
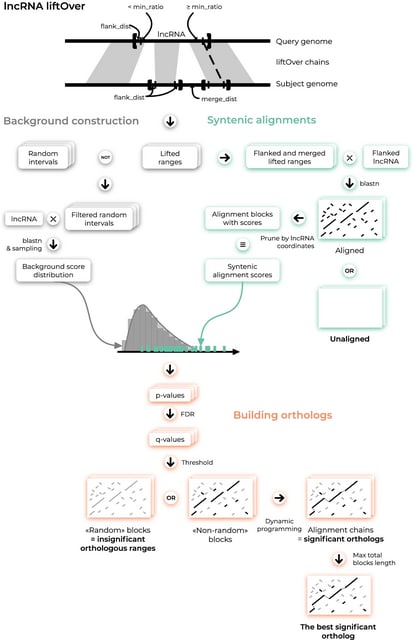
□ ortho2align: a sensitive approach for searching for orthologues of novel lncRNAs
>> https://bmcbioinformatics.biomedcentral.com/articles/10.1186/s12859-022-04929-y
ortho2align, a synteny-based approach for finding orthologues of novel lncRNAs with a statistical assessment of sequence conservation. ortho2align is in fact a versatile tool applicable to any genomic regions, especially weakly conserved ones, not just lncRNAs.
Implemented strategies of restricting the search to syntenic regions, statistical filtering of HSPs and selection of orthologues provide high levels of sensitivity and specificity as well as optimal computational time even when looking for orthologues in distant species.
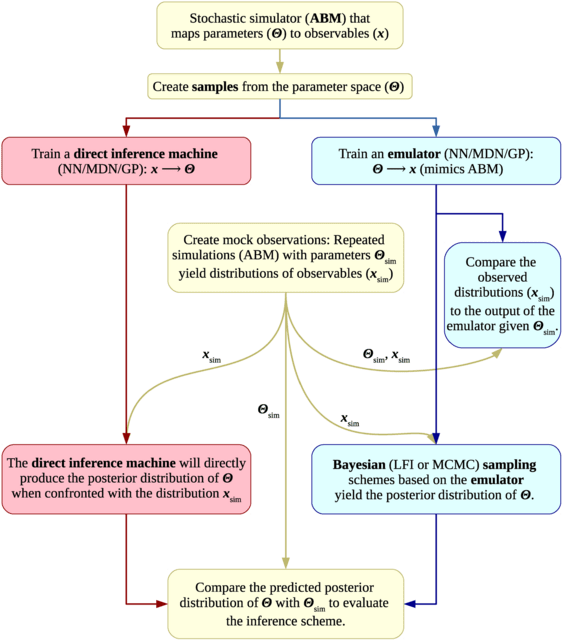
□ Efficient Bayesian inference for stochastic agent-based models
>> https://journals.plos.org/ploscompbiol/article?id=10.1371/journal.pcbi.1009508
Using two agent-based models (ABMs) describing two distinct real-world problems: The first model deals with a malignant type of brain cancer called glioblastoma multiforme. The second model describes the spread of infectious diseases in a population.
Employing three different emulators: a deep neural network (NN), a mixture density network (MDN), and Gaussian processes (GP). These methods were chosen because they can mimic the stochastic nature of the ABMs

□ MultiVelo: Multi-omic single-cell velocity models epigenome-transcriptome interactions and improves cell fate prediction
>> https://www.nature.com/articles/s41587-022-01476-y
MultiVelo uses a probabilistic latent variable model to estimate the switch time and rate parameters of gene regulation, providing a quantitative summary of the temporal relationship between epigenomic and transcriptomic changes.
MultiVelo accurately recovers cell lineages and quantifies the length of priming and decoupling intervals in which chromatin accessibility and gene expression are temporarily out of sync.
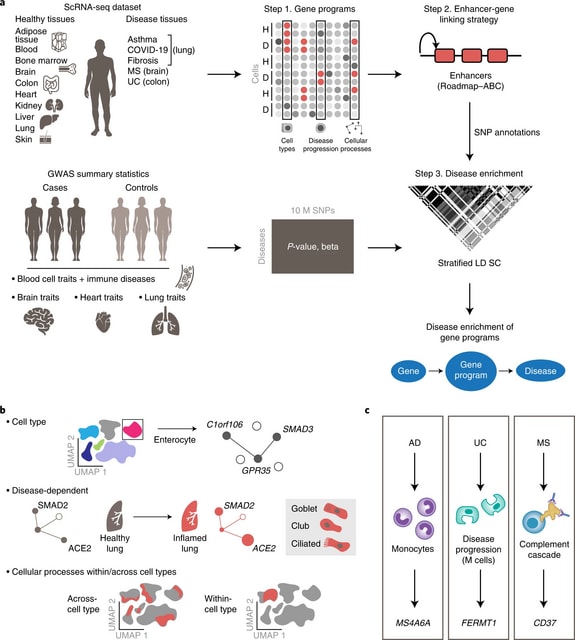
□ sc-linker: Identifying disease-critical cell types and cellular processes by integrating single-cell RNA-sequencing and human genetics
>> https://www.nature.com/articles/s41588-022-01187-9
sc-linker, an integrated framework to relate human disease and complex traits to cell types and cellular processes by integrating GWAS summary statistics, epigenomics and scRNA-seq data from multiple tissue types, diseases, individuals and cells.
sc-linker links the genes underlying these programs to SNPs that regulate them by incorporating two tissue-specific, enhancer–gene-linking strategies: Roadmap Enhancer-Gene Linking and the Activity-by-Contact (ABC) model.
