“One thought fills immensity.”

□ ptdalgorithms: Graph-based algorithms for phase-type distributions
>> https://www.biorxiv.org/content/10.1101/2022.03.12.484077v1.full.pdf
ptdalgorithms that implements graph-based algorithms for constructing and transforming unrewarded and rewarded continuous and discrete phase-type distributions and for computing their moments and distribution functions.
For generalized iterative state-space construction, ptdalgorithms allows the computation of moments for huge state spaces, and for the state probability vector of the underlying Markov chains of both time-homogeneous and time-inhomogeneous phase-type distributions.

□ SIEVE: joint inference of single-nucleotide variants and cell phylogeny from single-cell DNA sequencing data
>> https://www.biorxiv.org/content/10.1101/2022.03.24.485657v1.full.pdf
The previous methods do not operate within the statistical phylogenetic framework, in particular do not infer branch lengths of the tree. Moreover, either they fully follow the infinite-sites assumption (ISA).
SIEVE (SIngle-cell EVolution Explorer) exploits raw read counts for all nucleotides from scDNA-seq to reconstruct the cell phylogeny and call variants based on the inferred phylogenetic relations. SIEVE employs a statistical phylogenetic model following finite-sites assumption.
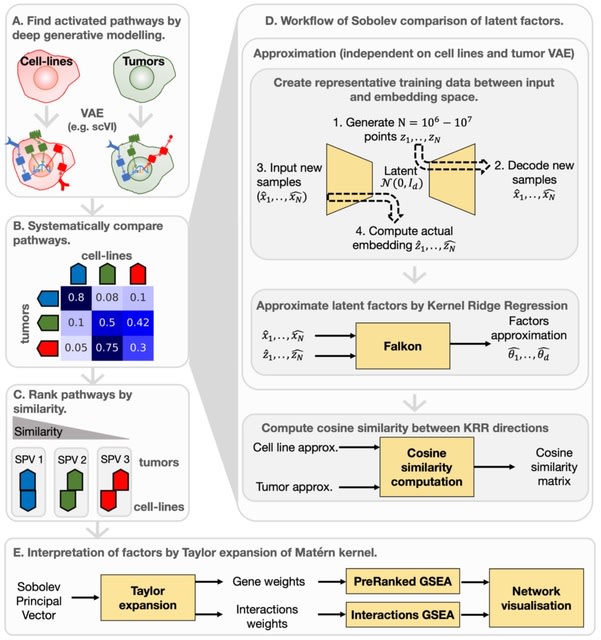
□ Sobolev Alignment: Identifying commonalities between cell lines and tumors at the single cell level using Sobolev Alignment of deep generative models
>> https://www.biorxiv.org/content/10.1101/2022.03.08.483431v1.full.pdf
Sobolev Alignment, a computational framework which uses deep generative models to capture non-linear processes in single-cell RNA sequencing data and kernel methods to align and interpret these processes.
Recent works have shown theoretical connections, demonstrating, for instance, the equivalence between the Laplacian kernel and the so-called Neural Tangent Kernel.
The interpretation scheme relies on the decomposition of the Gaussian kernel, which we extended to the Laplacian kernel by exploiting connections between the feature spaces of Gaussian and Laplacian kernels.
Mapping towards the latent factors using Falkon-trained kernel machines, which allows to calculate the contribution of each gene to each latent factors. Constructing a consensus space by interpolation b/n matched Sobolev Principal Vectors onto which all data can be projected.

□ scAllele: a versatile tool for the detection and analysis of variants in scRNA-seq
>> https://www.biorxiv.org/content/10.1101/2022.03.29.486330v1.full.pdf
scAllele, a versatile tool that performs both variant calling and functional analysis of the variants in alternative splicing using scRNA-seq. As a variant caller, scAllele reliably identifies SNVs and microindels (less than 20 bases) with low coverage.
scAllele calls nucleotide variants via local reassembly. scAllele enables read-level allelic linkage analysis. It refines read alignments and possible misalignments, and enhances variant detection accuracy per read. scAllele uses a GLM model to detect high confidence variants.

