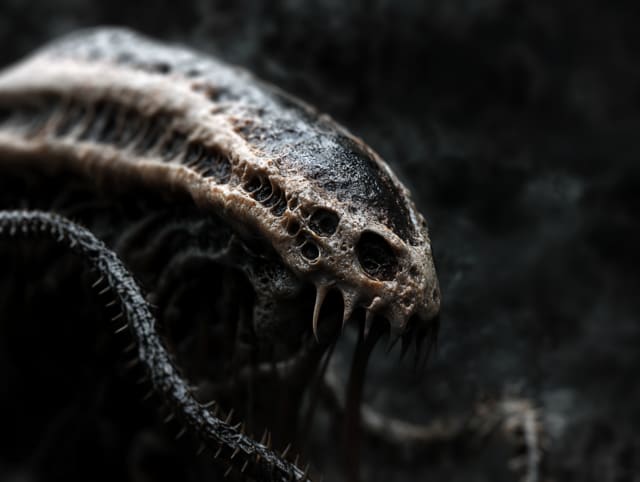
(Created with Midjourney v6.1)

□ scCello: Cell-ontology guided transcriptome foundation model https://arxiv.org/abs/2408.12373
scCello (single cell, Cell-ontology guided TFM) learns cell representation by integrating cell type information and cellular ontology relationships into its pre-training framework.
scCello's pre-training framework is structured with three levels of objectives:
Gene level: a masked token prediction loss to learn gene co-expression patterns. Intra-cellular level: an ontology-based cell-type coherence loss to encourage cell representations of the same cell type to aggregate. Inter-cellular level: a relational alignment loss to guide the cell representation learning by consulting the cell-type lineage from the cell ontology graph.
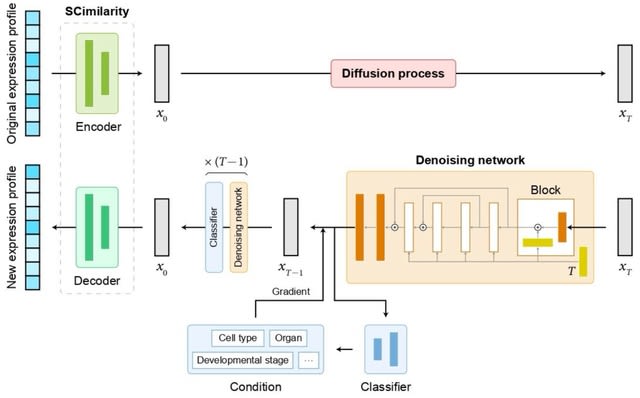
□ scDiffusion: Conditional generation of high-quality single-cell data using diffusion model
>> https://academic.oup.com/bioinformatics/advance-article/doi/10.1093/bioinformatics/btae518/7738782
scDiffusion, an in silico scRNA-seq data generation model combining latent diffusion model (LDM) w/ the foundation model, to generate single-cell gene expression data with given conditions. scDiffusion has 3 parts, an autoencoder, a denoising network, and a condition controller.
scDiffusion employs the pre-trained model SCimilarity as an autoencoder to rectify the raw distribution and reduce the dimensionality of scRNA-seq data, which can make the data amenable to diffusion modeling.
The denoising network was redesigned based on a skip-connected multilayer perceptron (MLP) to learn the reversed diffusion process. scDiffusion uses a new condition control strategy, Gradient Interpolation, to interpolate continuous cell trajectories from discrete cell states.
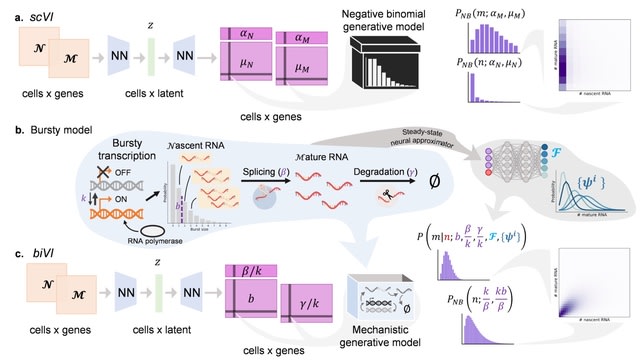
□ biVI: Biophysical modeling with variational autoencoders for bimodal, single-cell RNA sequencing data
>> https://www.nature.com/articles/s41592-024-02365-9
biVI combines the variational autoencoder framework of scVI w/ biophysical models describing the transcription and splicing kinetics. Bivariate distributions arising from biVI models can be used in variational autoencoders for principled integration of unspliced and spliced data.
biVI retains the variational autoencoder’s ability to capture cell type structure in a low-dimensional space while further enabling genome-wide exploration of the biophysical mechanisms, such as system burst sizes and degradation rates, that underlie observations.
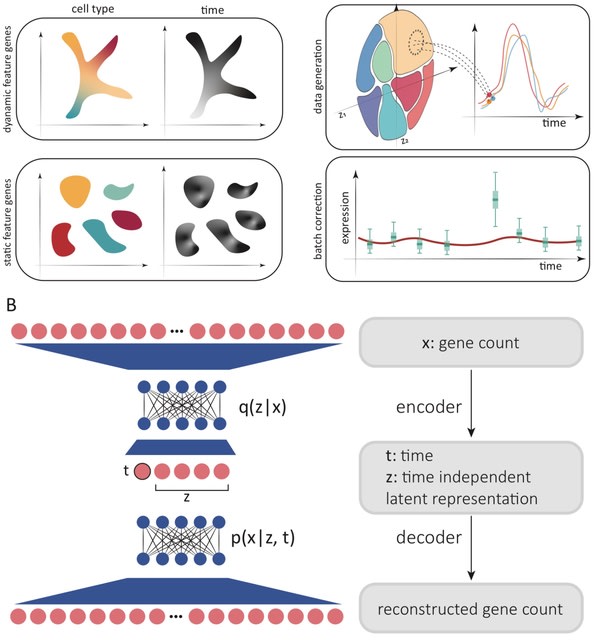
□ SNOW: Variational inference of single cell time series
>> https://www.biorxiv.org/content/10.1101/2024.08.29.610389v1
SNOW (SiNgle cell flOW map), a deep learning algorithm to deconvolve single cell time series data into time--dependent and time--independent contributions. SNOW enables cell type annotation based on the time--independent dimensions.
