
□ TXGNN: Zero-shot prediction of therapeutic use with geometric deep learning and clinician centered design
>> https://www.medrxiv.org/content/10.1101/2023.03.19.23287458v1
TXGNN is a graph neural network pre-trained on a comprehensive knowledge graph of 17,080 clinically-recognized diseases and 7,957 therapeutic candidates. The model can process various therapeutic tasks, such as indication and contraindication prediction, in a unified formulation.
TXGNN can perform zero-shot inference on new diseases without additional parameters or fine-tuning on ground truth labels. TXGNN uses a metric learning module that operates on the latent representation space.
TXGNN transforms points in the latent space representing the candidate and disease into predictions about their relationship. In TXGNN, we obtain a disease signature vector for each disease based on the set of neighboring proteins, exposures, and other biomedical entities.
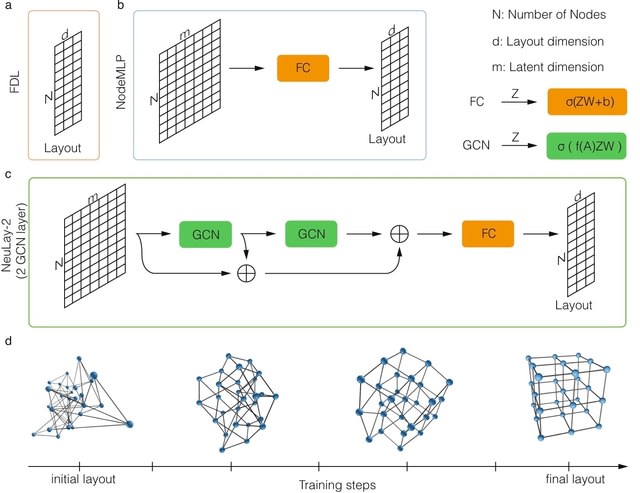
□ NeuLay: Accelerating network layouts using graph neural networks
>> https://www.nature.com/articles/s41467-023-37189-2
The NeuLay algorithm, a Graph Neural Network (GNN) developed to parameterize node features, significantly improves both the speed and the quality of graph layouts, opening up the possibility to quickly and reliably visualize large networks.
NeuLay allows for the use of different GNN architecture other than GCN, such as Graph Attention. NeuLay encodes the graph structure by graph neural networks that maps the adjacency matrix to the node positions. NeuLay-2 w/ two GCN layers has the fastest convergence of the energy.

□ Con-AAE: Contrastive Cycle Adversarial Autoencoders for Single-cell Multi-omics Alignment and Integration
>> https://academic.oup.com/bioinformatics/advance-article/doi/10.1093/bioinformatics/btad162/7091469
Con-AAE (Contrastive cycle adversarial Autoencoders), aiming at integrating and aligning the multi-omics data at the single-cell level. The contrastive loss minimizes the distance between positive pairs and maximizes the distance between negative pairs.
Con-AAE uses two autoencoders to map two modality data into two low-dimensional manifolds under the constrain of adversarial loss, trying to develop representations for each modality that are separated but cannot be identified by an adversarial network in a coordinated subspace.

□ Phenonaut; multiomics data integration for phenotypic space exploration
>> https://academic.oup.com/bioinformatics/advance-article/doi/10.1093/bioinformatics/btad143/7082955
Phenonaut is a framework for applying workflows to multi-omics data. Originally targeting high-content imaging and the exploration of phenotypic space, with different visualisations and metrics.
Phenonaut runs are accompanied by cryptographic hashes proving reported inputs. Phenonaut allows now operates in a data agnostic manner, allowing users to describe their data (multi-view/multi-omics) and apply a series of generic or specialised data-centric transforms.

□ Accurate Flow Decomposition via Robust Integer Linear Programming
>> https://www.biorxiv.org/content/10.1101/2023.03.20.533019v1
A new ILP formulation for the flow decomposition problem for dealing with edge weights not forming a flow. It enables a macroscopic management of errors by attaching an error to each solution path instead of each edge.
This formulation defines the minimum path-error flow decomposition problem as the problem of finding a set of weighted paths with associated error variables, such that the superposition difference of each edge is within the sum of the error variables of the paths using the edge.

□ multiWGCNA: an R package for deep mining gene co-expression networks in multi-trait expression data
>> https://bmcbioinformatics.biomedcentral.com/articles/10.1186/s12859-023-05233-z
multiWGCNA, a WGCNA-based procedure that can leverage the multidimensionality of experimental designs to study co-expression networks across variable conditions, such as space or time.
multiWGCNA generates a network for each condition separately, and subsequently maps these modules across designs, and performs relevant downstream analyses, incl. module-trait correlation and module preservation.

