
(Created with Midjourney v6.1)

□ Dynaformer: From Static to Dynamic Structures: Improving Binding Affinity Prediction with Graph-Based Deep Learning
>> https://onlinelibrary.wiley.com/doi/10.1002/advs.202405404
Dynaformer, a graph transformer framework to predict the binding affinities by learning the geometric characteristics of the protein-ligand interactions from the MD trajectories.
Dynaformer utilizes a roto-translation invariant feature encoding scheme, taking various interaction characteristics into account, including interatomic distances, angles between bonds, and various types of covalent or non-covalent interactions.


□ OmniBioTE: Large-Scale Multi-omic Biosequence Transformers for Modeling Peptide-Nucleotide Interactions
>> https://arxiv.org/abs/2408.16245
OmniBioTE is a large-scale multimodal biosequence transformer model that is designed to capture the complex relationships in biological sequences such as DNA, RNA, and proteins. OmniBioTE pushes the boundaries by jointly modeling nucleotide and peptide sequence.
Multi-omic biosequence transformers emergently learn useful structural information without any prior structural training. OmniBioTE excels in predicting peptide-nucleotide interactions, specifically the Gibbs free energy changes (ΔG) and the effects of mutations (ΔΔG).

□ TIANA: transcription factors cooperativity inference analysis with neural attention
>> https://bmcbioinformatics.biomedcentral.com/articles/10.1186/s12859-024-05852-0
TIANA (Transcription factors cooperativity Inference Analysis with Neural Attention), an MHA-based framework to infer combinatorial TF cooperativities from epigenomic data.
TIANA uses known motif weights to initialize convolution filters to ease the interpretation challenge, allowing convolution filter activations to be directly associated with known TF motifs.
TIANA uses integrated gradients to interpret the TF interdependencies from the attention units. We tested TIANA’s ability to recover TF co-binding pair motifs from ChIP-seq data, demonstrating that TIANA could identify key co-occurring TF motif pairs.

□ Amethyst: Single-cell DNA methylation analysis tool Amethyst reveals distinct noncanonical methylation patterns in human glial cells
>> https://www.biorxiv.org/content/10.1101/2024.08.13.607670v1
Amethyst is capable of efficiently processing data from hundreds of thousands of high-coverage cells in a relatively short time frame by performing initial computationally-intensive steps on a cluster followed by rapid local interaction of the output in RStudio.
By default, Amethyst calculates fast truncated singular values with the implicitly restarted Lanczos bidiagonalization algorithm (IRLBA). Amethyst provides a helper function for estimating how many dimensions are needed to achieve the desired amount of variance explained.