SNOW yields a probabilistic model that can be used to discriminate between biological temporal variation and batch effects contaminating individual timepoints, and provides an approach to mitigate batch effects.
SNOW is capable of projecting cells forward and backward in time, yielding time series at the individual cell level. This enables gene expression dynamics to be studied without the need for clustering or pseudobulking, which can be error prone and result in information loss.
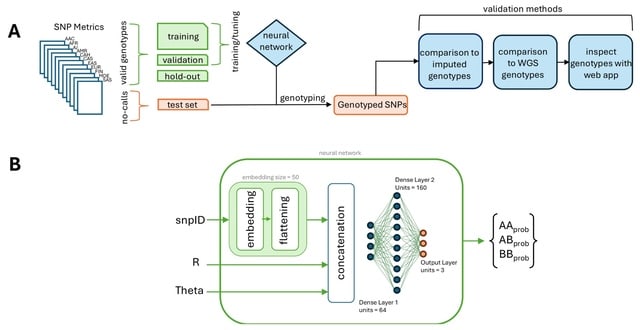
□ Cluster Buster: A Machine Learning Algorithm for Genotyping SNPs from Raw Data
>> https://www.biorxiv.org/content/10.1101/2024.08.23.609429v1
Cluster Buster is a system for recovering the genotypes of no-call SNPs on the Neurobooster array after genotyping with the Illumina Gencall algorithm. It is a genotype-predicting neural network and SNP genotype plotting system.
In the Cluster Buster workflow, SNP metrics files from all available ancestries in GP2 are split into valid gencall SNPs and no-call SNPs. Valid genotypes are split 80-10-10 for training, validation, and testing of the neural network. The trained neural network is then applied to no-call SNPs.
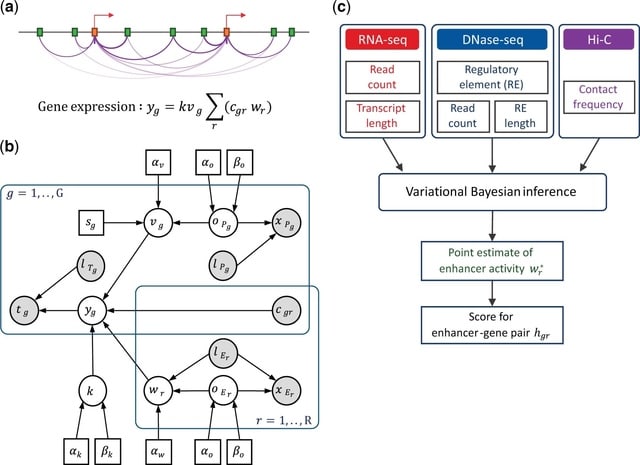
□ IVEA: an integrative variational Bayesian inference method for predicting enhancer–gene regulatory interactions
>> https://academic.oup.com/bioinformaticsadvances/article/4/1/vbae118/7737507
IVEA, an integrative variational Bayesian inference of regulatory element activity for predicting enhancer–gene regulatory interactions. Gene expression is modelled by hypothetical promoter/enhancer activities, which reflect the regulatory potential of the promoters/enhancers.
Using transcriptional readouts and functional genomic data of chromatin accessibility, promoter and enhancer activities were estimated through variational Bayesian inference, and the contribution of each enhancer–promoter pair to target gene transcription was calculated.
<br/ >
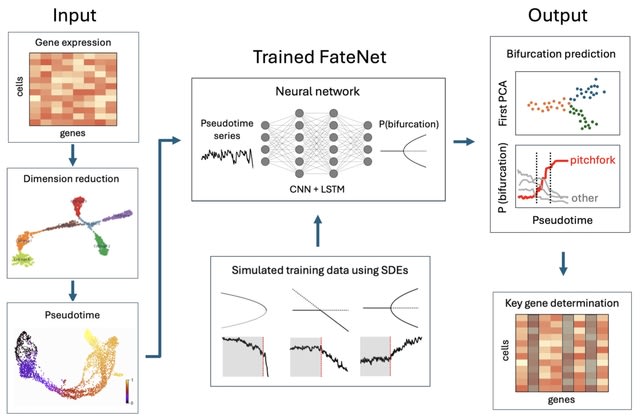
□ FateNet: an integration of dynamical systems and deep learning for cell fate prediction
>> https://academic.oup.com/bioinformatics/advance-article/doi/10.1093/bioinformatics/btae525/7739702
FateNet, a novel computational model that combines the theory of dynamical systems and deep learning to predict cell fate decision-making using scRNA-seq data. FateNet leverages universal properties of bifurcations such as scaling behavior and normal forms.
FateNet learns to predict and distinguish different bifurcations in pseudotime simulations of a 'universe' of different dynamical systems. The universality of these properties allows FateNet to generalise to high-dimensional gene regulatory network models and biological data.
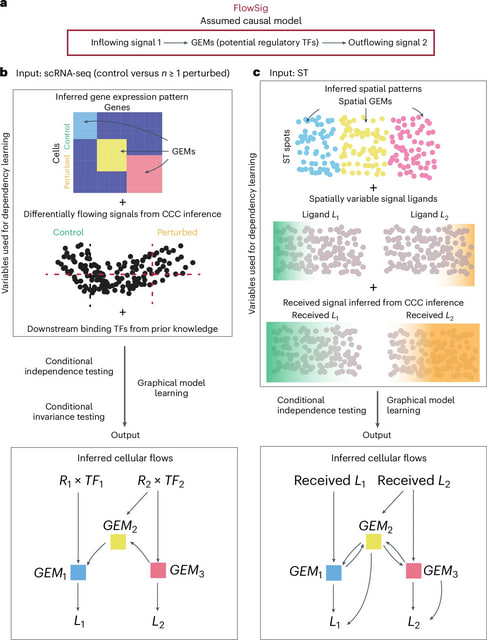
□ FlowSig: Inferring pattern-driving intercellular flows from single-cell and spatial transcriptomics
>> https://www.nature.com/articles/s41592-024-02380-w
FlowSig, a method that identifies ligand–receptor interactions whose inflows are mediated by intracellular processes and drive subsequent outflow of other intercellular signals.
