
(Made with Midjourney v5.2)

□ GEARS: Predicting transcriptional outcomes of novel multigene perturbations
>> https://www.nature.com/articles/s41587-023-01905-6
GEARS (graph-enhanced gene activation and repression simulator), a computational method that integrates deep learning with a knowledge graph of gene–gene relationships to simulate the effects of a genetic perturbation.
GEARS initializes a gene embedding vector and a gene perturbation embedding vector. GEARS optimizes model parameters to fit the predicted postperturbation gene expression to true postperturbation gene expression using stochastic gradient descent.

□ multiDGD: A versatile deep generative model for multi-omics data
>> https://www.biorxiv.org/content/10.1101/2023.08.23.554420v1
multiDGD is a generative model of transcriptomics and chromatin accessibility data. It consists of a decoder mapping shared representations of both modalities to data space, and learned distributions defining latent space.
multiDGD employs a Gaussian Mixture Model (GMM) as a distribution over latent space increases the ability of the latent distribution to capture clusters in comparison to the standard Gaussian used in applied VAEs.

□ Geniml: Genomic interval machine learning: Methods for evaluating unsupervised vector representations of genomic regions
>> https://www.biorxiv.org/content/10.1101/2023.08.28.555137v1
There exists no method for evaluating the quality of these embeddings in the absence of metadata, making it difficult to assess the reliability of analyses based on the embeddings, and to tune model training to yield optimal results.
To bridge this gap, they propose four evaluation metrics: the cluster tendency test (CTT), the reconstruction test (RCT), the genome distance scaling test (GDST), and the neighborhood preserving test (NPT).
The GDST and NPT exploit the biological tendency of regions close in genomic space to have similar biological functions; they measure how much such information is captured by individual region embeddings and a set of region embeddings.


□ Aligned Diffusion Schrödinger Bridges
>> https://arxiv.org/abs/2302.11419
Diffusion Schrödinger bridges (DSB) have recently emerged as a powerful framework for recovering stochastic dynamics via their marginal observations at different time points.
SBALIGN is a novel algorithmic framework derived from the Schrödinger bridge theory and Doob's h-transform. SBALIGN recovers a stochastic trajectory from the unbound to the bound structure.

□ Genetics of circulating inflammatory proteins identifies drivers of immune-mediated disease risk and therapeutic targets
>> https://www.nature.com/articles/s41590-023-01588-w
pQTLs provide valuable insights into the molecular basis of complex traits and diseases by identifying proteins that lie b/n genotype and phenotype. Integration of pQTL data with eQTL and GWAS provided insight into pathogenesis, implicating lymphotoxin-α in multiple sclerosis.
Using Mendelian randomization (MR) to assess causality in disease etiology, they identified both shared and distinct effects of specific proteins across immune-mediated diseases. Two-sided P values are from meta-analysis of linear regression estimates.

□ Σ-monoids: Categories of sets with infinite addition
>> https://arxiv.org/abs/2308.15183
Σ-monoids, a set with infinite addition. Their most general Σ-monoid structure admits additive inverses and generalises partially commutative monoids. Every Hausdorff commutative monoid is an instance of a Σ-monoid and that the corresponding forgetful functor has a left adioint.
Σ-monoids have well-defined tensor products, unlike topological abelian groups. Thus we may enrich categories over Σ-monoids, where composition respects addition of morphisms. This can be applied to categorical semantics of while loops for (quantum) computer programs.


□ Reverse Physics: Geometric and physical interpretation of the action principle
>> https://www.nature.com/articles/s41598-023-39145-y
Reverse Physics, an approach that examines current theories to find a set of starting physical assumptions that are sufficient to rederive them.
Hamiltonian system and Lagrangian mechanics is equivalent to three assumptions: determinism/reversibility, independence of degrees of freedom and kinematics/dynamics equivalence.

□ Totem: Cell-connectivity-guided trajectory inference from single-cell data
>> https://academic.oup.com/bioinformatics/advance-article/doi/10.1093/bioinformatics/btad515/7251030
Totem generates a large number of clustering results with a k-medoids algorithm (CLARA) and constructs an minimum spanning trees (MST) for each clustering. Totem estimates their topologies as minimum spanning trees, and uses them to measure the connectivity of the cells.
Totem smoothens the MSTs of the selected clustering results using the simultaneous principal curves algorithm of Slingshot to obtain directed trajectories that include pseudotime.