□ GITIII: Investigation of pair-wise single-cell interactions by statistically interpreting spatial cell state correlation learned by self-supervised graph inductive bias transformer
>> https://www.biorxiv.org/content/10.1101/2024.08.21.608964v1
GITIII (Graph Inductive Transformer for Intercellular Interaction Investigation), an interpretable self-supervised graph transformer-based language model that treats cells as words (nodes) and their cell neighborhood as a sentence to explore the communications among cells.
Enhanced by multilayer perceptron-based distance scaler, physics-informed attention, and graph transformer model, GITIII infers CCI by investigating how the state of a cell is influenced by the spatial organization, ligand expression, cell types and states of neighboring cells.
GITIII employs the Graph Inductive Bias Transformer (GRIT) model which encodes input tensors in a language model manner. It effectively encodes both the graph structure and expression profiles within cellular neighborhoods.
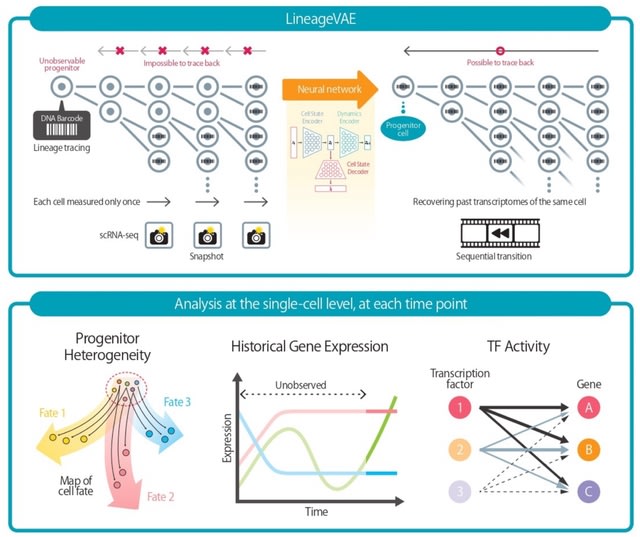
□ LineageVAE: Reconstructing Historical Cell States and Transcriptomes toward Unobserved Progenitors
>> https://academic.oup.com/bioinformatics/advance-article/doi/10.1093/bioinformatics/btae520/7738923
LineageVAE is a deep generative model that transforms scRNA-seq observations with identical lineage barcodes into sequential trajectories toward a common progenitor in a latent cell state space.
LineageVAE depicts sequential cell state transitions from simple snapshots and infers cell states over time. It generates transcriptomes at each time point using a decoder. LineageVAE utilizes the property that the progenitors of cells introduced with a shared barcode are identical.
LineageVAE can reconstruct the historical cell states and their expression profiles from the observed time point toward these progenitor cells under the constraint that the cell state of each lineage converges to the progenitor state.

□ tombRaider: improved species and haplotype recovery from metabarcoding data through artefact and pseudogene exclusion.
>> https://www.biorxiv.org/content/10.1101/2024.08.23.609468v1
tombRaider, an open-source software package for improved species and
haplotype recovery from metabarcoding data through accurate artefact and pseudogene exclusion.
tombRaider features a modular algorithm capable of evaluating multiple criteria, including sequence similarity, co-occurrence patterns, taxonomic assignment, and the presence of stop codons.

□ PICASO: Profiling Integrative Communities of Aggregated Single-cell Omics data
>> https://www.biorxiv.org/content/10.1101/2024.08.28.610120v1
PICASO creates biomedical networks to identify explainable disease-associated gene communities and potential drug targets by using gene-regulatory network modeling on biomedical network representations.
The PICASO architecture can be used to embed single-cell transcriptomics data within a plentitude of available biomedical databases such as OpenTargets, Omnipath, GeneOntology, KEGG, STRING, Reactomeand Uniprot, and extract condition specific communities and associations.
The full PICASO network consists of 111032 nodes and 1617389 edges collected from the above 7 disparate resources. PICASO provides an implementation for calculating node and edge scores within the network by the MeanNetworkScorer.

□ LoRNASH: A long context RNA foundation model for predicting transcriptome architecture
>> https://www.biorxiv.org/content/10.1101/2024.08.26.609813v1
LoRNASH, the long-read RNA model with StripedHyena, an RNA foundation model that learns how the nucleotide sequence of unspliced pre-mRNA dictates transcriptome architecture-the relative abundances and molecular structures of mRNA isoforms.
LoRNASH uses causal language modeling and an expanded RNA token set. LoRNAS handles extremely long sequence inputs (~65 kilobase pairs), allowing for zero-shot prediction of all aspects of transcriptome architecture, incl isoform structure and the impact of DNA sequence variants.

□ pyVIPER: A fast and scalable Python package for rank-based enrichment analysis of single-cell RNASeq data
>> https://www.biorxiv.org/content/10.1101/2024.08.25.609585v1
pyVIPER, a fast, memory-efficient, and highly scalable Python-based VIPER implementation. The pyVIPER package leverages AnnData objects and is seemingly integrated with standard single cell analysis packages, such as Scanpy and others from the scverse ecosystem.
pyVIPER can directly interface with scikit-learn and TensorFlow to allow plug-and-play ML analyses that leverage VIPER-assessed protein activity profiles. pyVIPER scales more efficiently with the number of cells, enabling the analysis of 4x cells with the same memory allocation.

