
我々は『今』を証明する為に、未来を生きている。

□ Tunings for leapfrog integration of Hamiltonian Monte Carlo for estimating genetic parameters
>> https://www.biorxiv.org/content/biorxiv/early/2019/10/16/805499.full.pdf
Hamiltonian Monte Carlo is based on Hamiltonian dynamics, and it follows Hamilton’s equations, which are expressed as two differential equations.
In the sampling process of Hamiltonian Monte Carlo, a numerical integration method called leapfrog integration is used to approximately solve Hamilton’s equations, and the integration is required to set the number of discrete time steps and the integration stepsize.

□ Cumulus: a cloud-based data analysis framework for large-scale single-cell and single-nucleus RNA-seq
>> https://www.biorxiv.org/content/biorxiv/early/2019/10/30/823682.full.pdf
Cumulus consists of a cloud analysis workflow, a Python analysis package (Pegasus), and a visualization application (Cirrocumulus).
Cumulus executes the first two steps – sequence read extraction and gene-count matrix generation – parallelly across a large number of computer nodes, and executes the last step of analysis in a single multi-CPU node, using its highly efficient analysis module - Pegasus.
□ GraphAligner: Rapid and Versatile Sequence-to-Graph Alignment
>> https://www.biorxiv.org/content/biorxiv/early/2019/10/21/810812.full.pdf
GraphAligner is 12x faster and uses 5x less memory, making it as efficient as aligning reads to linear reference genomes. When employing GraphAligner for error correction, almost 3x more accurate and over 15x faster than extant tools.
GraphAligner, Seed-and-extend program for aligning long error-prone reads to genome graphs. For a description of the bitvector alignment extension algorithm.

□ Linnaeus: Interpretable Deep Learning Classification of Single Cell Transcript Data https://www.biorxiv.org/content/biorxiv/early/2019/10/29/822759.full.pdf
Linnaeus using the python Shapley Additive Explanations (SHAP) module, dataset feature importance is evaluated as Shapley values.
layers can be individually pretrained in the form of a Restricte Boltzmann Machine (RBM), which is an energy-based Markov Random Field model. In Linnaeus, a new model is first created by pretraining each layer as an RBM using the contrastive-divergence method for 50 epochs.
Linnaeus leverages Deep Learning architectures and GA meta-optimization to create optimized novel classifiers and generate feature importance information for high-throughput datasets using a simple genetic algorithm as well as an autoencoder to classifier transfer learning.

□ Genome Constellation: A new method for rapid genome classification, clustering, visualization, and novel taxa discovery from metagenome
>> https://www.biorxiv.org/content/biorxiv/early/2019/10/21/812917.full.pdf
Genome Constellation calculates similarities between genomes based on their whole genome sequences, and subsequently uses these similarities for classification, clustering and visualization.
The clusters of reference genomes formed by Genome Constellation closely resemble known phylogenetic relationships while simultaneously revealing unexpected connections.

□ SLR: a scaffolding algorithm based on long reads and contig classification
>> https://bmcbioinformatics.biomedcentral.com/articles/10.1186/s12859-019-3114-9
Through the alignment information of long reads and contigs, SLR classifies the contigs into unique contigs and ambiguous contigs for addressing the problem of repetitive regions.
Next, SLR uses only unique contigs to produce draft scaffolds. Then, SLR inserts the ambiguous contigs into the draft scaffolds and produces the final scaffolds.

□ Unsupervised generative and graph representation learning for modelling cell differentiation
>> https://www.biorxiv.org/content/biorxiv/early/2019/10/16/801605.full.pdf
unsupervised generative neural methods, based on the variational autoencoder, that can model cell differentiation by building meaningful representations from the high dimensional and complex gene expression data.
a disentangled generative probabilistic framework based on information theory to improve the data representation and achieve better separation of the latent biological factors of variation in the gene expression data.
□ Investigating tissue-relevant causal molecular mechanisms of complex traits using probabilistic TWAS analysis
>> https://www.biorxiv.org/content/biorxiv/early/2019/10/17/808295.full.pdf
probabilistic TWAS (PTWAS) provides novel functionalities to evaluate the causal assumptions and estimate tissue- or cell-type specific causal effects of gene expression on complex traits.
PTWAS is built upon the causal inference framework of IV analysis, and utilizes probabilistic eQTL annotations derived from multi-variant Bayesian fine-mapping analysis conferring higher power to detect TWAS associations than existing methods.
□ OCSANA+: Optimal Control and Simulation of Signaling Networks from Network Analysis
>> https://www.biorxiv.org/content/biorxiv/early/2019/10/16/806315.full.pdf
OCSANA+ identifies driver nodes that control non-linear systems’ long-term dynamics, prioritizing combinations of interventions in large scale complex networks, and estimating the effects of node perturbations in signaling networks, all based on the analysis of the network’s structure.
the ability of OCSANA+ to successfully reproduce simulated and experimental results from two biological signaling networks with non-linear dynamics.
OCSANA+, FC and SFA algorithms are able to reproduce the results of the Boolean simulation, and has an accuracy of about 60-80% for estimation of steady state values based only on topological information provided by non-linear dynamics.

