
Announcing the Haven-1 and Vast-1 missions to low-Earth orbit. Launched by @SpaceX, Haven-1 is scheduled to be the world’s first commercial space station and will be visited by a crew of four aboard a Dragon spacecraft during Vast-1 → https://t.co/ToxFSiyQJj pic.twitter.com/YSPrM9Krtr
— VΛST (@vast) May 10, 2023

□ Mark
>> https://www.vastspace.com/roadmap
Very exciting timeline from Haven-1 in 2025 on F9 to 2030 Starship class space station/modules to 100m spinning station in the 2040’s.
Excellent plan and realistic timeline.

□ NextPolish2: a repeat-aware polishing tool for genomes assembled using HiFi long reads
>> https://www.biorxiv.org/content/10.1101/2023.04.26.538352v1
NextPolish2 can fix base errors in “highly accurate” draft assemblies without introducing overcorrections, even in regions with highly repetitive elements. Through the built-in phasing module, it can not only correct the error bases, but also maintain the original haplotype consistency.
NextPolish2 follows the Kmer Score Chain (KSC) algorithm of its previous version to perform an initial rough correction, and detect low-quality positions (LQPs) where the chosen alleles account for ≤ 0.95 of the total during a traceback procedure.
NextPolish2 repeats the above procedure until all conflict communities are resolved (the number of iterations can be adjusted according to user settings) and then use the KSC algorithm to generate a draft consensus sequence.

□ CODEC: Single duplex DNA sequencing with CODEC detects mutations with high sensitivity
>> https://www.nature.com/articles/s41588-023-01376-0
CODEC (Concatenating Original Duplex for Error Correction), a hybrid method that combines the massively parallel nature of NGS and the resolution of single-molecule sequencing by reading both strands of each DNA duplex with single NGS read pairs.
The CODEC structure can be built by replacing a typical adapter duplex with the CODEC adapter quadruplex, containing all elements required for NGS.
CODEC to physically concatenate the Watson strand with the reverse complement of the Crick strand into a single strand without forming a prohibitive hairpin or inverted repeat structure from two complementary sequences.
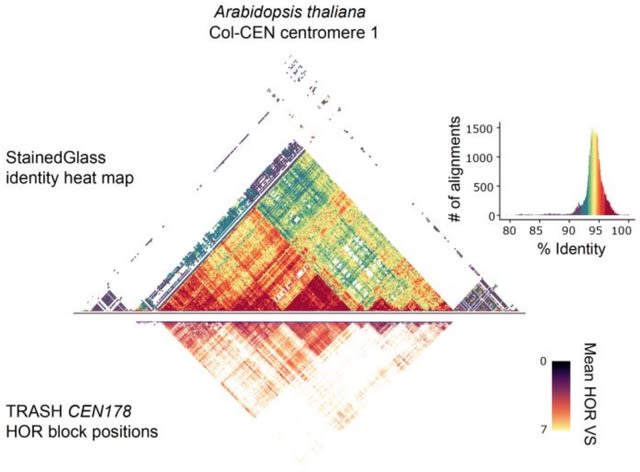
□ TRASH: Tandem Repeat Annotation and Structural Hierarchy
>> https://academic.oup.com/bioinformatics/advance-article/doi/10.1093/bioinformatics/btad308/7159186
TRASH (Tandem Repeat Annotation and Structural Hierarchy) is a tool that identifies and maps tandem repeats in nucleotide sequence, without prior knowledge of repeat composition.
TRASH analyses a fasta assembly file, identifies regions occupied by repeats and then precisely maps them and their higher order structures.
TRASH searches for continuous, highly similar, tandemly arranged DNA repeats of a similar unit size. This excludes transposable elements and interspersed repeats from analysis and allows for precise definition of tandemly arranged repeats.

□ GraNA: Supervised biological network alignment with graph neural networks
>>
GraNA, a deep learning framework for the supervised NA paradigm for the pairwise network alignment problem. GraNA utilizes within-network interactions and across-network anchor links for learning protein representations and predicting functional correspondence.
GraNA integrates sequence similarity edges as additional anchor links to guide the alignment and pre-computed network embeddings as node features to better encode the topological roles of network nodes.

