
体制側の瑕疵に適応、あるいは逸脱する他に、内側から食い破ることも可能だ。後者の手段が実効性を持つには、組織の内殻に局在する瑕疵について、社会的に共有されているパースペクティブを還流する必要がある。


□ Exactly solvable models of stochastic gene expression
>> https://www.biorxiv.org/content/10.1101/2020.01.05.895359v1.full.pdf
The method was employed to approximate solutions to a broad class of linear multi-state gene expression models, which termed l-switch models, most of which currently have no known analytic solution.
While a characterisation of precisely which models the method is applicable to is not yet known, the results presented here suggest the recurrence method is an accurate and flexible tool for analysing stochastic models of gene expression.
Multistate models of gene expression which generalise the canonical Telegraph process, and are capable of capturing the joint effects of e.g. transcription factors, heterochromatin state and DNA accessibility (or, in prokaryotes, Sigma-factor activity) on transcript abundance.

□ TALC: Transcription-Aware Long Read Correction https://www.biorxiv.org/content/10.1101/2020.01.10.901728v1.full.pdf
In TALC, a path in the DBG is defined as an ordered list of connected k-mers (nodes) which are weighted by their number of occurrence in the SR dataset. any sequence of transcripts expressed in the RNA-seq sample should appear as a unique path of the graph.
TALC favours coverage-consistent exploration of the DBG. All paths passing the test described above are explored in parallel, according to a breadth-first approach.
Paths in the DBG that successfully bridge two Solid Regions are first ranked according to their sequence similarity with the Weak Region’s. The similarity is computed as the edit distance between sequences.
By integrating coverage information, TALC can efficiently account for the existence of multiple transcript isoforms. By eliminating inconsistent nodes, TALC reduces the exploration space in the graph and thus reduces the probability of exploring false paths.

□ LPWC: Lag Penalized Weighted Correlation for Time Series Clustering
>> https://bmcbioinformatics.biomedcentral.com/articles/10.1186/s12859-019-3324-1
Countless clustering algorithms group data points with similar characteristics, but the meaning of “similar” is inherently subjective and application-specific. In time series datasets, similarity must account for the temporal structure.
Dynamic Time Warping (DTW) aligns timepoints so that the distance between the aligned samples is minimized. LEAP allows time delays when constructing co-expression networks.
The LPWC similarity score is derived from weighted correlation, but the correlations of lagged temporal profiles are penalized using a Gaussian kernel. The kernel is also used to account for irregular time sampling.
LPWC is designed to identify groups of biological entities that exhibit the same pattern of activity changes over time. A solution can be verified in polynomial time and that the NP-complete Weighted Maximum Cut problem can be reduced to Lag Optimization in polynomial time.

□ ProphAsm: Simplitigs as an efficient and scalable representation of de Bruijn graphs https://www.biorxiv.org/content/10.1101/2020.01.12.903443v1.full.pdf
ProphAsm is a tool for computing simplitigs from k-mer sets and for k-mer set manipulation. Simplitigs are genomic sequences computed as disjoint paths in a bidirectional vertex-centric de Bruijn graph.
Compared to unitigs, simplitigs provide an improvement in the total number of sequences and their cumulative length, while both representations contain exactly the same k-mers.
ProphAsm, a tool implementing a greedy heuristic to compute maximal simplitigs. ProphAsm proceeds by building the associated de Bruijn graph in memory, followed by a greedy enumeration of maximum vertex-disjoint paths.

□ sstGPLVM: A Bayesian nonparametric semi-supervised model for integration of multiple single-cell experiments
>> https://www.biorxiv.org/content/10.1101/2020.01.14.906313v1.full.pdf
sstGPLVM the semi-supervised t-distributed Gaussian process latent variable model, which projects the data onto a mixture of fixed and latent dimensions, can learn a unified low-dimensional embedding for multiple single cell experiments with minimal assumptions.
sstGPLVM is the robust semi-supervised Gaussian process latent variable model to estimate a manifold that eliminates variance from un-wanted covariates and enables the imputation of missing covariates for other types of multi-modal data.

□ d-PBWT: dynamic positional Burrows-Wheeler transform
>> https://www.biorxiv.org/content/10.1101/2020.01.14.906487v1.full.pdf
d-PBWT, a dynamic version of the PBWT data structure. d-PBWT data structure can be initialized by direct bulk conversion from an existing PBWT. This algorithms open new research avenues for developing efficient genotype imputation and phasing algorithms.
two search algorithms for set maximal matches and long matches with worst case linear time complexity, but requiring multiple passes, and one search algorithm for long matches with average case linear time complexity with single pass without additional LEAP arrays data structures.

