
(Art by Megs)


□ ConDecon: Clustering-independent estimation of cell abundances in bulk tissues using single-cell RNA-seq data
>> https://www.biorxiv.org/content/10.1101/2023.02.06.527318v2
ConDecon uses the gene expression count matrix and latent space of the reference single-cell RNA-seq dataset. ConDecon accurately estimates cell abundances in bulk tissues composed of discrete cell types and continuous cellular processes.
ConDecon computes the rank correlation between the gene expression profiles of the bulk RNA-seq dataset and each cell in the single-cell dataset using the most variable genes.
The resulting correlations are represented by a point in the space of possible correlation distributions. ConDecon then maps that point into a point in the space of possible cell abundance distributions with support on the single-cell RNA-seq latent space.

□ MIRACLE: Continual integration of single-cell multimodal data
>> https://www.biorxiv.org/content/10.1101/2024.09.24.613833v1
MIRACLE is a deep generative model combined with continual learning to incrementally integrate single-cell multimodal mosaic datasets of assays for transposase-accessible chromatin (ATAC), RNA and antibody-derived tags.
MIRACLE leverages the strategies including dynamic architecture and data rehearsal to adapt to new information while preventing forgetting. It employs MIDAS as the base model to handle multimodal mosaic data, allowing online integration of trimodal mosaic data.
MIRACLE can precisely map query data onto a reference atlas via an innovative use of online integration, thereby enhancing label transfer. MIRACLE continually integrates new cross-tissue and cross-modal data, effectively enabling online updates and expansions of the atlas.

□ CLERA: Discovering Governing Equations of Biological Systems through Representation Learning and Sparse Model Discovery
>> https://www.biorxiv.org/content/10.1101/2024.09.19.613953v1
CLERA (Cellular Latent Equation and Representation Analysis), a novel end-to-end computational framework that combines the power of data-driven model discovery, specifically Sparse Identification of Nonlinear Dynamics (SINDy), and representation learning.
CLERA extracts a compact and relevant representation from high-dimensional data and uses it to discover the underlying low-dimensional, non-linear dynamical model governing the system. This learned embedding further allows us track their transitions over time across cell types.

□ Fragment Topological Order DAGs: Accurate Multiple Sequence Alignment of Ultramassive Genome Sets
>> https://www.biorxiv.org/content/10.1101/2024.09.22.613454v1
Fragment topological order is of special importance due to its explicit utility; while implicit in all present algorithms, it plays a pivotal role in their construction of directed acyclic graphs. Correspondingly, graphs constructed in this work are termed FTO-DAGs.
FTO-DAGs provide an alternative to current pan-genome graphs with cycles. In the parallelization scheme of pHMM training on FTO-DAGs, linear path segments are stored as separate computing tasks by graph traversal, and dependencies among tasks are controlled by topological order.

scAdam

scEve

scNoah
□ scParadise: Tunable highly accurate multi-task cell type annotation and surface protein abundance prediction
>> https://www.biorxiv.org/content/10.1101/2024.09.23.614509v1
scParadise includes three sets of tools: scAdam - fast multi-task multi-class cell type annotation with Sparse Attention Mechanism - Attentive Transformer + Feature Transformer; scEve - modality prediction. scNoah - unifying cell type annotation and modality prediction.
scParadise enables users to utilize a selection (scAdam or scEve) as well as to develop and train custom models tailored to specific research needs. scNoah allowed visualization of prediction performance using a confusion matrix.

□ Complex genetic variation in nearly complete human genomes
>> https://www.biorxiv.org/content/10.1101/2024.09.24.614721v1
Sequence 65 diverse human genomes and build 130 haplotype-resolved assemblies (130 Mbp median continuity), closing 92% of all previous assembly gaps and reaching telomere-to-telomere (T2T) status for 39% of the chromosomes.
They highlight complete sequence continuity of complex loci, including the major histocompatibility complex (MHC), SMN1|SMN2, NBPF8, and AMY1/AMY2, and fully resolve 1,852 complex structural variants (SVs).
Completely assemble and validate 1,246 human centromeres. We find up to 30-fold variation in a-satellite high-order repeat (HOR) array length and characterize the pattern of mobile element insertions into a-satellite HOR arrays.
Generated haplotype-resolved assemblies from all 65 diploid samples using Verkko. The phasing signal for the assembly process was produced with Graphasing , leveraging Strand-seq to globally phase assembly graphs at a quality on par with trio-based workflows.

