

(Art by Neptali Cisneros)
□ Klur / “Stellation”

□ Tokenized and Continuous Embedding Compressions of Protein Sequence and Structure
>> https://www.biorxiv.org/content/10.1101/2024.08.06.606920v1
CHEAP (Compressed Hourglass Embedding Adaptations of Proteins) is a compact representation of both protein structure and sequence, sheds light on information content asymmetries between sequence and structure, democratizes representations captured by large models.
HPCT (The Hourglass Protein Compression Transformer), an autoencoder with a bottleneck layer for protein embedding compression. HPCT includes a linear downsampling operation using a shortening factor. A linear projection further compresses the channel dimension.
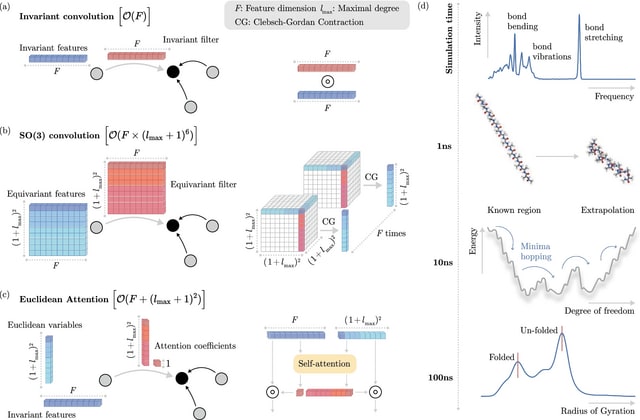
□ SO3KRATES: A Euclidean transformer for fast and stable machine learned force fields
>> https://www.nature.com/articles/s41467-024-50620-6
SO3KRATES, a transformer architecture that combines sparse equivariant representations (Euclidean variables) with a self-attention mechanism that separates invariant and equivariant information, eliminating the need for expensive tensor products.
SO3KRATES enables the analysis of quantum properties of matter on extended time/system size scales. Their orthonormality makes projections correspond to the trace of the product tensor, which can be expressed in terms of a linear-scaling inner product of the spherical harmonics.

□ LitGene: a transformer-based model that uses contrastive learning to integrate textual information into gene representations
>> https://www.biorxiv.org/content/10.1101/2024.08.07.606674v1
LitGene, an interpretable model leveraging the transformer-based BERT. LitGene employs the method based on contrastive learning. This method predicates that embeddings of genes with common GO annotations should converge, whereas those without common GO annotations should diverge.
LitGene enables zero-shot learning and harnesses the wealth of information in the unstructured data. LitGene uses a supervised multimodal predictor merging embeddings from ProteinBERT, indicating textual information meaningfully complements data from amino acid sequences.

□ BertSNR: an interpretable deep learning framework for single nucleotide resolution identification of transcription factor binding sites based on DNA language model
>> https://academic.oup.com/bioinformatics/advance-article/doi/10.1093/bioinformatics/btae461/7728457
BertSNR adopts a multilayer bi-directional Transformer encoder. Upon inputting the DNA sequence into BertSNR, It involves k-mer tokenization. Embedding vectors are generated for each token, and these vectors undergo feature extraction through a multi-layer Transformer.
BertSNR employs multi-task learning to generate token labels, which are further transformed into nucleotide labels. All TFBSs underwent alignment, and motifs were subsequently generated based on the nucleotide frequencies at their respective positions.

