
(Art by MΞV)



□ Large Language Models as Markov Chains
>> https://arxiv.org/abs/2410.02724
An equivalence between generic autoregressive language models with vocabulary of size T and context window of size K and Markov chains defined on a finite state space of size O(T^K).
The stationary distribution is the long-term equilibrium of the Markov chain defined by the LLM and can be interpreted as a proxy of its understanding of natural language in its token space.

□ PHLOWER - Single cell trajectory analysis using Decomposition of the Hodge Laplacian
>> https://www.biorxiv.org/content/10.1101/2024.10.01.613179v1
PHLOWER uses the Hodge Laplacian (HL) and its associated Hodge decomposition. The zero-order Hodge Laplacianis a matrix representation of graphs, where samples are encoded as vertices and distances as edge weights, representing the nonlinear manifold of gene expression space.
PHLOWER uses a zero order Laplacian decomposition and random-walk to estimate pseudo-time (terminally differentiated cells) from progenitor cells. Next, cells with low (progenitors) and high pseudotime are connected and a simplicial complex is obtained by Delaunay triangulation.

□ CREATE: cell-type-specific cis-regulatory elements identification via discrete embedding
>> https://www.biorxiv.org/content/10.1101/2024.10.02.616391v1
CREATE (Cis-Regulatory Elements identificAtion via discreTe Embedding), a novel CNN-based supervised learning model that leverages the Vector Quantized Variational AutoEncoder (VQ-VAE) framework.
CREATE integrates genomic sequences w/ epigenetic features to offer a comprehensive approach for the identification and classification of multi-class CREs. VQ-VAE is particularly suited for this task because it can distill genomic and epigenomic data into discrete CRE embeddings.

□ Universal Cell Embeddings: A Foundation Model for Cell Biology
>> https://www.biorxiv.org/content/10.1101/2023.11.28.568918v2
Universal Cell Embedding (UCE) generates representations of new single-cell gene expression datasets with no model fine-tuning or retraining while still remaining robust to dataset and batch-specific artifacts.
UCE is a 33 layer model consisting of over 650 million parameters. UCE enables the mapping of new data into a universal embedding space, already populated with annotated reference states. This strategy addresses issues such as noisy measurements that limit data alignment.

□ Graphasing: phasing diploid genome assembly graphs with single-cell strand sequencing
>> https://genomebiology.biomedcentral.com/articles/10.1186/s13059-024-03409-1
Graphasing, a Strand-seq alignment-to-graph-based phasing and scaffolding workflow that assembles telomere-to-telomere (T2T) human haplotypes using data from a single sample.
Graphasing leverages a robust cosine similarity clustering approach to synthesize global phase signal from Strand-seq alignments with assembly graph topology, producing accurate haplotype calls and end-to-end scaffolds.

□ sylph: Rapid species-level metagenome profiling and containment estimation
>> https://www.nature.com/articles/s41587-024-02412-y
sylph is a statistical model based on zero-inflated Poisson statistics to debias containment ANI under low coverage, solving the low-abundance ANI calculation problem.
Sylph estimates the containment ANI between a reference genome and a shotgun metagenomic sample by searching the genome against the reads. Sylph measures the similarity of the reference genome to the metagenome and generalizes the standard genome-to-genome ANI.

□ Bio informatics: Integrate negative controls to get the good data
>> https://www.biorxiv.org/content/10.1101/2024.10.08.617225v1
COALISPR, a program for explicit and transparent application of negative control data in the comparison of high-throughput sequencing results.
This yields mapping coordinates that guide fast counting of reads, bypassing the need for a reference file, and is especially relevant when small RNA sequencing libraries contaminated with breakdown products are analysed for poorly annotated organisms.

□ C-ziptf: stable tensor factorization for zero-inflated multi-dimensional genomics data
>> https://bmcbioinformatics.biomedcentral.com/articles/10.1186/s12859-024-05886-4
Consensus-ZIPTF (C-ZIPTF) uses a novel factorization approach for high-dimensional sparse count data with excess zeros, namely Zero Inflated Poisson Tensor Factorization.
C-ZIPTF employs a stochastic optimization algorithm known as the Black Box Inference Algorithm. This algorithm operates by stochastically optimizing the variational objective using Monte Carlo samples from the variational distribution to compute the noisy gradient.

