
One is the loneliest number.

□ scPADGRN: A preconditioned ADMM approach for reconstructing dynamic gene regulatory network using single-cell RNA sequencing data
>> https://www.biorxiv.org/content/biorxiv/early/2019/10/09/799189.full.pdf
a quantity called Differentiation Genes’ Interaction Enrichment (DGIE) to quantify the changes in the interactions of a certain set of genes in a DGRN.
scPADGRN clusters scRNA-seq data for different cells based on cell pseudotrajectories to convert single-cell-level data into cluster-level data. The second step is to cluster the cells on the pseudotime line into clusters on the real timeline.
□ Cassiopeia: Inference of Single-Cell Phylogenies from Lineage Tracing Data
>> https://www.biorxiv.org/content/biorxiv/early/2019/10/10/800078.full.pdf
Cassiopeia - a suite of scalable and theoretically grounded maximum parsimony approaches for tree reconstruction. Cassiopeia provides a simulation framework for evaluating algorithms and exploring lineage tracer design principles.
Cassiopeia’s framework consists of three modules: a greedy algorithm - Cassiopeia-Greedy, which attempts to construct trees efficiently based on mutations that occurred earliest in the experiment;
a near-optimal algorithm that attempts to find the most parsimonious solution using a Steiner-Tree approach - Cassiopeia-ILP;
and a hybrid algorithm - Cassiopeia-Hybrid - that blends the scalability of the greedy algorithm and the exactness of the Steiner-Tree approach to support massive single-cell lineage tracing phylogeny reconstruction.

□ Efficient chromosome-scale haplotype-resolved assembly of human genomes
>> https://www.biorxiv.org/content/biorxiv/early/2019/10/18/810341.full.pdf
a method that leverages long accurate reads and long-range conformation data for single individuals to generate chromosome-scale phased assembly within a day.
In comparison to other single-sample phased assembly algorithms, this is the only method capable of chromosome-long phasing.
A potential solution is to retain heterozygous events in the initial assembly graph and to scaffold and dissect these events later to generate a phased assembly.

□ Fast and precise single-cell data analysis using hierarchical autoencoder
>> https://www.biorxiv.org/content/biorxiv/early/2019/10/10/799817.full.pdf
a non-negative kernel autoencoder that provides a non-negative, part-based representation of the data. Based on the weight distribution of the encoder, scDHA removes genes or components that have insignificant contribution to the representation.
a Stacked Bayesian Self-learning Network that is built upon the Variational Autoencoder to project the data onto a low dimensional space.
The single-cell Decomposition using Hierarchical Autoencoder conducts cell segregation through unsupervised learning, dimension reduction and visualization, cell classification, and time-trajectory inference.
□ GPseudoClust: deconvolution of shared pseudo-profiles at single-cell resolution
>> https://academic.oup.com/bioinformatics/advance-article/doi/10.1093/bioinformatics/btz778/5586890
GPseudoClust: deconvolution of shared pseudo-trajectories at single-cell resolution. GPseudoClust is a novel approach that jointly infers pseudotemporal ordering and gene clusters, and quantifies the uncertainty in both.
GPseudoClust combines a recent method for pseudotime inference with nonparametric Bayesian clustering using Dirichlet process mixtures of hierarchical GPs, efficient MCMC sampling, and novel subsampling strategies which aid computation.

□ LEMMA: Gene-environment interactions using a Bayesian whole genome regression model
>> https://www.biorxiv.org/content/biorxiv/early/2019/10/09/797829.full.pdf
a new method called Linear Environment Mixed Model Analysis (LEMMA) which aims to combine the advantages of WGR and modelling GxE with multiple environments.
Instead of assuming that the GxE effect over multiple environments is independent at each variant, as StructLMM does, we learn an environmental score (ES) which is a single linear combination of environmental variables, that has a common role in interaction effects genome wide.

□ t-SNE transformation: a normalization method for local features of single-cell RNA-seq data
>> https://www.biorxiv.org/content/biorxiv/early/2019/10/09/799288.full.pdf
a method called t-SNE transformation to replace log transformation. When the cluster number was changed, t-SNE transformation was steadier than log transformation.
t-SNE transformation is an alternative normalization for detecting local features, especially interests arouse in cell types with rare populations or highly-variated but independently expressed genes.
t-SNE is considered as a dimension reduction method, the dimension of outcomes is not restricted to be lower than that of original space. Therefore, t-SNE transformation is defined to refer mapping data to space with the same dimension of the original space by the rule of t-SNE.
□ SPARSim Single Cell: a count data simulator for scRNA-seq data
>> https://academic.oup.com/bioinformatics/advance-article-abstract/doi/10.1093/bioinformatics/btz752/5584234
SPARSim allows to generate count data that resemble real data in terms of count intensity, variability and sparsity. SPARSim simulated count matrices well resemble the distribution of zeros across different expression intensities observed in real count data.
SPARSim is a scRNA-seq count data simulator based on a Gamma-Multivariate Hypergeometric model.