□ A Bioinformatician, Computer Scientist, and Geneticist lead bioinformatic tool development - which one is better?
>> https://www.biorxiv.org/content/10.1101/2024.08.25.609622v1
Medical Informatics is identified as the top-performing group in developing accurate bioinformatic software tools. The tools include a number of methods for structural variation detection, single-cell profiling, long-read assembly, multiple sequence alignment.
Bioinformatics and Engineering ranked lower in terms of software accuracy. Tools developed by authors who affiliated with "Bioinformatics" typically had slightly lower accuracy than that of other fields. However, this was not a statistically significant finding.

□ TRACS: Enhanced metagenomics-enabled transmission inference
>> https://www.biorxiv.org/content/10.1101/2024.08.19.608527v1
TRACS (TRAnsmision Clustering of Strains), a highly accurate and easy-to-use algorithm for establishing whether two samples are plausibly related by a recent transmission event.
The TRACS algorithm distinguishes the transmission of closely related strains by identifying genetic differences as small as a few Single Nucleotide Polymorphisms (SNP)s, which is crucial when considering slow-evolving pathogens.
TRACS was designed to estimate a lower bound of the SNP distance and can incorporate sampling date information. TRACS controls for major sources of error including variable sequencing coverage, within-species recombination and sequencing errors.

□ Pandagma: A tool for identifying pan-gene sets and gene families at desired evolutionary depths and accommodating whole genome duplications
>> https://academic.oup.com/bioinformatics/advance-article/doi/10.1093/bioinformatics/btae526/7740678
Pandagma provides methods for efficiently and sensitively identifying pangene and gene family sets for annotation sets from eukaryotic genomes, with methods for handling polyploidy and for targeting family construction at specified taxonomic depths.
Pandagma is a set of configurable workflows for identifying and comparing pan-gene sets and gene families for annotation sets from eukaryotic genomes, using a combination of homology, synteny, and expected rates of synonymous change in coding sequence.

□ diffGEK: Differential Gene Expression Kinetics
>> https://www.biorxiv.org/content/10.1101/2024.08.21.608952v1
diffGEK assumes that rates can vary over a trajectory, but are smooth functions of the differentiation process. diffGEK initially estimates per-cell and per-gene kinetic parameters using known lineage and pseudo-temporal ordering of cells for a specific condition.
diffGEK integrates a statistical strategy to discern whether a gene exhibits differential kinetics between any two biological con-ditions, across all possible permutations.

□ GTAM: A Molecular Pretraining Model with Geometric Triangle Awareness
>> https://academic.oup.com/bioinformatics/advance-article/doi/10.1093/bioinformatics/btae524/7739699
Geometric Triangle Awareness Model (GTAM). GTAM aims to maximize the mutual information using contrastive self-supervised learning (SSL) and generative SSL. GTAM uses diffusion generative models for generative SSL which can lead to a more accurate estimation in generative SSL.
GTAM employs the new molecular encoders that incorporate a novel geometric triangle awareness mechanism to enhance edge-to-edge updates in molecular representation learning, in addition to node-to-edge and edge-to-node updates, unlike other molecular graph encoders.
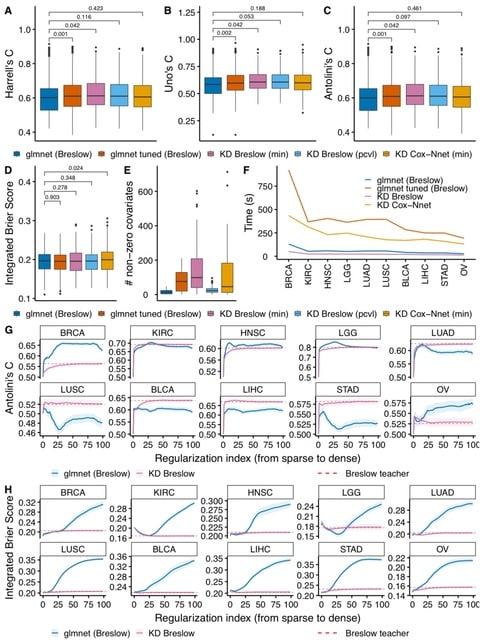
□ sparsesurv: A Python package for fitting sparse survival models via knowledge distillation
>> https://academic.oup.com/bioinformatics/advance-article/doi/10.1093/bioinformatics/btae521/7739697
sparsesurv, a Python package that contains a set of teacher-student model pairs, including the semi-parametric accelerated failure time and the extended hazards models as teachers.
sparsesurv also contains in-house survival function estimators, removing the need for external packages. Sparsesurv is validated against R-based Elastic Net regularized linear Cox proportional hazards models, based on kernel-smoothing the profile likelihood.