□ Alignment of single-cell RNA-seq samples without over-correction using kernel density matching
>> https://www.biorxiv.org/content/10.1101/2020.01.05.895136v1.full.pdf
Dmatch uses an external panel of primary cells to identify shared pseudo cell-types across scRNA-seq samples, and then finds a set of common alignment parameters that minimize gene expression level differences between cells that are determined to be the same pseudo cell-types.
the consistency of cell-type assignment was generally increased when all Pearson correlations of the 95-dimensional vectors were set to zero except for those between the cell and the top five reference atlas cell-types with the highest Pearson correlation coefficient.

□ Supervised Adversarial Alignment of scRNA-seq Data
>> https://www.biorxiv.org/content/10.1101/2020.01.06.896621v1.full.pdf
scDGN, Single Cell Domain Generalization Network includes three modules: scRNA encoder, label classifier and domain discriminator.
Gradient Reversal Layers (GRL) have no effect in forward propagation, but flip the sign of the gradients that flow through them during backpropagation.

□ Nanopore Sequencing at Mars, Europa and Microgravity Conditions
>> https://www.biorxiv.org/content/10.1101/2020.01.09.899716v1.full.pdf
Now ubiquitous on Earth and previously demonstrated on the International Space Station (ISS), nanopore sequencing involves translocation of DNA through a biological nanopore on timescales of milliseconds per base.
confirming the ability to sequence at Mars, Europa and Lunar g levels, the sequencing protocols under parabolic flight, and consistent performance across g level, during dynamic accelerations, and despite vibrations with significant power at translocation-relevant frequencies.
□ ABEMUS: platform specific and data informed detection of somatic SNVs in cfDNA
>> https://academic.oup.com/bioinformatics/advance-article-abstract/doi/10.1093/bioinformatics/btaa016/5699904
Next generation sequencing assays allow for the simultaneous interrogation of extended sets of somatic single nucleotide variants (SNVs) in circulating cell free DNA (cfDNA), a mixture of DNA molecules originating both from normal and tumor tissue cells.
Adaptive Base Error Model in Ultra-deep Sequencing data (ABEMUS), which combines platform-specific genetic knowledge and empirical signal to readily detect and quantify somatic SNVs in cfDNA.

□ DEcode: Decoding differential gene expression
>> https://www.biorxiv.org/content/10.1101/2020.01.10.894238v1.full.pdf
Combining the strengths of systems biology and deep learning in a model called DEcode, it is able to predict DE more accurately than traditional sequence-based methods, which do not utilize systems biology data.
the DEcode framework integrates a wealth of genomic data into a unified computational model of transcriptome regulations to predict multiple transcriptional effects, the absolute expression differences across, genes and transcripts, tissue- and person-specific transcriptomes.
DEcode builds a prediction model for tissue-specific gene expression for each tissue via XGBoost based on the training script from the ExPecto using the same hyper-parameters for XGBoost as in the script.

□ CaSpER identifies and visualizes CNV events by integrative analysis of single-cell or bulk RNA-sequencing data https://www.nature.com/articles/s41467-019-13779-x
CaSpER utilizes a non-linear median-based filtering of RNA-seq expression and allele-frequency signal. The median filtering preserves the edges of the signal much better compared to the kernel-based linear filters.
After the assignment of HMM states, CaSpER integrates the BAF shift signal with the assigned states to generate the final CNV calls.
□ Fast Zero-Inflated Negative Binomial Mixed Modeling Approach for Analyzing Longitudinal Metagenomics Data
>> https://academic.oup.com/bioinformatics/advance-article-abstract/doi/10.1093/bioinformatics/btz973/5697093
The FZINBMM approach is based on zero-inflated negative binomial mixed models (ZINBMMs) for modeling longitudinal metagenomic count data and a fast EM-IWLS algorithm for fitting ZINBMMs.
FZINBMM takes advantage of a commonly used procedure for fitting linear mixed models (LMMs), which allows us to include various types of fixed and random effects and within-subject correlation structures and quickly analyze many taxa.

□ LiPLike: Towards gene regulatory network predictions of high certainty
>> https://academic.oup.com/bioinformatics/advance-article/doi/10.1093/bioinformatics/btz950/5697090
the Linear Profile Likelihood (LiPLike), that assumes a regression model and iteratively searches for interactions that cannot be replaced by a linear combination of other predictors.
LiPLike could successfully remove false positive identifications from GRN predictions of other methods, and recognised this feature to be useful whenever high accuracy GRN predictions are sought from gene expression data.