□ Spectral Jaccard Similarity: A new approach to estimating pairwise sequence alignments
>> https://www.biorxiv.org/content/biorxiv/early/2019/10/10/800581.full.pdf
a min-hash-based approach for estimating alignment sizes called Spectral Jaccard Similarity which naturally accounts for an uneven k-mer distribution in the reads being compared. The Spectral Jaccard Similarity is computed by considering a min-hash collision matrix.
The leading left singular vector provides the Spectral Jaccard Similarity for each pair of reads. an approximation to the Spectral Jaccard Similarity that can be computed with a single matrix-vector product, instead of a full singular value decomposition.

□ TRACE: transcription factor footprinting using DNase I hypersensitivity data and DNA sequence
>> https://www.biorxiv.org/content/biorxiv/early/2019/10/10/801001.full.pdf
Trace is an unsupervised method that accurately annotates binding sites for specific TFs automatically with no requirement on pre-generated candidate binding sites or ChIP-seq training data.
Trace incorporates DNase-seq data and PWMs within a multivariate Hidden Markov Model (HMM) to detect footprint-like regions with matching motifs.

□ Trans-NanoSim characterizes and simulates nanopore RNA-seq data
>> https://www.biorxiv.org/content/biorxiv/early/2019/10/10/800110.full.pdf
Trans-NanoSim, the first tool that simulates reads with technical and transcriptome-specific features learnt from nanopore RNA-seq data.
benchmarking the performance of Trans-NanoSim against DeepSimulator by generating sets of synthetic reads, Trans-NanoSim shows the robustness in capturing the characteristics of nanopore cDNA and direct RNA reads.

□ S-conLSH: Alignment-free gapped mapping of noisy long reads
>> https://www.biorxiv.org/content/biorxiv/early/2019/10/10/801118.full.pdf
a new mapper called S-conLSH that uses Spaced context based Locality Sensitive Hashing. With multiple spaced patterns, S-conLSH facilitates a gapped mapping of noisy long reads to the corresponding target locations of a reference genome.
The spaced-context of a sequence is a substring formed by extracting the symbols corresponding to the ‘1’ positions in the pattern. S-conLSH provides alignment-free mappings of the SMRT reads to the reference genome.

□ Out of the abyss: Genome and metagenome mining reveals unexpected environmental distribution of abyssomicins
>> https://www.biorxiv.org/content/biorxiv/early/2019/10/10/789859.full.pdf
the environmental distribution and evolution of the abyssomicin BGC through the analysis of publicly available genomic and metagenomic data.
The results strongly support the potential of genome and metagenome mining as a key preliminary tool to inform bioprospecting strategies aiming at the identification of new bioactive compounds such as -but not restricted to- abyssomicins.
□ libbdsg: Optimized bidirected sequence graph implementations for graph genomics
>> https://github.com/vgteam/libbdsg
The main purpose of libbdsg is to provide high performance implementations of sequence graphs for graph-based pangenomics applications.
The repository contains three graph implementations with different performance tradeoffs: HashGraph: prioritizes speed, ODGI: balances speed and low memory usage, PackedGraph: prioritizes low memory usage.
□ raxmlGUI 2.0 beta: a graphical interface and toolkit for phylogenetic analyses using RAxML
>> https://www.biorxiv.org/content/biorxiv/early/2019/10/10/800912.full.pdf
raxmlGUI 2.0-beta, a complete rewrite of the GUI, which replaces raxmlGUI and seamlessly integrates RAxML binaries for all major operating systems providing an intuitive graphical front-end to set up and run phylogenetic analyses.
a sequence of three RAxML calls to infer the maximum likelihood tree through a user-defined number of independent searches; run a user-defined number of thorough non-parametric bootstrap replicates; and draw the bootstrap support values onto the maximum likelihood tree.
An important feature of raxmlGUI 2.0 is the automated concatenation and partitioning of alignments, which simplifies the analysis of multiple genes or combination of different data types, e.g. amino acids sequences and morphological data.