□ scPriorGraph: constructing biosemantic cell–cell graphs with prior gene set selection for cell type identification from scRNA-seq data
>> https://genomebiology.biomedcentral.com/articles/10.1186/s13059-024-03357-w
scPriorGraph is a dual-channel graph neural network that integrates multi-level gene biological semantic information. Initially scPriorGraph extracts intercellular communication information from ligand-receptor network using Metapath-based random walks.
scPriorGraph obtains intracellular gene interaction information from a pathway database. These information are integrated with scRNA-seq data, resulting in multi-level gene biological semantics, and two cell KNN graphs are constructed based on different semantic information.
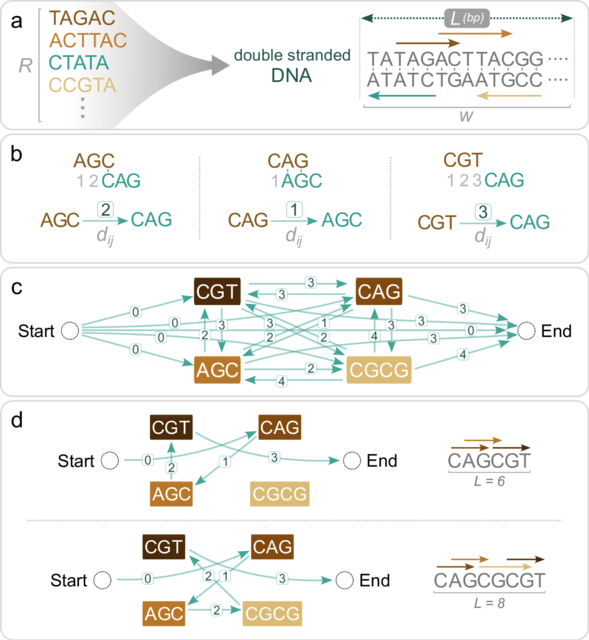

□ SPP: Generating information-dense promoter sequences with optimal string packing
>> https://journals.plos.org/ploscompbiol/article?id=10.1371/journal.pcbi.1012276
String Packing Problem (SPP), a novel computational method for the design of nucleotide sequences with densely packed DNA-protein binding sites, r elated to the classical Shortest Common Superstring problem.
SPP can be solved efficiently using integer linear programming to identify the densest arrangements of binding sites for a specified sequence length. It efficiently assembles sets of DNA-protein binding sites into dense, contiguous stretches of double-stranded DNA.

□ EPInformer: A Scalable Deep Learning Framework for Gene Expression Prediction by Integrating Promoter-enhancer Sequences with Multimodal Epigenomic Data
>> https://www.biorxiv.org/content/10.1101/2024.08.01.606099v1
EPInformer is a transformer-based framework for predicting gene expression by explicitly modeling promoter and enhancer interactions. The model integrates genomic sequences, epigenomic signals, and chromatin contacts through a flexible architecture to capture their interactions.
EPInformer uses multi-head attention modules to directly model interactions between promoters and the potential enhancers. It first creates embeddings for the promoter and putative enhancer sequences of a given gene using residual and dilated convolutions in the sequence encoder.

□ MODIFY: Machine learning-guided co-optimization of fitness and diversity facilitates combinatorial library design in enzyme engineering
>> https://www.nature.com/articles/s41467-024-50698-y
MODIFY leverages pre-trained protein language models and multiple sequence alignment (MSA)-based sequence density models to build an ensemble ML model for zero-shot fitness predictions, effectively eliminating evolutionarily unfavorable variants.
MODIFY co-optimizes the library’s diversity and predicted fitness. MODIFY offers diversity control at a residue resolution, enabling researchers to either explore a diverse range of amino acids or focus on a subset of compatible amino acids based on biophysical insights.

□ MethSCAn: Analyzing single-cell bisulfite sequencing data
>> https://www.nature.com/articles/s41592-024-02347-x
MethSCAn takes as input a number of single-cell methylation files and obtains a cell × region matrix for downstream analysis. It facilitates quality control, discovers variably methylated regions (VMRs), quantifies methylation in genomic intervals, and stores sc-methylomes.
MethSCAn obtains a methylation matrix, with one row per cell and one column per VMR, that is (in a sense) richer in information and has better signal-to-noise ratio than the matrix obtained by the simple analysis sketched at the very beginning.

