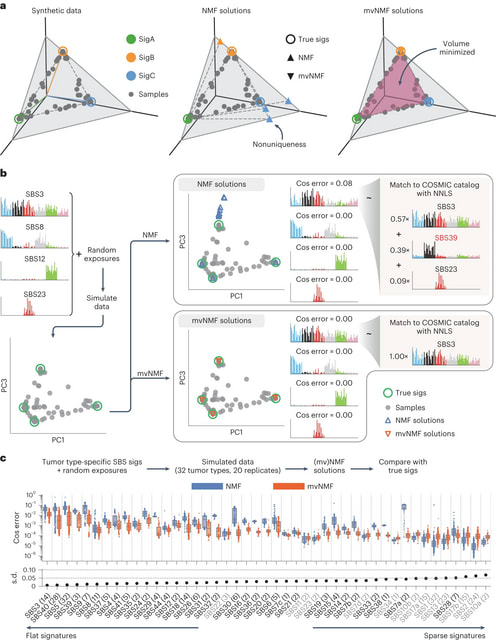
□ MuSiCal: Accurate and sensitive mutational signature analysis
>>
https://www.nature.com/articles/s41588-024-01659-0/figures/1
MuSiCal (Mutational Signature Calculator) decomposes a mutation count matrix into a signature matrixand an exposure matrix through four main modules: preprocessing, de novo discovery, matching and refitting, and in silico validation/optimization.
MuSiCal leverages several new methods, including minimum-volume nonnegative matrix factorization (mvNMF), likelihood-based sparse nonnegative least squares (NNLS) and a data-driven approach for systematic parameter optimization and in silico validation.
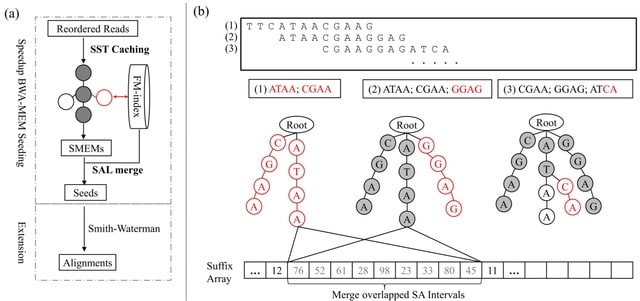
□ CompSeed: A compressive seeding algorithm in conjunction with reordering-based compression
>>
https://academic.oup.com/bioinformatics/advance-article/doi/10.1093/bioinformatics/btae100/7611649
CompSeed, in collaboration with the reordering-based compression tools, finishes the BWA-MEM seeding in about half the time by caching all intermediate seeding results in compact trie structures to directly answer repetitive inquiries that frequently cause random memory accesses.
CompSeed demonstrates better performance as sequencing coverage increases, as it focuses solely on the small informative portion of sequencing reads after compression.
CompSeed fully utilizes the redundancy information provided from upstream compressors using trie structures, and avoids ~50% of the redundant time-consuming FM-index operations during the BWA-MEM seeding process.

□ Finimizers: Variable-length bounded-frequency minimizers for k-mer sets
>>
https://www.biorxiv.org/content/10.1101/2024.02.19.580943v1
finimizers (frequency-bounded minimizers) uses an order relation < for minimizer comparison that depends on the frequency of the minimizers within the indexed k-mers.
With finimizers, the length m of the m-mers is not fixed, but is allowed to vary depending on the context, so that the length can increase to bring the frequency down below a user-specified threshold t.
Setting a maximum frequency solves the issue of very frequent minimizers and gives us a worst-case guarantee for the query time. They show how to implement a particular finimizer scheme using the Spectral Burrows-Wheeler Transform augmented with longest common suffix information.

□ stMMR: accurate and robust spatial domain identification from spatially resolved transcriptomics with multi-modal feature representation
>>
https://www.biorxiv.org/content/10.1101/2024.02.22.581503v1
stMMR utilizes spatial location information as a bridge to establish adjacency relationships between spots. It encodes gene expression data and morphological features extracted from histological images using Graph Convolutional Networks.
stMMR achieves joint learning of intra-modal and inter-modal features. stMMR employs self-attention mechanisms to learn the relationships of different spots. stMMR utilizes similarity contrastive learning along with the reconstruction of GE features and adjacency information.

