Biblically accurate angels. Read more: https://t.co/cBzwJnUS3u pic.twitter.com/6X0peeuCZi
— History Defined (@historydefined) April 25, 2023
“Their entire bodies, including their backs, hands, and wings, were full of eyes all around, as were their four wheels.” (Ezekiel 10:12)
□ Biblically Accurate Angels Would Actually Be Pretty Scary
>> https://www.historydefined.net/biblically-accurate-angels-would-actually-be-pretty-scary/

□ nanoSHAPE: Direct detection of RNA modifications and structure using single-molecule nanopore sequencing
>> https://www.cell.com/cell-genomics/fulltext/S2666-979X(22)00014-3
nanoSHAPE combines long-read, direct RNA sequencing with a new SHAPE reagent that, by virtue of its high reactivity and small adduct size, enables full-length probing of structure in long RNAs.
The nanoSHAPE centroid structure includes fewer long-range base pairs and has larger loop sizes than does the SHAPE-MaP-based structure. nanoSHAPE features a 3′-to-5′ read direction, may over-detect reactivity at loop-closing base pairs.

□ GraphCPLMQA: Assessing protein model quality based on deep graph coupled networks using protein language model
>> https://www.biorxiv.org/content/10.1101/2023.05.16.540981v1
The GraphCPLMQA consists of a graph encoding module and a transform-based convolutional decoding module. The underlying relational representations of sequence and high-dimensional geometry structure are extracted by protein language models with Evolutionary Scale Modeling.
The mapping connection between structure and quality are inferred by the representations and low-dimensional features. The triangular location and residue level contact order features are designed to enhance the association between the local structure and the overall topology.

□ The emergence of clusters in self-attention dynamics
>> https://arxiv.org/abs/2305.05465
Characterizing clustered representations of a trained Transformer by studying the asymptotic behavior of a sequence of tokens (X1 (t), ..., In (t)) as they evolve through the layers of a transformer architecture using the dynamics.
Particles, representing tokens, tend to cluster toward particular limiting objects as time tends to infinity. The type of limiting object that emerges depends on the spectrum of the value matrix. In the one-dimensional case, the self-attention matrix converges to a low-rank Boolean matrix.

□ The edge of chaos: quantum field theory and deep neural networks
>> https://scipost.org/10.21468/SciPostPhys.12.3.081
The edge of chaos is determined by the point at which the largest Lyapunov exponent becomes positive, which yields precisely the criticality condition.
They compute both the O(1) corrections quantifying fluctuations from typicality in the ensemble of networks, and the subleading O(T/N) corrections due to finite-width effects.
These provide corrections to the correlation length that controls the depth to which information can propagate through the network, and thereby sets the scale at which such networks are trainable by gradient descent.
This analysis provides a first-principles approach to the rapidly emerging NN-QFT correspondence, and opens several interesting avenues to the study of criticality in deep neural networks.