□ GVC: efficient random access compression for gene sequence variations
>> https://bmcbioinformatics.biomedcentral.com/articles/10.1186/s12859-023-05240-0
The Genomic Variant Codec(GVC), a novel approach for compressing gene sequence variations with random access capability. The genotypes are extracted from a VCF file and divided into blocks. Each block represents genotypes of all samples in a certain range of loci in a chromosome.
GVC uses two alternative binarization approaches to decompose the allele matrix into a binary representation: bit plane binarization and row binarization. GVC uses the Hamming distance to measure the similarity b/n adjacent rows/columns. Each binary matrix is entropy-encoded.

□ SoCube: an innovative end-to-end doublet detection algorithm for analyzing scRNA-seq data
>> https://academic.oup.com/bib/advance-article-abstract/doi/10.1093/bib/bbad104/7081128
Several doublet detection algorithms are currently available, but their generalization performance could be further improved due to the lack of effective feature-embedding strategies with suitable model architectures.
SoCube proposed a novel 3D composite feature-embedding strategy that embedded latent gene information and constructed a multikernel, multichannel CNN-ensembled architecture in conjunction with the feature-embedding strategy.

□ OASIS: An interpretable, finite sample valid alternative to Pearson's X2 for scientific discovery
>> https://www.biorxiv.org/content/10.1101/2023.03.16.533008v1
OASIS (Optimized Adaptive Statistic for Inferring Structure) constructs a test-statistic which is linear in the normalized data matrix, providing closed form p-value bounds through classical concentration inequalities.
OASIS computes a bilinear form of residuals. OASIS provides a decomposition of the table, lending interpretability to its rejection of the null. The finite-sample bounds correctly characterize the p-value bound derived up to a variance term.

□ AIM: A Framework for High-throughput Sequence Alignment using Real Processing-in-Memory Systems
>> https://academic.oup.com/bioinformatics/advance-article/doi/10.1093/bioinformatics/btad155/7087101
Alignment-in-Memory (AIM), a framework for PIM-based sequence alignment that targets the UPMEM system. AIM dispatches a large number of sequence pairs across different memory modules and aligns each pair using compute cores within the memory module where the pair resides.
AIM supports multiple alignment algorithms including NW, SWG, GenASM, WFA, and WFA-adaptive. Each algorithm has alternate implementations that manage the UPMEM memory hierarchy differently and are suitable for different read lengths.

□ scQA: Clustering scRNA-seq data via qualitative and quantitative analysis
>> https://www.biorxiv.org/content/10.1101/2023.03.25.534232v1
scQA (an architecture for clustering Single-Cell RNA-seq data based on Qualitative and Quantitative Analysis), which can efficiently cluster cells at various scale based on so called landmarks and each indicates the consensus of genes with similar expression patterns.
scQA constructs the consensus vector of genes whose qualitative expressions under certain cells are of similar trend: quasi-trend-preserved genes. scQA identifies distinct cell types, it proceeds to analyze the characteristics of the ID landmarks both internally / externally.