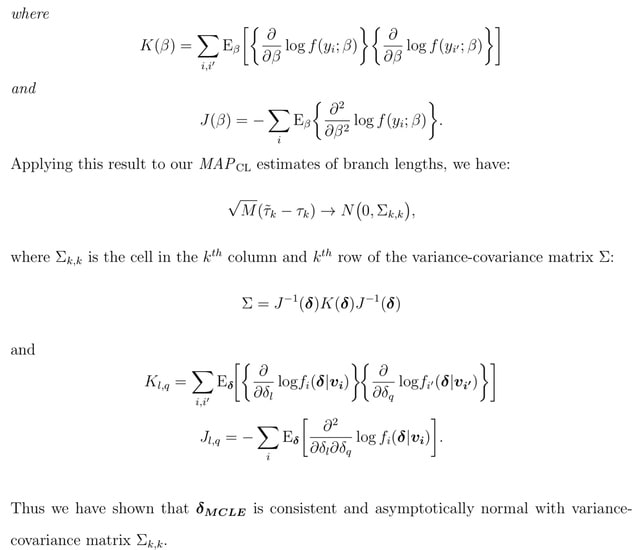
□ MAPCL: Estimation of Speciation Times Under the Multispecies Coalescent
>> https://academic.oup.com/bioinformatics/advance-article-abstract/doi/10.1093/bioinformatics/btac679/6760259
A maximum a posteriori estimator based on composite likelihood (MAPCL) for inferring these speciation times under a model of DNA sequence evolution for which exact site pattern probabilities can be computed under the assumption of a constant θ throughout the species tree.
MAPCL estimates are statistically consistent and asymptotically normally distributed, and we show how this result can be used to estimate their asymptotic variance. Use of the nonparametric bootstrap provides a more accurate estimate of the variance of the estimates.
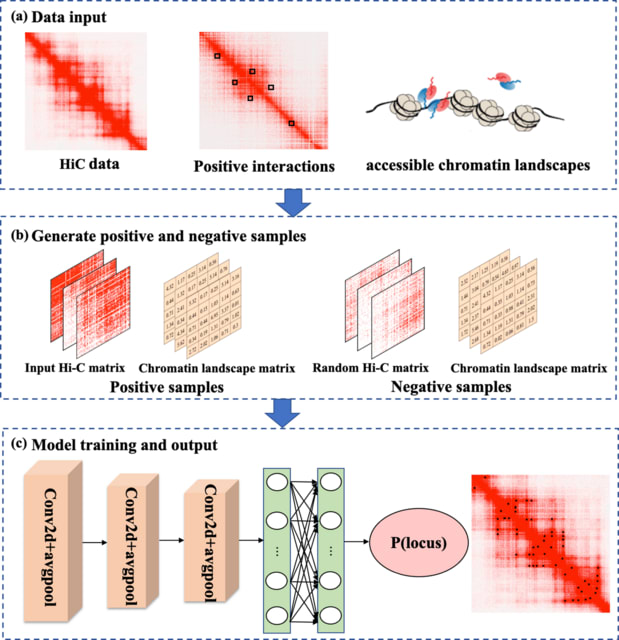
□ DLoopCaller: A deep learning approach for predicting genome-wide chromatin loops by integrating accessible chromatin landscapes
>> https://journals.plos.org/ploscompbiol/article?id=10.1371/journal.pcbi.1010572
DLoopCaller transforms the task of detecting chromatin loops into a binary classification problem by using enriched experimental data such as ChIA-PET/HiChIP and Capture Hi-C as positive interactions and non-interaction regions as negative samples.
DLoopCaller mainly include the following aspects: (i) efficiently combining one dimensional (1D) open chromatin landscapes with 3D genomic data for chromatin loops prediction; (ii) improving the identification accuracy of chromatin loops on wider chromatin contact matrix.