□ MIDAS: Protein-metabolite interactomics of carbohydrate metabolism reveal regulation of lactate dehydrogenase
>> https://www.science.org/doi/10.1126/science.abm3452
MIDAS (mass spectrometry integrated with equilibrium dialysis for the discovery of allostery systematically) probes interactions for 33 enzymes from human carbohydrate metabolism identified 830 protein-metabolite interactions.
MIDAS can detect many new interactions, incl. regulation of lactate dehydrogenase by ATP and long-chain acyl coenzyme A, which may help to explain known physiological relations between fat and carbohydrate metabolism in different tissues.
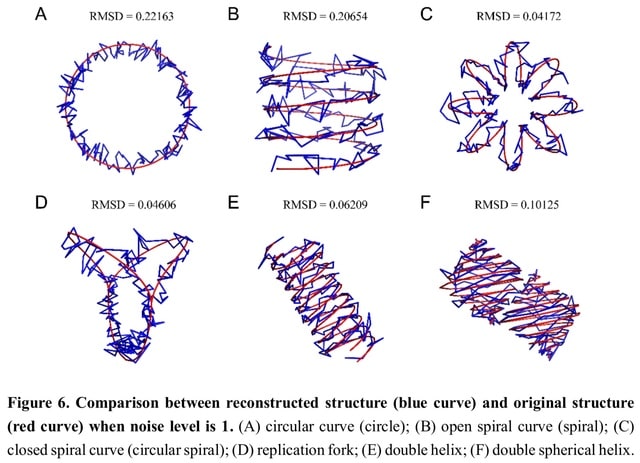
□ EVRC: Reconstruction of chromosome 3D structure models using Error-Vector Resultant algorithm with Clustering coefficient
>> https://www.biorxiv.org/content/10.1101/2023.05.11.540436v1
The EVRC algorithm that utilizes Hi-C experiments data to reconstruct the 3D structure of chromatin. EVRC relies on the co-clustering coefficient and error-vector resultant. The EVRC algorithm begins by calculating the co-clustering coefficient between chromatin fragments.
In the 3D structure, the reciprocal of the space distance between each two points is taken as the interaction frequency between the two points, and the interaction matrix of the structure is obtained in this way.
The single-chain structure generates only the interaction matrix within a single curve (simulating single chromosome), while the double-chain structure generates the interaction matrix within each of the two curves and the interaction matrix.

□ Cactus: a user-friendly and reproducible ATAC-Seq and mRNA-Seq analysis pipeline for data preprocessing, differential analysis, and enrichment analysis
>> https://www.biorxiv.org/content/10.1101/2023.05.11.540110v1
Cactus conducts preprocessing on raw sequencing reads, followed by differential analysis between conditions. Results are split into Differential Analysis Subsets (DASs) based on significance threshold, direction of change, annotated genomic regions, and experiment type.
Cactus conducts preprocessing on raw sequencing reads, followed by differential analysis between conditions. Results are split into Differential Analysis Subsets (DASs) based on significance threshold, direction of change, annotated genomic regions, and experiment type.

□ Baldur: Bayesian hierarchical modeling for label-free proteomics exploiting gamma dependent mean-variance trends
>> https://www.biorxiv.org/content/10.1101/2023.05.11.540411v1
Baldur, a novel Bayesian regression model to characterize local mean-variance trends in the data to describe measurement uncertainty and to estimate the decision model hyperparameters.
Baldur vastly improves over state-of-the-art methods (Limma-Trend and t-test) in several spike-in datasets by having competitive performance in detecting true positives while showing superiority by greatly reducing false positives.

□ MethPhaser: methylation-based haplotype phasing of human genomes
>> https://www.biorxiv.org/content/10.1101/2023.05.12.540573v1
MethPhaser, a tool that operates on a set of already phased variants based on SNVs from, e.g., WhatsHap or Hapcut2. MethPhaser then utilizes the heterozygous methylation information across the autosomes to connect phaseblocks together and thus improve the overall phasing.
MethPhaser improves variant-based phasing with minimal impact on phasing errors. MethPhaser is able to combine both phaseblocks and thus generate a single larger block by leveraging the heterozygous methylation signal in this region.

□ Resolving the unsolved: Comprehensive assessment of tandem repeats at scale
>> https://www.biorxiv.org/content/10.1101/2023.05.12.540470v1
Tandem Repeat Genotyping Tool (TRGT), a novel method for repeat analysis of long reads, as well as a companion method for Tandem Repeat Visualization (TRVZ). TRGT makes it possible to analyze structurally complex tandem repeats.
TRVZ affords a visual inspection of repeat alleles. TRGT reports haplotype-resolved germline variation together with methylation status across simple and complex TRs, and can detect mosaic mutations.

□ Towards Computing Attributions for Dimensionality Reduction Techniques
>> https://www.biorxiv.org/content/10.1101/2023.05.12.540592v1
An efficient implementation for the gradient computation for this dimensionality reduction technique. We show that our explanations identify significant features using novel validation methodology; using synthetic datasets and the popular MNIST benchmark dataset.
Inspecting the gradients of t-SNE in the same manner as one would look at gradients with respect to their inputs in relation to supervised classifiers trained also via Stochastic Gradient Descent.