□ BioLSL: Effective type label-based synergistic representation learning for biomedical event trigger detection
>> https://bmcbioinformatics.biomedcentral.com/articles/10.1186/s12859-024-05851-1
BioLSL (Biomedical Label-based Synergistic representation Learning) effectively utilizes event type labels by learning their correlation with trigger words and enriches the representation contextually.
The BioLSL model consists of three modules. Firstly, the Domain-specific Joint Encoding module employs a transformer-based, domain-specific pre-trained architecture to jointly encode input sentences and pre-defined event type labels.
Secondly, the Label-based Synergistic Representation Learning module learns the semantic relationships between input texts and event type labels, and generates a Label-Trigger Aware Representation and a Label-Context Aware Representation for enhanced semantic representations.

□ BLEND: Probabilistic Cellular Deconvolution with Automated Reference Selection
>> https://www.biorxiv.org/content/10.1101/2024.08.02.606458v1
BLEND, a hierarchical Bayesian method that leverages multiple reference datasets. BLEND learns the most suitable references for each bulk sample by exploring the convex hulls of references and employs a "bag-of-words" representation for bulk count data for deconvolution.
Unlike conventional Latent Dirichlet Allocation (LDA)-based deconvolution methods, BLEND allows references to be sample-specific and uses the data to learn each sample's most appropriate reference among all possible references in the convex hull of available references.

□ sciRED: Interpretable single-cell factor decomposition
>> https://www.biorxiv.org/content/10.1101/2024.08.01.605536v1
sciRED (Single-Cell Interpretable Residual Decomposition) enables factor discovery and interpretation in the context of known covariates. It provides an intuitive visualization of the associations b/n factors / covariates via a set of interpretability metrics for all factors.
sciRED removes known confounding effects, factorizes the residual matrix to identify additional factors not accounted for by these confounding effects, and uses rotations to maximize factor interpretability. sciRED automatically matches factors with covariates of interest.

□ FoldMason: Multiple Protein Structure Alignment at Scale
>> https://www.biorxiv.org/content/10.1101/2024.08.01.606130v1
FoldMason, a progressive multiple structural alignment (MSTA) method that leverages the structural alphabet from Foldseek, a pairwise structural aligner, for multiple alignment of hundreds of thousands of protein structures.
FoldMason represents input protein structures as strings using the 3Di+AA alphabet and computes an ungapped alignment between each pair. Pairs are sorted by alignment score and used to construct a minimum spanning guide tree.
Progressive alignment of AA +3Di structure profiles is performed following the guide tree leaf-to-root, with independent alignments computed in parallel based on rank within the guide tree.


□ Manifold learning in Wasserstein space
>> https://arxiv.org/abs/2311.08549
Infinite dimensional Riemannian geometry is an active field of research, driven, for instance, by applications in shape analysis. However, for W, the interpretation as a Riemannian manifold is purely intuitive and formal.
Aiming at building the theoretical foundations for manifold learning algorithms in the space of absolutely continuous probability measures Pac(Ω) a with Ω compact and convex subset, metrized with the Wasserstein-2 distance W.
A class of subsets A of Pac(Ω) that is not flat but still allows bounds on the approximation error of linearized optimal transport in the spirit of finite-dimensional Riemannian geometry.

□ BEAM: Bootstrap Evaluation of Association Matrices for Integrating Multiple Omics Profiles with Multiple Outcomes
>> https://www.biorxiv.org/content/10.1101/2024.07.31.605805v1
BEAM relies on bootstrapping rather than permutation, and thus has some unique capabilities. It allows the evaluation of any number of omics profiles with multiple outcomes.
BEAM computes an empirical p-value as the proportion of bootstrap association estimate matrices (AEMs) that are farther from the observed AEM in Mahalanobis distance than the complete null.

