
□ scSpace: Reconstruction of the cell pseudo-space from single-cell RNA sequencing data
>> https://www.nature.com/articles/s41467-023-38121-4
scSpace (single-cell spatial position associated co-embeddings), an integrative method that uses ST data as a spatial reference to reconstruct the pseudo-space. A space-informed clustering is conducted to identify spatially variable cell subpopulations within the scRNA-seq data.
scSpace uses a transfer component analysis (TCA), it enables eliminating the batch effect between single-cell and ST data and extracting the shared latent feature. TCA projects the scRNA-seq and spatial transcriptomics data into a Reproducing Kernel Hilbert Space.

□ DEGAP: Dynamic Elongation of a Genome Assembly Path
>> https://www.biorxiv.org/content/10.1101/2023.04.25.538224v1
DEGAP (Dynamic Elongation of a Genome Assembly Path), a novel gap-filling software that can resolve gap regions in genomes. DEGAP optimizes HiFi reads by identifying the differences b/n reads and provides ‘GapFiller’ or ‘CtgLinker’ modes to eliminate or shorten gaps in genomes.
DEGAP elongates all contigs with supplied HiFi data, assesses the potentially neighbored contigs. DEGAP adopts a cyclic elongation strategy that automatically and dynamically adjusts parameters according to the complexity of the sequences and selects the optimal extension path.

□ scDisInFact: disentangled learning for integration and prediction of multi-batch multi-condition single-cell RNA-sequencing data
>> https://www.biorxiv.org/content/10.1101/2023.05.01.538975v1
scDisInFact (single cell disentangled Integration preserving condition-specific Factors) learns latent factors that disentangle condition effects from batch effects, enabling it to simultaneously perform: batch effect removal, CKG detection, and perturbation prediction.
The disentangled latent space allows scDisInFact to perform the CKG detection and perturbation prediction, and to overcome the limitation of existing methods for each task. scDisInFact can remove batch effect while keeping the condition effect in gene expression data.
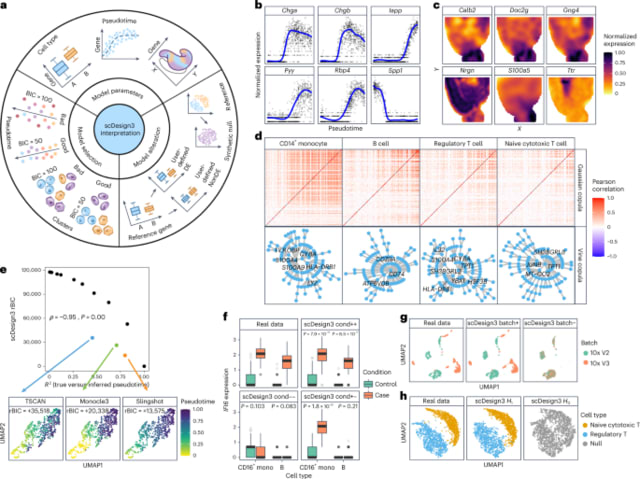
□ scDesign3 generates realistic in silico data for multimodal single-cell and spatial omics
>> https://www.nature.com/articles/s41587-023-01772-1
The scDesign3 model is flexible to incorporate cell covariates (such as cell type, pseudotime, and spatial coordinates) via the use of generalized additive models, making the scDesign3 model fit well to various single-cell and spatial omics data a property confirmed by scDesign3's realistic simulation.
scDesign3 has a model alteration functionality enabled by its transparent probabilistic modeling: given the scDesign3 model parameters estimated on real data, users can alter the model parameters to reflect a hypothesis and generate the corresponding synthetic data that bear real data characteristics.
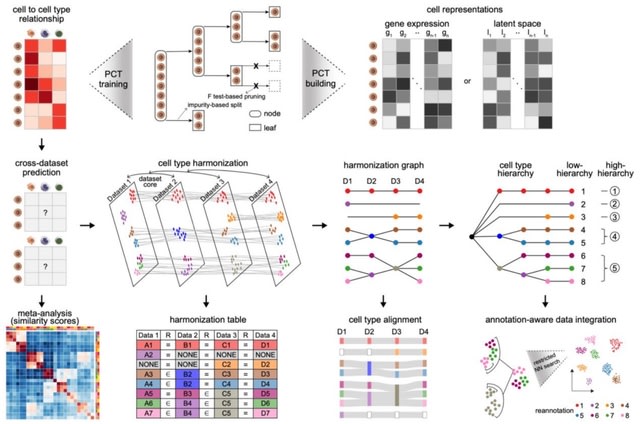
□ CellTypist v2.0: Automatic cell type harmonization and integration across Human Cell Atlas datasets
>> https://www.biorxiv.org/content/10.1101/2023.05.01.538994v1
CellTypist v2.0 accurately guantifies cell-cell transcriptomic similarities and enables robust and efficient cross-dataset meta-analyses. Cell types are placed into a relationship graph that hierarchically defines shared and novel cell subtypes.
CellTypist uses PCT, a multi-target regression tree algorithm. CellTypist defines semantic relationships among cell types / captures their underlying hierarchies, which are further leveraged to guide the downstream data integration at different levels of annotation granularities.