□ The complexity of the Structure and Classification of Dynamical Systems
>> https://arxiv.org/pdf/2203.10655v1.pdf
A survey of the complexity of structure, anti-structure, classification and anti-classification results in dynamical systems. Focussing primarily on ergodic theory, with excursions into topological dynamical systems, but suggest methods and problems in related areas.
Every perfect Polish space contains a non-Borel analytic set. Moreover, the analytic sets are closed under countable intersections and unions. Hence the co- analytic sets are also closed under unions and intersections.
Are there complete numerical invariants for orientation preserving diffeomorphisms of the circle up to conjugation by orientation preserving diffeomorphisms?

□ A glimpse of the toposophic landscape: Exploring mathematical objects from custom-tailored mathematical universes
>> https://arxiv.org/pdf/2204.00948.pdf
There are toposes in which the axiom of choice and the intermediate value theorem from undergraduate calculus fail, toposes in which any function R → R is continuous and toposes in which infinitesimal numbers exist.
In the semantic view, the effective topos is an alternative universe which contains its own version of the natural numbers. “There are infinitely many primes in Eff” is equivalent to the statement “for any number n, there effectively exists a prime number p > n”.

□ ALFATClust: Clustering biological sequences with dynamic sequence similarity threshold
>> https://bmcbioinformatics.biomedcentral.com/articles/10.1186/s12859-022-04643-9
ALFATClust exploits rapid pairwise alignment-free sequence distance calculations and community detection. Although ALFATClust computes a full Mash distance matrix for its graph clustering, the matrix can be significantly reduced using a divide-and-conquer approach.
ALFATClust is conceptually similar to hierarchical agglomerative clustering since its algorithm begins with each sequence (vertex) as a singleton graph cluster, and the graph clusters are gradually merged through iterations with decreasing resolution parameter γ.

□ The Graphical R2D2 Estimator for the Precision Matrices
>> https://www.biorxiv.org/content/10.1101/2022.03.22.485374v1.full.pdf
Graphical R2D2 (R2-induced Dirichlet Decomposition) draws Monte Carlo samples from the posterior distribution based on the graphical R2D2 prior, to estimate the precision matrix for multivariate Gaussian data.
GR2D2 estimator has attractive properties in estimating the precision ma- trices, such as greater concentration near the origin and heavier tails than current shrinkage priors.
When the true precision matrix is sparse and of high dimension, The graphical R2D2 hierarchical model provides estimates close to the true distribution in Kullback-Leibler divergence and with the smallest bias for nonzero elements.

□ PORTIA: Fast and accurate inference of Gene Regulatory Networks through robust precision matrix estimation
>> https://academic.oup.com/bioinformatics/advance-article/doi/10.1093/bioinformatics/btac178/6553011
The possible cell transcriptional states are determined by the underlying Gene Regulatory Network (GRN), and reliably inferring such network would be invaluable to understand biological processes and disease progression.
PORTIA, a novel algorithm for GRN inference based on power transforms and covariance matrix inversion. A key aspect of GRN inference is the need to disentangle direct from indirect correlations. PORTIA has thus been conceptually inspired by Direct Coupling Analysis methods.

□ CAISC: A software to integrate copy number variations and single nucleotide mutations for genetic heterogeneity profiling and subclone detection by single-cell RNA sequencing
>> https://bmcbioinformatics.biomedcentral.com/articles/10.1186/s12859-022-04625-x
Clonal Architecture with Integration of SNV and CNV (CAISC), an R package for scRNA-seq data analysis that clusters single cells into distinct subclones by integrating CNV and SNV genotype matrices using an entropy weighted approach.
Entropy measures the structural complexity of a network, thus its concept can be utilized to integrate multiple weighted graphs or networks, or in this case, to integrate the cell–cell distance matrices generated by the DENDRO and infercnv analyses.

□ Haplotype-resolved assembly of diploid genomes without parental data
>> https://www.nature.com/articles/s41587-022-01261-x
An algorithm that combines PacBio HiFi reads and Hi-C chromatin interaction data to produce a haplotype-resolved assembly without the sequencing of parents.
the algorithm consistently outperforms existing single-sample assembly pipelines and generates assemblies of similar quality to the best pedigree-based assemblies.
It reduce unitig bipartition to a graph max-cut problem and find a near optimal solution with a stochastic algorithm in the principle of simulated annealing,and also consider the topology of the assembly graph to reduce the chance of local optima.