□ SeuratExtend: Streamlining Single-Cell RNA-Seq Analysis Through an Integrated and Intuitive Framework
>> https://www.biorxiv.org/content/10.1101/2024.08.01.606144v1
SeuratExtend offers a user-friendly and intuitive interface for performing a wide range of analyses, including functional enrichment, trajectory inference, gene regulatory network reconstruction, and denoising.
SeuratExtend seamlessly integrates multiple databases, such as Gene Ontology and Reactome, and incorporates popular Python tools like scVelo, Palantir, and SCENIC through a unified R interface.
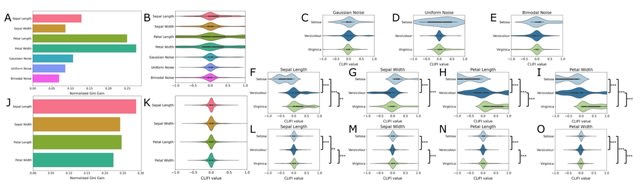
□ CLIFI: Topological embedding and directional feature importance in ensemble classifiers for multi-class classification
>> https://www.biorxiv.org/content/10.1101/2024.08.01.605982v1
CLIFI: a class-based directional feature importance metric for decision tree methods and demonstrated its use for the The Cancer Genome Atlas proteomics data.
CLIFI is incorporated into four algorithms, Random Forest, LAtent VAriable Stochastic Ensemble of Trees (LAVASET), and Gradient Boosted Decision Trees, and LAVABOOST. Both LAVA methods incorporate topological information from protein interactions into the decision function.

□ BRACE: A novel Bayesian-based imputation approach for dimension reduction analysis of alternative splicing at single-cell resolution
>> https://www.biorxiv.org/content/10.1101/2024.08.01.606201v1
BRACE, a novel Bayesian-based imputation method for PSI estimation and demonstrated its application on dimension reduction analysis of single-cell alternative splicing dataset to enable dimension reduction analysis across a range of datasets with differing complexity.
The numerator is total number of splice junctions supporting the inclusion of the alternative exon. The denominator is the total number of splice junctions supporting the inclusion or exclusion of the alternative exon, i.e, total coverage at that site across all isotorm molecules.

□ Cellular proliferation biases clonal lineage tracing and trajectory inference
>> https://academic.oup.com/bioinformatics/advance-article/doi/10.1093/bioinformatics/btae483/7727666
A mathematical analysis that proves that the relative abundance of subpopulations is changed, or biased, in multi-time clonal datasets. The source of the bias is heterogeneous growth rates; cells with more descendants are more likely to be represented in multi-time clones.
The performance of trajectory inference methods such as CoSpar, which rely on this biased information, may be negatively impacted by the presence of this sampling bias. LineageOT-MT incorporates information from multi-time clonal barcodes.
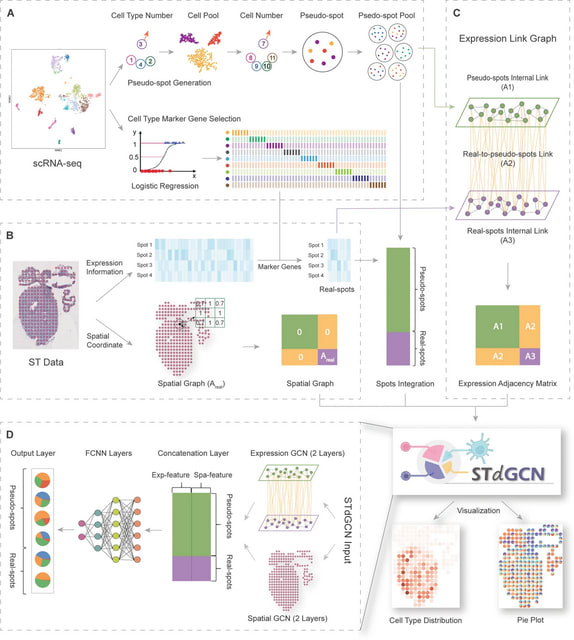
□ STdGCN: spatial transcriptomic cell-type deconvolution using graph convolutional networks
>> https://genomebiology.biomedcentral.com/articles/10.1186/s13059-024-03353-0
STdGCN employs the scRNA-seq reference data to identify cell-type marker genes and generate a pseudo-spot pool. It then builds two link graphs: a spatial graph and an expression graph.
The expression graph is a hybrid graph composed of three sub-graphs, a pseudo-spot internal graph, a real-spot internal graph, and a real-to-pseudo-spot graph.
These sub-graphs are formed using mutual nearest neighbors (MNN) based on expression similarity. Based on the two link graphs, a GCN-based model is utilized to propagate information from both real- and pseudo-spots.