□ scPCA: Joint Modeling of Cellular Heterogeneity and Condition Effects with scPCA in Single-Cell RNA-Seq
>> https://www.biorxiv.org/content/10.1101/2024.09.22.614322v1
scPCA, a flexible factorization model for analyzing multi-condition single-cell datasets. This model incorporates conditioning variables, enabling scPCA to extract condition-specific bases.
scPCA factors explain a greater proportion of the variance compared to conventional factor models at equivalent decomposition ranks and eliminate the necessity for factors that explicitly address variance attributed to the conditioning variable.
A scPCA decomposition enables the analyst to assess the change of individual sPCs across conditions, thereby providing insights into how the components vary under different conditions.

□ dScaff: an automatic bioinformatics framework for scaffolding draft de novo assemblies based on reference genome data
>> https://www.biorxiv.org/content/10.1101/2024.09.23.614313v1
dScaff (the Digital Scaffolding) procedure, an original framework for simple and straightforward minimal scaffolding that can even accommodate basic gene annotations of draft de novo genomic assemblies.
dScaff uses BLAST in order to align the draft assembly of interest against the sequences of annotated genes gathered from the corresponding reference genome.
dScaff is circumventing the inherent fragmentation of the result in various sub-alignments when there are nucleotide differences between a long query and a comparable or longer subject sequence.
Upon running dScaff, the number of the reference chromosomes/scaffolds of the envisioned species will be identified based on the indexed gene table and specific output folders will be created for each chromosome. It eliminates table entries that are not associated w/ chromosomes.
The automatic contigs mapping making a draft variant of a minimal continuous scaffold. The relatively large spaces between adjacent contigs are due to some specific filtration of positive cells based on the alignment length.

□ RNA-DCGen: Dual Constrained RNA Sequence Generation with LLM-Attack
>> https://www.biorxiv.org/content/10.1101/2024.09.23.614570v1
RNA-DCGen, a generalized framework for RNA sequence generation that is adaptable to any structural or functional properties through straightforward finetuning with an RNA language model (RNA-LM).
RNA-DCGen can enforce conditions on the generated sequences by fixing specific conserved regions. The finetuned LLM generates predictions for the input sequence, and we compute the loss between the predicted properties and the desired properties.
This loss is backpropagated through the LLM to generate a gradient distribution at each position for all possible words in the vocabulary. Next, It utilizes a modified Gradient Coordinate Search to calculate a gradient-guided search to modify certain portions of the sequence.
Finally, to validate the quality of the generated sequence, RNA-DCGen uses another finetuned RNA language model (RiNALMo) as an independent discriminator and compare the desired property quality between the ground properties and those of the generated sequences.

□ scEMB: Learning context representation of genes based on large-scale single-cell transcriptomics
>> https://www.biorxiv.org/content/10.1101/2024.09.24.614685v1
ScEMB, an attention-based deep learning model pretrained on large-scale transcriptomic data. It uses self-attention to focus on the most critical genes expressed in each single cell, optimizing predictive accuracy through various learning objectives.
ScEMB captures complex biologically meaning alteration underlying cell type state transition and perturbation response. SCEMB represents each single cell's transcriptome as a rank-binned gene expression encoding, ranking genes by their expression within individual cells.

□ INSPIRE: interpretable, flexible and spatially-aware integration of multiple spatial transcriptomics datasets from diverse sources
>> https://www.biorxiv.org/content/10.1101/2024.09.23.614539v1
INSPIRE incorporates a tailored adversarial learning mechanism to adaptively distinguish complex unwanted variations across multiple batches, samples, platforms and developmental stages from intrinsic biological variations, when certain datasets present unique biological signals.
INSPIRE eliminates unwanted variations in latent space, providing harmonized representations of cells among slices. The latent space enables INSPIRE to achieve an integrated NMF for multiple slices, further decomposing biological signals into a set of interpretable factors.