□ CASi: A multi-timepoint scRNAseq data analysis framework
>> https://www.biorxiv.org/content/10.1101/2023.08.16.553543v1
CASi providea a full analvsis pipeline for analyzing scRNA-seq data from multi-timepoint designs, Ultimately creating an informative profle of dynamic cellular changes.
CASi uses the neural network classifier to achieve cross-time points cell annotation. It avoids the overclustering issue. CASi uses the levels of similarity b/n the known cell types and new cells to identify potential novel cell types that may have appeared at later time points.

□ Sigmoni: classification of nanopore signal with a compressed pangenome index
>> https://www.biorxiv.org/content/10.1101/2023.08.15.553308v1
Sigmoni extends the r-index framework for read classification – first used in SPUMONI – to the problem of classifying raw nanopore electrical signal. Sigmoni uses an ultra-fast signal discretization method to project the current signal into a small alphabet for exact match querying with the r-index.
Sigmoni adapts the r-index classification framework to analysis of nanopore signal data using a combination of picoamp binning and a sampled document array structure for computing co-linearity statistics.
Sigmoni uses a novel classification method that accurately classifies reads using pseudo-matching lengths. By avoiding the complexities of the seed-chain-extend paradigm, Sigmoni's core algorithm consists only of a simple linear-time loop.

□ scNCL: transferring labels from scRNA-seq to scATAC-seq data with neighborhood contrastive regularization
>> https://academic.oup.com/bioinformatics/advance-article/doi/10.1093/bioinformatics/btad505/7243158
scNCL transforms scATAC- seq features into gene activity matrix based on prior knowledge. Since feature transformation can cause information loss, scNCL introduces neighborhood contrastive learning to preserve the neighborhood structure of scATAC-seq cells in raw feature space.
scNCL uses a feature projection loss and a alignment loss to harmonize embeddings between scRNA-seq and scATAC- seq. scNCL not only realizes accurate and robust label transfer for common types, but also achieves reliable detection of novel types.

□ GEDI: A unified model for interpretable latent embedding of multi-sample, multi-condition single-cell data
>> https://www.biorxiv.org/content/10.1101/2023.08.15.553327v1
GEDI (Gene Expression Decomposition and Integration), a generative model to identify latent space variations in multi-sample, multi-condition single cell datasets and attribute them to sample-level covariates.
GEDI can further project pathway and regulatory network activities onto the cellular state space, enabling the computation of the gradient fields of transcription factor activities and their association with the transcriptomic vector fields of sample covariates.

□ MarsGT: Multi-omics analysis for rare population inference using single-cell graph transformer
>> https://www.biorxiv.org/content/10.1101/2023.08.15.553454v1
MarsGT (Multi-omics analysis for rare population inference using single-cell Graph Transformer) employs a novel probability-based subgraph-sampling method, can highlight rare cell-related genes and peaks in a heterogeneous graph.
MarsGT calculates an entropy score to contrast the differences between the base and predicted cell clustering outcomes. The base cell clusters are ascertained by implementing the Louvain clustering method on the initial cell embeddings.

□ The unphysicality of Hilbert spaces
>> https://arxiv.org/abs/2308.06669
Hilbert spaces should not be considered the “correct” spaces to represent quantum states mathematically. Proving the requirements posited by complex inner product spaces are physically justified.
Completeness in the infinite-dimensional case requires the inclusion of states with infinite expectations, coordinate transformations that take finite expectations to infinite ones and vice-versa, and time evolutions that transform finite expectations to infinite ones in finite time.

□ Internal Grothendieck construction for enriched categories
>> https://arxiv.org/abs/2308.14455
Fundamental constructions in algebra, geometry, and topology can be understood as categorical concepts defined by certain universal properties.
The cartesian product of sets, the kernel of a linear map b/n vector spaces, and the fiber over a point in a topological space, are all instances of a universal construction called limit. The internal Grothendieck construction is closely related to internal discrete fibrations.