□ SVarp: pangenome-based structural variant discovery
>>
https://www.biorxiv.org/content/10.1101/2024.02.18.580171v1
SVarp addresses the gap by calling SVs on graph genomes using third generation long sequencing reads. It enables us to find additional SVs that are currently missing, including SVs on top of alternative sequences present in the pangenome but not in a linear reference.
SVarp calls novel phased variant sequences, which they call ‘svtigs’. The variant representation is not tied to a single linear reference and allows for flexible downstream workflows that derive variant calls. The svtigs can serve as a basis to amend a pangenome graph.

□ CoCoPyE: feature engineering for learning and prediction of genome quality indices
>
https://www.biorxiv.org/content/10.1101/2024.02.07.579156v1
CoCoPyE is a fast tool based on a novel two-stage feature extraction and transformation scheme. CoCoPyE identifies genomic markers and then refines the marker-based estimates with a machine learning approach.
The original feature space comprises more than 10,000 dimensions which correspond to different protein domain families. Large-scale machine learning within such a high-dimensional space is burdensome.
CoCoPyE mapps the original profile space to a lower dimensional histogram space. A count ratio histogram (CRH) arises from the comparison of a candidate profile with a reference profile in terms of the observed ratios between the corresponding protein domain counts.

□ T-S2Inet: Transformer-based sequence-to-Image network for accurate nanopore sequence recognition
>>
https://academic.oup.com/bioinformatics/advance-article/doi/10.1093/bioinformatics/btae083/7609038
T-S2Inet, the transformer-based model to capture the accurate Nanopore Sequence Recognition. T-S2Inet uses a Sequence-to-Image (S2I) module that applies transformation rules to convert the unequal length sequence to a fixed-size image.
The objective of the S2I module is to convert sequences of unequal lengths into images of uniform dimensions. T-S2Inet utilizes GASF/GADF for nanopore sequence transformation, and trains and predicts the model through a subsequent deep neural network.

□ BootCellNet, a resampling-based procedure, promotes unsupervised identification of cell populations via robust inference of gene regulatory networks.
>>
https://www.biorxiv.org/content/10.1101/2024.02.06.579236v1
BootCellNet employs smoothing and resampling to infer GRNs. Using the inferred GRNs, BootCellNet further infers the minimum dominating set (MDS), a set of genes that determines the dynamics of the entire network.
In BootCellNet, GRN reconstruction is performed with the ARACNe method. NestBoot utilizes a nested bootstrap to control FDR in GRN inference, and they showed that the bootstrapping procedure improved the accuracy of the GRN inference by various inference methods such as GENIE3.
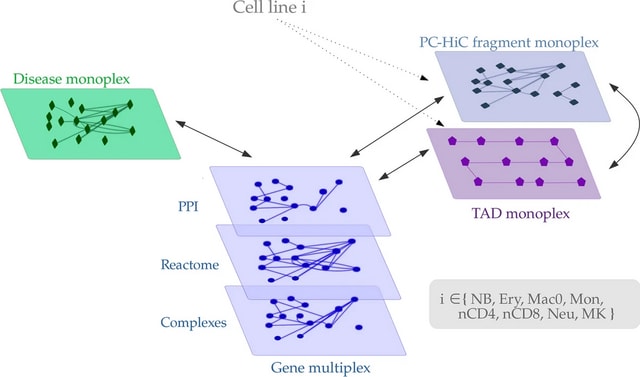
□ MultiXrank: Random walk with restart on multilayer networks: from node prioritisation to supervised link prediction and beyond
>>
https://bmcbioinformatics.biomedcentral.com/articles/10.1186/s12859-024-05683-z
MultiXrank, a Random Walk with Restart algorithm able to explore such multilayer networks. MultiXrank outputs scores reflecting the proximity between an initial set of seed node(s) and all the other nodes in the multilayer network.
In this multilayer framework, all the networks can also be weighted and/or directed. MultiXrank outputs scores representing a measure of proximity between the seed(s) and all the nodes of the multilayer network.