□ GATE: Moving Fast With Broken Data
>> https://arxiv.org/pdf/2303.06094.pdf
GATE, the Partition Summarization (PS) approach to data validation. The method creates a vector of statistics for each time step and performs a k-nearest neighbor algorithm against historical vectors to label the current time step's vector as anomalous or acceptable.
GATE significantly outperforms other methods in terms of mitigating false positives when ML pipelines have many correlated features because of GATE's clustering component, which only triggers an alert when an entire group of correlated features is anomalous.
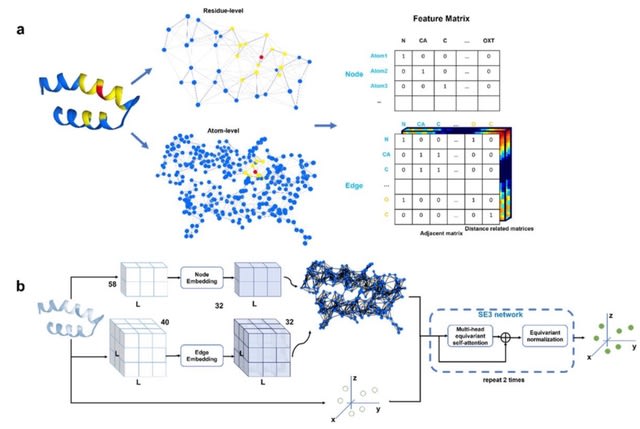
□ ATOMRefine: Atomic protein structure refinement using all-atom graph representations and SE(3)-equivariant graph transformer
>> https://academic.oup.com/bioinformatics/advance-article/doi/10.1093/bioinformatics/btad298/7152976
ATOMRefine, a deep learning-based, end-to-end, all-atom protein structural model refinement method. It uses a SE(3)-equivariant graph transformer network to directly refine protein atomic coordinates in a predicted tertiary structure represented as a molecular graph.
ATOMRefine enables the network to leverage sequence-based and spatial information from the entire protein structures to update node and edge features and catch the global and local structural variation from the initial model to the native structure iteratively.

□ Restrander: rapid orientation and QC of long-read cDNA data
>> https://www.biorxiv.org/content/10.1101/2023.05.02.539165v1
Restrander was faster than Oxford Nanopore Technologies’ existing tool Pychopper, and correctly restranded more reads due to its strategy of searching for polyA/T tails in addition to primer sequences from the reverse transcription and template-switch steps.
Each read from the reverse strand is replaced with reverse-complement, ensuring all reads in the output have the same orientation as the original transcripts. Restrander classifies artefactual reads for QC and ensure only high-quality reads are taken for downstream processing.