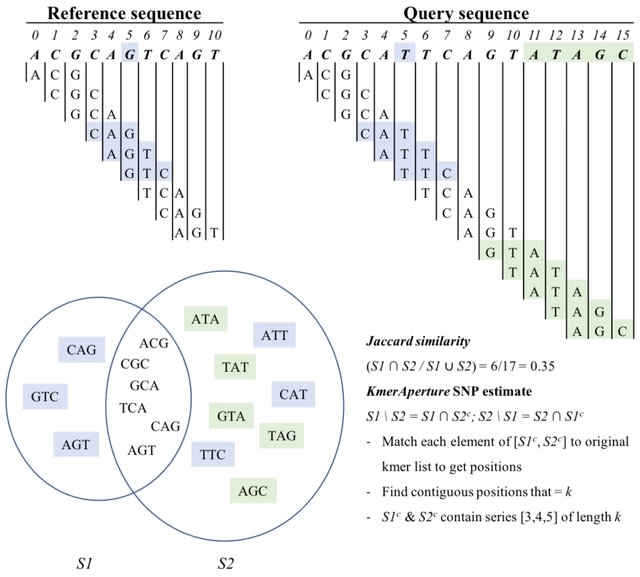
□ KmerAperture: Retaining k-mer synteny for alignment-free estimation of within-lineage core and accessory differences
>> https://www.biorxiv.org/content/10.1101/2022.10.12.511870v1
KmerAperture takes the relative complements of a pair of whole genome k-mer sets and matches back to the enumerated k-mer lists to gain positional information. A new algorithm that w/ the few available axioms of how core and accessory sequence diversity is represented in k-mers.
KmerAperture was benchmarked against Jaccard similarity and ‘split k-mer analysis’ using a diverse lineage, a lower core diversity sub-lineage w/ a large accessory genome and a very low core diversity simulated population w/ accessory content not associated with number of SNPs.

□ GSA-MREMA: Random-effects meta-analysis of effect sizes as a unified framework for gene set analysis
>> https://journals.plos.org/ploscompbiol/article?id=10.1371/journal.pcbi.1010278
A unifying framework for GSA that first fits effect size distributions, and then tests for differences in these distributions between gene sets. These differences can be in the proportions of genes that are perturbed or in the sign or size of the effects.
In MRENA, the log fold change for genes in a given set is modeled as a mixture of Gaussian distributions, with distinct components corresponding to up-regulated, down-regulated and non-DE genes. MRENA uses the EM algorithm to estimate the parameters of this mixture distribution.
Inspired by meta-analysis, the standard error of the DE effect size estimate is incorporated into the estimation procedure, w/ genes w/ large standard errors having less influence on the parameter estimates than genes for which the DE effect is estimated with greater precision.

□ CMIC: predicting DNA methylation inheritance of CpG islands with embedding vectors of variable-length k-mers
>> https://bmcbioinformatics.biomedcentral.com/articles/10.1186/s12859-022-04916-3
CMIC (CGI Methylation Inheritance Classifier), a Gated Recurrent Units - based model to augment CGI sequence by converting it into variable-length k-mers, where the length k is randomly selected from the range kmin to kmax, N times, which were then used as neural network input.
splitDNA2vec is a new embedding vector generator for k-mers. The sequence of the embedding vectors is passed to a BiGRU layer to predict the DNA methylation status of the input sequence, which we designated as CGI methylation classification method CMIC.
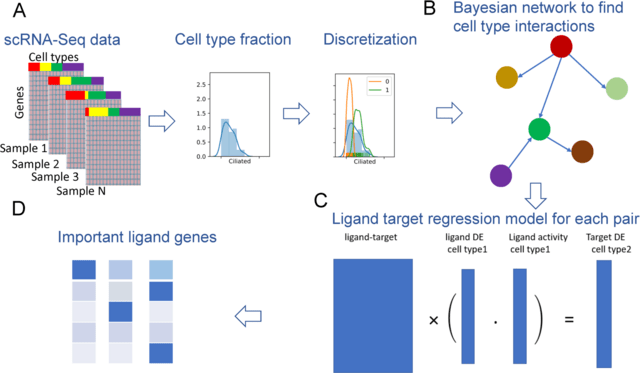
□ CINS: Cell Interaction Network inference from Single cell expression data:
>> https://journals.plos.org/ploscompbiol/article?id=10.1371/journal.pcbi.1010468
CINS combines Bayesian network learning with constrained regression analysis. CINS scRNA-Seq data from multiple samples of a similar condition to learn Bayesian networks which highlight the cell types whose distributions are co-varying under different conditions.
CINS discretizes the data for each cell type using a Gaussian Mixture Model with only two components and learns a BN that models the joint probability distribution of the cell type mixtures. High scoring differential causal relationships are determined based on bootstrapping.

□ Deep6: Classification of Metatranscriptomic Sequences into Cellular Empires and Viral Realms Using Deep Learning Models
>> https://www.biorxiv.org/content/10.1101/2022.09.13.507819v1
Deep6 is trained on reference coding sequences, but classification of query sequences is done reference-independent and alignment-free. The provided model is optimized for marine samples and can process sequences as short as 250 nucleotides.
Deep6 is a multi-class Convolutional Neural Network (CNN) model, consisting of 500 convolutions, 500 dense layers, a default kernel size of ten and a maximum of 40 epochs of training.

□ Prophaser: A joint use of pooling and imputation for genotyping SNPs
>> https://bmcbioinformatics.biomedcentral.com/articles/10.1186/s12859-022-04974-7
IMPUTE2 and MACH form the HMM hidden states by selecting h template haplotypes, such there is a constant number h^2 hidden states at each of the j diploid markers. Hence, these methods have a complexity O(jh^2) in time for individual, and the time complexity grows linearly.