□ GenomeSpy: Deciphering cancer genomes with GenomeSpy: a grammar-based visualization toolkit
>> https://academic.oup.com/gigascience/article/doi/10.1093/gigascience/giae040/7727441
GenomeSpy, a grammar-based toolkit for authoring tailored, interactive visualizations for genomic data analysis. By using combinatorial building blocks and a declarative language, users can implement new visualization designs easily and embed them.
GenomeSpy core library parses the specification and renders it using GPU-accelerated graphics to ensure smooth interactions such as zooming and panning. The score-based semantic zoom controls overplotting during navigation.

□ Chromatin-dependent motif syntax defines differentiation trajectories
>> https://www.biorxiv.org/content/10.1101/2024.08.05.606702v1
Uncovering a chromatin-dependent motif syntax with high predictive value that is composed of preexisting DNA accessibility, motif variations including flanking bases, motif occurrence, and their relative positions.
NGN2 and MyoD1 open chromatin depending on single base-pair differences in their motifs, with patterns that surprisingly differ from their mere binding strength.
Cellular and in vitro assays reveal that other transcription factors, as well as NGN2 and MyoD1 dimerization-partners, differentially interact with these motif variants.

□ mosGraphFlow: a novel integrative graph AI model mining disease targets from multi-omic data
>> https://www.biorxiv.org/content/10.1101/2024.08.01.606219v1
mosGraphFlow enhances the analysis and prediction capabilities in multi-omics data, which aims to leverage the strengths of both models to provide a comprehensive and interpretable analysis.
The integrated model combines the detailed graph construction The integrated model combines the detailed graph construction capabilities of mosGraphGen with the advanced predictive functionalities of M3NetFlow.

□ mosGraphGPT: a foundation model for multi-omic signaling graphs using generative AI
>> https://www.biorxiv.org/content/10.1101/2024.08.01.606222v1
mosGraphGPT, a foundation model for multi-omic signaling (mos) graphs, in which the multi-omic data was integrated and interpreted using a multi-level signaling graph.
mosGraphGPT leverages extensive pre-training capabilities to capture complex gene-gene and gene-cell interactions with high accuracy and contextual relevance. Earlier stage message passing was accomplished to propagate information to the protein nodes.

□ scMaui: a widely applicable deep learning framework for single-cell multiomics integration in the presence of batch effects and missing data
>> https://bmcbioinformatics.biomedcentral.com/articles/10.1186/s12859-024-05880-w
scMaui (Single-cell Multiomics Autoencoder Integration) can model all possible kinds of modalities with a flexible reconstruction loss function that supports varied probabilistic distributions including not only negative binomial but also Poisson, negative multinomial distributions.
Each single-cell multiomics assay is given to an encoder and batch effect factors are independently handled by covariates and adversary networks.
Latent factors created by scMaui can be used for downstream analyses to find cellular heterogeneity and reconstructed assays by the decoders can be used for imputation.

□ iSODA: A Comprehensive Tool for Integrative Omics Data Analysis in Single- and Multi-Omics Experiments
>> https://www.biorxiv.org/content/10.1101/2024.08.02.605811v1
iSODA, an interactive web-based application for the analysis of single-as well as multi-omics omics data. The software tool emphasizes intuitive, interactive visualizations designed for user-driven data exploration.
iSODA incorporates Multi-Omics Factor Analysis - MOFA, and Similarity Network Fusion - SNF. All results are presented in interactive plots with the possibility of downloading plots and associated data.