FlowSig learns a completed partial directed acyclic graph (CPDAG) describing intercellular flows between three types of constructed variables: inflowing signals, intracellular gene modules and outflowing signals.
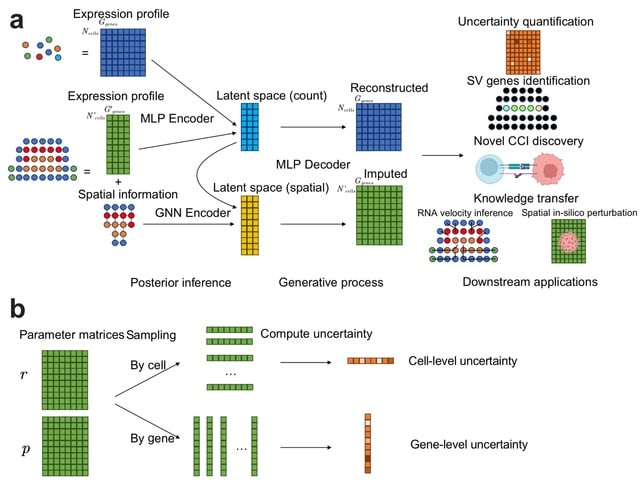
□ VISTA Uncovers Missing Gene Expression and Spatial-induced Information for Spatial Transcriptomic Data Analysis
>> https://www.biorxiv.org/content/10.1101/2024.08.26.609718v1
VISTA leverages a novel joint probabilistic modeling approach to predict the expression levels of unobserved genes. VISTA jointly models scRNA-seq data and SST data based on variational inference and geometric deep learning, and incorporates uncertainty quantification.
VISTA uses a Multi-Layer Perceptron (MLP) to encode information from the expression domain and a GNN to encode information from the spatial domain. VISTA facilitates RNA velocity analysis and signaling direction inference by imputing dynamic properties of genes.
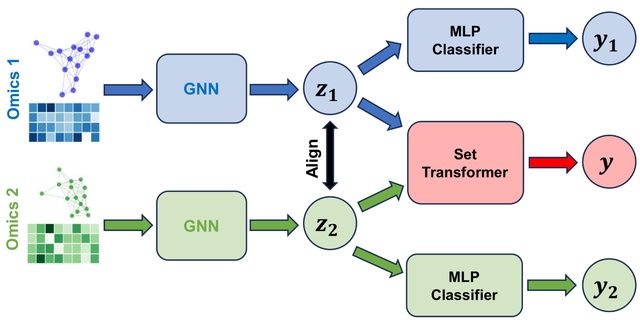
□ GNNRAI: An explainable graph neural network approach for integrating multi-omics data with prior knowledge to identify biomarkers from interacting biological domains.
>> https://www.biorxiv.org/content/10.1101/2024.08.23.609465v1
GNNRAI (GNN-derived representation alignment and integration) uses graphs to model relationships among modality features (for example, genes in transcriptomics and proteins in proteomics data). This enables us to encode prior biological knowledge as graph topology.
Integrated Hessians was applied to this transformer model to derive interaction scores between its input tokens. The biodomains partition gene functions into distinct molecular endophenotypes.

□ SCellBOW: Pseudo-grading of tumor subpopulations from single-cell transcriptomic data using Phenotype Algebra
>> https://elifesciences.org/reviewed-preprints/98469v1
SCellBOW, a Doc2vec20 inspired transfer learning framework for single-cell representation learning, clustering, visualization, and relative risk stratification of malignant cell types within a tumor. SCellBOW intuitively treats cells as documents and genes as words.
SCellBOW learned latent representations capture the semantic meanings of cells based on their gene expression levels. Due to this, cell type or condition-specific expression patterns get adequately captured in cell embeddings.
SCellBOW can replicate this feature in the single-cell phenotype space to introduce phenotype algebra. The query vector was subtracted from the reference vector to calculate the predicted risk score using a bootstrapped random survival forest.
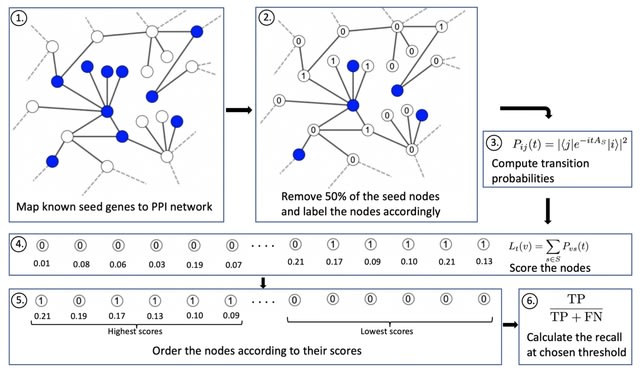
□ QDGP: Disease Gene Prioritization With Quantum Walks
>> https://academic.oup.com/bioinformatics/advance-article/doi/10.1093/bioinformatics/btae513/7738783
By encoding self-loops for the seed nodes into the underlying Hamiltonian, the quantum walker was shown to remain more local to the seed nodes, leading to improved performance.
QDGP is a novel method centered around quantum walks on the interactome. Continuous-time quantum walks are the quantum analogues of continuous-time classical random walks, which describe the propagation of a particle over a graph.