□ 123VCF: an intuitive and efficient tool for filtering VCF files
>>
https://bmcbioinformatics.biomedcentral.com/articles/10.1186/s12859-024-05661-5
123VCF filters input variants in accordance with a predefined filter sequence applied to the input variants. Users are provided the flexibility to define various filtering parameters, such as quality, coverage depth, and variant frequency within the populations.
123VCF can generate a Tab-Separated Values (TSV) file containing all passed variants, which can be easily imported into spreadsheet-based programs for further analysis. 123VCF can also generate another TSV file specifically for variants that overlap w/ a user-provided BED file.

□ KRANK: Memory-bound k-mer selection for large evolutionary diverse reference libraries
>>
https://www.biorxiv.org/content/10.1101/2024.02.12.580015v1
KRANK (K-mer RANKer) combines several components, including a hierarchical selection strategy with adaptive size restrictions and an equitable coverage strategy.
KRANK is centered around a hierarchical traversal of the taxonomy, constructing hash tables separately for each taxon, and merging these to represent the parent taxa. Thus, instead of constructing a global hash table once at the root, it builds the library gradually.

□ Klumpy: A tool to evaluate the integrity of long-read genome assemblies and illusive sequence motifs
>>
https://www.biorxiv.org/content/10.1101/2024.02.14.580330v1
Klumpy, a bioinformatic tool designed to detect genome misassemblies, misannotations, and incongruities in long-read-based genome assemblies and their constituent raw reads.
Klumpy scans through a genome assembly and provide users with a list of potentially misassembled regions, and annotate sequences of interest (e.g., an assembled genome or its underlying raw reads) given a query of interest.
These two modes of operation can work synergistically to annotate an assembly and the constituent raw reads together, based on a supplied, specific query (defined as any nucleotide sequence including, e.g., genes, regulatory motifs, or transposable elements).

□ RUBICON: a framework for designing efficient deep learning-based genomic basecallers
>>
https://genomebiology.biomedcentral.com/articles/10.1186/s13059-024-03181-2
RUBICON, the first framework for specializing and optimizing a machine learning-based basecaller. RUBICON uses two machine learning techniques to develop hardware-optimized basecallers that are specifically designed for basecalling.
RUBICON uses QABAS, an automatic architecture search for computation blocks and optimal bit-width precision, and SkipClip, a dynamic skip connection removal module. QABAS uses neural architecture search to evaluate millions of different basecaller architectures.
RUBICALL is the first hardware-optimized basecaller, demonstrates fast, accurate, and efficient basecalling, achieving 6.88× reductions in model size with 2.94× fewer neural network parameters.

□ Fasta2Structure: a user-friendly tool for converting multiple aligned FASTA files to STRUCTURE format
>>
https://bmcbioinformatics.biomedcentral.com/articles/10.1186/s12859-024-05697-7
Fasta2Structure, a graphical user interface (GUI) application designed to simplify the process of converting multiple sequence alignments into a single, cohesive file that is compatible with the STRUCTURE software.
fasta2structure incorporates all variable sites present in the alignments. fasta2structure exhibits a higher degree of robustness in converting a wider array of data types, encompassing those with significant genetic variation.

□ pipesnake : Generalized software for the assembly and analysis of phylogenomic datasets from conserved genomic loci
>>
https://www.biorxiv.org/content/10.1101/2024.02.13.580223v1
ausarg/pipesnake is a bioinformatics best-practice analysis pipeline for phylogenomic reconstruction starting from short-read 'second-generation' sequencing data.
pipesnake workflow generates a number of output files that are stored in process-specific directories. This allows the user to store and inspect intermediate files such as individual sample PRGs, alignment files, and locus trees.

□ PIMENTA: PIpeline for MEtabarcoding through Nanopore Technology used for Authentication
>>
https://www.biorxiv.org/content/10.1101/2024.02.14.580249v1
PIMENTA, a PIpeline for MEtabarcoding through Nanopore Technology used for Authentication. PIMENTA is a pipeline for rapid taxonomic identification in samples using MinION metabarcoding sequencing data.
The PIMENTA pipeline consists of eight linked tools, and data analysis passes through 3 phases: 1) pre-processing the MinION data through read calling, demultiplexing, trimming sequencing adapters, quality trimming and filtering the reads,
2) clustering the reads, continued by MSA and consensus building per cluster, 3) reclustering of consensus sequences, followed by another MSA and consensus building per cluster, 4) Taxonomy identification with the use of a BLAST analysis.

