
“想うより疾く 願うより強く”
私たちはコンテクストである。
如何なる別れ途を経ても、辿った道のりの果てに己はある。
手繰り寄せてきた糸は全て途絶えているわけでなく、遥か遠くの一点へと収束している。
哀しみを負わされた意図を計りたいのなら、忘れてはならない。
ここで為されたこと為すことの総ては、先にある己の墓標に刻まれているのだ。

□ ProteinNet: a standardized data set for machine learning of protein structure
>> https://arxiv.org/abs/1902.00249
ProteinNet integrates sequence, structure, and evolutionary information in programmatically accessible file formats tailored for machine learning frameworks. Multiple sequence alignments of all structurally characterized proteins were created using substantial high-performance computing resources. Standardized data splits were also generated to emulate the difficulty of past CASP (Critical Assessment of protein Structure Prediction) experiments by resetting protein sequence and structure space to the historical states that preceded six prior CASPs.

□ Pyramid Model: A general framework for moment-based analysis of genetic data:
>> https://link.springer.com/article/10.1007/s00285-018-01325-0
a novel Hierarchical Beta approximation is developed, a Pyramidal Hierarchical Beta model, for the generalized time-reversible and single-step mutation processes.
the validity of the Dirichlet distribution has never been systematically investigated in a general framework. Attempt to address this problem by providing a general overview of how allele fraction data under the most common multi-allelic mutational structures should be modeled. The Dirichlet and alternative models are investigated by simulating allele fractions from a diffusion approximation of the multi-allelic Wright–Fisher process with mutation, and applying a moment-based analysis method.
The Dirichlet model is only an exceptionally good approximation for the pure drift, Jukes–Cantor and parent-independent mutation processes with small mutation rates. Alternative models are required and proposed for the other mutation processes, such as a Beta–Dirichlet model for the infinite alleles mutation process, and a Hierarchical Beta model.
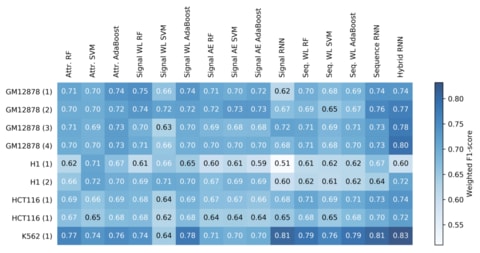
□ ATAC-seq signal processing and recurrent neural networks can identify RNA polymerase activity:
>> https://www.biorxiv.org/content/biorxiv/early/2019/01/26/531517.full.pdf
a frequency space analysis of the “signal” generated from ATAC-seq experiments’ short reads, at a single-nucleotide resolution, using a discrete wavelet transform. analyzing the performance of different data encoding schemes and machine learning architectures, and show how a hybrid signal/sequence representation classified using recurrent neural networks (RNNs) yields the best performance across different cell types.
□ From expression footprints to causal pathways: contextualizing large signaling networks with CARNIVAL:
>> https://www.biorxiv.org/content/biorxiv/early/2019/02/05/541888.full.pdf
CARNIVAL (CAusal Reasoning pipeline for Network identification using Integer VALue programming) integrates different sources of prior knowledge, including signed and directed protein-protein interactions, transcription factor targets, and pathway signatures. CARNIVAL allows the capture of a broad set of upstream cellular processes & regulators, which in turn delivered results w/ higher accuracy when benchmarked against related tools. Implementation as an integer linear programming (ILP) problem also guarantees efficient computation.
□ VEF: a Variant Filtering tool based on Ensemble methods:
>> https://www.biorxiv.org/content/biorxiv/early/2019/02/05/540286.full.pdf
VEF, a novel filtering tool based on supervised learning. In particular, VEF trains a Random Forest (RF) on a variant call set from a sample for which a high-confidence set of “true” variants (i.e., a ground truth of gold standard) is available. VEF generalizes well, in that it can be trained and applied to VCF files generated from data of different coverages, as well as data produced by different sequencing machines.
□ Fast and scalable genome-wide inference of local tree topologies from large number of haplotypes based on tree consistent PBWT data structure:
>> https://www.biorxiv.org/content/biorxiv/early/2019/02/06/542035.full.pdf
an alternative form of the positional Burrows-Wheeler transform (PBWT), which they call the “tree consistent PBWT ” or shortly tcPBWT. the tcPBWT algorithm will find the correct topology of the tree in case of the perfect phylogeny (without recombinations, and with at most one mutation at each site). tcPBWT method scales linearly both in the number and in the length of sequence, and these tree topologies can capture both global population structure and local tree structure.
□ CKN-seq: Biological Sequence Modeling with Convolutional Kernel Networks:
>> https://academic.oup.com/bioinformatics/advance-article-abstract/doi/10.1093/bioinformatics/btz094/5308597
CKN-seq is a hybrid approach between convolutional neural networks and kernel methods to model biological sequences. This method enjoys the ability of convolutional neural networks to learn data representations that are adapted to a specific task, while the kernel point of view yields algorithms.
□ Two-Tier Mapper, an unbiased topology-based clustering method for enhanced global gene expression analysis:
>> https://academic.oup.com/bioinformatics/advance-article-abstract/doi/10.1093/bioinformatics/btz052/5308599
TTMap discerns divergent features in the control group, adjusts for them, and identifies outliers. the deviation of each test sample from the control group in a high-dimensional space is computed, and the test samples are clustered using a new Mapper-based topological algorithm at two levels: a global tier and local tiers.