□ Probabilistic gene expression signatures identify cell-types from single cell RNA-seq data
>> https://www.biorxiv.org/content/10.1101/2020.01.05.895441v1.full.pdf
a computationally light statistical approach, based on Naive Bayes, that leverages public datasets to combine information across thousands of genes and probabilistically assign cell-type identity.
To overcome the problem where the estimated rates are zero for hundreds of genes due to the sparsity of the data, using a hierarchical model that defines a cell-type-specific distribution with the hierarchical aspect providing statistical power in the presence of said sparsity.
□ isONcorrect: Error correction enables use of Oxford Nanopore technology for reference-free transcriptome analysis
>> https://www.biorxiv.org/content/10.1101/2020.01.07.897512v1.full.pdf
applying isONcorrect to direct RNA reads is a direction for future work that should enable the reference-free use of direct RNA reads.
IsONcorrect is able to jointly use all isoforms from a gene during error correction, thereby allowing it to correct reads at low sequencing depths.
As structural differences and variable coverage is at the heart of transcriptomic error correction, solving the partitioning problem by formulating it as a global with respect to the read k-mer anchor optimization problem over anchor depth.

□ SparkINFERNO: A scalable high-throughput pipeline for inferring molecular mechanisms of non-coding genetic variants
>> https://www.biorxiv.org/content/10.1101/2020.01.07.897579v1.full.pdf
SparkINFERNO (Spark-based INFERence of the molecular mechanisms of NOn-coding genetic variants), a scalable bioinformatics pipeline characterizing noncoding GWAS association findings.
SparkINFERNO algorithm integrates GWAS summary statistics with large-scale functional genomics datasets spanning enhancer activity, transcription factor binding, expression quantitative trait loci, and other functional datasets across more than 400 tissues and cell types.
SparkINFERNO is 61-times faster and scales well with the amount of computational resources. SparkINFERNO identified 1,418 and 15,343 candidate causal variants and 149 and 1,002 co-localized target gene-tissue combinations for IGAP and IBD.

□ BEM: Mining Coregulation Patterns in Transcriptomics via Boolean Matrix Factorization
>> https://academic.oup.com/bioinformatics/advance-article-abstract/doi/10.1093/bioinformatics/btz977/5698267
Boolean matrix factorization via expectation maximization (BEM) is more aligned with the molecular mechanism of transcriptomic coregulation and can scale to matrix with over 100 million data points.
BEM is applicable to all kinds of transcriptomic data, including bulk RNAseq, single cell RNAseq, and spatial transcriptomic datasets. Given appropriate binarization, BEM was able to extract coregulation patterns consistent with disease subtypes, cell types, or spatial anatomy.
□ A U-statistics for integrative analysis of multi-layer omics data
>> https://academic.oup.com/bioinformatics/advance-article-abstract/doi/10.1093/bioinformatics/btaa004/5698271
the proposed method is flexible for analyzing different types of outcomes as it makes no assumptions about their distributions, and outperformed the commonly used kernel regression-based methods.
a U-statistics-based non-parametric framework for the association analysis of multi-layer omics data, where consensus and permutation-based weighting schemes are developed to account for various types of disease models.
□ Gapsplit: Efficient random sampling for non-convex constraint-based models
>> https://academic.oup.com/bioinformatics/advance-article-abstract/doi/10.1093/bioinformatics/btz971/5698269
Combinatorial models may require an astronomical number of solutions to form a stable sampling distribution.For non-convex models the relationships between variables can change drastically from subspace to subspace.
Gapsplit provides uniform coverage of linear, mixed-integer, and general nonlinear models. Gapsplit generates random samples from convex and non-convex constraint-based models by targeting under-sampled regions of the solution space.
□ Lep-Anchor: Automated construction of linkage map anchored haploid genomes
>> https://academic.oup.com/bioinformatics/advance-article-abstract/doi/10.1093/bioinformatics/btz978/5698268
Lep-Anchor has been developed to efficiently anchor genomes into chromosomes using Lep-MAP3 and the additional information provided by long reads and contig-contig alignments to link contigs and to collapse haplotypes. Lep-Anchor supports millions of markers over multiple maps.
Lep-Anchor anchors genome assemblies automatically using dense linkage maps. the anchoring accuracy can be improved by utilising information about map position uncertainty.