□ SpaceWalker: Interactive Gradient Exploration for Spatial Transcriptomics Data
>> https://www.biorxiv.org/content/10.1101/2023.03.20.532934v1
The intrinsic dimensionality can serve to guide the user to anatomically distinct regions, that changes in local intrinsic dimensionality in many cases mirror transitions between cell subclasses.
SpaceWalker consists of two key innovations: an interactive, real-time flood-fill and spatial projection of the local topology of the High-Dimensional space, and a gradient gene detector.
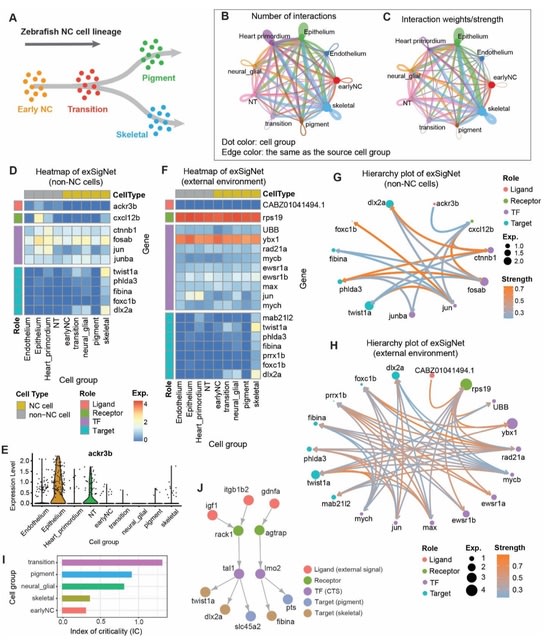
□ exFINDER: identify external communication signals using single-cell transcriptomics data
>> https://www.biorxiv.org/content/10.1101/2023.03.24.533888v1
exFINDER analyzes the exSigNet by predicting signaling strength, calculating the maximal signal flow, clustering different ligand-target signaling paths, quantifying the signaling activities using the activation index, and evaluating the GO analysis outputs of exSigNet.
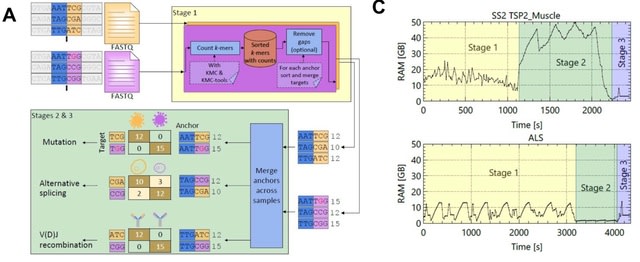
□ NOMAD2 provides ultra-efficient, scalable, and unsupervised discovery on raw sequencing reads
>> https://www.biorxiv.org/content/10.1101/2023.03.17.533189v1
NOMAD2 rapidly identifies candidate RNA editing de novo, including detecting potentially hyperedited events, filling a gap in existing bioinformatic tools. classified anchors as “mismatch” defined as cases where the two most abundant targets differ by single-base mismatches.
NOMAD2 enumerates all (a+g+t)-mers, these sequences are sorted lexicographically with KMC-tools. All occurrences of unique anchors are adjacent, which enables efficient gap removal and unique targets collapsing in the third step via a linear traversal over the (a+g+t)-mers.

□ PWN: enhanced random walk on a warped network for disease target prioritization
>> https://bmcbioinformatics.biomedcentral.com/articles/10.1186/s12859-023-05227-x
PWN (Prioritization with a Warped Network) uses the Forman–Ricci curvature instead of the Ollivier–Ricci curvature. PWN can be used for identifying the targets with properly given prior knowledge and gene scores.
PWN is designed to be an efficient variant of random walk with restart (RWR). PWN uses a weighted asymmetric network that is generated from an unweighted and undirected network. The weights come from two distinct features.
PWN is designed to manage the proportion of information circulating in and flowing out of certain regions by controlling the internal feature. PWN warps the network by assigning higher weights to prior knowledge-related edges.

□ Multi-Omics Integration For Disease Prediction Via Multi-Level Graph Attention Network And Adaptive Fusion
>> https://www.biorxiv.org/content/10.1101/2023.03.19.533326v1
This framework involves constructing co-expression and co-methylation networks for each subject, followed by applying multi-level graph attention to incorporate biomolecule interaction information.
The true-class-probability strategy is employed to evaluate omics-level confidence for classification, and the loss is designed using an adaptive mechanism to leverage both within- and across-omics information.
The initial feature is generated by the multi-level Graph Attention Network for each type of omics data respectively. The dicision feature of each type of omics data is generated by the TCP module. The decision features of each omics are concatenated into one fusion feature.

□ QADD: De Novo Drug Design by Iterative Multi-Objective Deep Reinforcement Learning with Graph-based Molecular Quality Assessment
>> https://academic.oup.com/bioinformatics/advance-article/doi/10.1093/bioinformatics/btad157/7085596
QADD designs a multi-objective deep reinforcement learning pipeline to generate molecules w/ multiple desired properties iteratively, where a graph neural network-based model for accurate molecular quality assessment on drug potentials is introduced to guide molecule generation.
QADD uses the Deep Q-Network, a value-based reinforcement learning method, to estimate the action-value function under different action selection strategies. Since it does not require a fixed-dimensional action space, it is particularly suitable for discontinuous space search.

□ Distances and their visualization in studies of spatial-temporal genetic variation using single nucleotide polymorphisms (SNPs)
>> https://www.biorxiv.org/content/10.1101/2023.03.22.533737v1
They recommend selection of a distance measure for SNP genotype data that does not give differing outcomes depending on the arbitrary choice, and consideration of which state should be considered as zero when applying binary distance measures to fragment presence-absence data.

□ BSP: Dimension-agnostic and granularity-based spatially variable gene identification
>> https://www.biorxiv.org/content/10.1101/2023.03.21.533713v1
BSP (big-small patch), a spatial granularity-guided and non-parametric model to identify spatially variable genes SVGs from two or three- dimensional spatial transcriptomics data in a fast and robust manner.
BSP selects a set of neighboring spots within a certain distance to capture the regional means with different granularities. The variances of the expression mean across all spots are then calculated under different scales, and genes with high ratios are identified as the SVGs.