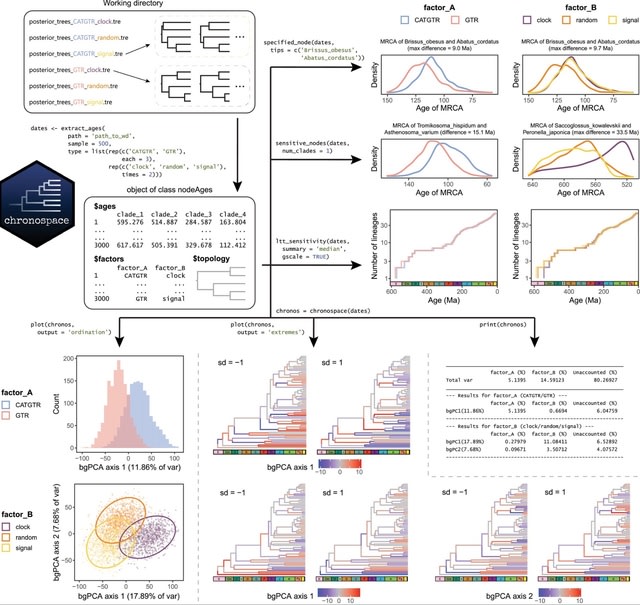
□ Chronospaces: An R package for the statistical exploration of divergence times promotes the assessment of methodological sensitivity
>> https://besjournals.onlinelibrary.wiley.com/doi/10.1111/2041-210X.14404
Chronospaces are low-dimensional graphical representations. It provides novel ways of visualizing, quantifying and exploring the sensitivity of divergence time estimates, contributing to the inference of more robust evolutionary timescales.
By representing chronograms as collections of node ages, standard multivariate statistical approaches can be readily employed on populations of Bayesian posterior timetrees.
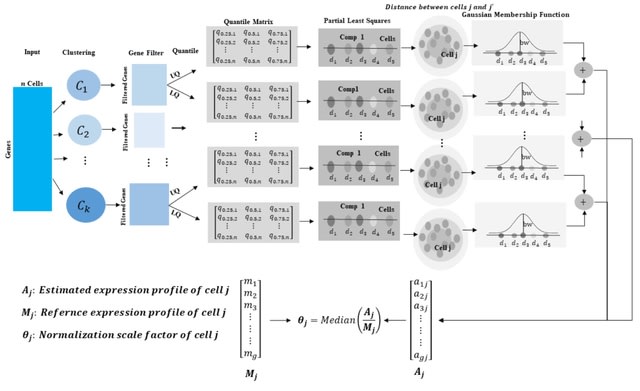
□ Normalization of Single-cell RNA-seq Data Using Partial Least Squares with Adaptive Fuzzy Weight
>> https://www.biorxiv.org/content/10.1101/2024.08.18.608507v1
The present approach overcomes biases due to library size, dropout, RNA composition, and other technical factors and is motivated by two different methods: pooling normalization, and scKWARN, which does not rely on specific count-depth relationships.
A partial least squares (PLS) regression was performed to accommodate the variability of gene expression in each condition, and upper and lower quantiles with adaptive fuzzy weights were utilized to correct unwanted biases in scRNA-seq data.
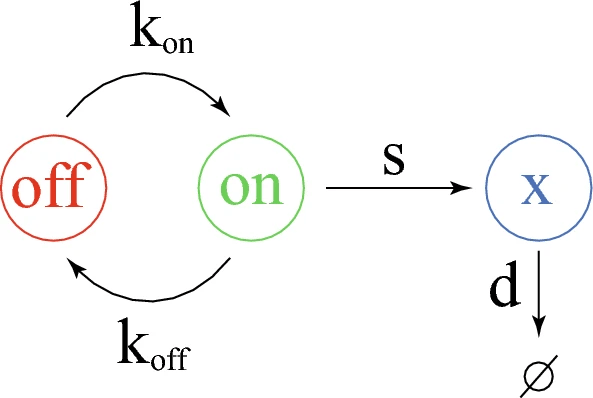
□ Modeling relaxation experiments with a mechanistic model of gene expression
>> https://bmcbioinformatics.biomedcentral.com/articles/10.1186/s12859-024-05816-4
They recently proposed a piecewise deterministic Markov process (PDMP) version of the 2-state model which rigorously approximates the original molecular model.
A moment-based method has been proposed for estimating parameter values from a experimental distribution assumed to arise from the functioning of a 2-states model. They recall the mathematical description of the model through the piecewise deterministic Markov process formalism.
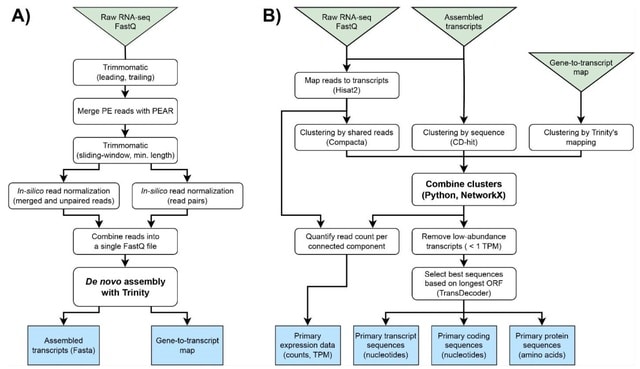
□ UnigeneFinder: An automated pipeline for gene calling from transcriptome assemblies without a reference genome
>> https://www.biorxiv.org/content/10.1101/2024.08.19.608648v1
UnigeneFinder converts the raw output of de novo transcriptome assembly software such as Trinity into a set of predicted primary transcripts, coding sequences, and proteins, similar to the gene sequence data commonly available for high-quality reference genomes.
UnigeneFinder achieves better precision while improving F-scores than the individual clustering tools it combines. It fully automates the generation of primary sequences for transcripts, coding regions, and proteins, making it suitable for diverse types of downstream analyses.
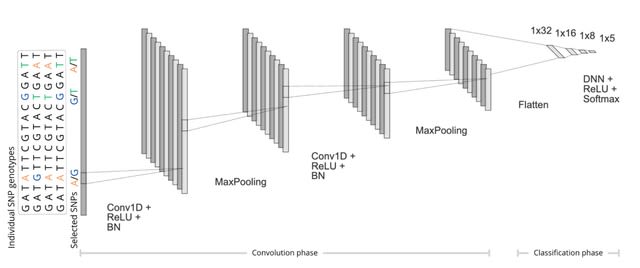
□ Approaches to dimensionality reduction for ultra-high dimensional models
>> https://www.biorxiv.org/content/10.1101/2024.08.20.608783v1
The mechanistic approach (SNP tagging) and two approaches considering biological and statistical contexts by fitting a multiclass logistic regression model followed by either 1-dimensional clustering (1D-SRA) or multi-dimensional feature clustering (MD-SRA).
