in biological cells like division and adhesion. Users of this package can create 2D and 3D environments with any number of cell types, sizes, and behaviors.
CPMs operate on a discretized space and over discrete time intervals which make them difficult to combine with continuous time models like systems of ordinary differential equations (ODEs).
CellularPotts.jl only saves how the model changes over time as opposed to a full copy of the model at each timepoint.

□ The BioGenome Portal: a web-based platform for biodiversity genomics data management
>> https://www.biorxiv.org/content/10.1101/2023.12.20.572408v1
The BioGenome Portal (BGP), a platform that tracks, integrates and manages the data generated under a given biodiversity genomics project (not necessarily an Earth Biogenome Project node).
The portal generates sequence status reports that can be eventually ingested by designated meta-data tracking systems, facilitating the coordination task of these systems.
The BGP helps in the coordination among the groups within the same project and, by generating a GoaT compliant sequencing status report, contributes to keep the sequencing status of the EBP up to date.

□ KAGE 2: Fast and accurate genotyping of structural variation using pangenomes
>> https://www.biorxiv.org/content/10.1101/2023.12.23.572333v1
KAGE2, a genotyper that is able to efficiently and accurately genotype structural variation from short reads by using a pangenome representation of a population.
KAGE2 employs an improved strategy for picking kmers to represent variants, which is needed since structural variants are often multiallelic and contain repetitive sequence.

□ Semi-supervised learning with pseudo-labeling for regulatory sequence prediction
>> https://www.biorxiv.org/content/10.1101/2023.12.21.572780v1
A novel semi-supervised learning (SSL) method based on cross-species pseudo-labeling, which greatly augments the size of the available labeled data for learning. The method consists in remapping regulatory sequences from a labeled genome to other closely related genomes.
Pseudo-labeled data allows to pretrain a neural network from multiple orders of magnitude larger data than labeled data. After pretraing with pseudo-labeled data, the model is then fine-tuned on the original labeled data.
The proposed SSL was used to train multiple state-of-the-art models, including DeepBind, DeepSea and DNABERT2, and showed sequence classification accuracy improvement in many cases.

□ Characterizing uncertainty in predictions of genomic sequence-to-activity models
>> https://www.biorxiv.org/content/10.1101/2023.12.21.572730v1
Analyzing uncertainty in the predictions of genomic sequence-to-activity models by measuring prediction consistency across Basenji2 models, when applied to reference genome sequences, reference genome sequences perturbed with TF motifs, eQTLs, and personal genome sequences.
For sequences that require models to generalize to out-of-distribution regulatory variation - eQTLs and personal genome sequences - predictions show high replicate inconsistency. Surprisingly, consistent predictions for both reference and variant sequences are often incorrect.

□ Perturbation Analysis of Markov Chain Monte Carlo for Graphical Models
>> https://arxiv.org/abs/2312.14246
The basic question in perturbation analysis of Markov chains is: how do small changes in the transition kernels of Markov chains translate to chains in their stationary distributions?
Much larger errors, up to size roughly the square root of the convergence rate, are permissible for many target distributions associated with graphical models.
The main motivation for this work comes from computational statistics, where there is often a tradeoff between the per-step error and per-step cost of approximate MCMC algorithms.

□ FunctanSNP: an R package for functional analysis of dense SNP data (with interactions)
>> https://academic.oup.com/bioinformatics/article/39/12/btad741/7461185
FunctanSNP, the first portable and friendly package that takes a functional perspective and analyzes densely measured SNP data (without and with interac-tions) along with scalar covariates.
FunctanSNP requires basic R settings, can be easily installed and utilized, and exhibits satisfactory performance. Beyond SNP data, it is also applicable to other densely measured data types and can be extended to other types of outcomes and models.

□ Deconer: A comprehensive and systematic evaluation toolkit for reference-based cell type deconvolution algorithms using gene expression data
>> https://www.biorxiv.org/content/10.1101/2023.12.24.573278v1
Deconer (Deconvolution Evaluator) facilitates the systematic comparisons. Deconer incorporates numerous simulation data generation methods based on both bulk and single-cell gene expression data, as well as a wide range of evaluation metrics and visualization tools.
Deconer integrates a variety of evaluation metrics and plotting programs. Furthermore, it offers several evaluation functions, such as stability testing of the model under simulated noise conditions, and accuracy analysis of rare component deconvolution.

□ alignmentFilter: A comprehensive alignment-filtering methodology improves phylogeny particularly by filtering overly divergent segments
>> https://www.biorxiv.org/content/10.1101/2023.12.26.573321v1
alignmentFilter, a R package for comprehensive alignment filtration. The power of this newly developed and other prevalent alignment-filtering tools on phylogenetic inference was examined and compared based on both empirical and simulated data.
The alignment-filtering method alone can largely affect inferred phylogeny, and in most cases after alignment filtration by using alignmentFilter both the topological conflict and root-to-tip length heterogeneity are simultaneously minimized most efficiently.

□ ASCT: automatic single-cell toolbox in julia
>> https://www.biorxiv.org/content/10.1101/2023.12.27.573479v1
ASCT is an automatic single-cell toolbox for analyzing single-cell RNA-Seq data. This toolbox can analyze the output data of 10X Cellranger for quality checking, preprocessing, dimensional reduction, clustering, marker genes identification and samples integration.
ASC completely runs all functions by automatic methods without artificial intervention and can tune the parameters for advanced user. It is implemented by pure Julia language, and the overall runtime of basic steps is less than Seurat V4.