□ Shifting spaces: which disparity or dissimilarity metrics best summarise occupancy in multidimensional spaces?
>> https://www.biorxiv.org/content/biorxiv/early/2019/10/11/801571.full.pdf
no one metric describes all changes through a trait-space and the results from each metric are dependent on the characteristics of the space and the hypotheses.
Furthermore, because there can potentially be an infinite number of metrics, it would be impossible to propose clear generalities to space occupancy metrics behavior.
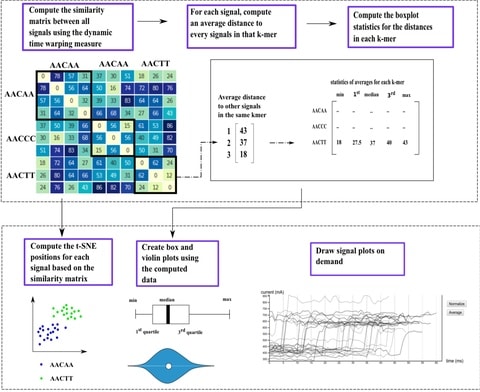
□ Sequoia: An interactive visual analytics platform for interpretation and feature extraction from nanopore sequencing datasets
>> https://www.biorxiv.org/content/biorxiv/early/2019/10/11/801811.full.pdf
Sequia accepts Fast5 files generated by the ONT and then, using the dynamic time warping similarity measure, displays the relative similarities between signals using the t-SNE algorithm.
Given that the signal lengths were largely related to the compression and expansion of signals during the dynamic time warping process, the dynamic time warping penalty was now set to 100, as opposed to 0.

□ MetaCell: analysis of single-cell RNA-seq data using K-nn graph partitions
>> https://genomebiology.biomedcentral.com/articles/10.1186/s13059-019-1812-2
A metacell (abbreviated MC) is in theory a group of scRNA-seq cell profiles that are statistically equivalent to samples derived from the same RNA pool.
The approach is somewhat similar to methods using mutual K-nn analysis to normalize batch effects, or more generally to approaches using symmetrization of the K-nn graph to facilitate dimensionality reduction.
MetaCell provides, especially as the size of single-cell atlases increases, an attractive universal first layer of analysis on top of which quantitative and dynamic analysis can be developed further.
□ Treerecs: an integrated phylogenetic tool, from sequences to reconciliations
>> https://www.biorxiv.org/content/biorxiv/early/2019/10/11/782946.full.pdf
Treerecs can compute the phylogenetic likelihood of a tree given a multiple sequence alignment, using the Phylogenetic Likelihood Library.
Treerecs is based on duplication-loss reconciliation, and simple to install and to use, fast, versatile, with a graphic output, and can be used along with methods for phylogenetic inference on multiple alignments like PLL and Seaview.

□ TriMap: Large-scale Dimensionality Reduction Using Triplets
>> https://arxiv.org/pdf/1910.00204v1.pdf
TriMap, a dimensionality reduction technique based on triplet constraints that preserves the global accuracy of the data better than the other commonly used methods such as t-SNE, LargeVis, and UMAP.
TriMap is particularly robust to the number of sampled triplet for constructing the embedding. This can be explained by the high amount of redundancy in the triplets. using large number of triplets can introduce an overhead and require larger number of iterations to converge.

□ DECA: scalable XHMM exome copy-number variant calling with ADAM and Apache Spark
>> https://bmcbioinformatics.biomedcentral.com/articles/10.1186/s12859-019-3108-7
DECA, a horizontally scalable implementation of XHMM using ADAM and Apache Spark. XHMM is not parallelized, although the user could partition the input files for specific steps themselves and invoke multiple instances of the XHMM executable.
DECA performed CNV discovery from the read-depth matrix in 2535 exomes in 9.3 min on a 16-core workstation (35.3× speedup vs. XHMM), 12.7 min using 10 executor cores on a Spark cluster (18.8× speedup vs. XHMM), and 9.8 min using 32 executor cores on AWS Elastic MapReduce.

□ Imputing missing RNA-seq data from DNA methylation by using transfer learning based-deep neural network https://www.biorxiv.org/content/biorxiv/early/2019/10/13/803692.full.pdf
TDimpute method is designed to impute multi-omics dataset where large, contiguous blocks of features go missing at once. TDimpute perform missing gene expression imputation by building a highly nonlinear mapping from DNA methylation data to gene expression data.
TDimpute method is capable of processing large-scale multi-omics dataset including hundreds of thousands of features, while TOBMI and SVD suffer poor scalability due to the computational complexity of distance matrix computation and singular value decomposition operations.
□ Imputing missing RNA-seq data from DNA methylation by using transfer learning based-deep neural network
>> https://www.biorxiv.org/content/biorxiv/early/2019/10/13/803692.full.pdf
TDimpute method is designed to impute multi-omics dataset where large, contiguous blocks of features go missing at once. TDimpute perform missing gene expression imputation by building a highly nonlinear mapping from DNA methylation data to gene expression data.
TDimpute method is capable of processing large-scale multi-omics dataset including hundreds of thousands of features, while TOBMI and SVD suffer poor scalability due to the computational complexity of distance matrix computation and singular value decomposition operations.
□ Hierarchical Modeling of Linkage Disequilibrum: Genetic Structure and Spatial Relations
>> https://www.cell.com/ajhg/fulltext/S0002-9297(07)60544-8
a framework for hierarchical modeling of Linkage disequilibrium (HLD), a simulation study assessing the performance of HLD under various scenarios, and an application of HLD to existing data.
This approach incorporates higher-level information on genetic structure and the spatial relations of markers along a chromosomal region to improve the localization of disease-causing genes.