□ PATH: Defining heritability, plasticity, and transition dynamics of cellular phenotypes in somatic evolution
>> https://www.nature.com/articles/s41588-024-01920-6
PATH (phylogenetic analysis of trait heritability), a framework to quantify cell state heritability versus plasticity and infer cell state transition and proliferation dynamics from single-cell lineage tracing data.
The PATH framework further allows for the inference of cell state transition dynamics by linking a model of cellular evolutionary dynamics with the measure of heritability versus plasticity.
PATH builds upon auto-correlative methods classically used to measure phylogenetic signal, the phylogenetic clustering of species phenotypes. PATH provides a measure of how distinct phenotypes co-cluster on phylogenies, and thus defining a pairwise measure of phylogenetic signal.

□ scMultiMap: Cell-type-specific mapping of enhancers and target genes from single-cell multimodal data
>> https://www.biorxiv.org/content/10.1101/2024.09.24.614814v1
scMultiMap uses single-cell multimodal data to map cell-type-specific enhancer-gene pairs. scMultiMap is based on a multivariate latent-variable model that simultaneously models the gene counts and peak counts from multimodal data, and makes minimal parametric assumptions.
scMultiMap measures peak-gene association via the correlation between underlying gene expression and peak accessibility levels while accounting for variations in sequencing depths and across biological samples.

□ DeepRVAT: Integration of variant annotations using deep set networks boosts rare variant association testing
>> https://www.nature.com/articles/s41588-024-01919-z
DeepRVAT is an end-to-end genotype-to-phenotype model that first accounts for nonlinear effects from rare variants on gene function (gene impairment module) to then model variation in one or multiple traits as linear functions of the estimated gene impairment scores.
The gene impairment module estimates a gene and trait-agnostic gene impairment scoring function that accounts for the combined effect of rare variants, thereby allowing the model to generalize to new traits and genes.
Technically, a deep set neural network architecture is used to aggregate the effects from multiple discrete and continuous annotations for an arbitrary number of rare variants.

□ LoVis4u: Locus Visualisation tool for comparative genomics
>> https://www.biorxiv.org/content/10.1101/2024.09.11.612399v1
LoVis4u (Locus Visualisation), a scalable software tool designed for customisable and fast visualisation of multiple genomic loci. LoVis4u offers a command-line interface without requiring user-side scripting and provides a Python API for additional customization and integration.
LoVis4u constructs a matrix with pairwise proteome composition similarity scores that reflect the fraction of shared homologous proteins between sequences, and a corresponding proteome composition distance matrix.

□ TSTA: Thread and SIMD-Based Trapezoidal Pairwise/Multiple Sequence Alignment Method
>> https://www.biorxiv.org/content/10.1101/2024.09.18.613655v1
The TSTA algorithm leverages both vector-level and thread-level parallelism to accelerate pairwise and multiple sequence alignments. The algorithm integrates four methods: the difference method, the stripe method, the SIMD instruction set, and multi-threading.
The TSTA algorithm divides the entire scoring matrix into multiple blocks of equal length and width along the anti-diagonal. These blocks are then computed in parallel threads to substantially minimize the overhead associated with invoking threads.

□ DiffPaSS: High-performance differentiable pairing of protein sequences using soft scores
>> https://www.biorxiv.org/content/10.1101/2024.09.20.613748v1
DiffPaSS ("Differentiable Pairing using Soft Scores"), a family of flexible, fast and hyperparameter-free algorithms for pairing interacting sequences among the paralogs of two protein families.
DiffPaSS optimizes smooth extensions of coevolution or similarity scores to "soft" permutations of the input sequences, using gradient methods. It can be used to optimize any score, including coevolution scores and sequence similarity scores.

□ TwinC: Prediction and functional interpretation of inter-chromosomal genome architecture from DNA sequence
>> https://www.biorxiv.org/content/10.1101/2024.09.16.613355v1
TwinC is a convolutional neural network that predicts trans contacts b/n 2 genomic loci. The input to the model is two one-hot-encoded, 100 kbp nucleotide sequences. Both input sequences pass through the same encoder, whose architecture is derived from the Akita and Orca models.
TwinC moves away from the regression setup of predicting the frequency of Hi-C contacts to a classification setup where robust positive and negative labels are extracted from multiple replicate experiments. TwinC combines Gradients and TF motifs score from JASPAR and ChromBPNet.