□ DeepTRs: Deep Learning Enhanced Tandem Repeat Variation Identification via Multi-Modal Conversion of Nanopore Reads Alignment
>> https://www.biorxiv.org/content/10.1101/2023.08.17.553659v1
DeepTRs, a novel method for identifying TR variations, which enables direct TR variation identification from raw Nanopore sequencing reads and achieves high sensitivity and completeness results through the multi-modal conversion of Nanopore reads alignment and deep learning.
DeepTR aligns the resulting nanopore reads and transformes into a positional weighted matrix (PWM). Subsequently, DeepTR converts the PWM into transformed similarity matrices (TSM) using modal conversion, which serve as inputs for the DeepTR Predictor.

□ CeLEry: Leveraging spatial transcriptomics data to recover cell locations in single-cell RNA-seq
>> https://www.nature.com/articles/s41467-023-39895-3
CeLEry (Cell Location recovEry) uses a deep neural network to learn the relationships between gene expression and spatial locations by minimizing a loss function that is specified according to the specific problem.
CeLEry generates replicates of the ST data via a variational autoencoder. The generated embedding and the gene cluster embedding are concatenated, which is used as input for a CNN to decode the concatenated embedding into a 2D matrix with the same dimension as the GE input.

□ SCA: recovering single-cell heterogeneity through information-based dimensionality reduction
>> https://genomebiology.biomedcentral.com/articles/10.1186/s13059-023-02998-7
Surprisal Component Analysis leverages the notion of surprisal, whereby less probable events are more informative when they occur, to assign a surprisal score to each transcript. SCA enables dimensionality reduction that better preserves information from rare defined cell types.
SCA projects the input data to a linear subspace spanned by a set of basis vectors. SCA is highly efficient, requires no information aside from transcript counts, and generalizes to data comprised of discrete cell types or continuous trajectories.

□ expiMap: Biologically informed deep learning to query gene programs in single-cell atlases
>> https://www.nature.com/articles/s41556-022-01072-x
ExpiMap learns to map cells into biologically understandable components representing known ‘gene programs’. The activity of each cell for a gene program (GP) is learned while simultaneously refining them and learning de novo programs.
The probabilistic representation learned by expiMap as a Bayesian model allows the performance of hypothesis testing on the integrated latent space of the query.

□ Data-driven discovery of oscillator models using SINDy: Towards the application on experimental data in biology
>> https://www.biorxiv.org/content/10.1101/2023.08.25.554817v1
Exploring the limitations of the SINDy approach in the specific context of oscillatory systems. By directly applying SINDy to experimental data, we define the main limiting aspects: data availability and quality, complexity of interactions, and dimensionality of systems.
SINDy struggles especially when the data resolution is low and the oscillatory behavior is characterized by strong time scale separation. When the variables forming the limit cycle are separated, SINDy identifies important dynamical features of the system from the phase space.

□ The phenotype-genotype reference map: Improving biobank data science through replication
>> https://www.cell.com/ajhg/fulltext/S0002-9297(23)00275-6
The GWAS catalog diseases and traits are annotated with the Experimental Factor Ontology (EFO). They attempted to annotate all EFO terms present in the filtered list of associations with a matching phecode.
The phenotype-genotype reference map (PGRM), a set of 5,879 genetic associations from 523 GWAS publications. The use of phecodes in the PGRM ensures interoperability with international ICD standards and a familiar context for researchers who work with EHR-linked biobanks.

□ dRFEtools: Dynamic recursive feature elimination for omics
>> https://academic.oup.com/bioinformatics/advance-article/doi/10.1093/bioinformatics/btad513/7252233
Recursive feature elimination (RFE) is an iterative process that optimally removes one feature at a time. We can eliminate a substantial number of features; however, it can be difficult to balance computational time and model performance degradation.
dRFEtools that implements dynamic RFE, reducing computational time with high accuracy compared to standard RFE, expanding dynamic RFE to regression algorithms, and outputting the subsets of features that hold predictive power with and without peripheral genes.
<bt />

□ sc-fGAIN: A novel f-divergence based generative adversarial imputation method for scRNA-seq data analysis
>> https://www.biorxiv.org/content/10.1101/2023.08.28.555223v1
sc-fGAIN, a novel f-divergence based generative adversarial imputation method for the scRNA-seq data imputation. The imputed values generated by sc-fGAIN have a smaller root-mean-square error, and it is robust to varying missing rates, moreover, it can reduce imputation bias.
Using sc-fGAIN algorithm, they identified four f-divergence functions: cross-entropy / Kullback-Leibler / reverse KL / Jensen-Shannon that can be integrated with GAIN to generate imputed values w/o any assumptions, and mathematically prove that the distribution of imputed data.