□ DDIA: data dependent-independent acquisition proteomics - DDA and DIA in a single LC-MS/MS run
>> https://www.biorxiv.org/content/biorxiv/early/2019/10/13/802231.full.pdf
Deep learning based LC-MS/MS property prediction tools, developed previously can be used repeatedly to produce spectral libraries facilitating DIA scan extraction.
The machine learning field has developed many strategies to deal with the problem of “finding a needle in a haystack”, generally called anomaly detection.

□ BioSfer: Exploiting Transfer Learning for the Reconstruction of the Human Gene Regulatory Network
>> https://academic.oup.com/bioinformatics/advance-article/doi/10.1093/bioinformatics/btz781/5586888
the transfer learning method BioSfer, which is able to exploit the knowledge about a (reconstructed) source gene regulatory network to improve the reconstruction of a target regulatory network.
BioSfer is natively able to work in the Positive-Unlabeled setting, where no negative example is available, by fruitfully exploiting a (possibly large) set of unlabeled examples.
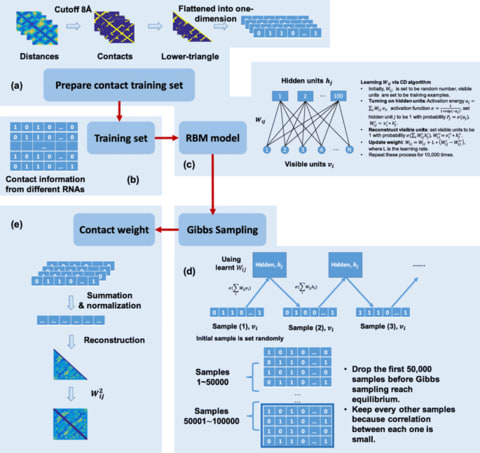
□ DIRECT: RNA contact predictions by integrating structural patterns
>> https://bmcbioinformatics.biomedcentral.com/articles/10.1186/s12859-019-3099-4
DIRECT outperforms the state-of-the-art DCA predictions for long-range contacts and loop-loop contacts.
DIRECT (Direct Information REweighted by Contact Templates) incorporates a Restricted Boltzmann Machine (RBM) to augment the information on sequence co-variations with structural features in contact inference.
□ An improved encoding of genetic variation in a Burrows-Wheeler transform
>> https://academic.oup.com/bioinformatics/advance-article-abstract/doi/10.1093/bioinformatics/btz782/5587763
a method that is able to encode many kinds of genetic variation (SNPs, MNPs, indels, duplications, transpositions, inversions, and copy-number variation) in a BWT.
The additional symbol marks variant sites in a chromosome and delimits multiple variants, which are added at the end of the ’marked chromosome’.
the backward search algorithm, which is used in BWT-based read mappers, can be modified in such a way that it can cope with the genetic variation encoded in the BWT.

□ doepipeline: a systematic approach to optimizing multi-level and multi-step data processing workflows
>> https://bmcbioinformatics.biomedcentral.com/articles/10.1186/s12859-019-3091-z
Optimal parameter settings are first approximated in a screening phase using a subset design that efficiently spans the entire search space, then optimized in the subsequent phase using response surface designs and OLS modeling.
Doepipeline was used to optimize parameters in four use cases; de-novo assembly, scaffolding of a fragmented genome assembly, k-mer taxonomic classification of Oxford Nanopore Technologies MinION reads, and genetic variant calling.
□ A-Star: an Argonaute-directed System for Rare SNV Enrichment and Detection
>> https://www.biorxiv.org/content/biorxiv/early/2019/10/15/803841.full.pdf
a simple but efficient single-tube PCR system, referred to as A-Star (Ago-directed specific target enrichment) specifically cleave wild-type sequences during the DNA denaturation step, leading to progressive and rapid (~ 3 h) enrichment of scarce SNV-containing alleles.
And further validated the A-Star system by multiplex detection of three rare oncogenic genes in complex genetic backgrounds. To address the precise cleavage of tDNA, the crucial concern in A-Star involves the design and selection of the gDNAs for the discrimination of SNVs.