□ GOLDBAR: A Framework for Combinatorial Biological Design
>> https://pubs.acs.org/doi/10.1021/acssynbio.4c00296
GOLDBAR, a combinatorial design framework. GOLDBAR enables synthetic biologists to intersect and merge the rules for entire classes of biological designs to extract common design motifs and infer new ones.
GOLDBAR can refine/validate design spaces for TetR-homologue transcriptional logic circuits, verify the assembly of a partial nif gene cluster, and infer novel gene clusters for the biosynthesis of rebeccamycin.
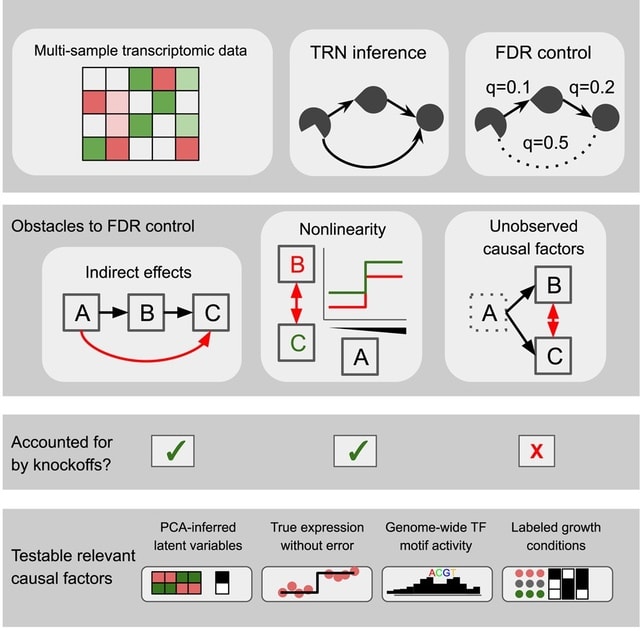
□ Model-X knockoffs: Transcriptome data are insufficient to control false discoveries in regulatory network inference
>> https://www.cell.com/cell-systems/fulltext/S2405-4712(24)00205-9
This approach centers on a recent innovation in high-dimensional statistics: model-X knockoffs. Model-X knockoffs were originally intended to be applied to individual regression problems, not network inference.
Model-X knockoffs builds a network by regressing each gene on all other genes. If done naively, this process requires time proportional to the fourth power of the number of genes. Model-X uses Gaussian knockoffs with covariance equal to the sample covariance matrix.

□ Seqrutinator: scrutiny of large protein superfamily sequence datasets for the identification and elimination of non-functional homologues
>> https://genomebiology.biomedcentral.com/articles/10.1186/s13059-024-03371-y
Seqrutinator is an objective, flexible pipeline that removes sequences with sequencing and/or gene model errors and sequences from pseudogenes from complex, eukaryotic protein superfamilies.
Seqrutinator removes Non-Functional Homologues (NFHs) rather than FHs. Pseudogenes have no functional constraint and an elevated evolutionary rate by which they stand out in phylogenies.