□ TDbasedUFE and TDbasedUFEadv: bioconductor packages to perform tensor decomposition based unsupervised feature extraction
>> https://www.biorxiv.org/content/10.1101/2023.05.14.540687v1
TDbasedUFE and TDbasedUFEadv are easy for a person who is not familiar with the concept of tensors to use. Since the matrix can be regarded as a two-mode tensor, TDbasedUFE and TDbasedUFEadv are also used to apply PCA-based unsupervised FE to the dataset.
TDbasedUFE focuses only on two popular functions among those possible by TD-based unsupervised FE, since TD-based unsupervised FE can perform numerous applications, not all of which are required by the majority of people.
TDbasedUFE and TDbasedUFEadv accept a multiple omics profile dataset formatted as a tensor to which TD is applied. They employed Tucker decomposition as a TD using the higher-order singular value decomposition (HOSVD) algorithm.
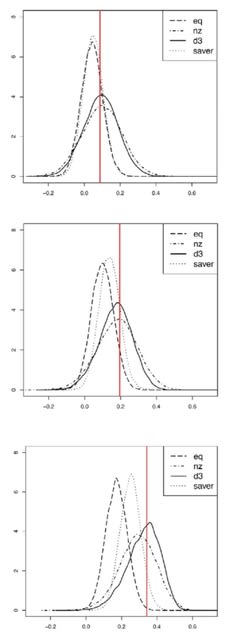
□ SCSC: Considering Zeros in Single Cell Sequencing Data Correlation Analysis
>> https://www.biorxiv.org/content/10.1101/2023.05.13.540566v1
SCSC is versatile and logically adaptable to single-cell multi-omic measures. It can be used for assessing the gene-gene co-exression and genetic feature-gene expression correlation. Four strategies (conventional, non-zero, dropout-weighted, imputation) were enabled.
Filtering out zeros reduces the MAE of correlation estimation compared to using all original or imputed data, in almost all scenarios with varying drop-out rates, expression levels, total number of cells, single- cell library sizes, overdispersion scales and variations.

□ uORF4u: a tool for annotation of conserved upstream open reading frames
>> https://academic.oup.com/bioinformatics/advance-article/doi/10.1093/bioinformatics/btad323/7162684
A tool for conserved uORF annotation in 5ʹ upstream sequences of a user-defined protein of interest or a set of protein homologues. It can also be used to find small conserved ORFs within a set of nucleotide sequences.
The output includes publication-quality figures with multiple sequence alignments, sequence logos and locus annotation of the predicted conserved uORFs in graphical vector format.
For identified potential frames, the tool searches for conserved ORFs using a greedy algorithm: uORF4u iterates through sequences and tries to maximise the sum of pairwise alignment scores between uORFs.

□ LENS: Landscape of Effective Neoantigens Software
>> https://academic.oup.com/bioinformatics/advance-article/doi/10.1093/bioinformatics/btad322/7162685
LENS (Landscape of Effective Neoantigen Software) predicts tumor-specific/associated antigens from single nucleotide variants, insertions and deletions, fusion events, splice variants, cancer testis antigens, overexpressed self-antigens, viruses, and endogenous retroviruses.
LENS includes phasing and germline variant information in epitope identification, and it harmonizes variant RNA expression across genomic sources to provide a more usable relative expression ranking each peptide epitope.

□ scAnno: a deconvolution strategy-based automatic cell type annotation tool for single-cell RNA-sequencing data sets
>> https://academic.oup.com/bib/advance-article-abstract/doi/10.1093/bib/bbad179/7161854
scAnno (scRNA-seq data annotation), an automated annotation tool for scRNA-seq data sets primarily based on the single-cell cluster levels, using a joint deconvolution strategy and logistic regression.
scAnno offers a possibility to obtain genes with high expression and specificity in a given cell type as cell type-specific genes (marker genes) by combining co-expression genes with seed genes as a core.