□ GAIA: an integrated metagenomics suite
>> https://www.biorxiv.org/content/biorxiv/early/2019/10/15/804690.full.pdf
on average GAIA obtained the highest scores at species level for WGS metagenomics and it also obtained excellent scores for amplicon sequencing.
for shotgun metagenomics, GAIA obtained the highest F-measures at species level above all tested pipelines (CLARK, Kraken, LMAT, BlastMegan, DiamondMegan and NBC). For 16S metagenomics, GAIA also obtained excellent F-measures comparable to QIIME.
□ T-Gene: Improved target gene prediction
>> https://www.biorxiv.org/content/biorxiv/early/2019/10/15/803221.full.pdf
T-Gene algorithm can be used to predict which genes are most likely to be regulated by a TF, and which of the TF’s binding sites are most likely involved in regulating particular genes.
T-Gene calculates a novel score that combines distance and histone/expression correlation, and this score accurately predicts when a regulatory element bound by a TF is in contact with a gene’s promoter, achieving median positive predictive value (PPV) above 50%.
T-Gene incorporates a heuristic that reduces false positives by increasing the influence of link length on the score of links where the transcript has very low expression across the tissue panel, rather than omitting such links entirely as CisMapper does.
□ Genetic design automation for autonomous formation of multicellular shapes from a single cell progenitor
>> https://www.biorxiv.org/content/biorxiv/early/2019/10/16/807107.full.pdf
a computer-aided design approach for designing recombinase-based genetic circuits for controlling the formation of multi-cellular masses into arbitrary shapes in human cells.
solving the problem with two alternative type of applied algorithms: a Maximum Leaf Spanning Tree (MLST) algorithm and a Minimum Spanning Tree (MST) algorithm.

□ DeepImpute: an accurate, fast, and scalable deep neural network method to impute single-cell RNA-seq data
>> https://genomebiology.biomedcentral.com/articles/10.1186/s13059-019-1837-6
DeepImpute, a deep neural network-based imputation algorithm that uses dropout layers and loss functions to learn patterns in the data, allowing for accurate imputation.
DeepImpute performs better than the six other recently published imputation methods mentioned above (MAGIC, DrImpute, ScImpute, SAVER, VIPER, and DCA).
DeepImpute is a deep neural network model that imputes genes in a divide-and-conquer approach, by constructing multiple sub-neural networks.
□ Peregrine: Fast Genome Assembler Using SHIMMER Index
>> https://github.com/cschin/Peregrine
Peregrine is a fast genome assembler for accurate long reads (length > 10kb, accuraccy > 99%). It can assemble a human genome from 30x reads within 20 cpu hours from reads to polished consensus.
Peregrine uses Sparse HIereachical MimiMizER (SHIMMER) for fast read-to-read overlaping without quadratic comparisions used in other OLC assemblers. Currently, the assembly graph process is more or less identical to the approaches used in the FALCON assembler.

□ Unifying single-cell annotations based on the Cell Ontology:
>> https://www.biorxiv.org/content/biorxiv/early/2019/10/20/810234.full.pdf
OnClass, an algorithm and accompanying software for automatically classifying cells into cell types represented by a controlled vocabulary derived from the Cell Ontology.
OnClass constructs a network of cell types based on the hierarchical “is_a” relationship in the Cell Ontology and embeds this network into a low-dimensional space that preserves network topology.
□ Titan: DNAnexus Titan powers the future of genomics research and clinical pipelines with trusted, high-performance data analysis solutions
>> https://www.dnanexus.com/product-overview/titan
DNAnexus Titan removes the heavy lift associated with scaling cloud-based NGS analysis by solving infrastructure challenges and increasing efficiencies.
Titan extends with CWL, WDL, or dockerized workflows. Automatically track data provenance to ensure reproducibility. Eliminate delays and accelerate turnaround time with exceptional uptime and powerful compute capacity, including parallelizable execution.
□ Whole-Genome Alignment
>> https://link.springer.com/protocol/10.1007/978-1-4939-9074-0_4
Whole-genome alignment (WGA) is the prediction of evolutionary relationships at the nucleotide level between two or more genomes.
WGA combines aspects of both colinear sequence alignment and gene orthology prediction and is typically more challenging to address than either of these tasks due to the size and complexity of whole genomes.
※コメント投稿者のブログIDはブログ作成者のみに通知されます