□ MELISSA: Modeling integration site data for safety assessment
>> https://biorxiv.org/cgi/content/short/2024.09.16.613352v1
MELISSA (ModELing IS for Safety Analysis) translates IS data into actionable safety assessment and evaluation insights. MELISSA provides statistical models for measuring and comparing gene targeting rates and their effects on clone growth within a gene-based approach.
MELISSA modeling consists of a regression approach that analyzes and combines data from complex experimental designs, including datasets with multiple patients or donors, replicates, and additional covariates of interest.
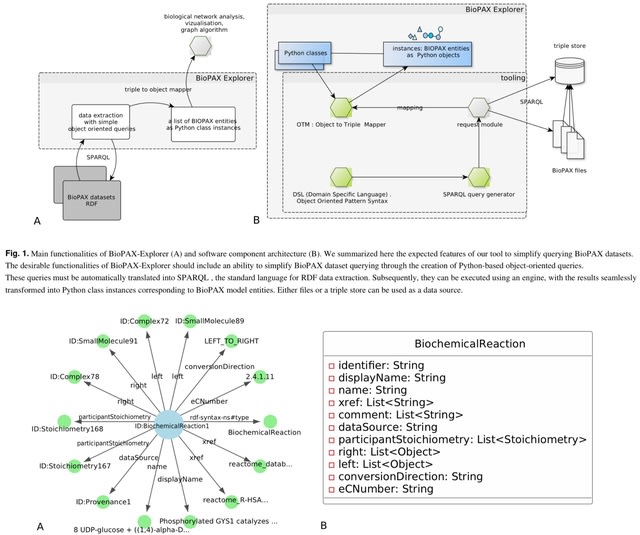
□ BioPAX-Explorer: a Python Object-Oriented framework for overcoming the complexity of querying biological networks
>> https://www.biorxiv.org/content/10.1101/2024.09.18.613626v1
BioPAX-Explorer is a Python package that provides an object-oriented data model automatically generated from the BioPAX OWL specification.
BioPAX-Explorer uses a simple object-oriented, domain specific syntax. It includes an Object Triple Mapping mechanism with a simple object-oriented query pattern syntax.

□ Motif distribution in genomes gives insights into gene clustering and co-regulation
>> https://www.biorxiv.org/content/10.1101/2024.09.18.613605v1
This method provides a practical means for assessing sequence patterns without relying on extensive alignments, particularly suited for analysing large genomic regions. In this study, they used a modification of the k-mer method to compare genome segments.
They employed the observed-to-expected ratio (OE ratio). It is a measure of whether a feature is over or underrepresented in a given dataset. It is calculated by normalising the observed frequency of the feature by the expected frequency based on the probability of occurrence.

□ The Unified Phenotype Ontology (uPheno): A framework for cross-species integrative phenomics
>> https://www.biorxiv.org/content/10.1101/2024.09.18.613276v1
uPheno has three main components: the uPheno ontology; a library of design patterns and templates for computationally tractable phenotype definitions; and a number of standardized mappings to connect disparate phenotype ontologies.
The uPheno ontology integrates 12 species-specific phenotype ontologies, which are used by a wide range of databases from the domain of model organisms, including all databases participating in the Alliance of Genome Resources.
uPheno ontology includes logical connections to all species-specific ontologies, standardized mapping tables are provided with direct links between species-specific and species-neutral ontologies.

□ GuaCAMOLE: GC-bias aware estimation improves the accuracy of metagenomic species abundances
>> https://www.biorxiv.org/content/10.1101/2024.09.20.614100v1
The GuaCAMOLE algorithm processes the raw sequencing reads of a metagenomic sample and outputs bias-correct abundances for all detected taxa. GuaCAMOLE produced virtually unbiased estimates and correctly recovered the GC-dependent sequencing efficiencies used for the simulation.
GuaCAMOLE also infers and outputs GC-dependent sequencing efficiencies which reflect the probability (relative to the maximum) that a DNA fragment with a certain GC content successfully undergoes all library preparation steps and sequencing.
GuaCAMOLE reports the estimated abundances either as sequence abundances proportional to the total amount of DNA present, or taxonomic abundance proportional to the number of genomes.

□ scooby: Modeling multi-modal genomic profiles from DNA sequence at single-cell resolution
>> https://www.biorxiv.org/content/10.1101/2024.09.19.613754v2
scooby, a model predicting single-cell accessibility and expression profiles from DNA sequence. Following the LoRA approach, scooby kept pre-trained weights frozen and added trainable low-rank matrices into the transformer and convolutional layers.
scooby leverages low dimensional, multiomic representations of cell states, derived from Poisson-MultiV|, to decode the fine-tuned sequence embedding generated by Borzoi. scooby accurately predicts cell-type-specific gene expression counts and generalizes to unseen cell states.