□ DeepPVP: phenotype-based prioritization of causative variants using deep learning:
>> https://bmcbioinformatics.biomedcentral.com/articles/10.1186/s12859-019-2633-8
an extension of the PhenomeNET Variant Predictor (PVP) system which uses deep learning & achieves significantly better performance in predicting disease-associated variants than the previous PVP, as well as competing algorithms that combine pathogenicity and phenotype similarity. DeepPVP not only uses a deep artificial neural network to classify variants into causative and non-causative but also corrects for a common bias in variant prioritization methods in which gene-based features are repeated and potentially lead to overfitting.

□ scGEApp: a Matlab app for feature selection on single-cell RNA sequencing data:
>> https://www.biorxiv.org/content/biorxiv/early/2019/02/08/544163.full.pdf
This method can be applied to single-sample or two-sample scRNA-seq data, identify feature genes, e.g., those with unexpectedly high CV for given μ and rdrop of those genes, or genes with the most feature changes. Users can operate scGEApp through GUIs to use the full spectrum of functions including normalization, batch effect correction, imputation, visualization, feature selection, and downstream analyses with GSEA and GOrilla.
□ The universal decay of collective memory and attention
>> https://www.nature.com/articles/s41562-018-0474-5
once we isolate the temporal dimension of the decay, the attention received by cultural products decays following a universal biexponential function. explain this universality by proposing a mathematical model based on communicative and cultural memory, which fits the data better than previously proposed log-normal and exponential models.
□ SwiftOrtho: a Fast, Memory-Efficient, Multiple Genome Orthology Classifier:
>> https://www.biorxiv.org/content/biorxiv/early/2019/02/08/543223.full.pdf
SwiftOrtho is orthology analysis tool which identifies orthologs, paralogs and co-orthologs for genomes. It is a graph-based approach. SwiftOrtho employs a seed-and-extension algorithm to find homologous gene pairs. At the extension phase, SwiftOrtho uses a variation of the Smith-Waterman algorithm, the k-banded Smith-Waterman or k-SWAT, which only allows for k gaps. k-SWAT fills a band of cells along the main diagonal of the similarity score matrix, and the complexity of k-swat is reduced to O(k · min(n, m)), where k is the maximum allowed number of gaps.
□ Adaboost-SVM-based probability algorithm for the prediction of all mature miRNA sites based on structured-sequence features:
>> https://www.nature.com/articles/s41598-018-38048-7
a computational method, matFinder, that uses an AdaBoost-SVM algorithm to predict all the process sites of the mature miRNA in a pre-miRNA transcript. the AdaBoost-SVM algorithm was used to construct the classifier. The classifier training process focuses on incorrectly classified samples, and the integrated results use the common decision strategies of the weak classifier with different weights.

□ Constrained incremental tree building: new absolute fast converging phylogeny estimation methods with improved scalability and accuracy:
>> https://almob.biomedcentral.com/articles/10.1186/s13015-019-0136-9
a new approach to large-scale phylogeny estimation that shares some of the features of DCMNJ but bypasses the use of supertree methods. this new approach is Absolute Fast Converging (AFC) and uses polynomial time and space. maximum likelihood (if solved exactly) is AFC under the standard sequence evolution models, and although it is NP-hard to solve exactly there are many seemingly good heuristics for maximum likelihood (e.g., RAxML).