□ Riboformer: A Deep Learning Framework for Predicting Context-Dependent Translation Dynamics
>> https://www.biorxiv.org/content/10.1101/2023.04.24.538053v1
Riboformer uses a transformer architecture that detects long-range dependencies in the regulation of elongation. Riboformer models the context-dependent changes in ribosome dynamics at codon resolution.
The transformer block consists of self-attention layers that gather the impact of distant codons based on their sequence representations, in contrast to convolutional neural network that relies on convolution operators to detect local sequence motifs.
Riboformer can be combined with in silico mutagenesis analysis to identify sequence motifs that contribute to ribosome stalling. It also utilizes a reference input to prevent the learning of noninformative signals due to the experimental bias.

□ CellANOVA: Signal recovery in single cell batch integration
>> https://www.biorxiv.org/content/10.1101/2023.05.05.539614v1
CellANOVA utilizes a “pool-of-controls”, applicable across diverse settings, to separate unwanted variation from biological variation. CellANOVA allows the recovery of subtle biological signals and corrects, to a large extent, the data distortion introduced by integration.
A control-pool is a set of samples whereby variation beyond what is preserved by the existing integration are not of interest to the study. The control-pool samples are utilized to estimate a latent linear space that captures cell- and gene-specific unwanted batch variations.
CellANOVA produces a batch corrected GE matrix which can be used for gene-pathway level downstream analyses. By using the control pool in the estimation of the batch variation space, CellANOVA recovers any variation in the non-control samples that lie outside this space.

□ ProteiNN: a Transformer-based model for end-to-end single-sequence protein structure prediction
>> https://www.biorxiv.org/content/10.1101/2023.04.26.538026v1
ProteiNN predicts protein secondary and tertiary structures directly from integer-encoded amino acid sequences. The model was trained and evaluated using the SideChainNet dataset, which provides the basis for complete model training.
The input to the module is a sequence of feature vectors mapped to these component spaces via linear transformations. The multi-head mechanism enables the model to learn relationships between amino acids in parallel.
ProteiNN uses a gating mechanism that modulates the information flow between the input and output, allowing the model to emphasize specific relationships and discard irrelevant information selectively.

□ DeepUMQA3: a web server for model quality assessment of protein complexes
>> https://www.biorxiv.org/content/10.1101/2023.04.24.538194v1
DeepUMQA and DeepUMQA2, new features were designed for complex structures, and the lDDT of each residue and the accuracy of interface residues were predicted using an improved deep neural network.
At the level of overall complex, the overall complex is regarded as a large monomer structure. DeepUMQA3 provides fast and accurate interface residue accuracy prediction and per-residue lDDT prediction services for protein complexes.

□ ecpc: an R-package for generic co-data models for high-dimensional prediction
>> https://bmcbioinformatics.biomedcentral.com/articles/10.1186/s12859-023-05289-x
ecpc originally accommodated various and possibly multiple co-data sources, including categorical co-data, i.e. groups of variables, and continuous co-data. Continuous co-data were handled by adaptive discretisation, potentially inefficiently modelling and losing information.
An extension to the method for generic co-data models, particularly for continuous co-data. At the basis lies a classical linear regression model, regressing prior variance weights on the co-data. Co-data variables are then estimated with empirical Bayes moment estimation.

□ MaxKAT: A maximum kernel-based association test to detect the pleiotropic genetic effects on multiple phenotypes
>> https://academic.oup.com/bioinformatics/advance-article-abstract/doi/10.1093/bioinformatics/btad291/7146028
MaxKAT reduces computational intensity greatly while maintaining high accuracy. Extensive simulations demonstrate that MaxKAT can properly control type I error rates and obtain remarkably higher power than KAT under most of the considered scenarios.
A generalized extreme value distribution is employed to calculate the statistical significance of MaxKAT under the null hypothesis. In addition, the proposed test can accommodate high-dimensional data and yield high power against various alternative hypotheses.

□ SeqImprove: Machine Learning Assisted Creation of Machine Readable Sequence Information
>> https://www.biorxiv.org/content/10.1101/2023.04.25.538300v1
SeqImprove is designed to aid authors in creating machine readable sequence data with complete metadata. It consists of a user-interface that was built using modular code. It can be reused by others to work as the front-end for their curation software.
As input, SeqImprove takes in a sequence file in the Synthetic Biology Open Language (SBOL) format or a link to a sequence stored in SynBioHub. It makes the information machine readable by using existing ontologies to structure the metadata.

□ CNV-ClinViewer: Enhancing the clinical interpretation of large copy-number variants online
>> https://academic.oup.com/bioinformatics/advance-article/doi/10.1093/bioinformatics/btad290/7146044
CNV-ClinViewer enables real-time interactive exploration of large CNV datasets in a user-friendly designed interface and facilitates semi-automated clinical CNV interpretation following the ACMG guidelines by integrating the ClassifCNV tool.
The CNV-ClinViewer allows analysis of single or multiple CNVs, of the used to identify them. Minimal required information for each CNV, including whole chromosome trisomies and monosomies, is the chromosome, start, end and CNV type.