□ ROptimus: a parallel general-purpose adaptive optimisation engine
>> https://academic.oup.com/bioinformatics/advance-article/doi/10.1093/bioinformatics/btad292/7152277
ROptimus, a general-purpose optimisation engine in R that can be plugged to any, simple or complex, modelling initiative through a few lucid interfacing functions, to perform a seamless optimisation with rigorous parameter sampling.
ROptimus features simulated annealing and replica exchange implementations equipped with adaptive thermoregulation to drive Monte Carlo optimisation process in a flexible manner, through constrained acceptance frequency but unconstrained adaptive pseudo temperature regimens.

□ Unifilar Machines and the Adjoint Structure of Bayesian Models
>> https://arxiv.org/abs/2305.02826
There is an adjunction between ‘dynamical’ and ‘epistemic’ models of a hidden Markov process. Concepts such as Bayesian filtering and conjugate priors arise as natural consequences of this adjunction.
Strongly representable Markov categories include BorelStoch (whose objects are standard Borel spaces and whose morphisms are Markov kernels) and the Kleisli category of the (real-valued) distribution monad, which is called Dist.
Unifilar machines outputs are stochastic but whose state updates are deterministic. Its state space consists of probability distributions over the hidden states of the system, and its dynamics are given by Bayesian updating.
□ StarCoder: A State-of-the-Art LLM for Code
>> https://huggingface.co/blog/starcoder
15B LLM with 8k context
Trained on permissively-licensed code
Acts as tech assistant
80+ programming languages
Open source and data
Online demos
VSCode plugin
1 trillion tokens

□ A Bayesian Noisy Logic Model for Inference of Transcription Factor Activity from Single Cell and Bulk Transcriptomic Data
>> https://www.biorxiv.org/content/10.1101/2023.05.03.539308v1
NLBayes: A noisy Boolean logic Bayesian model for TF activity inference from differential gene expression data and causal graphs. This approach provides a flexible framework to incorporate biologically motivated TF-gene regulation logic models.
NLBayes incorporates the prior information on causal regulatory interactions and makes posterior adjustments to further account for noise and determine the context-specific posterior network structure and active regulators through a Gibbs sampling procedure.

□ Dawnn: single-cell differential abundance with neural networks
>> https://www.biorxiv.org/content/10.1101/2023.05.05.539427v1
Dawnn uses a deep neural network model that has been trained to estimate the relative abundance of cells from each sample or condition in a cell’s neighbourhood. Dawnn predicts the probability w/ which each cell was drawn from a given sample or condition using simulated datasets.
Dawn controls the false discovery rate (FDR), the proportion of cells incorrectly cssified as belonging to regions exhibiting DA, using the Benjamini-Yekutieli procedure, a variant of the Benjamini-Hochberg procedure that does not assume independence between hypotheses.
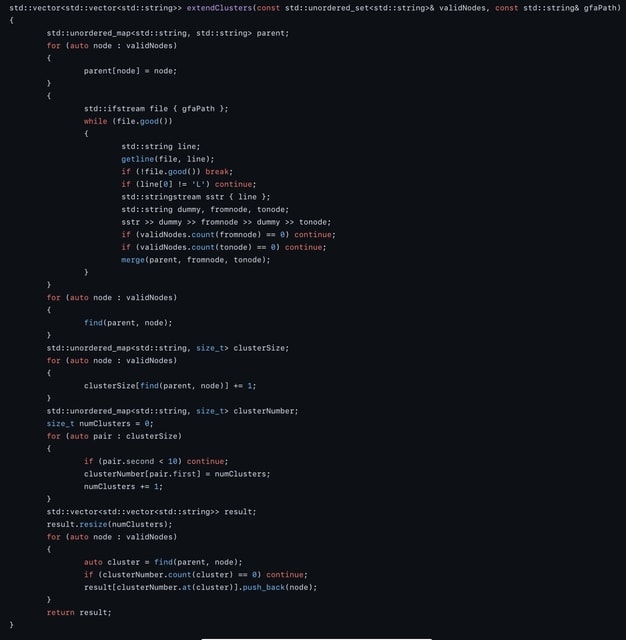
□ Ribotin: rDNA consensus sequence builder
>> https://github.com/maickrau/ribotin
Ribotin inputs hifi or duplex, and optionally ultralong ONT. Extracts rDNA-specific reads based on k-mer matches to a reference rDNA sequence or based on a verkko assembly
Ribotin builds a DBG out of them, extracts the most covered path as a consensus and bubbles as variants. Optionally assembles highly abundant rDNA morphs using the ultralong ONT reads.