□ Gfastats: conversion, evaluation and manipulation of genome sequences using assembly graphs
>> https://www.biorxiv.org/content/10.1101/2022.03.24.485682v1.full.pdf
Gfastats is a standalone tool to compute assembly summary statistics and manipulate assembly sequences in fasta, fastq, or gfa [.gz] format. Gfastats stores assembly sequences internally in a gfa-like format.
Gfastats builds a bidirected graph representation of the assembly using adjacency lists, where each node is a segment, and each edge is a gap. Walking the graph allows to generate different kinds of outputs, including manipulated assemblies and feature coordinates.

□ SEACells: Inference of transcriptional and epigenomic cellular states from single-cell genomics data
>> https://www.biorxiv.org/content/10.1101/2022.04.02.486748v1.full.pdf
SEACells outperforms existing algorithms in identifying accurate, compact, and well-separated metacells in both RNA and ATAC modalities across datasets with discrete cell types and continuous trajectories.
SEACells improves gene-peak associations, computes ATAC gene scores and measures gene accessibility. Using a count matrix as input, it provides per-cell weights for each metacell, per-cell hard assignments to each metacell, and the aggregated counts for each metacell as output.

□ Accelerated identification of disease-causing variants with ultra-rapid nanopore genome sequencing
>> https://www.nature.com/articles/s41587-022-01221-5
An approach for ultra-rapid nanopore WGS that combines an optimized sample preparation protocol, distributing sequencing over 48 flow cells, near real-time base calling and alignment, accelerated variant calling and fast variant filtration.
This cloud-based pipeline scales compute-intensive base calling and alignment across 16 instances with 4× Tesla V100 GPUs each and runs concurrently. It aims for maximum resource utilization, where base calling using Guppy runs on GPU and alignment using Minimap2.

□ PEER: Transcriptome diversity is a systematic source of variation in RNA-sequencing data
>> https://journals.plos.org/ploscompbiol/article?id=10.1371/journal.pcbi.1009939
Probabilistic estimation of expression residuals (PEER), which infers broad variance components in gene expression measurements, has been used to account for some systematic effects, but it has remained challenging to interpret these PEER factors.
PEER “hidden” covariates encode for transcriptome diversity – a simple metric based on Shannon entropy – explains a large portion of variability in gene expression and is the strongest known factor encoded in PEER factors.

□ DeepAcr: Predicting Anti-CRISPR with Deep Learning
>> https://www.biorxiv.org/content/10.1101/2022.04.02.486820v1.full.pdf
DeepAcr compiles the large protein sequence database to obtain secondary structure, relative solvent accessibility, evolutionary features, and Transformer features with RaptorX,.
DeepAcr applies Hidden Markov Model and uses it a baseline for Acr classification comparison. It outperforms macro-average metrics. Thus, DeepAcr is an unbiased predictor. DeepAcr captures the evolutionarily conserved pattern and the interaction between anti-CRISPR.

□ RecGen: Prediction of designer-recombinases for DNA editing with generative deep learning
>> https://www.biorxiv.org/content/10.1101/2022.04.01.486669v1.full.pdf
RecGen, an algorithm for the intelligent generation of designer-recombinases. RecGen is trained with 89 evolved recombinase libraries and their respective target sites, captures the affinities between the recombinase sequences and their respective DNA binding sequences.
RecGen uses CVAE (Conditional Variational Autoencoders) architecture for recombinase prediction. The latent space is designed to resemble a multivariate normal distribution. For each latent space dimension mean and standard deviation are learned for normal distribution sampling.

□ BiTSC2: Bayesian inference of tumor clonal tree by joint analysis of single-cell SNV and CNA data
>> https://academic.oup.com/bib/advance-article/doi/10.1093/bib/bbac092/6562684
BiTSC2 takes raw reads from scDNA-seq as input, accounts for the overlapping of CNA and SNV, models allelic dropout rate, sequencing errors and missing rate, as well as assigns single cells into subclones.
By applying Markov Chain Monte Carlo sampling, BiTSC2 can simultaneously estimate the subclonal scCNA and scSNV genotype matrices. BiTSC2 shows high accuracy in genotype recovery, subclonal assignment and tree reconstruction.

□ LSMMD-MA: Scaling multimodal data integration for single-cell genomics data analysis
>> https://www.biorxiv.org/content/10.1101/2022.03.23.485536v1.full.pdf
MMD-MA is a method for analyzing multimodal data that relies on mapping the observed cell samples to embeddings, using functions belonging to a Reproducing Kernel Hilbert Space.
LSMMD-MA, a large-scale Python implementation of the MMD-MA method for multimodal data integration. Reformulating the MMD-MA optimization problem using linear algebra and solve it with KeOps, a CUDA framework for symbolic matrix computation.