□ SonicParanoid2: fast, accurate, and comprehensive orthology inference with machine learning and language models
>> https://www.biorxiv.org/content/10.1101/2023.05.14.540736v1
Sonic aranoid2 performs de novo orthology inference using a novel graph-based algorithm that halves the execution time with an AdaBoost classifier and avoiding unnecessary alignments. SonicParanoid2 conducts domain-based orthology inference using Doc2Vec neural network models.
SonicParanoid2 uses fast profile searches on Pfam? to infer the domain architectures of the input proteins and converts them into "phrases", where "words" are the annotated functional domains and the amino-acid lengths of the inter-domain regions.
The orthologs predicted by these two algorithms are merged and input into the Markov cluster algorithm (MCL) to infer the ortholog groups (OGs) for the N input proteomes.

□ Transcription factor exchange enables prolonged transcriptional bursts
>> https://www.biorxiv.org/content/10.1101/2023.05.15.540758v1
A tracking system to understand how transient TF binding relates to transcription activation. Gal4 is naturally lowly abundant and only has 15 genomic binding sites and the shared GALI-GAL10 promoter is the promoter with the most binding sites.
To measure Gal4 binding and GAL10 transcription simultaneously, we developed a tracking algorithm to track the GAL10 TS in a single plane in the z direction with an active feedback loop.
The main kinetic mode of activation of GAL10 is that Gal4 molecules show cooperative binding / exchange during a burst. A general mechanism for TF-mediated regulation, where TF cooperative binding and TF exchange at multiple binding sites enable prolonged transcriptional bursts.

□ Speed reading the epigenome and genome
>> https://www.nature.com/articles/s41587-023-01757-0
Dual sequencing of the epigenome and genome could have broad implications in oncology.

□ The ENCODE4 long-read RNA-seq collection reveals distinct classes of transcript structure diversity
>> https://www.biorxiv.org/content/10.1101/2023.05.15.540865v1
A framework to systematically characterize and quantify the diversity between the detected transcripts from each gene by computing a summary gene triplet, which is related to but distinct from transcript triplets.
Using triplets in a simplex representation demonstrates how promoter selection, splice pattern, and 3’ processing are deployed across human tissues, with nearly half of multi-transcript protein coding genes showing a clear bias toward one of the three diversity mechanisms.

□ Cello scope: a probabilistic model for marker-gene-driven cell type deconvolution in spatial transcriptomics data
>> https://genomebiology.biomedcentral.com/articles/10.1186/s13059-023-02951-8
Cello scope, a novel Bayesian probabilistic graphical model of gene expression in ST data, which deconvolutes cell type composition in ST spots, and a method to infer model parameters based on an MCMC algorithm.
Cello scope implements a semi-automatic procedure of marker selection. Cello scope considers that the expression levels of marker genes in each cell type are unknown and are modeled using hidden variables Λ.

□ fimpera: drastic improvement of Approximate Membership Query data-structures with counts
>> https://academic.oup.com/bioinformatics/advance-article/doi/10.1093/bioinformatics/btad305/7169157
fimpera, that enables the improvement of any cAMQ performance. Applied to counting Bloom filters, our proposed algorithm reduces the false positive rate by two orders of magnitude and it improves the precision of the reported abundances.
fimpera allows for the reduction of the size of a counting Bloom filter by two orders of magnitude while maintaining the same precision. fimpera does not introduce any memory overhead and may even reduces the query time.

□ RabbitTClust: enabling fast clustering analysis of millions of bacteria genomes with MinHash sketches
>> https://genomebiology.biomedcentral.com/articles/10.1186/s13059-023-02961-6
RabbitTClust, an efficient clustering toolkit based on MinHash sketch distance measurement for large-scale genome datasets. Fast sketching (an approximate, compact summary of the original data) is used to compute similarities among genomes with a small memory footprint.
clust-mst relies on a graph-based linear space clustering algorithm based on minimum spanning tree (MST) computation to perform single-linkage hierarchical clustering.
This MST construction relies on dynamically generating and merging partial clustering results without storing the whole distance matrix, which in turn allows for both memory reduction and efficient parallelization.