□ Aggregating network inferences: towards useful networks
>> https://www.biorxiv.org/content/10.1101/2023.05.05.539529v1
They suggest to combine edge frequencies directly to reconstruct the network. This approach ensures that only robust and reproducible edges are included in the consensus network.
The first consensus step relies on selecting edges w/ high inclusion frequency in the networks reconstructed from resampled data. The 2nd aggregation step is the inference of a consensus network considering each method advantages and counter balancing each estimation's default.

□ Foldseek: Fast and accurate protein structure search
>> https://www.nature.com/articles/s41587-023-01773-0
Foldseek discretizes the query structures into sequences over the 3Di alphabet and uses a pre-trained 3Di substitution matrix to search through the 3Di sequences of the target structures using the double-diagonal k-mer-based prefilter and gapless alignment prefilter modules.
Foldseek uses vectorized Smith–Waterman local alignment combining 3Di and amino acid substitution scores. Alternatively, a global alignment is computed with a 1.7-times accelerated TM-align.

□ ProteinGenerator: Joint Generation of Protein Sequence and Structure with RoseTTAFold Sequence Space Diffusion
>> https://www.biorxiv.org/content/10.1101/2023.05.08.539766v1
Beginning from random amino acid sequences, ProteinGenerator generates sequence and structure pairs by iterative denoising, guided by any desired sequence and structural protein attributes.
ProteinGenerator readily generates sequence-structure pairs satisfying the input conditioning criteria, and experimental validation showed that the designs were monomeric by size exclusion chromatography, had the desired secondary structure content by circular dichroism.

□ Improving de novo protein binder design with deep learning
>> https://www.nature.com/articles/s41467-023-38328-5
The physically based Rosetta approach frames both the folding and binding problems in energetic terms; for the approach to succeed, the designed sequence must have as its lowest energy state in isolation the designed monomer structure.
ProteinMPNN, a novel deep learning-augmented de novo protein binder design protocol. It shows retrospectively and prospectively that this improved protocol has nearly 10-fold higher success rate than the original energy-based method.

□ HMMerge: an ensemble method for multiple sequence alignment
>> https://academic.oup.com/bioinformaticsadvances/article/3/1/vbad052/7126611
HMMerge builds on the technique from its predecessor alignment methods, UPP and WITCH, which build an ensemble of profile HMMs to represent the backbone alignment and add the remaining sequences into the backbone alignment using the ensemble.
HMMerge builds a new ‘merged’ HMM from the ensemble, and then using that merged HMM to align the query sequences. We show that HMMerge is competitive with WITCH, with an advantage over WITCH when adding very short sequences into backbone alignments.

□ Correcting gradient-based interpretations of deep neural networks for genomics
>> https://genomebiology.biomedcentral.com/articles/10.1186/s13059-023-02956-3
Even though DNNs can learn a function everywhere in Euclidean space, one-hot encoded DNA is a categorical variable that lives on a lower-dimensional simplex.
Random off-simplex function behavior can introduce a random gradient component orthogonal to the simplex, which manifest as spurious noise in the input gradients
This proposed gradient correction—subtracting the original gradient components by the mean gradients across components for each position—is general for all data with categorical inputs, including DNA, RNA, and protein sequences.