□ CNETML: Maximum likelihood inference of phylogeny from copy number profiles of spatio-temporal samples
>> https://www.biorxiv.org/content/10.1101/2022.03.18.484889v1.full.pdf
CNETML, a new maximum likelihood method based on a novel evolutionary model of copy number alterations (CNAs) to infer phylogenies from spatio-temporal samples taken within a single patient.
CNETML is the first program to jointly infer the tree topology, node ages, and mutation rates from total copy numbers when samples were taken at different time points. The change of copy number at each site follows a continuous-time non-reversible Markov chain.

□ BISER: Fast characterization of segmental duplication structure in multiple genome assemblies
>> https://almob.biomedcentral.com/articles/10.1186/s13015-022-00210-2
BISER (Brisk Inference of Segmental duplication Evolutionary stRucture) is a fast tool for detecting and decomposing segmental duplications in genome assemblies. BISER infers elementary and core duplicons and enable an evolutionary analysis of all SDs in a given set of genomes.
BISER uses a two-tiered local chaining algorithm from SEDEF based on a seed-and-extend approach and efficient O(nlogn) chaining method following by a SIMD-parallelized sparse dynamic programming algorithm to calculate the boundaries of the final SD regions and their alignments.

□ NIFA: Non-negative Independent Factor Analysis disentangles discrete and continuous sources of variation in scRNA-seq data
>> https://academic.oup.com/bioinformatics/advance-article-abstract/doi/10.1093/bioinformatics/btac136/6550501
NIFA (Non-negative Independent Factor Analysis), a new probabilistic single-cell factor analysis model that incorporates different interpretability inducing assumptions into a single modeling framework.
NIFA models uni- and multi-modal latent factors, and isolates discrete cell-type identity and continuous pathway activity into separate components. NIFA-derived factors outperform results from ICA, PCA, NMF and scCoGAPS in terms of disentangling biological sources of variation.

□ Coverage-preserving sparsification of overlap graphs for long-read assembly
>> https://www.biorxiv.org/content/10.1101/2022.03.17.484715v1.full.pdf
Accordingly, problem formulations for genome assembly which seek a single genome reconstruction, e.g., by finding a Hamiltonian cycle in an overlap graph, or computing the shortest common superstring of input reads, are not used in practice.
A novel theoretical framework that computes a directed multi-graph structure which is also a sub-graph of overlap graph, and it is guaranteed to be coverage-preserving.
The safe graph sparsification rules for vertex and edge removal from overlap graph Ok(R), k ≤ l2 which guarantee that all circular strings ∈ C(R, l1, l2, φ) can be spelled in the sparse graph.

□ Quantum algorithmic randomness
>> https://arxiv.org/pdf/2008.03584.pdf
Quantum Martin-Lo ̈f randomness (q-MLR) for infinite qubit sequences was introduced. Defining a notion of quantum Solovay randomness which is equivalent to q-MLR. The proof of this goes through a purely linear algebraic result about approximating density matrices by subspaces.
Quantum-K is intended to be a quantum version of K, the prefix-free Kolmogorov complexity. Weak Solovay random states have a characterization in terms of the incompressibility of their initial segments. ρ is weak Solovay random ⇐⇒ ∀ε > 0, limn QKε(ρn) − n = ∞.

□ mm2-ax: Accelerating Minimap2 for accurate long read alignment on GPUs
>> https://www.biorxiv.org/content/10.1101/2022.03.09.483575v1.full.pdf
Chaining in mm2 identifies optimal collinear ordered subsets of anchors from the input sorted list of anchors. mm2 does a sequential pass over all the predecessors and does sequential score comparisons to identify the best scoring predecessor for every anchor.
mm2-ax (minimap2-accelerated), a heterogeneous software- hardware co-design for accelerating the chaining step of minimap2. It extracts better intra-read parallelism from chaining without loosing mapping accuracy by forward transforming Minimap2’s chaining algorithm.
mm2-ax demonstrates a 12.6-5X Speedup and 9.44-3.77X Speedup:Costup over SIMD-vectorized mm2-fast baseline. mm2-ax converts a sparse vector which defines the chaining workload to a dense one in order to optimize for better arithmetic intensity.