□ Hist2Vec: Kernel-Based Embeddings for Biological Sequence Classification
>> https://www.biorxiv.org/content/10.1101/2023.08.24.554699v1
Hist2Vec, a kernel-based embedding generation approach for capturing sequence similarities. Hist2Vec combines the concept of histogram-based kernel matrices and Gaussian kernel functions. It constructs histogram-based representations using the unique k-mers in the sequences.
Hist2Vec transforms the representations into high-dimensional feature spaces, preserving important sequence information. Hist2Vec employs kernel Principal Component Analysis (KPCA) to generate low-dimensional embeddings from the kernel matrix.

□ Automappa: An interactive interface for metagenome-derived genome bins
>> https://www.biorxiv.org/content/10.1101/2023.08.25.554826v1
Autometa is an automated workflow which aims to scale to the most complex communities that have been assembled. Therefore, Automappa was implemented to handle manual curation of MAGs at the scale of these complex datasets.
Automappa was designed to visualize, verify and refine genome binning results to aid curation of high-quality MAGs. It is composed of interactive and inter-connected tables and figures that support selection with real-time MAG quality updates.

□ SDePER: A hybrid machine learning and regression method for cell type deconvolution of spatial barcoding-based transcriptomic data
>> https://www.biorxiv.org/content/10.1101/2023.08.24.554722v1
SDePER uses a machine learning approach to remove the systematic difference between ST and scRNA-seq data (platform effects) explicitly and efficiently to ensure the linear relationship between ST data and cell type-specific expression profile.
SDePER considers sparsity of cell types per capture spot and across-spots spatial correlation in cell type compositions. SDePER imputes cell type compositions and gene expression at unmeasured locations in a tissue map with enhanced resolution.

□ Using LLM Models and Explainable ML to Analyse Biomarkers at Single Cell Level for Improved Understanding of Diseases
>> https://www.biorxiv.org/content/10.1101/2023.08.24.554441v1
A novel approach that employs both an LLM-based framework and explainable machine learning to facilitate generalization across single-cell datasets and identify gene signatures to capture disease-driven transcriptional changes.
An approach that combines supervised learning and a large language model. This method, which involves fine tuning scBERT and utilizing the QLattice. enhances cell type annotation and improves interpretability, generalizability, and scalability for scRNA-seq analvsis.
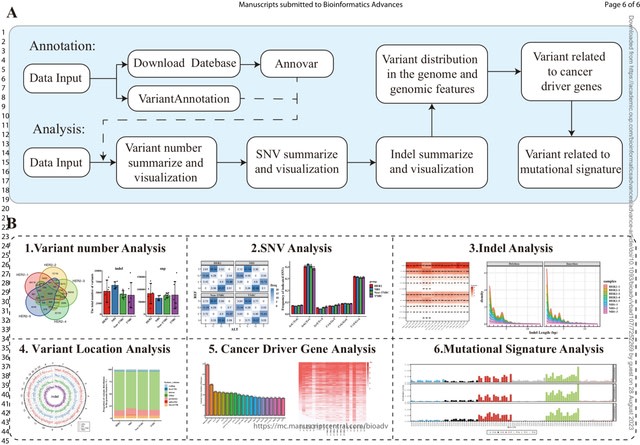
□ VCFshiny: An R/Shiny application for interactively analyzing and visualizing genetic variants
>> https://academic.oup.com/bioinformaticsadvances/advance-article/doi/10.1093/bioadv/vbad107/7252269
VCFshiny, an interactive R/Shiny application for analysing and visualizing VCF files. It allows non-bioinformatician researchers to upload VCF files to annotate and visualize detailed variant information without requiring any programming code.
VCFshiny accepts annotated VCF files for comparing and visualizing variants between different samples. VCFshiny offers two annotation methods, Annovar and VariantAnnotation, to add annotations such as genes or functional impact.