□ scMODAL: A general deep learning framework for comprehensive single-cell multi-omics data alignment with feature links
>> https://www.biorxiv.org/content/10.1101/2024.10.01.616142v1
scMODAL, a general deep learning framework for single-cell multi-omics data alignment with feature links. sMODAL is designed to integrate unpaired datasets with limited numbers of known positively correlated features, which are also referred as linked features in the literature.
scMODAL can project different single-cell datasets into a low-dimensional latent space and apply GANs to align cell embeddings. It utilizes prior information from known linked features to identify anchor cell pairs, while preserving topology structure of all input features.

□ PERT: Inferring replication timing and proliferation dynamics from single-cell DNA sequencing data
>> https://www.nature.com/articles/s41467-024-52544-7
PERT (Probabilistic Estimation of single-cell Replication Timing) infers S-phase cells and their scRT profiles from scWGS data. PERT jointly models RT and CN at a subclonal level which critically enables for high accuracy when analyzing samples with previously unseen RT and CN profiles.
PERT employs a Bayesian probabilistic model that takes observed scWGS binned read count as input and decomposes it into latent replication and somatic CN states which are then used to predict clone and cell cycle phase labels for all cells.

□ stFormer: a foundation model for spatial transcriptomics
>> https://www.biorxiv.org/content/10.1101/2024.09.27.615337v1
stFormer, a foundation model which incorporates ligand genes within spatial niches into Transformer encoders of single-cell transcriptomics. The model ultimately outputs the gene embeddings specific to the intracellular context and spatial niche.
stFormer calculates the self-attention among all gene embeddings within the center cell, then computes the cross-attention b/n these center cell gene embeddings and ligand gene embeddings, and finally propagates the gene embeddings through a two-layer feed-forward neural network.


□ MultiSC: a deep learning pipeline for analyzing multiomics single-cell data
>> https://academic.oup.com/bib/article/25/6/bbae492/7814652
MultiSC uses a single-cell hierarchical constraint autoencoder (scHCAE) for clustering cells and a matrix factorization–based model (scMF) for predicting gene regulatory network.
MultiSC utilizes multivariate linear regression to explore the gene regulatory relationship between TFs and target genes. MultiSC can also implement differential analysis, mediation analysis, and causal inference analysis for the multi-omics data.

□ STIX: Long-reads based Accurate Structural Variation Annotation at Population Scale
>> https://www.biorxiv.org/content/10.1101/2024.09.30.615931v1
STIX (Structural Variant Index) supports searching every discordant paired-end and split-read alignment from thousands of sample BAMs or CRAMs for the existence of an arbitrary SV.
STIX reports a per-sample count of all concurring evidence. From these counts we can, for example, conclude that an SV with high-level evidence in many samples is common and an SV with no evidence is rare.

□ Building pangenome graphs
>> https://www.biorxiv.org/content/10.1101/2023.04.05.535718v2
PanGenome Graph Builder (PGGB), a pipeline for constructing pangenome graphs without bias or exclusion. PGGB uses all-to-all alignments to build a variation graph in which we can identify variation, measure conservation, detect recombination events, and infer phylogenetic relationships.
The constructed graph is unbiased, i.e., all genomes are treated equivalently, regardless of input order or phylogenetic dependencies, and lossless: any input genome is completely retained in the graph and may be used as a frame of reference in downstream analysis.

□ ex-zd: A new compression strategy to reduce the size of nanopore sequencing data
>> https://www.biorxiv.org/content/10.1101/2024.10.02.616377v1
Ex-zd, a new data compression strategy that helps address the large size of raw signal data generated during nanopore experiments. Ex-zd encompasses both a lossless compression method, and a ‘lossy’ method, which can be used to achieve dramatic additional savings.
Ex-zd lossy compression uses a simple bit-reduction strategy. Ex-zd compresses the chain of sequential signal data values that make up a read, and should therefore be equally applicable to raw data written in ONT's FAST5 or POD5 format.

□ SAFAARI: Single-Cell Data Integration and Cell Type Annotation through Contrastive Adversarial Open-set Domain Adaptation
>> https://www.biorxiv.org/content/10.1101/2024.10.04.616599v1
SAFAARI can learn domain-invariant embedding and transfer labels in the presence of batch effects, biological domain shifts, and across diverse omics modalities using an adversarial domain adaptation strategy.
SAFAARI can identify novel cells not present in the reference dataset through Positive-Unlabeled Learning' and uses the synthetic minority oversampling technique (SMOTE) to mitigate class imbalance, enabling the annotation of rare cell types.
SAFAARI is a feedforward artificial neural network consisting of fully connected layers with nonlinear activation functions, which maps source and target cells into a shared low-dimensional latent space through representation learning.