□ EvoAug-TF: Extending evolution-inspired data augmentations for genomic deep learning to TensorFlow
>>
https://academic.oup.com/bioinformatics/advance-article/doi/10.1093/bioinformatics/btae092/7609674
EvoAug-TF adapts the functionality of the PyTorch-based EvoAug framework in TensorFlow, including the augmentation techniques (e.g., random transversion, insertion, translocation, deletion, mutation, and noise).
EvoAug-TF employs the same two-stage training curriculum, where stochastic augmentations are applied online to each mini-batch during training, followed by a finetuning step on the original, unperturbed data.
Since EvoAug-TF imposes transformations on the input data while maintaining the same labels as the wildtype sequence, in its current form, EvoAug-T only supports DNNs that output scalars in single-task or multi-task settings.

□ K2R: Tinted de Bruijn Graphs for efficient read extraction from sequencing datasets
>>
https://www.biorxiv.org/content/10.1101/2024.02.15.580442v1
K2R, a highly scalable index that implement such search efficiently within this framework. K2R consistently outperforms contemporary solutions in most metrics and is the only tool capable of scaling to larger datasets.
K2R's performance, in terms of index size, memory footprint, throughput, and construction time, is benchmarked against leading methods, including hashing techniques (e.g., Short Read Connector) and full-text indexing (e.g., Spumoni and Movi), across various datasets.

□ Delineating the Effective Use of Self-Supervised Learning in Single-Cell Genomics
>>
https://www.biorxiv.org/content/10.1101/2024.02.16.580624v1
Central to this framework is the use of fully connected autoencoder architectures, selected for their ubiquitous application in SCG tasks and for minimizing architectural influences on our study, yet still large enough to capture underlying biological variations.
In this framework, they integrate key SSL pretext tasks based on masked autoencoders and contrastive learning to benchmark their performance. The framework operates in two stages: The first stage is pre-training pretext task, where the model learns from unlabeled data.
They call the resulting model 'SSL-zero-shot' for its zero-shot evaluation. The second stage is the optional fine-tuning. Calling the resulting model the 'SSL' model, which is further trained to specific downstream tasks such as cell type annotation.
Thie SSL framework leverages Masked Autoencoder with Random Masking and Gene Program Masking (GP) strategies, along with the Isolated Masked Autoencoder (iMAE) approaches GP to GP and Gene Program to Transcription Factor masking, considering isolated sets of genes.
The strategies entail leveraging different degrees of biological insight, from random masking with a minimal inductive bias to isolated masking that intensively utilizes known gene functions, emphasizing targeted biological relationships.

□ The Backpack Quotient Filter: a dynamic and space-efficient data structure for querying k-mers with abundance.
>>
https://www.biorxiv.org/content/10.1101/2024.02.15.580441v1
The Backpack Quotient Filter (BQF) is an indexing data structure with abundance. Although the data can be anything, it's been thought to index genomic datasets. The BQF is a dynamic structure, with a correct hash function it can add, delete and enumerate elements.
BQF relies on a hash-table-like structure called Quotient Filter. Part of the information inserted is stored implicitly within the address in the table where it is written.
BQF inserts and query s-mers but virtualizes the presence of k-mers at query time. In other words, a query sequence is broken down into k-mers, and each k-mer is virtually queried through all of its s-mers.

□ Identifying Reproducible Transcription Regulator Coexpression Patterns with Single Cell Transcriptomics
>>
https://www.biorxiv.org/content/10.1101/2024.02.15.580581v1
Adopting a "TR-centric" approach towards aggregating single cell coexpression networks, with the primary goal of learning reproducible TR interactions. It assembles a diverse range of scRNA-seq data to better understand the coexpression range of all measurable.
The key aim was to prioritize the genes that are most frequently coexpressed with each TR, hypothesizing that this prioritization can facilitate the identification of direct TR-target interactions.