□ Capturing Spatiotemporal Signaling Patterns in Cellular Data with Geometric Scattering Trajectory Homology
>> https://www.biorxiv.org/content/10.1101/2023.03.22.533807v1
GSTH, a general framework that encapsulates time-lapse signals on a cell adjacency graph in a low-dimensional trajectory. GSTH integrates geometric scattering and topological data analysis (TDA) to provide a comprehensive understanding of complex cellular interactions.
Geometric scattering employs wavelet-based transformations to extract multiscale representations of the signaling data, capturing the intricate hierarchical structures present in the spatial organization of cells and the temporal evolution of signaling events.

□ Ensemble-GNN: federated ensemble learning with graph neural networks for disease module discovery and classification
>> https://www.biorxiv.org/content/10.1101/2023.03.22.533772v1
Ensemble-GNN allows to quickly build predictive models utilizing PPI networks consisting of various node features such as gene expression and/or DNA methylation.
Ensemble-GNNs were combined into a global federated model. In the federated case, each client has its dedicated data based on which a GNN classifier is trained. The trained models of the ensembles are shared among all clients, and predictions are again made via Majority Vote.

□ Scrooge: A Fast and Memory-Frugal Genomic Sequence Aligner for CPUs, GPUs, and ASICs
>> https://academic.oup.com/bioinformatics/advance-article/doi/10.1093/bioinformatics/btad151/7085594
Scrooge, a fast and memory-frugal genomic sequence aligner. Scrooge includes three novel algorithmic improvements which reduce the data movement, memory footprint, and the number of operations in the GenASM algorithm.
GenASM-DC uses only cheap bitwise operations to calculate the edit distance between two strings text and pattern. It builds an (n+1)×(k+1) dynamic programming (DP) table R, where n=length(text) and k is the maximum number of edits considered.

□ Estimation of a treatment effect based on a modified covariates method with L0 norm
>> https://www.biorxiv.org/content/10.1101/2023.03.22.533735v1
A new treatment effect estimation approaches based on the modified covariate method, one using lasso regression and the other ridge regression, using the L0 norm.
A modified covariate method based on the L0 norm and Lq norm (q = 1, 2). The first method estimates treatment effects using lasso regression with the L0 norm. The second method uses ridge regression with the L0 norm.

□ PENCIL: Supervised learning of high-confidence phenotypic subpopulations from single-cell data
>> https://www.biorxiv.org/content/10.1101/2023.03.23.533712v1
PENCIL can perform gene selection during the training process, which allows learning proper gene spaces that facilitate accurate subpopulation identifications from single-cell data.
PENCIL has the flexibility to address various phenotypes such as binary, multi-category and continuous phenotypes. PENCIL can order cells to reveal the subpopulations undergoing continuous transitions between conditions.
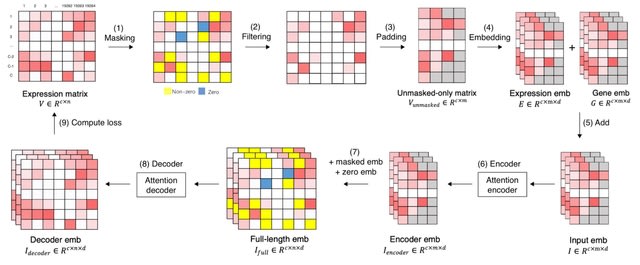
□ xTrimoGene: An Efficient and Scalable Representation Learner for Single-Cell RNA-Seq Data
>> https://www.biorxiv.org/content/10.1101/2023.03.24.534055v1
xTrimoGene reduces FLOPs by one to two orders of magnitude compared to classical transformers while maintaining high accuracy, enabling us to train the largest transformer models over the largest scRNA-seq dataset today.
xTrimoGene proposes an asymmetric encoder-decoder framework that takes advantage of the sparse gene expression matrix, and establishes the projection strategy of continuous values with a higher resolution.

□ EnsInfer: a simple ensemble approach to network inference outperforms any single method
>> https://bmcbioinformatics.biomedcentral.com/articles/10.1186/s12859-023-05231-1
EnsInfer, an ensemble approach to the network inference problem: each individual network inference method will work as a first level learning algorithm that gives a set of predictions from the gene expression input.
EnsInfer uses a combination of state-of-the-art inference approaches and combines them using a simple Naive Bayes ensemble model. EnsInfer essentially turns all the predictions from different inference algorithms into priors about each edge in the network.