□ GbyE: A New Genome Wide Association and Prediction Model based on Genetic by Environmental Interaction
>> https://www.biorxiv.org/content/10.1101/2023.05.17.541129v1
GbyE, a new genotype design model program for genome-wide association and prediction using Kronecker product, which can enhance the statistical power of GWAS and GS by utilizing the interaction effects of multiple environments or traits.
The GbyE model improves the prediction accuracy of the three Bayesian models BRR, BayesA, and BayesLASSO using information from GEI and increases the prediction accuracy by 9.4%, 9.1%, and 11%, respectively, relative to the Mean value method.

□ scNanoATAC-seq: a long-read single-cell ATAC sequencing method to detect chromatin accessibility and genetic variants simultaneously within an individual cell
>> https://www.nature.com/articles/s41422-022-00730-x
scNanoATAC-seq is a TGS platform-based long-read single-cell ATAC sequencing method that can be applied in various biological fields. It can detect chromatin accessibility and genetic variants (including SVs, SNPs, and CNVs) within an individual cell simultaneously.
scNanoATAC-seq provides the direct evidence of co-accessibility between neighboring peaks from scNanoATAC-seq, where the chromatin accessibility of two sites in the same single cell and in fact on the same allele was detected simultaneously by a long read.

□ Sequencing accuracy and systematic errors of nanopore direct RNA sequencing
>> https://www.biorxiv.org/content/10.1101/2023.03.29.534691v2
The systematic sequencing errors at single nucleotide and motif levels are also prevalent in the reads basecalled by RODAN, suggesting that the fundamental causes of sequencing between read quality scores and error rates, and how adaptor detection failure can impact the read quality of short sequences.
While read quality scores approximated error rates at base and read levels, failure to detect DNA adapters may lead to data loss. By comparing distinct basecallers, some sequencing errors are attributable to signal insufficiency rather than algorithmic (base-calling) artefacts.

□ CENTRE: A gradient boosting algorithm for Cell-type-specific ENhancer-Target pREdiction
>> https://www.biorxiv.org/content/10.1101/2023.05.16.541035v1
CENTRE is a machine learning framework that predicts enhancer target interactions in a cell-type-specific manner, using only gene expression and ChIP-seq data for three histone modifications for the cell type of interest.
CENTRE computes CT-specific and generic features for all potential ET pairs. ET feature vectors are then fed to a pre-trained XGBOOST classifier, and a probability of an interaction is assigned to ET pairs. ET pairs w/ higher probability than 0.5 are labeled as interacting pairs.
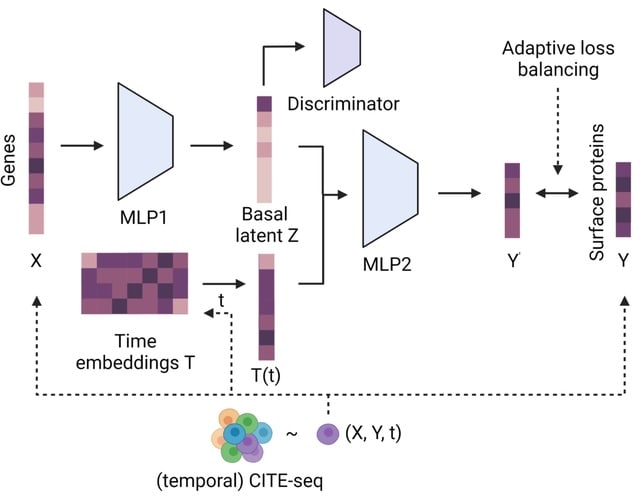
□ CrossmodalNet: Interpretable modeling of time-resolved single-cell gene-protein expression
>> https://www.biorxiv.org/content/10.1101/2023.05.16.541011v1
CrossmodalNet, an interpretable ML model with customized adaptive loss that learns to translate between modalities of genes and proteins using CITE-seq data while encoding temporal information. CrossmodalNet is capable of elucidating noise-free causal gene-protein relationships.
By combining the interpretability of linear models with the flexibility of non-linear models, CrossmodalNet decomposes transcriptional information of cells into basal and temporal domain, with the latter forming an easy-to-interpret time embedding.