□ Beyond generalization: Enhancing accurate interpretation of flexible models
>> https://www.biorxiv.org/content/biorxiv/early/2019/10/17/808261.full.pdf
This framework allows for direct comparison of the inferred hypotheses with the ground truth on synthetic data, thus testing the correctness of interpretation.
The gradient-descent optimization continuously improves the training likelihood, producing a sequence of models with increasing complexity.
re-sampling a new data realization on each gradient-descent iteration — mimicking the infinite data regime — results in a robust recovery of the ground truth.

□ MISC: Inferring the structures of signaling motifs from paired dynamic traces of single cells
>> https://www.biorxiv.org/content/biorxiv/early/2019/10/17/809434.full.pdf
If the assumption holds, then repeated measurements of upstream and downstream signaling dynamics in single cells could provide information about the underlying signaling motif for a given pathway, even when no prior knowledge of that motif exists.
MISC (Motif Inference from Single Cells) algorithm infers the underlying signaling motif from paired time-series measurements from individual cells. MISC predicted signaling motifs that were consistent with previous mechanistic models of transcription.
MISC can hypothesize unknown signaling intermediates based on the dynamical behaviors of interacting signaling factors.

□ GenIE-Sys: Genome Integrative Explorer System
>> https://www.biorxiv.org/content/biorxiv/early/2019/10/17/808881.full.pdf
GenIE-Sys can be installed in different infrastructures such as XAMP/MAMP. MySQL database is required only to load the genomic data and integrate with GenIE-Sys plugins.

□ manta - a clustering algorithm for weighted ecological networks
>> https://www.biorxiv.org/content/biorxiv/early/2019/10/17/807511.full.pdf
manta is a novel heuristic flow-based network clustering algorithm, which equals or outperforms existing algorithms on noise-free synthetic data.
manta represents an alternative to the popular flow-based MCL algorithm that in contrast to MCL can take optimal advantage of edge signs and does not need parameter optimization.
□ Scaled Simplex Representation for Subspace Clustering
>> https://ieeexplore.ieee.org/document/8871334
a scaled simplex representation (SSR) for the SC problem. the non-negative constraint is used to make the coefficient matrix physically meaningful, and the coefficient vector is constrained to be summed up to a scalar to make it more discriminative.
The proposed SSR-based SC (SSRSC) model is reformulated as a linear equality-constrained problem, which is solved efficiently under the alternating direction method of multipliers framework.
□ Unsupervised Rotation Factorization in Restricted Boltzmann Machines
>> https://ieeexplore.ieee.org/document/8870198
an extended novel RBM that learns rotation invariant features by explicitly factorizing for rotation nuisance in 2D image inputs within an unsupervised framework.
using the γ-score, a measure that calculates the amount of invariance, to mathematically and experimentally demonstrate that this approach indeed learns rotation invariant features.
□ Spatially-mapped single-cell chromatin accessibility
>> https://www.biorxiv.org/content/biorxiv/early/2019/10/22/815720.full.pdf
sciMAP-ATAC preserves cellular localization within intact tissues and generates thousands of spatially-resolved high quality single-cell ATAC-seq libraries.
clear waves of TF motif enrichment along cells ordered by pseudospace from the union dataset, thus enforcing the paradigm that resolve spatial epigenomic patterning from sciMAP-ATAC single cells and from cells which are not spatially resolved but co-cluster.

□ SORA: Using Apache Spark on genome assembly for scalable overlap-graph reduction
>> https://humgenomics.biomedcentral.com/articles/10.1186/s40246-019-0227-1
Scalable Overlap-graph Reduction Algorithms (SORA). SORA is an algorithm package that performs string graph reduction algorithms by Apache Spark.
SORA efficiently compacts the number of edges on enormous graphing paths by adapting scalable features of graph processing libraries provided by Apache Spark, GraphX and GraphFrames.