MD-SRA (Multi-Dimensional Supervised Rank Aggregation) provides a very good balance between classification quality, computational intensity, and required hardware resources.
SNP selection-based 1D-SRA approach integrates both biological and statistical contexts by assessing the importance of SNPs for the classification by fitting a multiclass logistic regression model and thus adding the biological component to the feature selection process.

□ The Lomb-Scargle periodogram-based differentially expressed gene detection along pseudotime
>> https://www.biorxiv.org/content/10.1101/2024.08.20.608497v1
The Lomb-Scargle periodogram can transform time-series data with non-uniform sampling points into frequency-domain data. This approach involves transforming pseudotime domain data from scRNA-seq and trajectory inference into frequency-domain data using LS.
By transforming complex structured trajectories into the frequency domain, these trajectories can be reduced to a vector-to-vector comparison problem. This versatile method is capable of analyzing any inferred trajectory, including tree structures with multiple branching points.
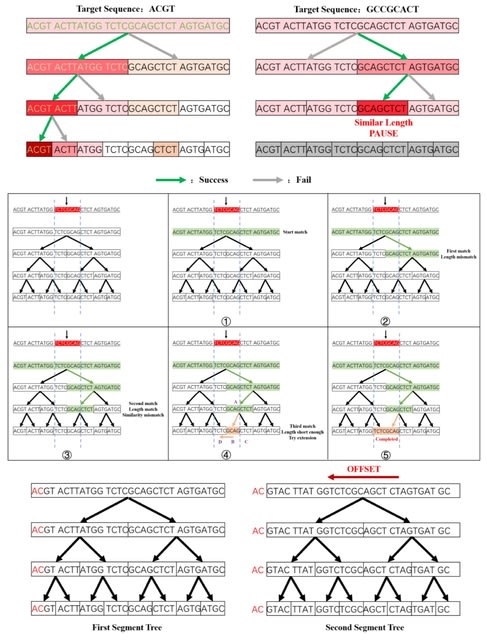
□ SMeta: a binning tool using single-cell sequences to aid reconstructing metageome species accurately
>> https://www.biorxiv.org/content/10.1101/2024.08.25.609542v1
SMeta (Segment Tree Based Metagenome Binning Algorithm) takes FASTA files of metagenomic and single-cell sequencing data as input and the binning results for each metagenomic sequence as output.
Tetranucleotide frequency is the frequency of combinations of 4 continuous base pattern in a DNA sequence. Tetranucleotides taken from sliding window on a sequence are 136-class counted and seen as a vector.
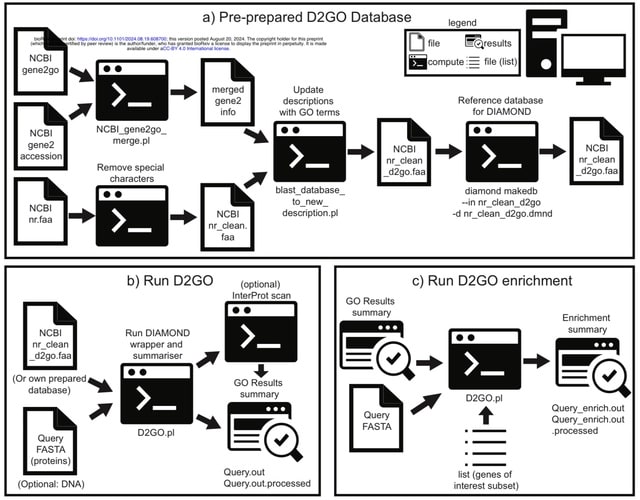
□ DIAMOND2GO: A rapid Gene Ontology assignment and enrichment tool for functional genomics
>> https://www.biorxiv.org/content/10.1101/2024.08.19.608700v1
DIAMONDGO (D2GO) is a new toolset to rapidly assign Gene Ontology (GO) terms to genes or proteins based on sequence similarity searches. D2GO uses DIAMOND for alignment, which is 100 - 20,000 X faster than BLAST.
D2GO leverages GO-terms already assigned to sequences in the NCBI non-redundant database to achieve rapid GO-term assignment on large sets of query sequences.
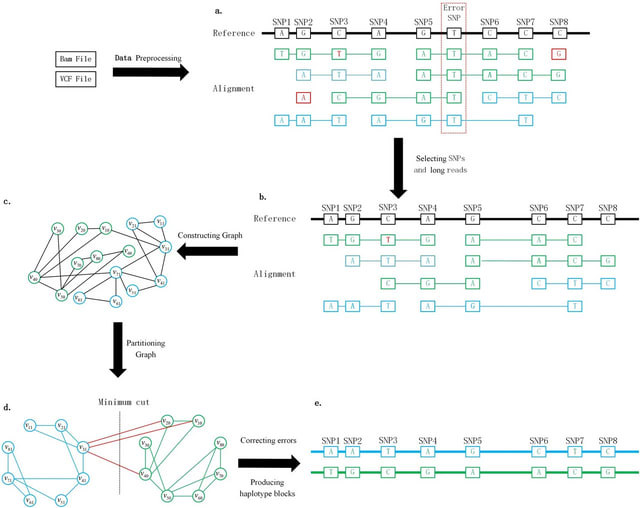
□ GCphase: an SNP phasing method using a graph partition and error correction algorithm
>> https://bmcbioinformatics.biomedcentral.com/articles/10.1186/s12859-024-05901-8
GCphase utilizes the minimum cut algorithm to perform phasing. First, based on alignment between long reads and the reference genome, GCphase filters out ambiguous SNP sites and useless read information.
GCphase constructs a graph in which a vertex represents alleles of an SNP locus and each edge represents the presence of read support; moreover, GCphase adopts a graph minimum-cut algorithm to phase the SNPs.
GCpahse uses two error correction steps to refine the phasing results obtained from the previous step, effectively reducing the error rate. Finally, GCphase obtains the phase block.