A statistical framework that formalizes pooling as a mathematical transformation of the genotype data. Prophaser algorithm, the coalescence assumption supports an imputation model that delivers high accuracy in pooled genotype reconstruction.

□ Transcription factor expression is the main determinant of variability in gene co-activity
>> https://www.biorxiv.org/content/10.1101/2022.10.11.511770v1
Focusing specifically on co-activity domains with variable co-activity between individuals to study the regulatory mechanisms driving co-activity, including genotype, TF abundance, and chromatin interactions.
Via approximate Bayesian modeling, expression count data, quantified in 10 kb genomic bins, are decomposed into a co-activity component, which is positionally dependent, and a positionally independent component. The co-activity component is modeled as a first-order random walk.
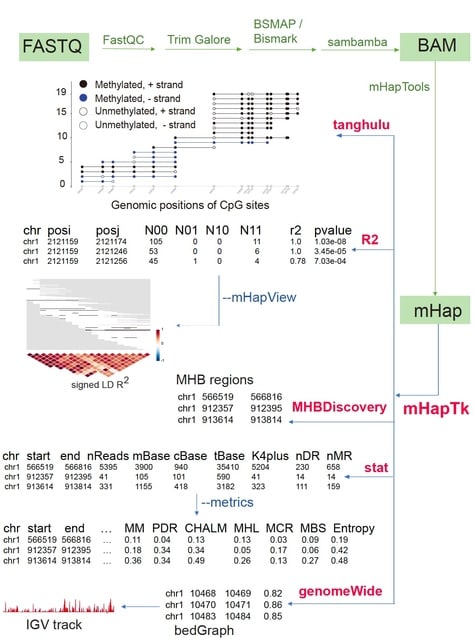
□ mHapTk: A comprehensive toolkit for the analysis of DNA methylation haplotypes
>> https://academic.oup.com/bioinformatics/advance-article-abstract/doi/10.1093/bioinformatics/btac650/6731920
The DNA methylation status of CpG sites on the same fragment represents a discrete methylation haplotype (mHap). However, most existing tools focus on average methylation and ne-glect mHap patterns.
mhapTk calculates eight mHap-level summary statistics in predefined regions or across individual CpG in a genome-wide manner. It identifies methylation haplotype blocks (MHBs), in which methylation of pairwise CpGs are tightly correlated.
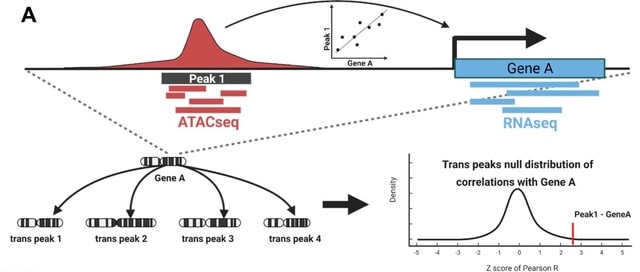
□ Major cell-types in multiomic single-nucleus datasets impact statistical modeling of links between regulatory sequences and target genes
>> https://www.biorxiv.org/content/10.1101/2022.09.15.507748v1
The Z-scores method results in a strong loss of power to detect the regulatory effect of cCREs with high read counts in the most abundant cell-type(s). A strong loss of power to detect a regulatory effect for cCREs with high read counts in the dominant cell-type.
This is largely due to cell-type-specific trans-ATACseq peak correlations creating bimodal null distributions. the raw Pearson correlation coefficients and/or physical distance is computationally advantageous and provides the best predictions of “ATACseq peak-target gene” links.
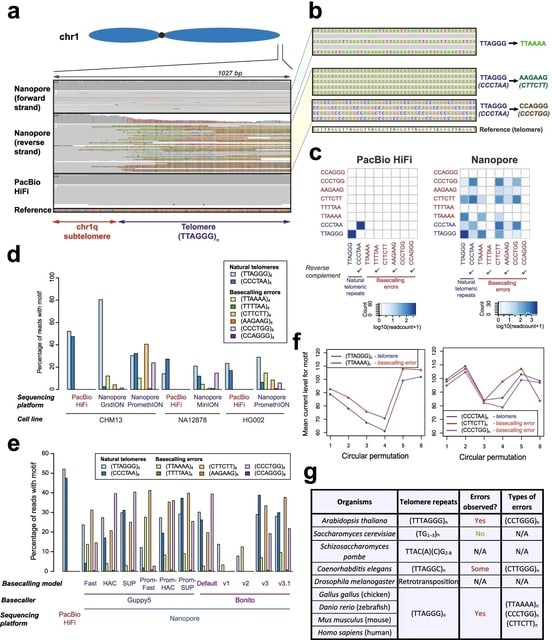
□ Identifying and correcting repeat-calling errors in nanopore sequencing of telomeres
>> https://genomebiology.biomedcentral.com/articles/10.1186/s13059-022-02751-6
Telomeric regions were frequently miscalled as other types of repeats in a strand-specific manner. Specifically, although human telomeres are typically represented by (TTAGGG)n repeats, these regions were frequently recorded as (TTAAAA)n repeats.