□ Examining dynamics of three-dimensional genome organization with multi-task matrix factorization
>> https://www.biorxiv.org/content/10.1101/2023.08.25.554883v1
Tree-Guided Integrated Factorization (TGIF), a multi-task learning framework using Non-negative Matrix Factorization (NMF) to enable joint identification of organizational units such as compartments and TADs across multiple conditions.
TGIF recovers ground-truth differential TAD boundaries with higher precision in simulated data and is more robust to calling false positive boundary changes arising due to differences in depth.

□ AutoHiC: a deep-learning method for automatic and accurate chromosome-level genome assembly
>> https://www.biorxiv.org/content/10.1101/2023.08.27.555031v1
AutoHiC harnesses the power of deep learning and Hi-C to automate
chromosome-level genome assembly and advance scaffold assembly. AutoHiC automates realize Hi-C assembly error correction, significantly improving genome assembly continuity and accuracy.
AutoHiC is based on the Swin Transformer architecture, which incorporates self-attention mechanisms. AutoHiC calculates the length of the inversion error based on the area of the peak on the interaction curve and then adjusts the sequence in that area in the opposite direction.

□ MAGinator enables strain-level quantification of de novo MAGs
>> https://www.biorxiv.org/content/10.1101/2023.08.28.555054v1
MAGinator provides de novo identification of subspecies-level microbes and accurate abundance estimates of metagenome-assembled genomes (MAGs).
MAGinator utilises the information from both gene- and contig-based methods yielding insight into both taxonomic profiles and the origin of genes as well as genetic content, used for inference of functional content of each sample by host organism.
MAGinator facilitates the reconstruction of phylogenetic relationships between the MAGs, providing a framework to identify clade-level differences within subspecies MAGs.

□ Joint-snhmC-seq: Joint single-cell profiling resolves 5mC and 5hmC and reveals their distinct gene regulatory effects
>> https://www.nature.com/articles/s41587-023-01909-2
Existing single-cell bisulfite sequencing methods cannot resolve 5mC and 5hmC, leaving the cell-type-specific regulatory mechanisms of TET and 5hmC largely unknown.
joint single-nucleus (hydroxy)methylcytosine sequencing (Joint-snhmC-seq), a scalable and quantitative approach that simultaneously profiles 5hmC and true 5mC in single cells by harnessing differential deaminase activity of APOBEC3A toward 5mC and chemically protected 5hmC.

□ A multimodal Transformer Network for protein-small molecule interactions enhances drug-target affinity and enzyme-substrate predictions
>> https://www.biorxiv.org/content/10.1101/2023.08.21.554147v1
PrpSmith facilitates the exchange of all relevant information between the two molecule types during the calculation of their numerical representations, allowing the model to account for their structural and functional interactions.
ProSmith combines gradient boosting predictions based on the resulting multimodal Transformer Network with independent predictions based on separate deep learning representations of the proteins and small molecules.

□ Sampling with flows, diffusion and autoregressive neural networks: A spin-glass perspective
>> https://arxiv.org/abs/2308.14085
A comparatively good grasp of parameter regions where traditional sampling methods like Monte Carlo sampling or Langevin dynamics are effective and where they are not.
Disordered models that exhibit a phase diagram of the random-first-order-theory type, called discontinuous one-step replica symmetry breaking, are typical in the mean-field theory of glass transition, but they also appear in a variety of random constraint satisfaction problems.
The tools available for outlining the phase diagrams of these problems turn out to be highly effective in analytically describing the performance of generative techniques such as flow-based, diffusion-based, or autoregressive networks for the respective probability measures.

□ SIMVI reveals intrinsic and spatial-induced states in spatial omics data
>> https://www.biorxiv.org/content/10.1101/2023.08.28.554970v1
SIMVI generates highly accurate SE inferences in synthetic datasets and unveils intrinsic variation in complex real datasets. SIMVI disentangles intrinsic and spatial variations in gene expression. It models the gene expression of each cell by two sets of low-dimensional latent variables. The spatial latent variables.are modeled by graph neural network variational posteriors.