□ Multiset sparse partial least squares path modeling for high dimensional omics data analysis
>> https://bmcbioinformatics.biomedcentral.com/articles/10.1186/s12859-019-3286-3
Multiset sparse Partial Least Squares path modeling (msPLS), a generalized penalized form of Partial Least Squares path modeling, for the simultaneous modeling of biological pathways across multiple omics domains.
msPLS is an multiset multivariate method for the integrative analysis of multiple high dimensional omics data sources. It accounts for the relationship between multiple high dimensional data sources while it provides interpretable results through its sparse solutions.

□ GSR: Partitioning gene-based variance of complex traits by gene score regression
>> https://www.biorxiv.org/content/10.1101/2020.01.08.899260v1.full.pdf
The rationale of Gene Score Regression (GSR) is based on the insight that genes that are highly correlated with the causal genes in the causal gene set or pathways will exhibit high marginal TWAS statistic.
Consequently, by regressing on the genes’ marginal statistic using the sum of the gene-gene correlation scores in each gene set, GSR can assess the amount of phenotypic variance explained by the predicted expression of the genes in that gene set.
And then calculate the statistical significance of each gene set based on the z-score of the linear regression coefficients in the GSR model.
□ DeepECA: an end-to-end learning framework for protein contact prediction from a multiple sequence alignment
>> https://bmcbioinformatics.biomedcentral.com/articles/10.1186/s12859-019-3190-x
DeepECA can obtain good results compared with existing ECA methods such as PSICOV, CCMpred, DeepCOV, and ResPRE when tested on the CASP11 and CASP12 datasets.
Deep ECA models to achieve improvement in the respective precisions of both shallow and deep MSAs. And expanded this model to a multi-task model to increase the prediction accuracy by incorporation with predictions of secondary structures and solvent-accessible surface areas.
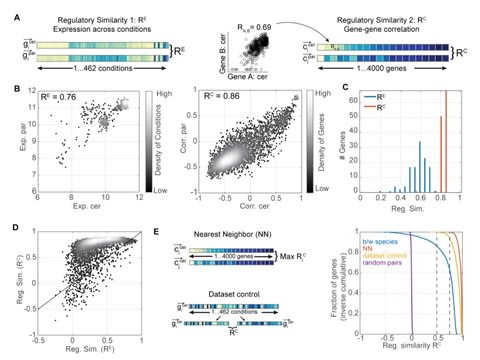
□ Independent evolution of transcript abundance and gene regulatory dynamics
>> https://www.biorxiv.org/content/10.1101/2020.01.22.915033v1.full.pdf
Profiling the interspecific hybrid provided insights into the basis of variations, showed that trans-varying alleles interact dominantly, and revealed complementation of cis-variations by variations in trans.
The data suggests that gene expression diverges primarily through changes in promoter strength that do not alter gene positioning within the transcription network.
□ Tagsteady: a metabarcoding library preparation protocol to avoid false assignment of sequences to samples
>> https://www.biorxiv.org/content/10.1101/2020.01.22.915009v1.full.pdf
Tagsteady, a metabarcoding Illumina library preparation protocol for pools of nucleotide-tagged amplicons that enables efficient and cost-effective generation of metabarcoding data with virtually no tag-jumps.
Tagsteady protocol is developed as a single-tube library preparation protocol, circumventing both the use of T4 DNA Polymerase in the end-repair step and the post-ligation PCR amplification step.
□ PARC: ultrafast and accurate clustering of phenotypic data of millions of single cells
>> https://academic.oup.com/bioinformatics/advance-article/doi/10.1093/bioinformatics/btaa042/5714737
PARC can cluster a single cell data set of 1.1M cells within 13 minutes, compared to > 2 hours for the next fastest graph-clustering algorithm.
PARC, “phenotyping by accelerated refined community-partitioning” - is a fast, automated, combinatorial graph-based clustering approach that integrates hierarchical graph construction (HNSW) and data-driven graph-pruning with the new Leiden community-detection algorithm.
□ Transfer index, NetUniFrac and some useful shortest path-based distances for community analysis in sequence similarity networks
>> https://academic.oup.com/bioinformatics/advance-article-abstract/doi/10.1093/bioinformatics/btaa043/5714743
the shortest path concept can be extended to sequence similarity networks by defining five new distances, NetUniFrac, Spp, Spep, Spelp and Spinp, and the Transfer index, between species communities present in the network.
NetUniFrac and the Transfer index can be computed in linear time with respect to the number of edges in the network.