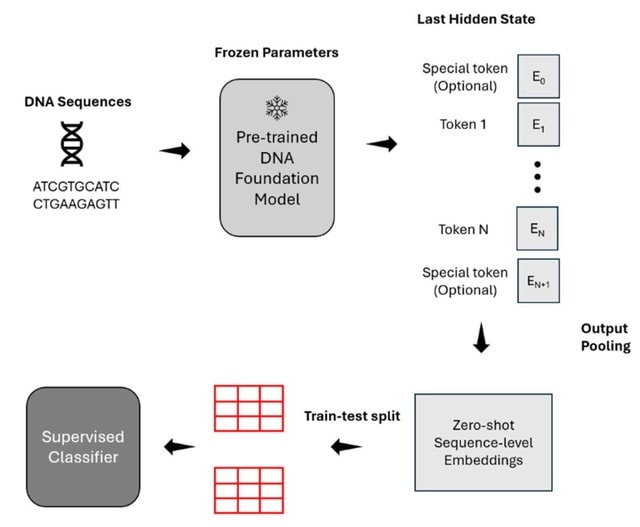
□ Benchmarking DNA Foundation Models for Genomic Sequence Classification
>> https://www.biorxiv.org/content/10.1101/2024.08.16.608288v1
A benchmarking study of three recent DNA foundation language models, including DNABERT-2, Nucleotide Transformer version-2 (NT-v2), and HyenaDNA, focusing on the quality of their zero-shot embeddings across a diverse range of genomic tasks and species.
DNABERT-2 exhibits the most consistent performance across human genome-related tasks, while NT-v2 excels in epigenetic modification detection. HyenaDNA stands out for its exceptional runtime scalability and ability to handle long input sequences.
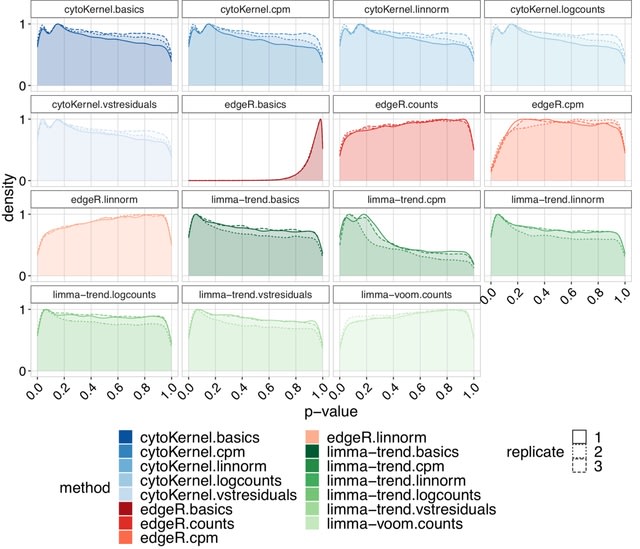
□ cytoKernel: Robust kernel embeddings for assessing differential expression of single cell data
>> https://www.biorxiv.org/content/10.1101/2024.08.16.608287v1
cytoKernel, a methodology for generating robust kernel embeddings via a Hilbert Space approach, designed to identify differential patterns between groups of distributions, especially effective in scenarios where mean changes are not evident.
CytoKernel diverges from traditional methods by conceptualizing the cell type-specific gene expression of each subject as a probability distribution, rather than as a mere aggregation of single-cell data into pseudo-bulk measures.
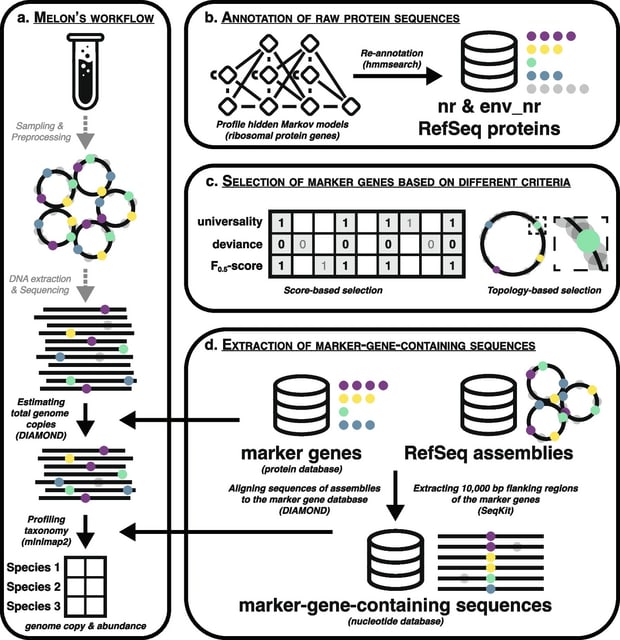
□ Melon: metagenomic long-read-based taxonomic identification and quantification using marker genes
>> https://genomebiology.biomedcentral.com/articles/10.1186/s13059-024-03363-y
Melon, a new DNA-to-marker taxonomic profiler that capitalizes on the unique attributes of long-read sequences. Melon is able to estimate total prokaryotic genome copies and provide species-level taxonomic abundance profiles in a fast and precise manner.
Melon first extracts reads that cover at least one marker gene using a protein database, and then profiles the taxonomy of these marker-containing reads using a separate, nucleotide database.
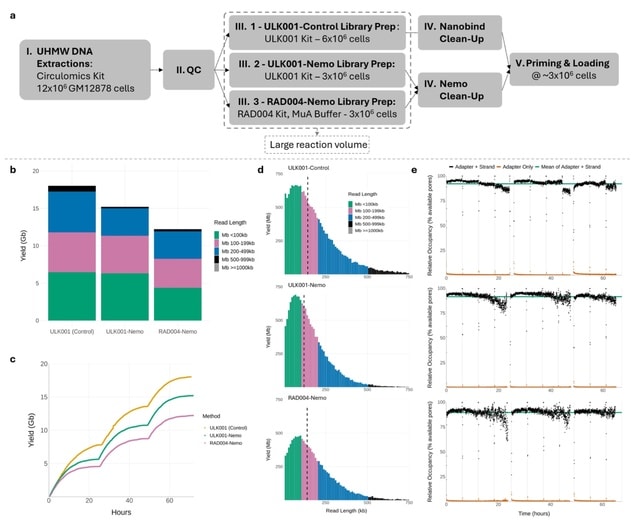
□ FindingNemo: A Toolkit for DNA Extraction, Library Preparation and Purification for Ultra Long Nanopore Sequencing
>> https://www.biorxiv.org/content/10.1101/2024.08.16.608306v1
The FindingNemo protocol for the generation of high occupancy ultra-long reads on nanopore platforms. This protocol can generate equivalent or more throughput to disc-based methods and may have additional advantages in tissues and non-human cell material.