□ BioLLMNet: Enhancing RNA-Interaction Prediction with a Specialized Cross-LLM Transformation Network
>> https://www.biorxiv.org/content/10.1101/2024.10.02.616044v1
BioLLMNet focuses on embedding processes for RNA, protein, and small molecules, as well as the transformation and gated combination of multimodal feature spaces. After transforming the feature spaces to the same dimensionality, BioLLMNet combines them using a gated mechanism.
BioLLMNet dynamically balances the contribution of each modality by learning a gate for each feature dimension. The gate parameters are learned via backpropagation, and the final prediction is made through a 3-layer deep neural network, optimized with a combined loss function.

□ scChat: A Large Language Model-Powered Co-Pilot for Contextualized Single-Cell RNA Sequencing Analysis
>> https://www.biorxiv.org/content/10.1101/2024.10.01.616063v1
scChat, a platform that combines quantitative statistical learning algorithms, LLMs, and research context to offer contextualized scRNA-seq data analysis capabilities. scChat serves as a copilot for scientists, enabling natural language interaction through a GUI.
sChat leverages LLMs to provide contextualized insights. These include validating research hypotheses, offering explanations for unexpected experimental outcomes, and suggesting next steps in experimental design, such as treatment strategies for patients.
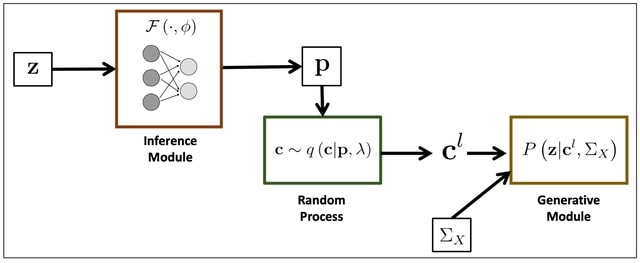
□ BEATRICE: Bayesian Fine-mapping from Summary Data using Deep Variational Inference
>> https://academic.oup.com/bioinformatics/advance-article/doi/10.1093/bioinformatics/btae590/7808857
BEATRICE, a novel Bayesian framework for fine-mapping that identifies potentially causal variants within GWAS risk loci through the shared LD structure. BEATRICE uses computationally efficient gradient-based optimization to minimize the KL divergence.
BEATRICE approximates the posterior probability of the causal locations via a binary concrete distribution. BEATRICE uses a new strategy to build a reduced set of causal configurations within the exponential search space that can be neatly folded into our optimization routine.

□ FindCSV: a long-read based method for detecting complex structural variations
>> https://bmcbioinformatics.biomedcentral.com/articles/10.1186/s12859-024-05937-w
FindCSV employs a multi-step approach. It first distinguishes and clusters different reads originating from both parents. Then, it generates consensus sequences based on the clustering results and performs remapping.
FindCSV determines CSVs by analyzing the new mapping results. The experimental results demonstrate that while the FindCSV algorithm performs slightly worse than SVcnn in detecting simple SVs, it outperforms the other methods in the detection of CSVs.

□ miniSNV: accurate and fast single nucleotide variant calling from nanopore sequencing data
>> https://academic.oup.com/bib/article/25/6/bbae473/7779241
miniSNV applies read pileup to recognize the candidate loci w/ divergences between reads and reference for variant calling. The candidate loci are divided into two categories, i.e. high- and low-quality loci, relying on the prebuilt variants and the complexity of the signatures.
miniSNV assigns the genotypes for high-quality loci by comparing the likelihoods of possible genotypes using a binomial model and uses WhatsHap, to phase all the heterozy-gotes and haplotag variants by the raw reads to generate haplotype-specific phased alignment.
miniSNV extracts all the overlapped reads and employs multiple sequence alignment or local assembly. miniSNV aligns the generated consensus sequence against the local reference sequence of the candidate region and identifies alternative alleles from the realigned information.

□ SAE-Impute: imputation for single-cell data via subspace regression and auto-encoders
>> https://bmcbioinformatics.biomedcentral.com/articles/10.1186/s12859-024-05944-x
SAE-Impute, a new computational method for imputing single-cell data by combining subspace regression and auto-encoders for enhancing the accuracy and reliability of the imputation process. A subspace regression method was employed to address missing values within the dataset.
SAE-Impute reduces false negative signals and enhances the retrieval of dropout values, gene-gene and cell-cell correlations. It captures the intrinsic relationships within the data through a linear combination of observations, enhancing the accuracy of interpolation.