□ SBOL Visual 2 Ontology
>> https://www.biorxiv.org/content/10.1101/2020.01.24.918417v1.full.pdf
the SBOL Visual 2 Ontology, which provides a machine-readable representation of the constraints attached to genetic circuit glyphs and their relationships to other ontological terms.
SBOL-VO can act as a a catalogue that can be converted into different formats and hence can be used to autogenerate portions of the SBOL Visual specification in the future.
Ontological axioms restricting the use of glyphs for different sequence features, molecules, or molecular interactions can directly be utilised in ontological queries and be submitted to existing reasoners, such as HermiT.
□ Pathway Mining and Data Mining in Functional Genomics. An Integrative Approach to Delineate Boolean Relationships Between Src and Its targets
>> https://www.biorxiv.org/content/10.1101/2020.01.25.919639v1.full.pdf
Boolean relationships between molecular components of cells suffer from too much simplicity regarding the complex identity of molecular interactions.
applying hierarchical clustering on expression of all DEGs just in Src over-activated samples. Pearson correlation coefficient was used as the distance in the clustering method.
Using information in KEGG and OmniPath databases to construct pathways from Src to DEGs (Differentially Expressed Genes) and between DEGs themselves.
□ FitHiC2: Identifying statistically significant chromatin contacts from Hi-C data
>> https://www.nature.com/articles/s41596-019-0273-0
FitHiC2, it is possible to perform genome-wide analysis for high-resolution Hi-C data, including all intra-chromosomal distances and inter-chromosomal contacts.
FitHiC2 also offers a merging filter module, which eliminates indirect/bystander interactions, leading to significant reduction in the number of reported contacts without sacrificing recovery of key loops such as those between convergent CTCF binding sites.
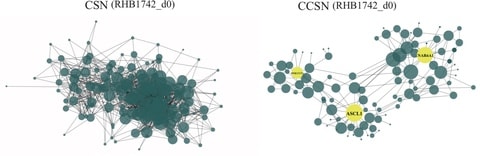
□ CCSN: Single Cell RNA Sequencing Data Analysis by Conditional Cell-specific Network
>> https://www.biorxiv.org/content/10.1101/2020.01.25.919829v1.full.pdf
To quantify the differentiation state of cells, developed a new method “network flow entropy” (NFE) to estimate the differentiation potency of cells by exploiting the gene-gene network constructed by CCSN.
CCSN reveals the network dynamics over the differentiation trajectory. The normalized gene expression profile and CSN/CCSN is used when compute the network flow entropy.
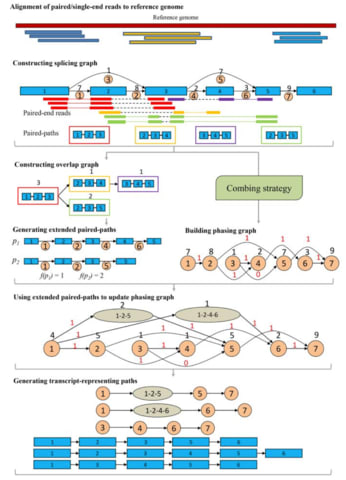
□ iPAC: A genome-guided assembler of isoforms via phasing and combing paths
>> https://academic.oup.com/bioinformatics/advance-article/doi/10.1093/bioinformatics/btaa052/5716327
iPAC, a new genome-guided assembler for reconstruction of isoforms, which revolutionizes the usage of paired-end and sequencing depth information via phasing and combing paths over a newly designed phasing graph.
Another new graph model, the phasing graph is introduced for solving the ambiguity of the connections of the in- and out- splicing junctions at each exon by effectively integrating the paired-end and sequence depth information.

□ HASLR: Fast Hybrid Assembly of Long Reads
>> https://www.biorxiv.org/content/10.1101/2020.01.27.921817v1.full.pdf
HASLR, a hybrid assembler which uses both second and third generation sequencing reads to efficiently generate accurate genome assemblies. HASLR is capable of assembling large genomes on a single computing node.
HASLR, similar to hybridSPAdes, Unicycler, and Wengan builds SR contigs using a fast SR assembler (i.e. Minia).
HASLR builds a novel data structure called backbone graph to put short read contigs in the order expected to appear in the genome and to fill the gaps between them using consensus of long reads.

□ SPARK: Statistical analysis of spatial expression patterns for spatially resolved transcriptomic studies
>> https://www.nature.com/articles/s41592-019-0701-7
SPARK identifies spatial expression patterns of genes in data generated from various spatially resolved transcriptomic techniques.
SPARK directly models spatial count data through generalized linear spatial models. It relies on recently developed statistical formulas for hypothesis testing, providing effective control of type I errors and yielding high statistical power.