□ Current sequence-based models capture gene expression determinants in promoters but mostly ignore distal enhancers
>> https://genomebiology.biomedcentral.com/articles/10.1186/s13059-023-02899-9
Enformer were not trained on GTEx / Cardoso-Moreira et al. data. specifically and do not directly give predictions for many human tissues. To match CAGE tracks to tissues and stages of development in a simple, yet data-driven, way, they fitted a ridge regression.
Enformer can predict endogenous RNA abundance very well and consistently outperforms previous models. Enformer substantially outperformed Basenji2 even when it is restricted to the latter model‘s input window and even on tasks where the receptive field size is irrelevant.

□ ElasticBLAST: accelerating sequence search via cloud computing
>> https://bmcbioinformatics.biomedcentral.com/articles/10.1186/s12859-023-05245-9
ElasticBLAST can handle anywhere from a few to many thousands of queries and run the searches on thousands of virtual CPUs.
ElasticBLAST leverages the cloud to provide multiple worker nodes to parallelize the computation by breaking the queries into query batches. ElasticBLAST relies on BLAST DB metadata that is automatically generated to determine the amount of main memory needed for that database.

□ SiPSiC: A novel method to accurately estimate pathway activity in single cells for clustering and differential analysis
>> https://www.biorxiv.org/content/10.1101/2023.03.27.534310v1
SiPSiC, a novel method for inferring pathway scores from scRNA-seq data. It has a high sensitivity, accuracy, and consistency with existing knowledge across different data types, including findings often missed by the original conventional analyses.
SiPSiC scores can be used to cluster the cells and compute their UMAP projections in a manner that better captures the biological underpinnings of tissue heterogeneity.

□ cnnLSV: detecting structural variants by encoding long-read alignment information and convolutional neural network
>> https://bmcbioinformatics.biomedcentral.com/articles/10.1186/s12859-023-05243-x
cnnLSV can automatically adjust the images from different variants to a uniform size according to the length of each variant and the coverage of the dataset for training the filtering model.
cnnLSV converts the images in training set into one-dimensional arrays, and executes the principal component analysis and k-means clustering to eliminate the incorrectly labeled images to improve the filtering performance of the model.

□ KGETCDA: an efficient representation learning framework based on knowledge graph encoder from transformer for predicting circRNA-disease associations
>> https://www.biorxiv.org/content/10.1101/2023.03.28.534642v1
Knowledge Graph Encoder from Transformer for predicting CDA (KGETCDA) integrates more than 10 databases to construct a large heterogeneous non-coding RNA dataset, which contains multiple relationships between circRNA, miRNA, lncRNA and disease.
A biological knowledge graph is created based on this dataset and Transformer-based knowledge representation learning and attentive propagation layers are applied to obtain high-quality embeddings with accurately captured high-order interaction information.

□ C-DEPP: Scaling deep phylogenetic embedding to ultra-large reference trees: a tree-aware ensemble approach
>> https://www.biorxiv.org/content/10.1101/2023.03.27.534201v1
Clustered-DEPP (C-DEPP) uses carefully crafted techniques to enable quasi-linear scaling while maintaining accuracy. C-DEPP enables placing twenty million 16S fragments on the GG2 reference tree in 41 hours of computation.
C-DEPP trains a separate model for each of several overlapping subtrees; for each query, C-DEPP uses a 2-level classifier to select one or more subtrees, computes distances using those subtrees, and uses these distances as input to APPLES-II, leaving the other distances blank.

□ simpleaf: A simple, flexible, and scalable framework for single-cell transcriptomics data processing using alevin-fry
>> https://www.biorxiv.org/content/10.1101/2023.03.28.534653v1
simpleaf, a program that simplifies the processing of single-cell data using tools from the alevin-fry ecosystem, and adds new functionality and capabilities, while retaining the flexibility and performance of the underlying tools.
simpleaf quant, simpleaf quant will automatically recruit and parameterize the correct mapper, and will automatically locate and provide the file containing the transcript-to-gene mapping information to later quantification stages where appropriate.

□ Sequencing accuracy and systematic errors in nanopore direct RNA sequencing
>> https://www.biorxiv.org/content/10.1101/2023.03.29.534691v1
The presence of the same systematic error patterns in RODAN points to more fundamental causes of errors in the raw signal data, necessitating further development of better pore chemistry to produce higher quality dRNA-seq data.
Clearly, further development of dRNA-seq protocols, pore chemistry and basecalling algorithms are desirable. Appropriate quality control and error correction methods are needed to mitigate the effects of high error rates and systematic biases in downstream analyses.