The FindingNemo protocol can also be tuned to enable extraction from as few as one million human cell equivalents or 5 ug of human ultra-high molecular weight (UHMW) DNA as input and enables extraction to sequencing in one working day.
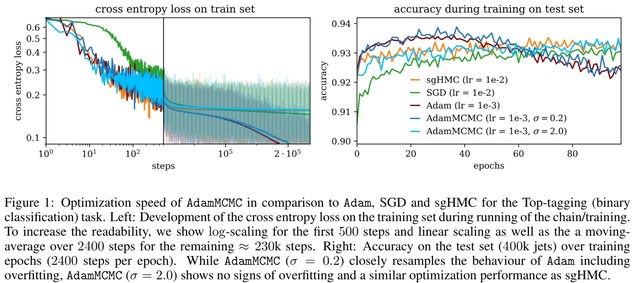
□ AdamMCMC: Combining Metropolis Adjusted Langevin with Momentum-based Optimization
>> https://arxiv.org/abs/2312.14027
AdamMCMC combines the well established Metropolis Adjusted Langevin Algorithm (MALA) with momentum-based optimization using Adam and leverages a prolate proposal distribution, to efficiently draw from the posterior.
The constructed chain admits the Gibbs posterior as an invariant distribution and converges to this Gibbs posterior in total variation distance.
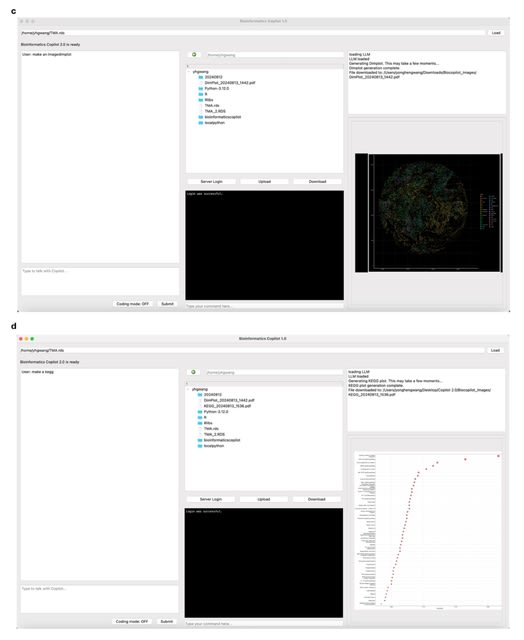
□ Bioinformatics Copilot 2.0 for Transcriptomic Data Analysis
>> https://www.biorxiv.org/content/10.1101/2024.08.15.607673v1
Bioinformatic Copilot 2.0 introduces several new functionalities and an improved user interface compared to its predecessor. A key enhancement is the integration of a module that allows access to an internal server, enabling them to log in and directly access server files.
Bioinformatic Copilot 2.0 broadens the spectrum of figure types that users can generate, including heatmaps, Kyoto Encyclopedia of Genes and Genomes (KEGG) pathway maps, and dimension plots.
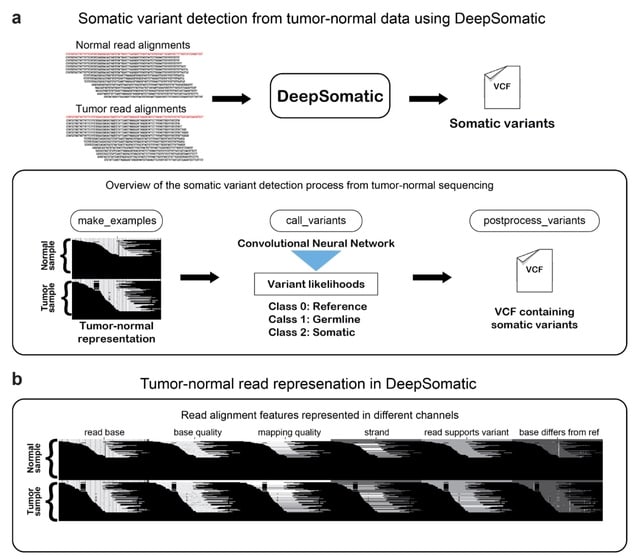
□ DeepSomatic: Accurate somatic small variant discovery for multiple sequencing technologies
>> https://www.biorxiv.org/content/10.1101/2024.08.16.608331v1
DeepSomatic, a short-read and long-read somatic small variant caller, adapted from Deep Variant. DeepSomatic is developed by heavily modifying Deep Variant, in particular, altering the pileup images to contain both tumor and normal aligned reads.
DeepSomatic takes the tensor-like representation of each candidate and evaluates it with the convolutional neural network to classify if the candidate is a reference or sequencing error, germline variant or somatic variant.
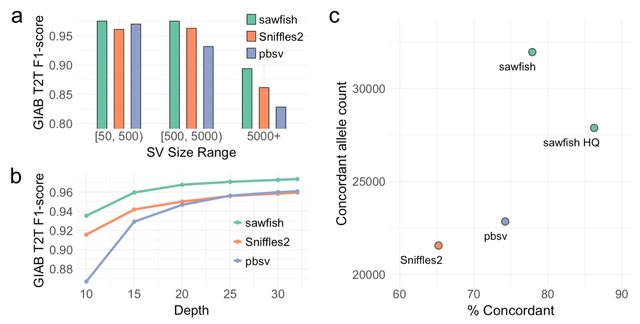
□ Sawfish: Improving long-read structural variant discovery and genotyping with local haplotype modeling
>> https://www.biorxiv.org/content/10.1101/2024.08.19.608674v1
Sawfish is capable of calling and genotyping deletions, insertions, duplications, translocations and inversions from mapped high-accuracy long reads.