These artefacts were not observed on the CHM13 reference genome, or PacBio HiFi reads from the same site, suggesting that these observed repeats are artefacts of nanopore sequencing or the base-calling process
The examination of each telomeric long read also indicates that these error repeats frequently co-occur with telomeric repeats at the ends of each read, and are observed on all chromosomal arms of CHM13.
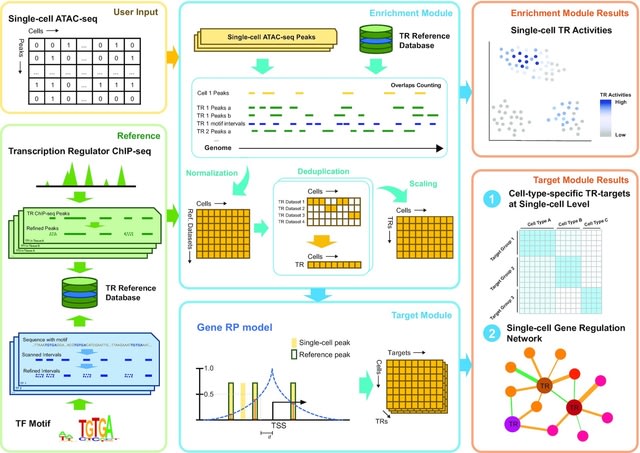
□ SCRIP: Single-cell gene regulation network inference by large-scale data integration
>> https://academic.oup.com/nar/advance-article/doi/10.1093/nar/gkac819/6717821
SCRIP infers single-cell TR activity and targets based on the integration of scATAC-seq and a large-scale TR ChIP-seq reference. SCRIP enables identifying TR target genes as well as building GRNs at the single-cell resolution based on a regulatory potential model.
SCRIP takes the scATAC-seq peak by count matrix or bin count matrix as input. SCRIP calculates the number of peak overlaps b/n each cell and the ChIP-seq peaks set or motif-scanned intervals set. SCRIP enables the trajectory analyses of scATAC-seq with known driver TR activity.
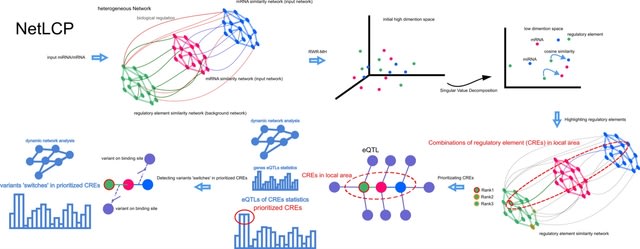
□ NetLCP: An R package for prioritizing combinations of regulatory elements in the heterogeneous network with variant 'switches' detection
>> https://www.biorxiv.org/content/10.1101/2022.10.06.511229v1
NetLCP prioritizes CREs by highlighting regulatory elements and detecting regulatory ‘switches’ in the heterogeneous network. By leveraging multidimensional biological knowledge, it provides a meaningful perspective on user-interested biological processes or functions.
NetLCP highlights regulatory elements (lncRNA, circRNA, KEGGPath, ReactomePath and WikipathwayPath) in the heterogeneous network, which have similar biological functions to the given input transcriptome (miRNA/mRNA).
NetLCP produces a tab-delimited text files which records the prioritized elements with column names of lncRNA/circRNA/pathway ID, FunScore, OfficialName and Empirical P-value.
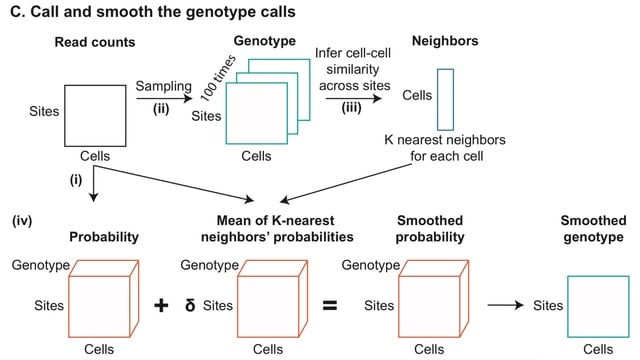
□ PhylinSic: Phylogenetic inference from single-cell RNA-seq data
>> https://www.biorxiv.org/content/10.1101/2022.09.27.509725v1
PhylinSic is robust to the low read depth, drop-out, and noisiness of scRNA-Seq data. This method called nucleotide bases from scRNA-Seq reads using a probabilistic smoothing approach, and then estimated a phylogenetic tree using a Bayesian modeling algorithm.
PhylinSic first identified sites that varied across the cells and thus might best reveal phylogenetic structure. PhylinSic assigns reference and alternate bases according to the base seen in the alignments, and if the genotype was heterozygous, it assigns an arbitrary surrogate base. Finally, to estimate the phylogeny of the cells, using BEAST2.