□ Denoising of Aligned Genomic Data
>> https://www.nature.com/articles/s41598-019-51418-z
the quality score updating step of SAMDUDE is crucial to improving variant calling outcome, and that denoising reads alone is insufficient for higher quality of variant calls.
□ PhISCS: a combinatorial approach for subperfect tumor phylogeny reconstruction via integrative use of single-cell and bulk sequencing data
>> https://genome.cshlp.org/content/early/2019/10/18/gr.234435.118.abstract
the optimal subperfect phylogeny problem which asks to integrate SCS data with matching bulk sequencing data by minimizing a linear combination of potential false negatives, false positives among mutation calls, and the number of mutations that violate ISA - infinite sites assumption.

□ Analysis of single-cell gene pair coexpression landscapes by stochastic kinetic modeling reveals gene-pair interactions in development
>> https://www.biorxiv.org/content/biorxiv/early/2019/10/23/815878.full.pdf
From the computed landscapes, obtaining a low-dimensional “shape-space” describing distinct types of coexpression patterns. a high-computational-throughput approach to stochastic modeling of gene-pair coexpression landscapes, based on numerical solution of gene network Master Equations.
□ Tomcat: A sparse occupancy model to quantify species interactions in time and space
>> https://www.biorxiv.org/content/biorxiv/early/2019/10/23/815027.full.pdf
a Bayesian Time-dependent Occupancy Model for Camera Trap data (Tomcat), suited to estimate relative event densities in space and time.
Enforcing sparsity on the vector of coefficients avoids the problem of over-fitting in case the number of camera trap locations is smaller or on the same order as the number of environmental coefficients.
□ SINC: a scale-invariant deep-neural-network classifier for bulk and single-cell RNA-seq data
>> https://academic.oup.com/bioinformatics/advance-article-abstract/doi/10.1093/bioinformatics/btz801/5606713
an analysis method “scale-invariant” (SI) if it gives the same result under different estimates of sequencing depth and hence can use the original count data without scaling.
SINC, a deep-neural-network based SI classifier. On nine bulk and single-cell datasets, the classification accuracy of SINC is better than or competitive to the best of other classifiers. SINC is more reliable on data where proper sequencing depth is hard to determine.
Equipped with the modern training techniques such as data augmentation, batch normalization, dropout layers, and ReLU activation functions, SINC should have no difficulty in accommodating deeper networks.
□ bWGR: Bayesian Whole-Genome Regression
>> https://academic.oup.com/bioinformatics/advance-article-abstract/doi/10.1093/bioinformatics/btz794/5606714
bWGR implements a series of methods referred to as the Bayesian alphabet under the traditional Gibbs sampling and optimized Expectation-Maximization.
The bWGR offers a compendium of Bayesian methods with various priors available, allowing users to predict complex traits with different genetic architectures.

□ IMAGE: high-powered detection of genetic effects on DNA methylation using integrated methylation QTL mapping and allele-specific analysis
>> https://genomebiology.biomedcentral.com/articles/10.1186/s13059-019-1813-1
IMAGE (Integrative Methylation Association with GEnotypes), a new statistical method for mQTL mapping in bisulfite sequencing studies that both accounts for the count-based nature of the data and takes advantage of ASM analysis to improve power.
IMAGE uses a penalized quasi-likelihood (PQL) approximation-based algorithm to facilitate scalable model inference.

□ Accuracy, Robustness and Scalability of Dimensionality Reduction Methods for Single Cell RNAseq Analysis
>> https://www.biorxiv.org/content/biorxiv/early/2019/10/23/641142.full.pdf
With the extracted low-dimensional components, we applied two commonly used trajectory inference methods: Slingshot and Monocle3. Slingshot is a clustering dependent trajectory inference method, which requires additional cell label information.
therefore first using either k-means clustering algorithm, hierarchical clustering or Louvain method to obtain cell type labels, where the number of cell types in the clustering was set to be the known truth.

□ Controllability of heterogeneous multiagent systems with two-time-scale feature
>> https://aip.scitation.org/doi/full/10.1063/1.5090319
investigating the controllability problems for heterogeneous multiagent systems (MASs) with two-time-scale feature under fixed topology.
split the heterogeneous two-time-scale MASs into slow and fast subsystems to eliminate the singular perturbation parameter.
Subsequently, according to the matrix theory and the graph theory, proposing some necessary/sufficient criteria for the controllability of the heterogeneous two-time-scale MASs.