□ scINSIGHT for interpreting single-cell gene expression from biologically heterogeneous data
>> https://genomebiology.biomedcentral.com/articles/10.1186/s13059-022-02649-3
Based on a novel matrix factorization model, scINSIGHT learns coordinated gene expression patterns that are common among or specific to different biological conditions, offering a unique chance to jointly identify heterogeneous biological processes and diverse cell types.
scINSIGHT achieves sparse, interpretable, and biologically meaningful decomposition. scINSIGHT simultaneously identifies common and condition-specific gene modules and quantify their expression levels in each sample in a lower-dimensional space.

□ Gradient-k: Improving the performance of K-Means using the density gradient
>> https://www.biorxiv.org/content/10.1101/2022.03.30.486343v1.full.pdf
Gradient-k reduces the number of iterations required for convergence. This is achieved by correcting the distance used in the k-means algorithm by a factor based on the angle between the density gradient and the direction to the cluster center.
Gradient-k uses auxiliary information about how the data is distributed in space, enabling it to detect clusters regardless of their density, shape, and size. Gradient-k allows non-linear splits, can find clusters of non-Gaussian shapes, and has a reduced tessellation behavior.

□ Multigrate: single-cell multi-omic data integration
>> https://www.biorxiv.org/content/10.1101/2022.03.16.484643v1.full.pdf
Multigrate equipped with transfer learning enables mapping a query multimodal dataset into an existing reference atlas.
Multigrate learns a joint latent space combining information from multiple modalities from paired and unpaired measurements while accounting for technical biases within each modality.

□ Gapless provides combined scaffolding, gap filling and assembly correction with long reads
>> https://www.biorxiv.org/content/10.1101/2022.03.08.483466v1.full.pdf
The included assembly correction can remove errors in the initial assembly that are highlighted by the long-reads. The necessary mapping and consensus calling are performed with minimap2 and racon, but this can be quickly changed in the short accompanying bash script.
The scaffold module is the core of gapless. It requires the split assembly to extract the names and length of existing scaffolds, the alignment of the split assembly to itself to detect repeats and the alignment of the long reads to the split assembly.
The long read alignments are initially filtered, requiring a minimum mapping quality and alignment length, and in case of PacBio, only one subread per fragment is kept to avoid giving large weight to short DNA fragments that are repeatedly sequenced multiple times.

□ DiSCERN - Deep Single Cell Expression ReconstructioN for improved cell clustering and cell subtype and state detection
>> https://www.biorxiv.org/content/10.1101/2022.03.09.483600v1.full.pdf
DISCERN is based on a modified Wasserstein Autoencoder. DISCERN allows for the realistic reconstruction of gene expression information by transferring the style of hq data onto lq data, in latent and gene space.
DISCERN transfers the “style” of hq onto lq data to reconstruct missing gene expression, which operate in a lower dimensional representation. DISCERN models GE values realistically while retaining prior and vital biological information of the lq dataset after reconstruction.

□ DNA co-methylation has a stable structure and is related to specific aspects of genome regulation
>> https://www.biorxiv.org/content/10.1101/2022.03.16.484648v1.full.pdf
Highly correlated DNAm sites in close proximity are highly heritable, influenced by nearby genetic variants (cis mQTLs), and are enriched for transcription factor binding sites related to regulation of short RNAs essential for cellular function transcribed by RNA polymerase III.
DNA co-methylation of distant sites may be related to long-range cooperative TF interactions. Highly correlated sites that are either distant, or on different chromosomes, are driven by unique environmental factors, and methylation is less likely to be driven by genotype.

□ Element Biosciences
>> https://www.elementbiosciences.com/products/aviti
High data quality and throughput enable whole genome sequencing for rare disease. Our study with UCSD is the first of its kind to demonstrate the clinical potential of #AVITI System on previously unsolved cases.
#NGS #AviditySequencing
Comparative analysis shows Loopseq has the lowest error rate of all commercially available long read sequencing technologies.
>> https://www.elementbiosciences.com/news/element-launches-the-aviti-system-to-democratize-access-to-genomics
□ Jim Tananbaum
I'm excited to support the team at @ElemBio as they unveil their benchtop sequencer AVITI. I believe sequencing will touch all our lives. To enable it, we need high quality, inexpensive sequencing.