□ HiGlass: web-based visual exploration and analysis of genome interaction maps:
>> https://genomebiology.biomedcentral.com/articles/10.1186/s13059-018-1486-1
Projects such as ENCODE and 4D Nucleome are generating Hi-C data, annotating it with metadata, and making them available to the broader public. However, there is a need to make it easier for researchers to find and integrate the data that helps answer their biological questions. HiGlass provides a rich interface for rapid, multiplex, and multiscale navigation of 2D genomic maps alongside 1D genomic tracks, allowing users to combine various data types, synchronize multiple visualization modalities, and share fully customizable views with others.
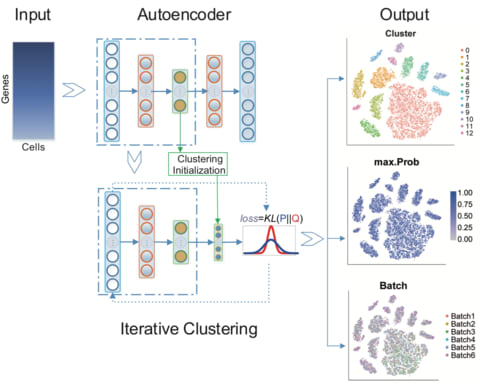
□ DESC: Deep learning enables accurate clustering and batch effect removal in single-cell RNA-seq analysis:
>> https://www.biorxiv.org/content/biorxiv/early/2019/01/25/530378.full.pdf
an unsupervised deep embedding algorithm for single-cell clustering (DESC) that iteratively learns cluster-specific gene expression signatures and cluster assignment. DESC significantly improves clustering accuracy across various datasets and is capable of removing complex batch effects while maintaining true biological variations.

□ DeepMNE-CNN: Integrating multi-network topology for gene function prediction using deep neural networks:
>> https://www.biorxiv.org/content/biorxiv/early/2019/01/29/532408.full.pdf
DeepMNE-CNN utilizes a convolutional neural network based on the integrated feature embedding to annotate unlabeled gene functions. DeepMNE-CNN mainly contains two components. One component is multi-networks integration framework, which applies a novel semi-supervised autoencoder to map input networks into a low-dimension and non-linear space based on prior information constraints. The other is CNN-based function predictor, which use convolutional neural network to learn feature embedding.
□ DeePaC: Predicting pathogenic potential of novel DNA with a universal framework for reverse-complement neural networks:
>> https://www.biorxiv.org/content/biorxiv/early/2019/01/31/535286.1.full.pdf
The universal framework for reverse-complement neural networks enables transformation of traditional deep learning architectures into their RC-counterparts, guaranteeing consistent predictions for any given DNA sequence, regardless of its orientation.

□ MuSiC: Bulk tissue cell type deconvolution with multi-subject single-cell expression reference:
>> https://www.nature.com/articles/s41467-018-08023-x
By appropriate weighting of genes showing cross-subject and cross-cell consistency, MuSiC enables the transfer of cell type-specific gene expression information from one dataset to another. MuSiC is a weighted non-negative least squares regression (W-NNLS), which does not require pre-selected marker genes. The iterative estimation procedure automatically imposes more weight on informative genes and less weight on non-informative genes.
□ C1 REAP-seq: Fluidigm Introduces REAP-Seq for Multi-Omic Single-Cell Analysis on the C1
>> https://globenewswire.com/news-release/2019/01/31/1708388/0/en/Fluidigm-Introduces-REAP-Seq-for-Multi-Omic-Single-Cell-Analysis-on-the-C1.html
□ EcoRI: Restriction enzymes use a 24 dimensional coding space to recognize 6 base long DNA sequences:
>> https://www.biorxiv.org/content/biorxiv/early/2019/02/01/538025.full.pdf
Information theory represents messages as spheres in high dimensional spaces. Better sphere packing leads to better communications systems. The densest known packing of hyperspheres occurs on the Leech lattice in 24 dimensions.

□ Laser light can contain intricate, beautiful fractals: Despite their simplicity, certain lasers can create the complex patterns
>> https://journals.aps.org/pra/abstract/10.1103/PhysRevA.99.013848
advance the existing theory of fractal laser modes, first by predicting three-dimensional self-similar fractal structure around the center of the magnified self-conjugate plane and second by showing, quantitatively, that intensity cross sections are most self-similar in the magnified self-conjugate plane.