The method is designed to discover breakpoint evidence from each sample, then merge and genotype variant calls across samples in a subsequent joint-genotyping step, using a process that emphasizes representation of each SV's local haplotype sequence to improve accuracy.
In a joint-genotyping context, sawfish calls many more concordant SVs than other callers, while providing a higher enrichment for concordance among all calls.
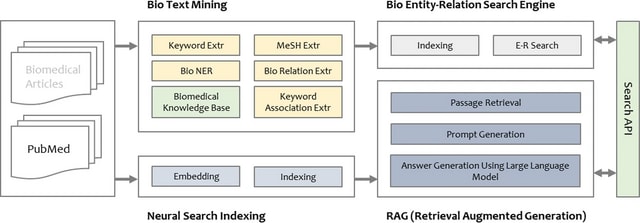
□ VAIV bio-discovery service using transformer model and retrieval augmented generation
>> https://bmcbioinformatics.biomedcentral.com/articles/10.1186/s12859-024-05903-6
VAIV Bio-Discovery, a novel biomedical neural search service which supports enhanced knowledge discovery and document search on unstructured text such as PubMed. It mainly handles w/ information related to chemical compound/drugs, gene/proteins, diseases, and their interactions.
VAIV Bio-Discovery system offers four search options: basic search, entity and interaction search, and natural language search.
VAIV Bio-Discovery employs T5slim_dec, which adapts the autoregressive generation task of the T5 (text-to-text transfer transformer) to the interaction extraction task by removing the self-attention layer in the decoder block.
VAIV assists in interpreting research findings by summarizing the retrieved search results for a given natural language query with Retrieval Augmented Generation. The search engine is built with a hybrid method that combines neural search with the probabilistic search, BM25.
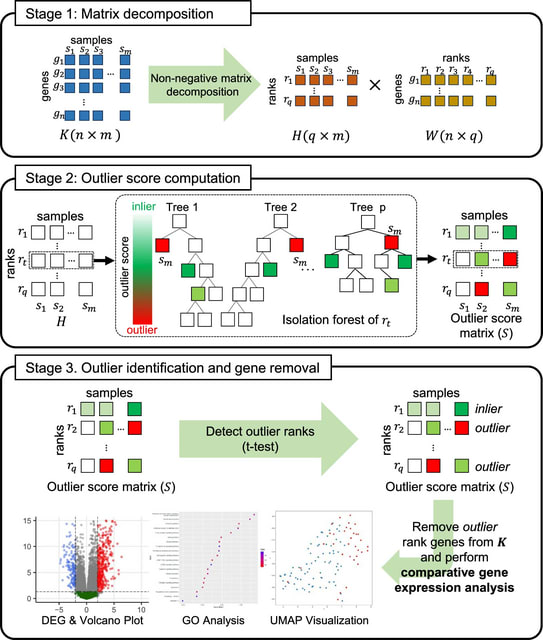
□ Denoiseit: denoising gene expression data using rank based isolation trees
>> https://bmcbioinformatics.biomedcentral.com/articles/10.1186/s12859-024-05899-z
DenoiseIt, that aims to remove potential outlier genes yielding a robust gene set with reduced noise. The gene set constructed by DenoiseIt is expected to capture biologically significant genes while pruning irrelevant ones to the greatest extent possible.
DenoiseIt processes the gene expression data and decomposes it into basis and loading matrices using NMF. In the second step, each rank feature from the decomposed result are used to generate isolation trees to compute its outlier score.

□ COATI-LDM: Latent Diffusion For Conditional Generation of Molecules
>> https://www.biorxiv.org/lookup/content/short/2024.08.22.609169v1
COATI-LDM, a novel latent diffusion models to the conditional generation of property-optimized, rug-like small molecules. Latent diffusion for molecule generation allows models trained on scarce or non-overlapping datasets to condition generations on a large data manifold.
Partial diffusion allows one to start with a given molecule and perform a partial diffusion propagation to obtain conditioned samples in chemical space. COATI-LDM relies on a large-scale pre-trained encoder-decoder that maps chemical space to fixed-length latent vector.
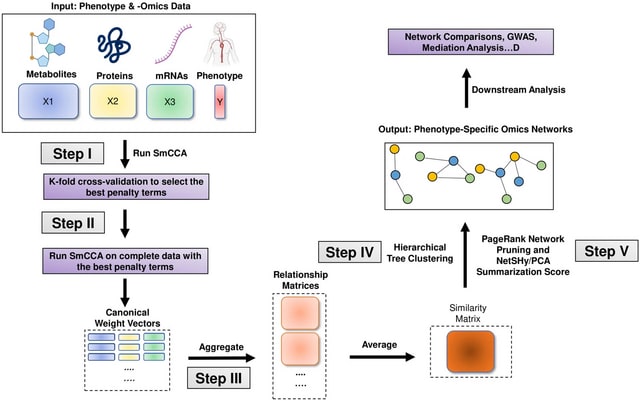
□ Smccnet 2.0: a comprehensive tool for multi-omics network inference with shiny visualization
>> https://bmcbioinformatics.biomedcentral.com/articles/10.1186/s12859-024-05900-9
SmCCNet (Sparse multiple Canonical Correlation Network Analysis) is a framework designed for integrating one or multiple types of omics data with a quantitative or binary phenotype.
It’s based on the concept of sparse multiple canonical analysis (SmCCA) and sparse partial least squared discriminant analysis (SPLSDA) and aims to find relationships between omics data and a specific phenotype.
SmCCNet uses LASSO for sparsity constraints to identify significant features w/in the data. It has two modes: weighted and unweighted. In the weighted mode, it uses different scaling factors for each data type, while in the unweighted mode, all scaling factors are equal.
※コメント投稿者のブログIDはブログ作成者のみに通知されます