□ scGNN+: Adapting ChatGPT for Seamless Tutorial and Code Optimization
>> https://www.biorxiv.org/content/10.1101/2024.09.30.615735v1
The scGNN+ workflow utilizes dual GPT-4 engines (Duo-GPT) to translate user queries and tutorials into executable commands and code. Duo-GPT outperformed the single GPT model in code localization and customization tasks.
scGNN+ is developed with strict code standards within the fed code for both the ScGNN model and analysis procedure pipeline codes. This not only improves the accuracy of code generation but also enables GPT to provide clear explanations for the generated code.

□ GoldPolish-Target: Targeted long-read genome assembly polishing
>> https://www.biorxiv.org/content/10.1101/2024.09.27.615516v1
GoldPolish-Target, a modular targeted sequence polishing pipeline. Coupled with GoldPolish, a linear-time genome assembly algorithm, GoldPolish-Target isolates user-specified assembly loci, offering a resource-efficient means for polishing targeted regions of draft genomes.
GP-Target improves polishing accuracy. Instead of generating one Bloom filter for each k-mer size per 'goldtig', GP-Target generates Bloom filters for each k-mer size per target region, only using reads mapped specifically to the target region, for error correction.

□ ScRNAbox: empowering single-cell RNA sequencing on high performance computing systems
>> https://bmcbioinformatics.biomedcentral.com/articles/10.1186/s12859-024-05935-y
scRNAbox, an innovative scRNAseq analysis pipeline meticulously crafted for HPC systems. This end-to-end solution, executed via the SLURM workload manager, efficiently processes raw data from standard and Hashtag samples.
scRNAbox incorporates quality control filtering, sample integration, clustering, cluster annotation tools, and facilitates cell type-specific differential gene expression analysis between two groups.


□ SAMURAI: Shallow Analysis of Copy nuMber alterations Using a Reproducible And Integrated bioinformatics pipeline
>> https://www.biorxiv.org/content/10.1101/2024.09.30.615766v1
SAMURAI integrates different methods for preprocessing data, performing CNA analysis, along with optional post-processing steps, leveraging the nf-core standards and vast array of pre-made analysis modules.
SAMURAI presents a matrix of normalized signature activities alongside a bar plot summarizing these activities, allowing users to easily interpret the CIN landscape within the report.

□ PoreMeth2: decoding the evolution of methylome alterations with Nanopore sequencing
>> https://www.biorxiv.org/content/10.1101/2024.10.03.616449v1
PoreMeth2 is an R package for the identification of Differentially Methylated Regions from Nanopore methylation data (inferred by methcallers such as Nanopolish, DeepSignal, Dorado or Guppy) of paired samples and for their functional interpretation.
The BiSLM algorithm and the novel annotation scheme were integrated PoreMeth2 that allows to automatically identify and annotate DMRs by comparing the Nanopore methylation data of a pair of test and matched normal samples.
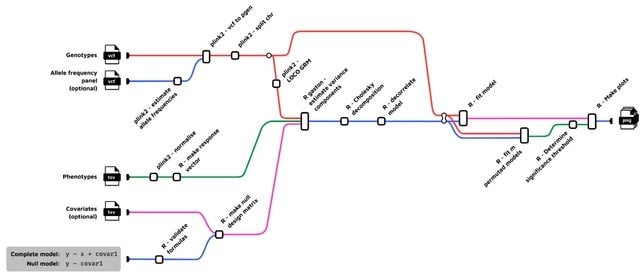
□ FlexLMM: a Nextflow linear mixed model framework for GWAS
>> https://arxiv.org/abs/2410.01533
birneylab/flexlmm is a bioinformatics pipeline that runs linear mixed models for Genome-Wide Association Studies. FlexLMM can natively run permutations. The main issue with permutations in LMMs is the fact that the samples are not exchangeable under the null hypothesis.
FlexLMM can take in input an arbitrary statistical model for the fixed terms (for example it is possible to modify the genotype encoding to account for dominance), and compares it to an arbitrary null model via a likelihood ratio test.
FlexLMM estimates the variance-covariance structure from the datasets, and regresses it out from the phenotype and design matrix. Only then the genotypes are jointly permuted, preserving the correlation structure across genetic markers and the exchangeability of the samples.