□ OrthoVenn3: an integrated platform for exploring and visualizing orthologous data across genomes
>> https://academic.oup.com/nar/advance-article/doi/10.1093/nar/gkad313/7146343
OrthoVenn3 provides gene family contraction and expansion analysis to support researchers better understanding the evolutionary history of gene families, as well as collinearity analysis to detect conserved and variable genomic structures.
OrthoVenn3 offers multiple out-puts, including the UpSet table, occurrence table, phylogenetic tree, and collinearity graph, which provides users with various perspectives on their data.

□ ELVAR: Cell-attribute aware community detection improves differential abundance testing from single-cell RNA-Seq data
>> https://www.biorxiv.org/content/10.1101/2023.04.28.538653v1
ELVAR uses cell attribute aware clustering when inferring differentially enriched communities within the single-cell manifold. ELVAR can detect disease relevant DA-shifts in other cell-types and biological conditions.
The improved sensitivity to detect DA-shifts, as displayed by ELVAR, was also seen when benchmarked against an analogous clustering-based DA-method that uses Louvain in place of EVA.

□ xQTLbiolinks: a comprehensive and scalable tool for integrative analysis of molecular QTLs
>> https://www.biorxiv.org/content/10.1101/2023.04.28.538654v1
xQTLbiolinks is a end-to-end bioinformatic tool for efficient mining and analyzing public and user-customized xQTLs data for the discovery of disease susceptibility genes.
xQTLbiolinks allows users to conveniently retrieve ×QTLs data and metainformation for further analysis through gene names/IDs, tissue names, or genomic regions of interest.

□ Combining LIANA and Tensor-cell2cell to decipher cell-cell communication across multiple samples
>> https://www.biorxiv.org/content/10.1101/2023.04.28.538731v1
Integrating LIANA and Tensor-cell2cell, which combined can deploy multiple existing methods and resources, to enable the robust and flexible identification of cell-cell communication programs across multiple samples.
In this protocol, the integration of the tools facilitates the choice of method to infer cell-cell communication and subsequently perform an unsupervised deconvolution to obtain and summarize biological insights.

□ Signed distance correlation (SiDCo): an online implementation of distance correlation and partial distance correlation for data-driven network analysis
>> https://academic.oup.com/bioinformatics/advance-article-abstract/doi/10.1093/bioinformatics/btad210/7151065
SiDCo is a GUI-platform for calculation of distance correlation in omics data, measuring linear and non-linear dependences between variables, as well as correlation between vectors of different lengths, e.g., different sample sizes.
Distance correlations can be selected as one-to-one / one-to-all correlations, showing relationships b/n each / all other features one at a time. SiDCo uses partial distance correlation, calculated using the Gaussian Graphical model approach adapted to distance covariance.
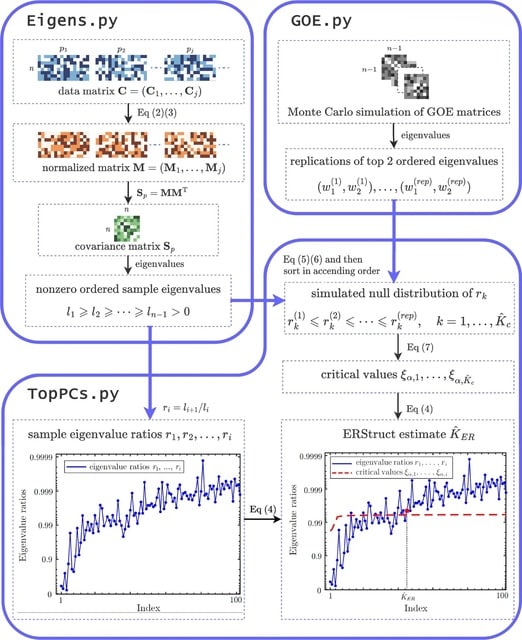
□ ERStruct: a fast Python package for inferring the number of top principal components from whole genome sequencing data
>> https://bmcbioinformatics.biomedcentral.com/articles/10.1186/s12859-023-05305-0
ERStruct enables the inference of population structure using whole-genome sequencing data. By leveraging parallel computing and GPU acceleration, ERStruct achieves significant improvements in the speed of matrix operations for large-scale data.
In GOE.py, Monte Carlo method is used in the ERStruct algorithm to obtain the null distribution of our proposed ERStruct test statistic, which starts by generating multiple replications of high-dimensional Gaussian Orthogonal Ensemble matrices.