□ GKLOMLI: a link prediction model for inferring miRNA–lncRNA interactions by using Gaussian kernel-based method on network profile and linear optimization algorithm
>> https://bmcbioinformatics.biomedcentral.com/articles/10.1186/s12859-023-05309-w
GKLOMLI, a novel link prediction model based on Gaussian kernel-based method and linear optimization algorithm for inferring miRNA–lncRNA interactions. The Gaussian kernel-based method was employed to output two similarity matrixes of miRNAs and lncRNAs.
Based on the integrated matrix combined with similarity matrixes and the observed interaction network, a linear optimization-based link prediction model was trained for inferring miRNA–lncRNA interactions.

□ Estimating the mean in the space of ranked phylogenetic trees
>> https://www.biorxiv.org/content/10.1101/2023.05.08.539790v1
A simulation study to validate our method and compare it to other tree summary approaches such as the Maximum Clade Credibility (MCC) method. They assess suitability of a treespace for statistical analyses, e.g. its "smoothness" w/ respect to probability distributions over trees.
The RNNI space is a treespace of ranked phylogenetic trees, which are rooted binary trees where internal nodes are ordered according to times of the corre-ponding evolutionary events, assuming no co-occurrence.
The RNNI space is then defined as a graph where vertices are ranked trees and edges are representing either a rank or an NNI move that transforms one tree into another.
The CENTROID algorithm minimizes the sum of squared (SoS) distances b/n a summary tree and a given tree sample and stops when it finds a locally optimal tree, approximating a centroid tree. The algorithm proceeds iteratively by computing the SoS values for all neighbors.