□ SwiftOrtho: A fast, memory-efficient, multiple genome orthology classifier
>> https://academic.oup.com/gigascience/article/8/10/giz118/5606727
SwiftOrtho, a new graph-based orthology analysis tool, which is optimized for speed and memory usage when applied to large-scale data, and identifies orthologs, paralogs and co-orthologs for genomes.
SwiftOrtho uses long k-mers to speed up homology search, while using a reduced amino acid alphabet and spaced seeds to compensate for the loss of sensitivity due to long k-mers.
Swiftortho uses an affinity propagation algorithm to reduce the memory usage when clustering large-scale orthology relationships into orthologous groups.

□ Lasso-TopX: Machine Learning Approaches Identify Genes Containing Spatial Information from Single-Cell Transcriptomics Data
>> https://www.biorxiv.org/content/biorxiv/early/2019/10/25/818393.full.pdf
Lasso-TopX allows a user to define a specific number of features they are interested in and the Neural Network approach utilizes weak supervision for linear regression to accommodate for uncertain or probabilistic training labels.
This methods were able to identify non-insitu genes that also contain spatial information. the Lasso-TopX and NN approaches both reported similar genes.
□ Kalign 3: multiple sequence alignment of large data sets
>> https://academic.oup.com/bioinformatics/advance-article/doi/10.1093/bioinformatics/btz795/5607735
Kalign now uses a SIMD accelerated version of the bit-parallel Gene Myers algorithm to estimate pariwise distances, adopts a sequence embedding strategy and the bi-secting K-means algorithm to rapidly construct guide trees for thousands of sequences.
the original Kalign program uses the unweighted pair group method with arithmetic mean (UPGMA) algorithm to construct a guide tree resulting in quadratic time complexity.

□ GenGraph: a python module for the simple generation and manipulation of genome graphs
>> https://bmcbioinformatics.biomedcentral.com/articles/10.1186/s12859-019-3115-8
A GenGraph graph is a directed sequence graph, where the individual genomes are encoded as walks within the graph along a labeled path.
GenGraph is able to create a genome graph using multiple whole genomes & existing multiple sequence alignment tools. The final NetworkX graph objects created by GenGraph may be exported as GraphML, XML, or as a serialised object, though various other formats or future algorithm.
□ ROGUE: an entropy-based universal metric for assessing the purity of single cell population
>> https://www.biorxiv.org/content/biorxiv/early/2019/10/27/819581.full.pdf
The ROGUE metric is generalizable across datasets, and enables accurate, sensitive and robust assessment of cluster purity on a wide range of simulated and real datasets.
Since ROGUE can provide direct purity quantification of a single cluster and is independent of methods used for normalization, dimensionality reduction and clustering, it could also be applied to guide the splitting (re-clustering) or merging of specific clusters in unsupervised clustering analyses.

□ DeepCAGE: Incorporating gene expression in genome-wide prediction of chromatin accessibility via deep learning
>> https://www.biorxiv.org/content/biorxiv/early/2019/10/28/610642.full.pdf
DeepCAGE, a deep learning framework that integrates a densely connected convolutional neural network to automatically extract DNA sequence signatures, capture TF binding motifs and implicate driving activity of transcription factors.
DeepCAGE takes both the DNA sequence information and TFs gene expression data into consideration and adopts the architecture of densely connected convolutional neural network which has been experimentally proved to alleviate vanishing-gradient problem.
□ BLMRM: Modeling allele-specific expression at the gene and SNP levels simultaneously by a Bayesian logistic mixed regression model
>> https://bmcbioinformatics.biomedcentral.com/articles/10.1186/s12859-019-3141-6
modeling the logistic transformation of the probability parameter in the binomial model as a linear combination of the gene effect, single nucleotide polymor-phism (SNP) effect, and biological replicate effect.
To compute posterior probabilities, combining the empirical Bayes method and Laplace approach to approximate integrations, leading to substantially reduced computational power requirements compared to MCMC.
□ Parallel and scalable workflow for the analysis of Oxford Nanopore direct RNA sequencing datasets
>> https://www.biorxiv.org/content/biorxiv/early/2019/10/28/818336.full.pdf
A direct RNA sequencing run produced by MinION or GridION devices, which typically comprises about 1M reads, takes ~2 hours to analyze on a cluster using 100 nodes, each one with 8 CPUs, and ~1 hour or less on a single GPU.