□ Parametric and non-parametric gradient matching for network inference: a comparison:
>> https://bmcbioinformatics.biomedcentral.com/articles/10.1186/s12859-018-2590-7
In order to avoid the computational cost of large-scale simulations, a two-step Gaussian process interpolation based gradient matching approach has been proposed to solve differential equations approximately. They use model averaging, based on the Bayesian Information Criterion (BIC), to combine the different inferences. The performance of different inference approaches is evaluated using area under the precision-recall curves.
□ PROSSTT: probabilistic simulation of single-cell RNA-seq data for complex differentiation processes:
>> https://academic.oup.com/bioinformatics/advance-article/doi/10.1093/bioinformatics/btz078/5305637
PROSSTT (PRObabilistic Simulations of ScRNA-seq Tree-like Topologies) is a package with code for the simulation of scRNAseq data for dynamic processes such as cell differentiation. PROSSTT can simulate scRNA-seq datasets for differentiation processes with lineage trees of any desired complexity, noise level, noise model, and size. PROSSTT also provides scripts to quantify the quality of predicted lineage trees.
□ Two-step graph mapper: Assessing graph-based read mappers against a novel baseline approach highlights strengths and weaknesses of the current generation of methods:
>> https://www.biorxiv.org/content/biorxiv/early/2019/02/01/538066.full.pdf
using the initial graph alignments to predict a linear path through the graph, and then re-aligning all the reads to this linear path using the linear mapper increases mapping accuracy. although the path-estimation in the first step of the two-step approach implicitly estimates variants present in the graph, the intention of this step is not to do variant calling – instead variant calling can be performed as a follow-up step based on the aligned reads.
□ Assembly Graph Browser: interactive visualization of assembly graphs:
>> https://academic.oup.com/bioinformatics/advance-article-abstract/doi/10.1093/bioinformatics/btz072/5306331
AGB includes a number of novel functions including repeat analysis, construction of the contracted assembly graphs (i.e., the graphs obtained by collapsing a selected set of edges). AGB uses d3-graphviz, GfaPy, NetworkX-METIS, and QUAST-LG. AGB visualizes the assembly graph produced by an assembler, where edges represent various genome segments (each genome segment is represented by its forward and reverse-complement edge).

□ Network hubs affect evolvability: how alterations in a gene central to a network affect evolutionary processes:
>> https://journals.plos.org/plosbiology/article?id=10.1371/journal.pbio.3000111
Fitness landscape and possible evolutionary trajectories. Perturbing a hub gene or a peripheral gene can both lead to a decrease in fitness, but the number of available evolutionary trajectories is higher when a hub gene is perturbed. adaptation to an altered hub occurred by optimizing the subnetworks the hub is connected to and not by restoring the hub itself. These subnetworks were different between the populations, and as a result, the evolved lineages showed a large variety in their phenotypic profiles.

□ Detecting anomalies in RNA-seq quantification:
>> https://www.biorxiv.org/content/biorxiv/early/2019/02/05/541714.full.pdf
a method to attribute the cause of anomalies to either the incompleteness of the reference transcriptome or the algorithmic mistakes, and this method precisely detects misquantifications with both causes. Applying anomaly detection to 30 GEUVADIS and 16 Human Body Map samples, they detect 103 genes with potential unannotated isoforms.

□ Performance of neural network basecalling tools for Oxford Nanopore sequencing:
>> https://www.biorxiv.org/content/biorxiv/early/2019/02/07/543439.full.pdf
Albacore, Guppy and Scrappie all use an architecture that ONT calls RGRGR – named after its alternating reverse-GRU and GRU layers. To test whether more complex networks perform better, they modified ONT’s RGRGR network by widening the convolutional layer and doubling the hidden layer size.
□ Waddington-OT: Optimal-Transport Analysis of Single-Cell Gene Expression Identifies Developmental Trajectories in Reprogramming:
>> https://www.cell.com/cell/fulltext/S0092-8674(19)30039-X
Waddington-OT, an approach for studying developmental time courses to infer ancestor-descendant fates and model the regulatory programs that underlie them. applying the method to reconstruct the landscape of reprogramming from 315,000 single-cell RNA sequencing (scRNA-seq) profiles, collected at half-day intervals across 18 days.
□ Coordinate-based mapping of tabular data enables fast and scalable queries:
>> https://www.biorxiv.org/content/biorxiv/early/2019/01/31/536979.full.pdf
Across the subfields of biology, researchers store a considerable proportion of tabular data in plain-text formats. This approach coincides with the Unix and “Pragmatic Programming” philosophies, which advocate for storing data and sharing data among computer programs as plain text.
The HDF5 format is designed primarily for numerical data, whereas we sought the ability to handle other data types as well. As a columnar storage solution, Parquet was efficient at projection.
□ Formal axioms in biomedical ontologies improve analysis and interpretation of associated data:
>> https://www.biorxiv.org/content/biorxiv/early/2019/02/02/536649.full.pdf
The axioms and meta-data of different ontologies contribute in varying degrees to improving data analysis. the formal axioms that have been created for the Gene Ontology and several other ontologies significantly improve ontology-based prediction models through provision of domain-specific background knowledge.