□ TAMC: A deep-learning approach to predict motif-centric transcriptional factor binding activity based on ATAC-seq profile
>> https://journals.plos.org/ploscompbiol/article?id=10.1371/journal.pcbi.1009921
TAMC (Transcriptional factor binding prediction from ATAC-seq profile at Motif-predicted binding sites using Convolutional neural networks) predicts motif-centric TF binding activity from paired-end ATAC-seq data. TAMC does not require bias correction during signal processing.
By leveraging a one-dimensional convolutional neural network (1D-CNN) model, TAMC make predictions based on both footprint and non-footprint features and outperforms existing footprinting tools in TFBS prediction particularly for ATAC-seq data with limited sequencing depth.
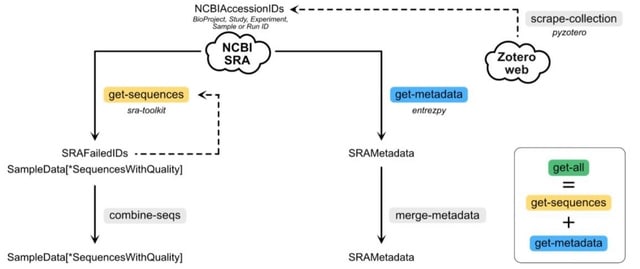
□ q2-fondue: Reproducible acquisition, management, and meta-analysis of nucleotide sequence (meta)data
>> https://academic.oup.com/bioinformatics/advance-article/doi/10.1093/bioinformatics/btac639/6706785
q2-fondue allows fully provenance-tracked programmatic access to and management of data from the NCBI Sequence Read Archive (SRA).
q2-fondue enables full data provenance tracking from data download to final visualization, integrates with the QIIME 2 ecosystem, prevents data loss upon space exhaustion, and allows download of (meta)data given a publication library.
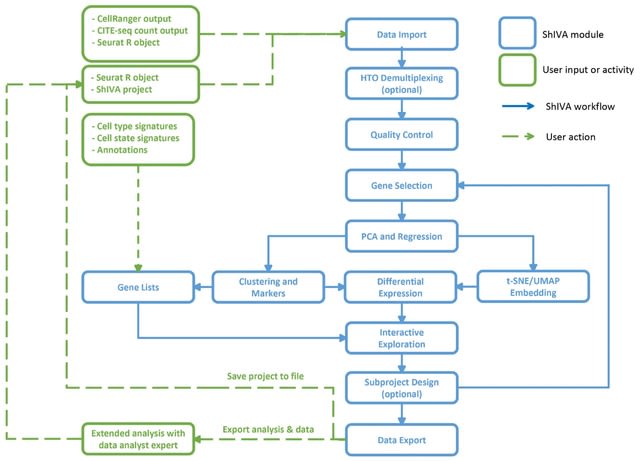
□ ShIVA, a user-friendly and interactive interface giving biologists control over their single-cell RNA-seq data.
>> https://www.biorxiv.org/content/10.1101/2022.09.20.508636v1
ShIVA supports cell hashing analysis and provides great flexibility in visualization, whether by dimensionality reduction maps, boxplots, violin plots, histograms, density plots, or count tables.
ShIVA keeps track of the user’s choice by defining a hierarchy of sub-projects, each of them containing the results of different user choices. Switching between sub-projects allows for comparison of analysis processes to optimize the deciphering of the dataset.
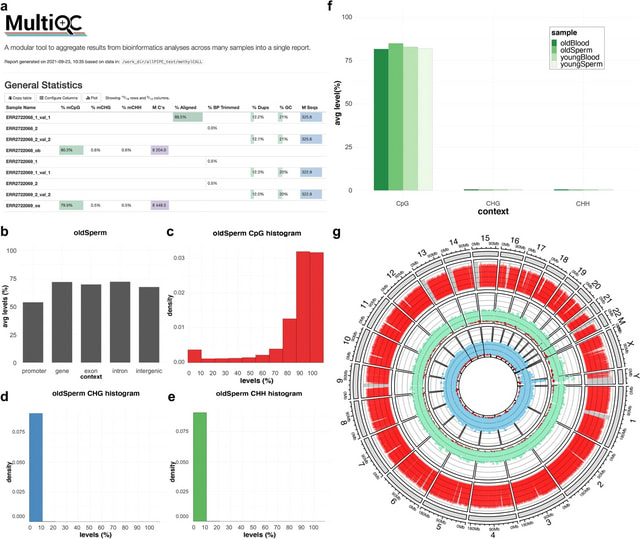
□ msPIPE: a pipeline for the analysis and visualization of whole-genome bisulfite sequencing data
>> https://bmcbioinformatics.biomedcentral.com/articles/10.1186/s12859-022-04925-2
The msPIPE pipeline consists of pre-processing, alignment & methylation calling, and methylation analysis & visualization steps. It generates a DNA methylation profile for each sample, which is a unit of analysis defined by user.
The msPIPE can be used to treat one or more replicates for each sample. In brief, the required reference files are prepared using the given UCSC assembly name of a reference, and the input bisulfite sequencing reads in each sample are trimmed first.