□ BioMANIA: Simplifying bioinformatics data analysis through conversation
>> https://www.biorxiv.org/content/10.1101/2023.10.29.564479v2
BioMANIA, an artificial intelligence (AI)-driven, natural language-oriented bioinformatics data analysis pipeline. BioMANIA is designed to directly understand natural language instructions from users and efficiently execute complex biological data analysis tasks.
Considering the limitations of LLMs in lack of domain-specific biological knowledge, BioMANIA explicitly learns about API information and their interactions from the source code (e.g., GitHub) and documentation of any off-the-shelf well-documented, open-source Python tools.

□ AsaruSim: a single-cell and spatial RNA-Seq Nanopore long-reads simulation workflow
>> https://www.biorxiv.org/content/10.1101/2024.09.20.613625v1
AsaruSim, a workflow that simulates single-cell long-read Nanopore data. This workflow aims to generate a gold standard dataset for the objective assessment and optimization of single-cell long-read methods.
AsaruSim takes as input a feature-by-cell (gene/cell or isoform/cell) UMI count matrix. AsaruSim generates more realistic synthetic reads from the previous read templates (perfect or post-PCR) using Badread simulator with a pre-trained error model on real Nanopore reads.

□ STANCE: a unified statistical model to detect cell-type-specific spatially variable genes in spatial transcriptomics
>> https://www.biorxiv.org/content/10.1101/2024.09.22.614385v1
STANCE (Spatial Transcriptomics ANalysis of genes with Cell-type-specific Expression), a unified statistical method that can identify both SVG and ctSVG. By integrating gene expression, spatial location, and cell type composition through a linear mixed-effect model.
STANCE uses spatial kernel matrices that rely solely on the relative distance between spatial spots and guarantees that the testing results are invariant to spatial rotation and transformation.

□ Long-read whole-genome sequencing-based concurrent haplotyping and aneuploidy profiling of single cells
>> https://www.biorxiv.org/content/10.1101/2024.09.24.614469v1
The first comprehensive analysis of IrWGS data from human single cells at an adequate depth of ~24x for SNV and indel calling, as well as haplotyping.
Using a Genome in a Bottle trio consisting of HG002 (offspring), HG003 (father), and HG004 (mother) for benchmarking, it demonstrates the feasibility of IrWGS data for concurrent haplotyping and aneuploidy profiling of single cells without requiring additional phasing references.

□ SeaMoon: Prediction of molecular motions based on language models
>> https://www.biorxiv.org/content/10.1101/2024.09.23.614585v1
SeaMoon (SEAquencetoMOtioON), a 1D convolutional neural network inputting a protein sequence pLM embedding and outputting a set of 3D displacement vectors.
SeaMoon identifies the transformation (rotation and scaling) minimising their discrepancy, computed as a sum-of-squares error (SSE).

□ LOCAS: Multi-label mRNA Localization with Supervised Contrastive Learning
>> https://www.biorxiv.org/content/10.1101/2024.09.24.614785v1
LOCAS (Localization with Supervised Contrastive Learning), which integrates an RNA language model to generate initial embeddings, employs supervised contrastive learning (SCL) to identify distinct RNA clusters.
LOCAS uses a multi-label classification head (ML-Decoder) with cross-attention for accurate predictions. LOCAS effectively captures complex relationships in RNA sequences, addressing challenges in multi-label classification and natural label overlap.

□ Strainy: phasing and assembly of strain haplotypes from long-read metagenome sequencing
>> https://www.nature.com/articles/s41592-024-02424-1
Strainy - an algorithm for strain-level metagenome assembly and phasing from Nanopore and HiFi reads. Strainy takes a de novo metagenomic assembly as input, identifies strain variants which are then phased and assembled into contiguous haplotypes.
Using simulated and mock Nanopore and HiFi metagenome data, we show that Strainy assembles accurate and complete strain haplotypes, outperforming current Nanopore-based methods and comparable with HiFi-based algorithms in completeness and accuracy.
Strainy aligns reads against the MAG contigs, identifies regions with collapsed strains and phases them into strain-resolved haplotigs. Haplotigs and strain-specific read connections are then used to update and simplify the original de novo assembly graph.