□ Biomedical Concept Recognition Using Deep Neural Sequence Models:
>> https://www.biorxiv.org/content/biorxiv/early/2019/01/25/530337.full.pdf
Deep learning methods for span detection were equivalent in performance to traditional conditional random field methods. As natural training data is limited to the concepts used in CRAFT annotations, the addition of synthetic training data, class names and synonyms, to the normalization step has the potential to improve recall on class not in CRAFT. The CRF+OpenNMT system also outperforms the other systems for most ontologies and is the best-performing system for the GO_BP/MF annotation set.
□ ComPath: an ecosystem for exploring, analyzing, and curating mappings across pathway databases:
>> https://www.nature.com/articles/s41540-018-0078-8
Although the absence of topological pathway information in ComPath is an irrefutable limitation in this study, gene-centric approaches enable a reduction of complexity in pathway comparison as well as integration of resources which do not provide topology information.
□ ChimeraUGEM: unsupervised gene expression modeling in any given organism:
>> https://academic.oup.com/bioinformatics/advance-article-abstract/doi/10.1093/bioinformatics/btz080/5305634
ChimeraUGEM provides tools for the analysis of gene sequences (coding and non-coding), as well as the design of protein coding sequences for optimized expression, based on the Chimera algorithms and codon usage optimization.

□ netNMF-sc: Leveraging gene-gene interactions for imputation and dimensionality reduction in single-cell expression analysis:
>> https://www.biorxiv.org/content/biorxiv/early/2019/02/08/544346.full.pdf
netNMF-sc combines network-regularized non-negative matrix factoriza- tion with a procedure for handling zero inflation in transcript count matrices. The matrix factorization results in a low-dimensional representation of the transcript count matrix, which imputes gene abundance for both zero and non-zero entries and can be used to cluster cells.
□ Spinning convincing stories for both true and false association signals
>> https://onlinelibrary.wiley.com/doi/full/10.1002/gepi.22189
“spinning a convincing story appears to be easy for any region in the genome, whether or not there is a true signal there.”
□ Lena Hall:
>> https://twitter.com/lenadroid/status/1089339284736229376
What are some of the additional applications of Practical Byzantine Fault Tolerance outside of the most obvious use in blockchain?
There're many challenges with their practical implementation in real-world systems, but there're many promising scenarios where it'd be extremely beneficial, starting with flight control/spacecraft flight systems, and other systems that need agreement and expect Byzantine errors.
□ Mesh: Compacting Memory Management for C/C++ Applications
>> https://arxiv.org/abs/1902.04738
Mesh combines novel randomized algorithms with widely-supported virtual memory operations to provably reduce fragmentation, breaking the classical Robson bounds with high probability.
□ Transcript expression-aware annotation improves rare variant discovery and interpretation
>> https://www.biorxiv.org/content/10.1101/554444v1
In gnomAD we see variants we don't expect (e.g. in haploinsufficient disease genes). Often found on alternative txs, with little evidence of expression. he pext score, which summarizes isoform expression for variants. Regions with high pext are more conserved, and nonsynonymous variation on them is more deleterious. Opposite true for low pext regions, which are enriched for false exon annotations.
□ Enrichment with Mathematical Biology (GEMB):
>> https://www.biorxiv.org/content/biorxiv/early/2019/02/18/554212.full.pdf
Mathematical models of biology can predict the relative contribution of a gene to a specific function of a pathway. The method combines gene weights from model predictions and gene ranks from genome-wide association studies into a weighted gene-set test.

□ clonealign assigns single-cell RNA-seq expression to clones by probabilistically mapping RNA-seq to clone-specific copy number profiles using reparametrization gradient variational inference:
>> https://github.com/kieranrcampbell/clonealign
clonealign is particularly useful when clones have been inferred using ultra-shallow single-cell DNA-seq meaning SNV analysis is not possible.
※コメント投稿者のブログIDはブログ作成者のみに通知されます