□ PascalX: a python library for GWAS gene and pathway enrichment tests
>> https://academic.oup.com/bioinformatics/advance-article/doi/10.1093/bioinformatics/btad296/7151067
PascalX allows for scoring genes and annotated gene sets for enrichment signals based on data from, both, single GWAS and pairs of GWAS. The gene scores take into account the correlation pattern between SNPs.
They are based on the cumulative density function of a linear combination of χ2 distributed random variables, which can be calculated either approximately or exactly to high precision.

□ CZ CELLxGENE Discover Census
>> https://chanzuckerberg.github.io/cellxgene-census/
The Census provides efficient computational tooling to access, query, and analyze all single-cell RNA data from CZ CELLxGENE Discover.
Using a new access paradigm of cell-based slicing and querying, you can interact with the data through TileDB-SOMA, or get slices in AnnData or Seurat objects, thus accelerating your research by significantly minimizing data harmonization.

□ kimma: flexible linear mixed effects modeling with kinship covariance for RNA-seq data
>> https://academic.oup.com/bioinformatics/advance-article/doi/10.1093/bioinformatics/btad279/7152273
kimma supports DEG analyzes incl. covariance random effects. Kimma is an open-source R package that provides flexible linear mixed effects modeling for bulk RNA-seq data including univariate, multivariate, random, and covariance random effects as well as gene-level weights.
kimma utilizes a single function, kmFit, for modeling, ensuring consistent syntax, inputs, and outputs. Moreover, kimma provides post-hoc pairwise tests, model fit metrics like AIC, and fit warnings on a per gene basis.

□ CAGECAT: The CompArative GEne Cluster Analysis Toolbox for rapid search and visualisation of homologous gene clusters
>>
CAGECAT has been designed to provide rapid interoperability between these functions, where homologous clusters of interest can be selected to be used in subsequent analysis.
CAGECAT can yield relevant matches that aid in the comparison, taxonomic distribution, or evolution of an unknown query. The search module leverages the cblaster pipeline, which utilises remote BLAST searches via NCBI’s servers as well as accelerated local Hidden Markov Model.

□ cellsnake: a user-friendly tool for single cell RNA sequencing analysis
>> https://www.biorxiv.org/content/10.1101/2023.05.03.539204v1
Cellsnake allows parallelization and readily utilizes high performance computing (HPC) platforms. cellsnake provides metagenome analysis capabilities if unmapped reads are available.
cellsnake can utilize different scRNA-seq algorithms to simplify tasks such as automatic mitochondrial gene trimming, selection of optimal clustering resolution, doublet filtering, visualization of marker genes, enrichment analysis and pathway analysis.

□ Whole-genome long-read sequencing downsampling and its effect on variant calling precision and recall
>> https://www.biorxiv.org/content/10.1101/2023.05.04.539448v1
Defining read-based methodologies as those requiring alignment of individual sequencing reads to a reference genome and applying specific read-based variant-calling algorithms to these alignments to identify variants.
Assembly-based methods first generate ab initio a whole-genome assembly from LRS reads without guidance from a particular reference genome, and then proceed analogously by aligning this assembly to a reference genome to call variants using assembly-based calling algorithms.
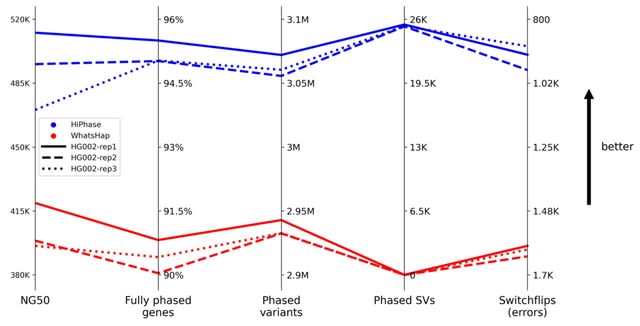
□ HiPhase: Jointly phasing small and structural variants from HiFi sequencing
>> https://www.biorxiv.org/content/10.1101/2023.05.03.539241v1
HiPhase jointly phases SNVs, indels, and structural variants called from PacBio HiFi sequencing on diploid organisms. HiPhase uses two novel approaches to solve the phasing problem: dual mode allele assignment and a phasing algorithm based on the A* search algorithm.
HiPhase offers additional benefits: no down-sampling, multi-allelic variation, logic to span coverage gaps with supplementary alignments, innate multi-threading, built-in statistics gathering, and assigning aligned reads to a haplotype (“haplotagging”) while phasing.