□ Network inference with ensembles of bi-clustering trees
>> https://bmcbioinformatics.biomedcentral.com/articles/10.1186/s12859-019-3104-y
Bi-clustering trees outperform existing tree-based strategies as well as machine learning methods based on other algorithms.
Network inference as a multi-label classification task, integrating background information from both item sets in the same network framework. The method proposed here is a global approach, extending multi-output decision tree learning to the interaction data framework.

□ annonex2embl: automatic preparation of annotated DNA sequences for bulk submissions to ENA
>> https://www.biorxiv.org/content/biorxiv/early/2019/10/28/820480.full.pdf
Based on the aggregate of all input information, annonex2embl reads and parses the aligned DNA sequences and their annotations from the NEXUS file.

□ deepMc: Deep Matrix Completion for Imputation of Single-Cell RNA-seq Data
>> https://www.liebertpub.com/doi/10.1089/cmb.2019.0278
a deep matrix factorization-based method, deepMc, to impute missing values in gene expression data. For the deep architecture of this approach, drawing the motivation from great success of deep learning in solving various machine learning problems.
deepMc presents the potency of deepImpute through rigorous experimentations including clustering accuracy, differential genes prediction and cell type separability, validating biologically relevant.

□ RNN-IMP: A Recurrent Neural Network Based Method for Genotype Imputation on Phased Genotype Data
>> https://www.biorxiv.org/content/biorxiv/early/2019/10/30/821504.full.pdf
RNN-IMP is a recurrent neural network based genotype imputation program, and haplotype data of a large number of individuals are encoded as its model parameters through the training step, which can be shared publicly due to the difficulty in restoring genotype data at the individual-level.
RNN-IMP considered binary vectors indicating alleles in the reference panel for the feature information of variants in input data, which are converted to the binary vectors to make input feature vectors of bidirectional RNN using kernel principal component analysis.
RNN-IMP takes phased genotypes in HAP/LEGEND format as input data and outputs imputation results in Oxford GEN format.
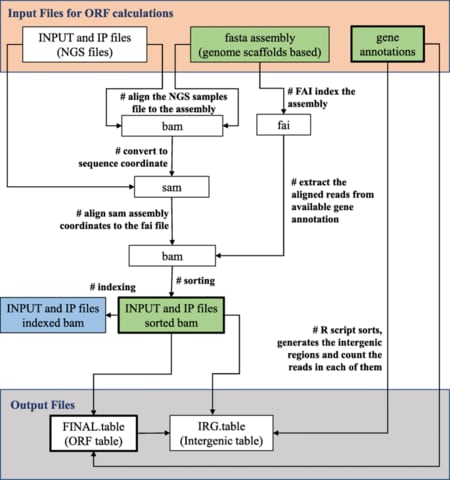
□ RACS: rapid analysis of ChIP-Seq data for contig based genomes
>> https://bmcbioinformatics.biomedcentral.com/articles/10.1186/s12859-019-3100-2
RACS is particularly useful for ChIP-Seq in organisms with contig-based genomes that have poor gene annotation to aid protein function discovery.

□ SECNVs: A Simulator of Copy Number Variants and Whole-Exome Sequences from Reference Genomes
>> https://www.biorxiv.org/content/biorxiv/early/2019/10/30/824128.full.pdf
SECNVs simulates test genomes and target regions to overcome some of the limitations of other WGS CNV simulation tools, and is the first ready-to-use WES CNV simulator.
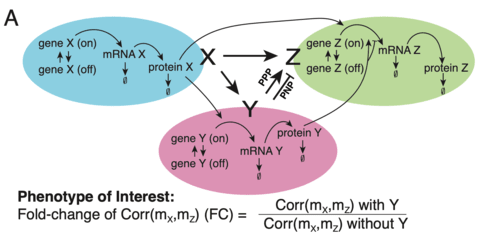
□ Machine learning of stochastic gene network phenotypes
>> https://www.biorxiv.org/content/biorxiv/early/2019/10/31/825943.full.pdf
a predictive ML model, and can use it as a “phenomenological” solution of the SME to efficiently predict phenotypes from parameters without using computationally intensive simulations.
※コメント投稿者のブログIDはブログ作成者のみに通知されます