□ Marsilea: An intuitive generalized visualization paradigm for complex datasets
>>
https://www.biorxiv.org/content/10.1101/2024.02.14.580236v1
Marsilea, a Python library designed for creating complex visualizations with ease. Marsilea is notable for its modularity, diverse plot types, compatibility with various data formats, and is available in a coding-free web-based interface for users of all experience levels.
For datasets with categorical axis, the paradigm allows incorporation of data-driven structure, for example, through hierarchical clustering showcasing similarities within and between data groups, adding a deeper analytical dimension.
Additionally, the paradigm offers versatility through concatenation and recursion: secondary plots can transform into central plots of new cross-layouts that are connected to the initial one, allowing for intricate and detailed visual representations of the data.
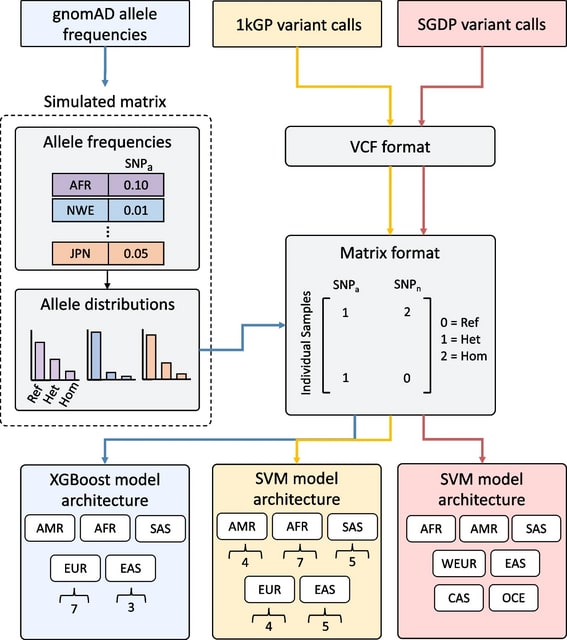
□ SNVstory: inferring genetic ancestry from genome sequencing data
>>
https://bmcbioinformatics.biomedcentral.com/articles/10.1186/s12859-024-05703-y
SNVstory incorporates samples/variants from three different curated datasets, expanding the number of labels and the granularity of the model classification beyond the main continental divisions.
Drawing upon the gnomAD database produces a much larger number of variants on which our models were trained, providing the opportunity to classify ancestry on a wider (or more diverse) range of features.
SNVstory excludes consanguineous samples, ensuring that the overrepresentation of closely related individuals does not bias the model. This implementation is optimized for individualized results rather than clustering large cohorts of samples into shared ancestral groups.

□ SF-Relate: Secure Discovery of Genetic Relatives across Large-Scale and Distributed Genomic Datasets
>>
https://www.biorxiv.org/content/10.1101/2024.02.16.580613v1
SF-Relate, a practical and secure federated algorithm for identifying genetic relatives across data silos. SF-Relate vastly reduces the number of individual pairs to compare while maintaining accurate detection through a novel locality-sensitive hashing approach.
SF-Relate constructs an effective hash function that captures identity-by-descent (IBD) segments in genetic sequences, which, along with a new bucketing strategy, enable accurate and practical private relative detection.
SF-Relate uses a novel encoding scheme that splits and subsamples genotypes into k-SNPs (similar to k-mers, but non-contiguous), such that the similarity between k-SNPs reflects extended runs of identical genotypes, typically indicative of relatedness.

□ Flexiplex: a versatile demultiplexer and search tool for omics data
>>
https://academic.oup.com/bioinformatics/advance-article/doi/10.1093/bioinformatics/btae102/7611801
Flexiplex, a versatile and fast sequence searching and demultiplexing tool, which is based on the Levenshtein distance. Given a set of reads as either .fastq or .fasta it will demultiplex and/or identify target sequences, reporting matching reads and read-barcode assignment.
Flexiplex first uses edlib to search for a left and right flanking sequence within each read. For the best match with an edit distance of “f” or less it will trim to the barcode + UMI sequence +/- 5 bp either side, and search for the barcode against a known list.
Occassionally reads are chimeric, meaning two or more molecules get sequence togther in the same read. Flexiplex will repeat the search again with the previously found primer to polyT sequence masked out. This is repeated until no new barcodes are found in the read.