□ SQANTI-reads: a tool for the quality assessment of long read data in multi-sample lrRNA-seq experiments.
>> https://www.biorxiv.org/content/10.1101/2024.08.23.609463v1
SQANTI-reads leverages SQANTI3, a tool for the analysis of the quality of transcript models, to develop a quality control protocol for replicated long-read RNA-seq experiments.
The number/distribution of reads, as well as the number/distribution of unique junction chains (transcript splicing patterns), in SQANTI3 structural categories are compiled. Multi-sample visualizations of QC metrics can also be separated by experimental design factors.

□ IL-AD: Adapting nanopore sequencing basecalling models for modification detection via incremental learning and anomaly detection
>> https://www.nature.com/articles/s41467-024-51639-5
IL-AD leverages machine learning approaches to adapt nanopore sequencing basecallers for nucleotide modification detection. It applies the incremental learning technique to improve the basecalling of modification-rich sequences, which are usually of high biological interests.
With sequence backbones resolved, IL-AD further runs anomaly detection on individual nucleotides to determine their modification status. By this means, IL-AD promises the single-molecule, single-nucleotide and sequence context-free detection of modifications.

□ grenedalf: Population genetic statistics for the next generation of Pool sequencing
>> https://academic.oup.com/bioinformatics/advance-article/doi/10.1093/bioinformatics/btae508/7741639
grenedalf, a command line tool to compute widely-used population genetic statistics for Pool-seq data. It aims to solve the shortcomings of previous implementations, and is several orders of magnitude faster, scaling to thousands of samples.
The core implementation of the command line tool grenedalf is part of GENESIS, the high-performance software library for working with phyogenetic and population genetic data.

□ Eliater: A Python package for estimating outcomes of perturbations in biomolecular networks
>> https://academic.oup.com/bioinformatics/advance-article/doi/10.1093/bioinformatics/btae527/7742268
Eliater checks the mutual consistency of the network structure and observational data with conditional independence tests, checks if the query is estimable from the available observational data.
Eliater detects and removes nuisance variables unnecessary for causal query estimation, generates a simpler network, and identifies the most efficient estimator of the causal query. Eliater returns an estimated quantitative effect of the perturbation.

□ funkea: Functional Enrichment Analysis in Python
>> https://www.biorxiv.org/content/10.1101/2024.08.24.609502v1
funkea, a Python package containing popular functional enrichment methods, leveraging Spark for effectively infinite scale. All methods have been unified into a single interface, giving users the ability to easily plug-and-play different enrichment approaches.
The variant selection and locus definitions are composed by the user, but each of the enrichment methods provided by funkea provide default configurations. The user can also define their own annotation component, which is required for all enrichment methods.

□ ARGV: 3D genome structure exploration using augmented reality
>> https://bmcbioinformatics.biomedcentral.com/articles/10.1186/s12859-024-05882-8
ARGV, an augmented reality 3D Genome Viewer. ARGV contains more than 350 pre-computed and annotated genome structures inferred from Hi-C and imaging data. It offers interactive and collaborative visualization of genomes in 3D space, using standard mobile phones or tablets.
ARGV allows users to overlay multiple annotation tracks onto a 3D chromosome model. ARGV is equipped with a database currently containing 343 whole-genome, high-resolution 3D models and annotations inferred from Hi-C and omics data, as well as several imaging-based structures.

□ NERD-seq: a novel approach of Nanopore direct RNA sequencing that expands representation of non-coding RNAs
>> https://genomebiology.biomedcentral.com/articles/10.1186/s13059-024-03375-8
NERD-seq expands the ncRNA representation in Nanopore direct RNA-seq to include multiple additional classes of ncRNAs genome-wide, while maintaining at the same time the ability to sequence high library complexity mRNA transcriptomes.
NERD-seq enables the generation of reads with higher coverage for the non-coding genome, while still detecting mRNAs and poly(A) ncRNAs. NERD-seq allows the successful detection of snoRNAs, snRNAs, scRNAs, srpRNAs, tRNAs, and other ncRNAs.