□ ADMET-AI: A machine learning ADMET platform for evaluation of large-scale chemical libraries
>> https://www.biorxiv.org/content/10.1101/2023.12.28.573531v1
ADMET-Al uses a graph neural network called Chemprop-RDKit (Figure 1), which was trained on 41 ADMET datasets from the Therapeutics Data Commons (TDC).
ADMET-Al surpasses existing ADMET prediction tools in terms of speed and accuracy. Moreover, it provides additional useful features such as local batch prediction and contextualized ADMET predictions using a reference set of approved drugs.
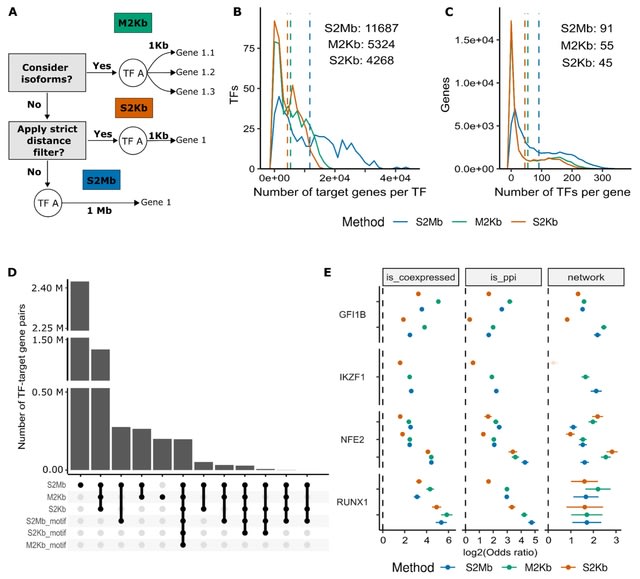
□ Specifying cellular context of transcription factor regulons for exploring context-specific gene regulation programs
>> https://www.biorxiv.org/content/10.1101/2023.12.31.573765v1
A straightforward method to define regulons that capture the cell-specific aspects of both TF binding and target gene expression. This approach uses data from ChIP-Seq and RNA-Seq experiments to construct regulons, and is easy to apply to any cell type with these data.
Fitting a univariate linear model to model gene expression as a function of TF regulations and estimate activities of transcription factors as regression coefficients of this model.

□ SORBET: Automated cell-neighborhood analysis of spatial transcriptomics or proteomics for interpretable sample classification via GNN
>> https://www.biorxiv.org/content/10.1101/2023.12.30.573739v1
Spatial 'Omics Reasoning for Binary labEl Tasks (SORBET), a geometric deep learning framework that infers emergent phenotypes, such as response to immunotherapy, from spatially resolved molecular profiling data.
SORBET learns phenotype-specific cell signatures, which are termed cell-niche embeddings (CNE), that synthesize the cell’s molecular profile, the molecular profiles of neighboring cells, and the local tissue architecture.

□ MHESMMR: a multilevel model for predicting the regulation of miRNAs expression by small molecules
>> https://bmcbioinformatics.biomedcentral.com/articles/10.1186/s12859-023-05629-x
MHESMMR, a computational model to predict whether the regulatory relationship between miRNAs and SMs is up-regulated or down-regulated.
MHESMMR uses the Large-scale Information Network Embedding (LINE) algorithm to construct the node features from the self-similarity networks.
MHESMMR uses the General Attributed Multiplex Heterogeneous Network Embedding (GATNE) algorithm to extract the topological information from the attribute network, and finally utilize the Light Gradient Boosting Machine algorithm to predict the regulatory relationship.

□ Sniffles2: Detection of mosaic and population-level structural variants
>> https://www.nature.com/articles/s41587-023-02024-y
Sniffles2, a redesign of Sniffles, with improved accuracy, higher speed and features that address the problem of population-scale SV calling for long reads.
Sniffles2 enables the detection of low-frequency SVs across datasets, which facilitates detection of somatic SVs and mosaicism studies and opens the field of cell heterogeneity for long-read applications.
Sniffles2 dynamically adapts clustering parameters during SV calling, allowing it to detect single SVs that have been scattered as a result of alignment artifacts.

□ Beyond benchmarking: towards predictive models of dataset-specific single-cell RNA-seq pipeline performance
>> https://www.biorxiv.org/content/10.1101/2024.01.02.572650v1
Single Cell p/peline PredIctiOn (SCIPIO-86), the first dataset of single-cell pipeline performance. Investigating whether AutoML approaches may be adapted for the optimization of scRNA-seq analysis pipelines in order to recommend an analysis pipeline for a given dataset.
288 clustering pipelines were run over each dataset and the success of each was quantified with 4 unsupervised metrics. Dataset- and pipeline-specific features were then computed and given as input to supervised machine learning models to predict metric values.

□ MntJULiP and Jutils: Differential splicing analysis of RNA-seq data with covariates
>> https://www.biorxiv.org/content/10.1101/2024.01.01.573825v1
MntJULiP detects intron-level differences in alternative splicing from RNA-seq data using a Bayesian mixture model. Jutils visualizes alternative splicing variation with heatmaps, PCA and sashimi plots, and Venn diagrams.
MntJULiP can detect both differences in the introns' splicing ratios (DSR), and changes in the abundance level of introns (DSA), and thus can capture alternative splicing variations in a comprehensive way.

□ ReUseData: an R/Bioconductor tool for reusable and reproducible genomic data management
>> https://bmcbioinformatics.biomedcentral.com/articles/10.1186/s12859-023-05626-0
ReUseData provides an easy-to-use R approach for the management of all reusable data, including both laboratory-specific experiment data and the curation of publicly available genomic data resources.