□ Model selection and robust inference of mutational signatures using Negative Binomial non-negative matrix factorization
>> https://bmcbioinformatics.biomedcentral.com/articles/10.1186/s12859-023-05304-1
A Negative Binomial NMF with a patient specific dispersion parameter to capture the variation across patients and derive the corresponding update rules for parameter estimation.
A novel model selection procedure inspired by cross-validation to determine the number of signatures. It uses the Kullback–Leibler divergence which would favor the Poisson model. This means that a direct comparison b/n the cost values for Po-NMF / NBN-NMF is not feasible.
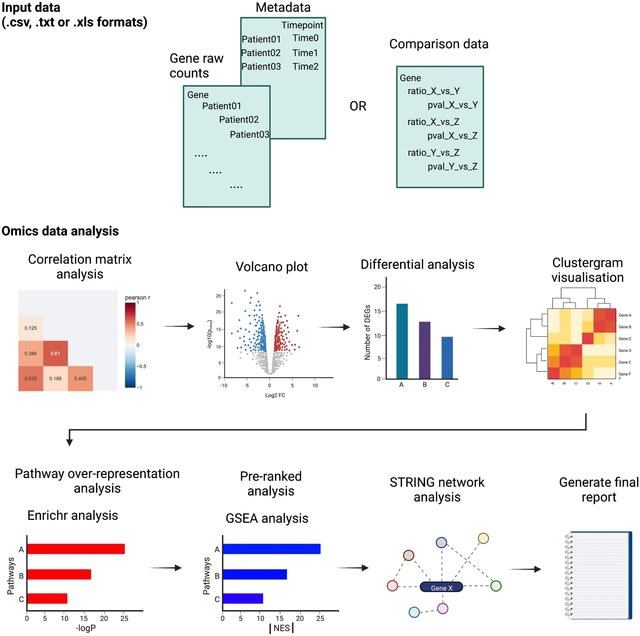
□ STAGEs: A web-based tool that integrates data visualization and pathway enrichment analysis for gene expression studies
>> https://www.nature.com/articles/s41598-023-34163-2
STAGEs (Static and Temporal Analysis of Gene Expression studies) is a web-based and high-throughput analysis pipeline with an intuitive user interface that allows systematic characterisation of static and temporal transcriptomic data.
STAGEs converts the ratio values to log2-transformed fold change values at backend, and the correlation matrix is generated by performing pairwise correlations of the log2-transformed fold changes between the different experimental conditions.
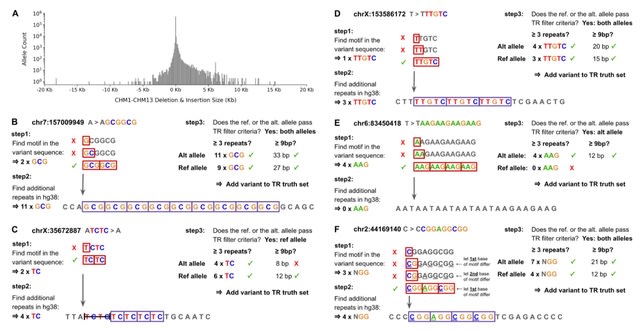
□ Insights from a genome-wide truth set of tandem repeat variation
>> https://www.biorxiv.org/content/10.1101/2023.05.05.539588v1
By identifying the subset of insertions and deletions that represent TR expansions or contractions with motifs between 2 and 50 base pairs, we obtained accurate genotypes for 139,795 pure and 6,845 interrupted repeats in a single diploid sample.
This approach did not require running existing genotyping tools on short read or long read sequencing data and provided an alternative, more accurate view of tandem repeat variation.
The Synthetic Diploid (SynDip) Benchmark provides genotypes for 5, 182,765 SNV, insertion and deletion variants, as well as a set of high-confidence regions spanning 2.71 gigabases where genotypes are highly accurate.
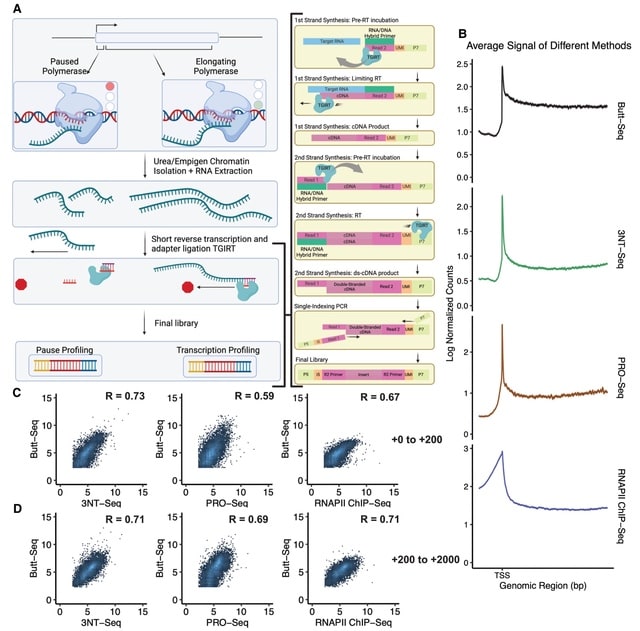
□ Butt-seq: a new method for facile profiling of transcription
>> https://genesdev.cshlp.org/content/early/2023/05/10/gad.350434.123.abstract
Butt-seq (bulk analysis of nascent transcript termini sequencing), which can produce libraries from purified nascent RNA in 6 h and from as few as 10,000 cells—an improvement of at least 10-fold over existing techniques.
Butt-seq shows that inhibition of the superelongation complex (SEC) causes promoter-proximal pausing to move upstream in a fashion correlated with subnucleosomal fragments.

□ NGBO: Introducing -omics metadata to biobanking ontology
>> https://www.biorxiv.org/content/10.1101/2023.05.09.539725v1
NGBO is based on available genomics standards (e.g., Minimum information about a microarray experiment (MIAME)), the College of American Pathologists (CAP) laboratory accreditation requirements, and the Open Biological and Biomedical Ontologies Foundry principles.
NGBO fills the need for semantically enabling the discovery and integration of omics datasets and realization of FAIR data representation, which will impact the efficiency of finding, integrating, and re-using biobanking data of interest.

□ Robust discovery of causal gene networks via measurement error estimation and correction
>> https://www.biorxiv.org/content/10.1101/2023.05.09.540002v1
A new framework for causal discovery that is robust against measurement noise by extending an established statistical approach CIT (Causal Inference Test).
RCD (Robust Causal Discovery) estimates measurement error from gene expression data and then incorporate it to get consistent parameter estimates that could be used with appropriately extended statistical tests of correlation or mediation done in the original CIT.