□ OrthoBrowser: Gene Family Analysis and Visualization
>> https://www.biorxiv.org/content/10.1101/2024.08.27.609986v1
OrthoBrowser, a static site generator that will index and serve phylogeny, gene trees, multiple sequence alignments, and novel multiple synteny alignments. This greatly enhances the usability of tools like OrthoFinder by making the detailed results much more visually accessible.
OrthoBrowser can scale reasonably up to hundreds of genomes. The multiple synteny alignment method uses a progressive hierarchical alignment approach in the protein space using orthogroup membership to establish orthology.

□ GageTracker: a tool for dating gene age by micro- and macro-synteny with high speed and accuracy
>> https://www.biorxiv.org/content/10.1101/2024.08.28.610050v1
Based on the micro- and macro-synteny algorithm, GageTracker was a one-command running software to search ortholog genome alignments suitable for multiple species and allow a fast and accurate trace gene age with minimal user inputs.
It obtained a high alignment quality as the optimized LastZ software but significantly saved the running time as well. GageTracker also showed a slightly higher support rate from orthoDB, FlyBase, and Ensembl ortholog database than the Gentree database.

□ Enhancement of network architecture alignment in comparative single-cell studies
>> https://www.biorxiv.org/content/10.1101/2024.08.30.608255v1
scSpecies pre-trains a conditional variational autoencoder-based model and fully re-initializes the encoder input layers and the decoder network during fine-tuning.
scSpecies aligns context scRNA-seq datasets with human target data, enabling the analysis of similarities and differences b/n the datasets. scSpecies enables nuanced comparisons of gene expression profiles by generating GE values for both species from a single latent variable.


□ LexicMap: efficient sequence alignment against millions of prokaryotic genomes
>> https://www.biorxiv.org/content/10.1101/2024.08.30.610459v1
LexicMap, a nucleotide sequence alignment tool for efficiently querying moderate length sequences (over 500 bp) such as a gene, plasmid or long read against up to millions of prokaryotic genomes.
A key innovation is to construct a small set of probe k-mers (e.g. n = 40,000) which "window-cover" the entire database to be indexed, in the sense that every 500 bp window of every database genome contains multiple seed k-mers each with a shared prefix with one of the probes.
Storing these seeds, indexed by the probes with which they agree, in a hierarchical index enables fast and low-memory variable-length seed matching, pseudoalignment, and then full alignment.
LexicMap is able to align with higher sensitivity than Blastn as the query divergence drops from 90% to 80% for queries ≥ 1 kb. Alignment of a single gene against 2.34 million prokaryotic genomes from GenBank and RefSeq takes 36 seconds (rare gene) to 15 minutes (16S RNA gene).

□ Enhlink infers distal and context-specific enhancer–promoter linkages
>> https://genomebiology.biomedcentral.com/articles/10.1186/s13059-024-03374-9
Enhlink detects biological effects and controls technical effects by incorporating appropriate covariates into a nonlinear modeling framework involving single cells, rather than aggregates.
Enhlink selects a parsimonious set of enhancers associated with a promoter to smooth the sparse representation of any individual enhancer while prioritizing those with the largest effect.
Enhlink uses a random forest-like approach, where cell-level (binary) accessibilities of enhancers and biological and technical factors are features and the cell-level accessibility of a promoter is the response variable.
Enhlink can further prioritize enhancers by associating them with the expression of the promoter’s target gene. Enhlink has the ability to predict both proximal and distal enhancer–gene linkages and identify linkage specific to biological covariates.

□ COBRA: Higher-order correction of persistent batch effects in correlation networks
>> https://academic.oup.com/bioinformatics/advance-article/doi/10.1093/bioinformatics/btae531/7748404
COBRA (Co-expression Batch Reduction Adjustment), a method for computing a batch-corrected gene co-expression matrix based on estimating a conditional covariance matrix.
COBRA estimates a reduced set of parameters expressing the co-expression matrix as a function of the sample covariates, allowing control for continuous and categorical covariates.
※コメント投稿者のブログIDはブログ作成者のみに通知されます