□ CellClear: Enhancing Single-cell RNA Data Quality via Biologically-Informed Ambient RNA Correction
>> https://www.biorxiv.org/content/10.1101/2024.08.05.606571v1
CellClear, which can accurately identify and correct ambient genes while preserving the biological features of the data. CellClear also provides an ambient expression level as a C metric to guide researchers in deciding whether to apply the correction.
The CellClear method employs clustering and Non-Negative Matrix Factorization (NMF) to derive cluster-relevant expression programs from foreground cell matrix, which is the cell associated matrix identified by primary analysis pipelines.

□ Pertpy: an end-to-end framework for perturbation analysis
>> https://www.biorxiv.org/content/10.1101/2024.08.04.606516v1
Pertpy provides access to harmonized perturbation datasets and metadata databases along with numerous fast and user-friendly implementations of both established and novel methods such as automatic metadata annotation or perturbation distances to efficiently analyze perturbation data.
Perty discriminates between two fundamental domains to embed and analyze data: the "cell space" and the "perturbation space". In this paradigm, the cell space represents configurations where discrete data points represent individual cells.
Conversely, the perturbation space departs from the individualistic perspective of cells and instead categorizes cells based on similar response to perturbation or expressed phenotype where discrete data points represent individual perturbations.

□ fastglmpca: Accelerated dimensionality reduction of single-cell RNA sequencing data
>> https://academic.oup.com/bioinformatics/advance-article/doi/10.1093/bioinformatics/btae494/7729117
fastgImpca implements fast algorithms for dimensionality reduction of count data based on the Poisson GLM-PCA model. fastgImpca is available on CRAN for all major computing platforms. It features a well-documented, user-friendly interface that aligns closely w/ gImpca and scGBM.
The Alternating Poisson Regression (APR) approach has strong convergence guarantees; the block-coordinatewise updates monotonically improve the log-likelihood, and under mild conditions converge to a (local) maximum of the likelihood.

□ LongReadSum: A fast and flexible quality control and signal summarization tool for long-read sequencing data
>> https://www.biorxiv.org/content/10.1101/2024.08.05.606643v1
LongReadSum, a computational tool for fast, comprehensive, and high throughput long read QC: It supports data format types for all major sequencing technologies (FASTA, FASTQ, POD5, FAST5, basecall summary files, unaligned BAM and aligned BAM).
LongReadSum provides a summary report of read and base alignment metrics, including a summary of each type of read and base alignment. High read and base alignment rates are indicative of high-quality sequencing data, and thus are important QC metrics.

□ Deciphering the role of structural variation in human evolution: a functional perspective
>> https://www.sciencedirect.com/science/article/pii/S0959437X24000893
As T2T assemblies and pangenomes of diverse primates and humans become routine, improved discovery of variation at recalcitrant regions - satellite repeats comprising centromeres and acrocentric regions — will allow to explore the most quickly evolving parts of our genomes.
Increasing the number of genomes across species will delineate variants that are fixed and divergent b/n primate species that might contribute to human universal features from polymorphic w/in species that can impact diverse phenotypes responsive to varied environmental factors.

□ GENEVIC: GENetic data exploration and visualization via intelli- gent interactive console
>> https://academic.oup.com/bioinformatics/advance-article/doi/10.1093/bioinformatics/btae500/7730006
GENEVIC is assessed using a curated database that ranks genetic variants associated with Alzheimer's disease, schizophrenia, and cognition, based on their effect weights from the Polygenic Score Catalog, enabling researchers to prioritize genetic variants in complex diseases.
GENEVIC leverages Domain-Specific Retrieval Augmented Generation (RAG) to enhance factual accuracy by integrating LLMs with curated databases, external sources such as bioinformatics APIs, and literature sites, ensuring responses are based on verified information.
※コメント投稿者のブログIDはブログ作成者のみに通知されます