□ RTF: An R package for modelling time course data
>> https://academic.oup.com/bioinformatics/advance-article/doi/10.1093/bioinformatics/btae597/7816322
The RTF R package implements the Retarded Transient Function (RTF) approach for modeling time- and dose-dependent responses typically observed in signaling pathways.
The package simplifies the fitting of the RTF using nonlinear optimization and offers additional functionalities, such as model reduction and low-dimensional representation of signaling compound dynamics.

□ Intelligence at the Edge of Chaos
>> https://arxiv.org/abs/2410.02536
Elementary Cellular Automata (ECAs) are a type of one-dimensional cellular automaton where each cell has a binary state, and its next state is determined by a simple rule that depends only on the current state of the cell and its two immediate neighbors.
Utilizing LLMs trained on elementary cellular automata (ECA) to study how intelligent behavior may emerge in large language models (LLMs) when trained on increasingly complex systems.
The best model performance occurs in systems operating at high but not excessive complexity, previously referred to as the "edge of chaos". Models trained on Class IV ECA rules, suggesting that intelligence may emerge in systems that balance predictability and complexity.

□ CONSTRUCT: an algorithmic tool for identifying functional or structurally important regions in protein tertiary structure
>> https://www.biorxiv.org/content/10.1101/2024.10.07.617015v1
CONSTRUCT is a software tool designed to identify functional and structurally important sites in proteins by searching amino acid sites evolving under strong purifying selection that cluster together in 3D structure.
CONSTRUCT calculates site-specific substitution rates using the Rate4site model, which are then weighted by the rates of neighboring amino acid sites within a range of window sizes. The optimal window size is determined by the strongest spatial correlation, if present.
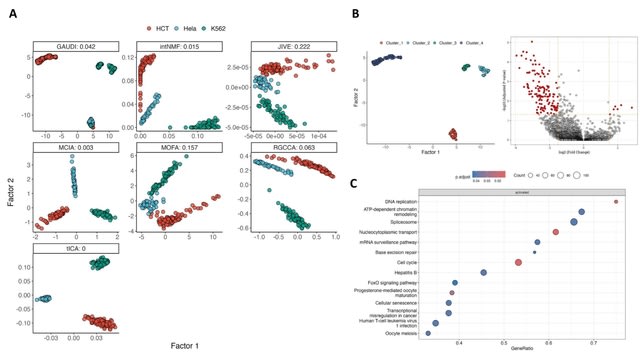
□ GAUDI: Interpretable multi-omics integration with UMAP embeddings and density-based clustering
>> https://www.biorxiv.org/content/10.1101/2024.10.07.617035v1
Group Aggregation via UMAP Data Integration (GAUDI) concatenates the individual UMAP embeddings into a unified dataset and then applies a second UMAP to this concatenated dataset. This step combines the distinct omics layers into a single, lower-dimensional representation.
GAUDI then employs Hierarchical Density-Based Spatial Clustering of Applications with Noise (HDBSCAN). HDBSCAN is effective as it handles clusters of varying densities and irregular shapes without assuming a predefined number of clusters.
GAUDI computes metagenes using a XBoost model to synthesize molecular features. This involves using XGBoost to predict UMAP embedding coordinates from molecular features and identifying key features that influence the positioning of samples in the integrated latent space.

□ LAMAR: Deciphering RNA regulation with a foundation language model
>> https://www.biorxiv.org/content/10.1101/2024.10.12.617732v1
LAMAR can effectively distinguish between regulatory elements or transcripts with distinct functions within the representation space, indicating that it has successfully captured the functional attributes solely from sequences.
LAMAR was leveraged as a foundation platform to fine-tune this pretrained model with labeled datasets across a range of tasks including supervised modeling of splice sites, translation efficiency, internal ribosome entry sites (IRESs), and degradation.

□ zMAP toolset: model-based analysis of large-scale proteomic data via a variance stabilizing z-transformation
>> https://genomebiology.biomedcentral.com/articles/10.1186/s13059-024-03382-9
zMAP toolset transforms each ILMS intensity into a z-statistic that essentially assesses the statistical significance of the deviation of this measurement from that (of the same protein) in the corresponding reference sample.
reverse-zMAP module is to fit sample-specific MVCs by separately comparing each sample to the corresponding reference sample, for which the M-values of all proteins are calculated and a sliding window is used to group proteins with close intensity levels.
※コメント投稿者のブログIDはブログ作成者のみに通知されます