□ Adaptive RAxML-NG: Accelerating Phylogenetic inference under Maximum Likelihood using dataset difficulty
>> https://www.biorxiv.org/content/10.1101/2023.05.15.540873v1
Adaptive RAxML-NG is based on two new mechanisms for faster and more efficient exploration, that is, NNI rounds and the 1% ML convergence interval to terminate the first more superficial phase of topological moves early.
Adaptive RAxML-NG modifies the thoroughness of the tree search strategy, as well as additional heuristic search parameters (e.g., the number of distinct starting trees or the maximum subtree re-insertion radius of SLOW-SPR moves), as a function of the predicted difficulty.

□ MASIv2 enables standardization and integration of multi-modal single-cell and spatial omics data with one general framework
>> https://www.biorxiv.org/content/10.1101/2023.05.15.540808v1
MASIv2 can scale to integrate multiple modalities at once, including gene expression, chromatin accessibility, DNA methylation, and chromatin structure.
MASIv2 uses Louvain community detection to identify a group of key points for each modality. Next, they train a linear regression model that can match two key points of the same cell type but from two modalities. It only adds K * (Q - 1) trainable weights with O - 1 bias terms into the framework.

□ Minmers are a generalization of minimizers that enable unbiased local Jaccard estimation
>> https://www.biorxiv.org/content/10.1101/2023.05.16.540882v1
Minmers are a novel "non-forward" winnowing scheme with a (w, s)-window guarantee. It generalizes the minimizer scheme by use of a rolling minhash with multiple sampled k-mers per window.
Minmers eliminate Jaccard estimator bias and enable new methods to reduce mapping runtime compared to MashMap2. MashMap3 with minmers not only produced unbiased and more accurate predictions of the ANI than Minimap2 and MashMap2, but it did so in a fraction of the time.

□ SPUMONI 2: improved classification using a pangenome index of minimizer digests
>> https://genomebiology.biomedcentral.com/articles/10.1186/s13059-023-02958-1
SPUMONI 2 is an efficient tool for sequence classification of both short and long reads. It performs multi-class classification using a novel sampled document array.
By incorporating minimizers, SPUMONI 2’s index is 65 times smaller than minimap2’s for a mock community pangenome. SPUMONI 2 achieves a speed improvement of 3-fold compared to SPUMONI and 15-fold compared to minimap2.
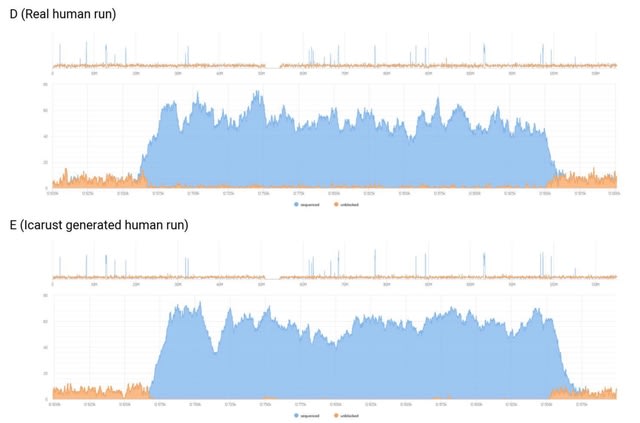
□ Icarust, a real-time simulator for Oxford Nanopore adaptive sampling.
>> https://www.biorxiv.org/content/10.1101/2023.05.16.540986v1
Icarust, a tool enabling more accurate approximations of sequencing runs. Icarust recreates all the required endpoints of MinKNOW to perform adaptive sampling and writes output compatible with current base-callers and analysis pipelines.
Icarust is capable of serving nanopore signal to simulate a MinION or PromethION flow cell experiment from any reference genome using either R9 or R10 pore signal.
※コメント投稿者のブログIDはブログ作成者のみに通知されます