□ Structured Prompt Interrogation and Recursive Extraction of Semantics (SPIRES): a method for populating knowledge bases using zero-shot learning
>>
https://academic.oup.com/bioinformatics/advance-article/doi/10.1093/bioinformatics/btae104/7612230
SPIRES with a model of chemical to disease (CTD) associations based on the Biolink Model. Biolink extends the simple triple model of associations to include qualifiers on the predicate, subject, and object.
SPIRES performs grounding and normalization with the Ontology Access Kit library (OAKlib), which provides interfaces for multiple annotation tools, including the Gilda entity normalization tool, the BioPortal annotator, and the Ontology Lookup Service.
For identifier normalization a number of services can be used, including OntoPortal mappings, with the default being the NCATS Biomedical Translator Node Normalizer.
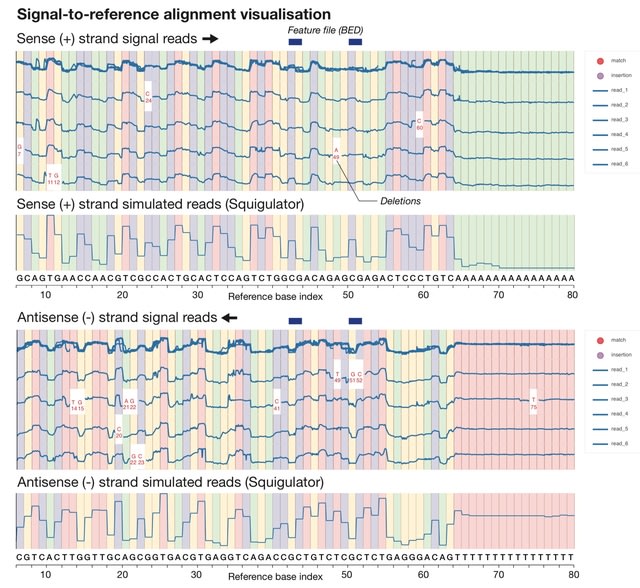
□ Squigualiser: Interactive visualisation of raw nanopore signal data
>>
https://www.biorxiv.org/content/10.1101/2024.02.19.581111v1
Squigualiser builds upon existing methodology for signal-to-sequence alignment in order to anchor raw signal data points to their corresponding positions within basecalled reads or within a reference genome/transcriptome sequence.
Squigualiser enables efficient representation of signal alignments and normalises outputs. A new method for k-mer-to-base shift correction addresses ambiguity in signal alignments to enable visualisation of genetic variants, modified bases, at single-base resolution.

□ SLIDE: Significant Latent Factor Interaction Discovery and Exploration across biological domains
>>
https://www.nature.com/articles/s41592-024-02175-z
Significant Latent factor Interaction Discovery and Exploration (SLIDE), a first-in-class interpretable machine learning technique for identifying significant interacting latent factors underlying outcomes of interest from high-dimensional omic datasets.
SLIDE makes no assumptions regarding data-generating mechanisms, comes with theoretical guarantees regarding identifiability of the latent factors/corresponding inference, outperforms/performs at least as well as state-of-the-art approaches in terms of prediction.

□ A tractable tree distribution parameterized by clade probabilities and its application to Bayesian phylogenetic point estimation
>>
https://www.biorxiv.org/content/10.1101/2024.02.20.581316v1
A new tractable tree distribution and associated point estimator that can be constructed from a posterior sample of trees. This point estimator performs at least as well and often better than standard methods of producing Bayesian posterior summary trees.

□ Fast and accurate short read alignment with hybrid hash-tree data structure
>>
https://www.biorxiv.org/content/10.1101/2024.02.20.581311v1
The actual sequencer should be able to generate many small fasta files for the data of one human genome, since actual reading process is highly parallel. They assume that input data are available as a number small fasta files.
This new hybrid hash-tree algorithm requires fairly large (around 100GB) table to express the reference genome. Therefore, this table must be shared by processes which handle the reads in parallel.
The SWG program performs the match through Smith-Waterman-Gotoh algorithm and calculates the matching sore, does not require large tables. It process one file and generate SAM-format output. For parallel processing they just run a fixed number of this program in parallel.