□ scMayoMap: an easy-to-use tool for cell type annotation in single-cell RNA-sequencing data analysis
>> https://www.biorxiv.org/content/10.1101/2023.05.03.538463v1
ScMayoMap takes the standard cluster marker gene list as input and returns the cell type prediction results in a plot and the mapped gene list. scMayoMap allows assignment of multiple cell types to the same cluster if their evidence is similar.
scMayoMap can predict PBMC cell types with small errors, suggesting that marker-based approach is still a promising approach if applied properly.

□ DeepGNN: Semi-supervised learning improves regulatory sequence prediction with unlabeled sequences
>> https://bmcbioinformatics.biomedcentral.com/articles/10.1186/s12859-023-05303-2
DeepGNN, a paradigm shift with semi-supervised learning, which does not only exploit labeled sequences (e.g. human genome with ChIP-seq experiment), but also unlabeled sequences available in much larger amounts.
In parallel, the model takes as a secondary input the graph matrix connecting homologous sequences between species. An improvement would be to infer the homology matrix from the sequence embedding itself during training.

□ Challenges and considerations for reproducibility of STARR-seq assays
>> https://genome.cshlp.org/content/early/2023/05/02/gr.277204.122.long
A strong advantage of STARR-seg is its ability to screen random fragments of DNA from any source for enhancer activity. To this effect, DNA can be sourced from commercially available DNA repositories, from specific populations carrying non-coding mutations or SNPs to be assayed.
Cloning strategies such as In-fusion HD, Gibson assembly, and NEBuilder HiFi DNA Assembly allow for fast and one-step reactions that use complimentary overhang sequences on the inserts and the vector.
Highlighting the different challenges in performing STARR-seg, a particularly long and difficult assay with huge potential to identify detailed enhancer landscapes and validate enhancer function.

□ STEMSIM: a simulator of within-strain short-term evolutionary mutations for longitudinal metagenomic data
>> https://academic.oup.com/bioinformatics/advance-article/doi/10.1093/bioinformatics/btad302/7156836
STEMSIM (short-term evolutionary mutations simulator), which can generate mutations incl. SNV and InDel with various frequency distributions within strains in raw metagenomic sequencing data under a specified nucleotide substitution model.
STEMSIM directly takes the output of CAMISIM as input data. Next, the raw sequencing reads are mapped to the original reference genomes to obtain the alignment files (sam/bam) by Bowtie2.
Then, the details of mutations are gerated according to the specified parameters, such as the number of nucleotide substitutions, and the distribution and trajectory of allele frequency.

□ scDist: Robust identification of perturbed cell types in single-cell RNA-seq data
>> https://www.biorxiv.org/content/10.1101/2023.05.06.539326v1
scDist estimates the distance between condition means in high-dimensional gene expression space for each cell type. scDist can recover biologically relevant between-group differences while also controlling for sample-level variability.
scDist is based on a linear mixed-effects model of single-cell GE counts. scDist uses an approximation for the between-group differences, based on a low-dimensional embedding, which results in a computationally convenient implementation that is substantially faster than Augur.

□ crosshap: Local haplotype visualization for trait association analysis
>> https://www.biorxiv.org/content/10.1101/2023.05.07.539781v1
crosshap performs density-based clustering of variants based on their linkage profiles to capture haplotype structures in local genomic regions. Tightly linked variants are clustered into MGs, and individuals are grouped into local haplotypes by shared allelic combinations.
Visualization tools are provided by crosshap for choosing optimal clustering parameters and producing intuitive crosshap figures that present information on the complex relationships between linked variants, haplotype combinations, and phenotypic/metadata traits of individuals.

□ SpatialData: an open and universal data framework for spatial omics
>> https://www.biorxiv.org/content/10.1101/2023.05.05.539647v1
SpatialData, a framework that establishes a unified and extensible multi-platform file-format, lazy representation of larger-than-memory data, transformations, and alignment to common coordinate systems.
SpatialData facilitates spatial annotations and cross-modal aggregation and analysis, the utility of which is illustrated via multiple vignettes including integrative analysis on a multi-modal Xenium and Visium breast cancer study.
※コメント投稿者のブログIDはブログ作成者のみに通知されます