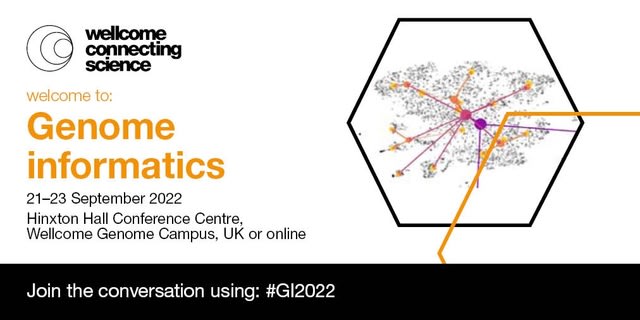
□ Genome Informatics 2022 #GI2022
>> https://coursesandconferences.wellcomeconnectingscience.org/event/genome-informatics-20220921/
Wellcome Connecting Science Courses RT
Get ready for 3 days of inspiring discussion and networking at Genome Informatics 2022! 🙌
A huge welcome to all our delegates: 106 in-person & 432 online, joining us from 72 countries.
Make sure to Tweet your community using #GI2022 and tag in @eventsWCS

□ Verticall: Tool for recombination-free phylogrnies:
>> https://github.com/rrwick/Verticall/tree/main/verticall
Assemblies as input / Makes a distance matrix / points the genomes vertical / horizontal #GI2022
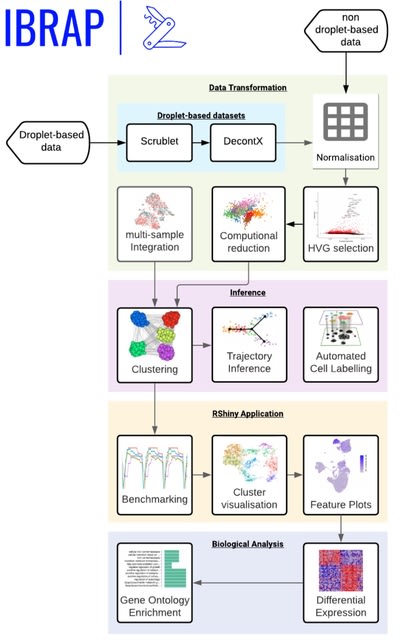
□ IBRAP: Integrated Benchmarking Single-cell RNA-sequencing Analytical Pipeline
>> https://www.biorxiv.org/content/10.1101/2022.09.26.509481v1
IBRAP contains a range of analytical components that can be interchanged throughout the pipeline alongside multiple benchmarking metrics that enables users to compare results and determine the optimal pipeline combinations for their data.
IBRAP performs clustering, trajectory inference and automated cell labelling. Within the clustering step, a selection of popular clustering techniques was integrated, including k-means, PAM, SC3, Louvain, Louvain with Multilevel Refinement, Smart Local Moving, and Leiden.
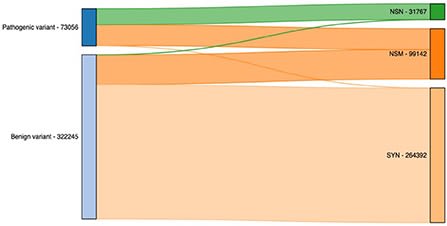
□ SNPAAMapper-Python: A highly efficient genome-wide SNP variant analysis pipeline for Next-Generation Sequencing data
>> https://www.frontiersin.org/articles/10.3389/frai.2022.991733/full
In the Python version of SNPAAMapper, the second script for processing exon annotation files and generating feature start and gene mapping files performs extremely better than the one in the original Perl version.
Steps of predicting amino acid change type and prioritizing mutation effects of variants were executed within 1 s for both pipelines. SNPAAMapper-Python was developed and tested on the ClinVar database, a NCBI database of information on genomic variation.

□ Xenium: High resolution, high-target analysis
>> https://www.10xgenomics.com/in-situ-technology
The Xenium workflow starts with sectioning tissues onto a microscope slide. The sections are then treated to access the RNA for labeling with circularizable DNA probes.
Ligation of the probes then generates a circular DNA probe which is enzymatically amplified and bound with fluorescent oligos that has a high signal-to-noise ratio. An optical signature specific to each gene is generated, enabling identification of the target gene.

□ A workflow reproducibility scale for automatic validation of biological interpretation results.
>> https://www.biorxiv.org/content/10.1101/2022.10.11.511695v1
A new metric, a reproducibility scale of workflow execution results, to evaluate the reproducibility of results. This metric is based on the idea of evaluating the reproducibility of results using biological feature values representing their biological interpretation.
The workflow built by the workflow developer is executed by WES, which is a combination of Sapporo and Yevis, and the workflow provenance, including feature values of the output files, is generated in RO-Crate format.
Using Tonkaz, the user then compares the shared provenance with the provenance generated by the user’s workflow execution and verifies the reproducibility.

□ scGNN 2.0: a graph neural network tool for imputation and clustering of single-cell RNA-Seq data
>> https://academic.oup.com/bioinformatics/advance-article-abstract/doi/10.1093/bioinformatics/btac684/6762077
The implementation of scGNN 2.0 is significantly faster than scGNN thanks to a simplified close-loop architecture. Cell clustering performance was increased by 85.02% on average in terms of adjusted rand index, and the imputation Median L1 Error was reduced by 67.94% on average.