□ Simple Tidy GeneCoEx: A gene co-expression analysis workflow powered by tidyverse and graph-based clustering in R
>> https://acsess.onlinelibrary.wiley.com/doi/10.1002/tpg2.20323
Simple Tidy GeneCoEx detects co-expression modules enriched in specific cell types, which were used to discover candidate genes in a biosynthetic pathway for complex plant natural products.
Simple Tidy GeneCoEx detects modules that are, on average, equivalently tight or tighter than those detected by WGCNA. A potential reason underlying the differences in module tightness might be due to the module detection methods.
By default, WGCNA uses hierarchical clustering followed by tree cutting to detect modules. Simple Tidy GeneCoEx uses the Leiden algorithm to detect modules, which returns modules that are highly interconnected.

□ Fulgor: A fast and compact k-mer index for large-scale matching and color queries
>> https://www.biorxiv.org/content/10.1101/2023.05.09.539895v1
Fulgor is a colored compacted de Bruijn graph index for large-scale matching and color queries, powered by SSHash. Fulgor has a generic intersection algorithm that can work over any compressed color sets, provided that an iterator over each color supports two primitives - Next and NextGEQ(x).
Themisto, an index for alignment-free matching that substantially outperforms these prior methods in the context of indexing and mapping against large collections of genomes. Compared to Bifrost, Themisto uses practically the same space, but is faster to build and query.
Compared to the fastest variant of Metagraph, Themisto offers similar query performance, but is much more space-efficient; on the other hand, Themisto is much faster to query than Metagraph-BRWT, the most-space efficient variant of Metagraph.

□ RaPID-Query for Fast Identity by Descent Search and Genealogical Analysis
>> https://academic.oup.com/bioinformatics/advance-article/doi/10.1093/bioinformatics/btad312/7160137
A new method, random projection-based identical-by-descent (IBD) detection (RaPID) query, is introduced to make fast genealogical search possible. RaPID-Query identifies IBD segments between a query haplotype and a panel of haplotypes.
By integrating matches over multiple PBWT indexes, RaPID- Query manages to locate IBD segments quickly with a given cutoff length while allowing mismatched sites.

□ CARMA is a new Bayesian model for fine-mapping in genome-wide association meta-analyses
>> https://www.nature.com/articles/s41588-023-01392-0
CARMA, a Bayesian model for fine-mapping that includes flexible specification of the prior distribution of effect sizes, joint modeling of summary statistics and functional annotations and accounting for discrepancies b/n summary statistics and external linkage disequilibrium in meta-analyses.
CARMA has higher power and lower false discovery rate (FDR) when including functional annotations, and higher power, lower FDR and higher coverage for credible sets in meta-analyses.

□ DeCOIL: Optimization of Degenerate Codon Libraries for Machine Learning-Assisted Protein Engineering
>> https://www.biorxiv.org/content/10.1101/2023.05.11.540424v1
DEgenerate Codon Optimization for Informed Libraries (DeCOIL), a generalized method which directly optimizes DC libraries to be useful for protein engineering: to sample protein variants that are likely to have both high fitness and high diversity in the sequence search space.
DeCOIL can be used to generate a designed library for screening based on computational predictors (ZS scores or ML models) at many possible points along the route to engineering a protein. DeCOIL enables protein engineering using ftMLDE with comparable outcomes.

□ moscot: Mapping cells through time and space
>> https://www.biorxiv.org/content/10.1101/2023.05.11.540374v1
moscot supports multimodal data throughout the framework by exploiting joint cellular representations. moscot improves scalability by adapting and demonstrating the applicability of recent methodological innovations to atlas-scale datasets.
moscot unifies previous single-cell applications of OT in the temporal and spatial domain and introduces a novel spatiotemporal application. All of this is achieved with a robust and intuitive API that interacts with the broader scverse ecosystem.
※コメント投稿者のブログIDはブログ作成者のみに通知されます