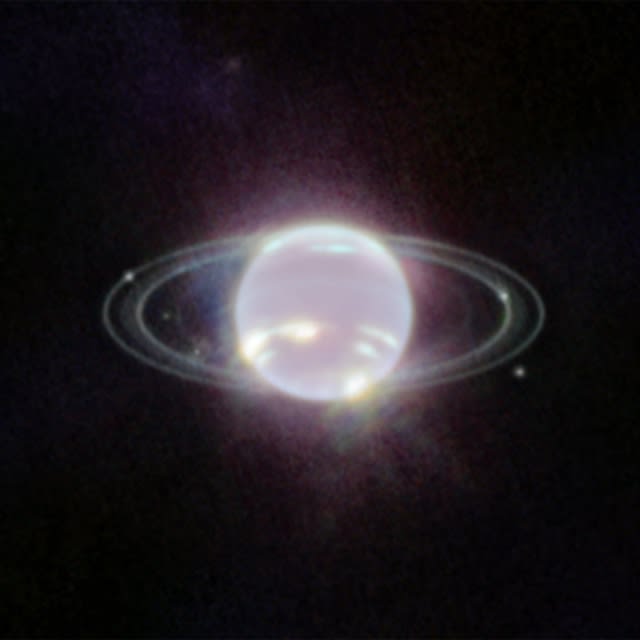
□ NASA Webb Telescope RT
Hey Neptune. Did you ring? 👋
Webb’s latest image is the clearest look at Neptune's rings in 30+ years, and our first time seeing them in infrared light. Take in Webb's ghostly, ethereal views of the planet and its dust bands, rings and moons: go.nasa.gov/3RXxoGq #IAC2022
>> https://www.nasa.gov/feature/goddard/2022/new-webb-image-captures-clearest-view-of-neptune-s-rings-in-decades
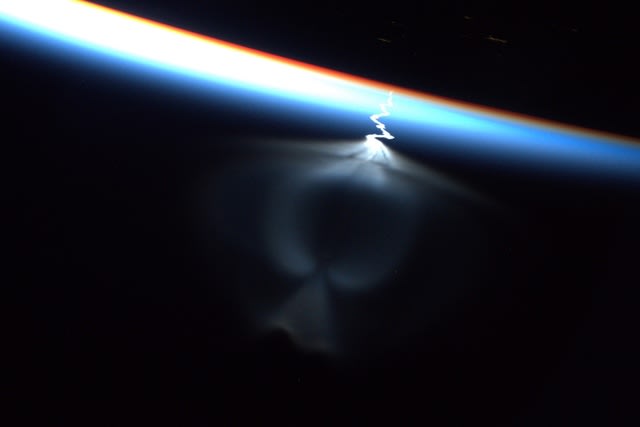
□ Samantha Cristoforeti RT
>> https://twitter.com/astrosamantha/status/1572600896038526977?s=21&t=YABVz4FJdfY_W1IKQXF2nA
We had a spectacular view of the #Soyuz launch!
Sergey, Dmitry and Frank will come knocking on our door in just a couple of hours… looking forward to welcoming them to their new home! #MissionMinerva
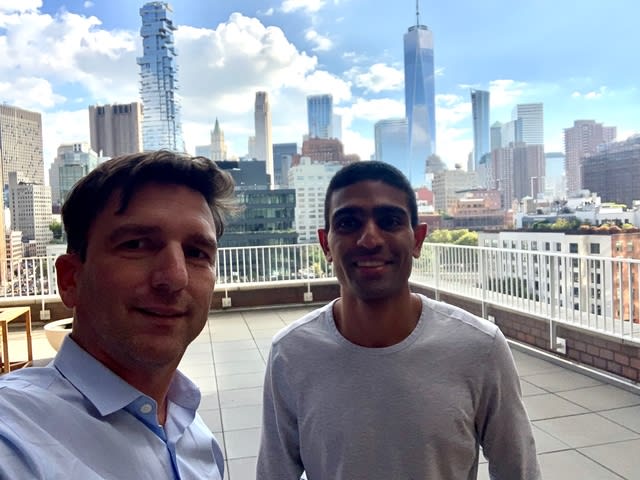
□ Nicolas Robine RT
>> https://twitter.com/notsojunkdna/status/1568265804658909187?s=21&t=rVGpMaySUH1R1C8hf9T-_g
>> http://haymakersforhope.org/event/new-york
With @polyethnic1000, we're fighting against cancer health disparity, but this young fellow is doing it literally (with boxing gloves), and fundraising for the project. Please support Rahul's effort!

□ Anna Cuomo RT
>> https://www.singlecells.org.au/
>> https://twitter.com/annasecuomo/status/1570672816093278210?s=21&t=rVGpMaySUH1R1C8hf9T-_g
An absolute pleasure attending and presenting at my first Oz conference! Amazing science and a stunning location 🧬🌊 #ozsinglecell22
※コメント投稿者のブログIDはブログ作成者のみに通知されます