
□ MaxFuse: Integration of spatial and single-cell data across modalities with weakly linked features
>> https://www.nature.com/articles/s41587-023-01935-0
MaxFuse (matching X-modality via fuzzy smoothed embedding), a cross-modal data integration method that, through iterative coembedding, data smoothing and cell matching, uses all information in each modality to obtain high-quality integration even when features are weakly linked.
MaxFuse is modality-agnostic. MaxFuse computes distances between all cross-modal cell pairs based on the smoothed, linked features and applies linear assignment on the cross-modal pairwise distances of the fuzzy-smoothed joint embedding coordinates.

□ Autometa 2: A versatile tool for recovering genomes from highly-complex metagenomic communities
>> https://www.biorxiv.org/content/10.1101/2023.09.01.555939v1
Autometa first performs pre-processing tasks where assembled contiguous sequences (contigs) are filtered by length and taxon. The latter process assigns contigs to kingdom-level taxonomies, effectively separating eukaryotic host-associated genomes from prokaryotic symbionts.
Contigs are recursively binned using nucleotide composition and read coverage, with successive rounds first splitting the remaining contigs into groups from less to more specific canonical ranks (i.e. kingdom, phylum, class, order, family, genus, species).
Autometa attempts to recruit any remaining unclustered sequences into one of the recovered putative metagenome- assembled genomes (MAGs) through classification by a decision tree classifier, or optionally, a random forest classifier.

□ EpiSegMix: A Flexible Distribution Hidden Markov Model with Duration Modeling for Chromatin State Discovery
>> https://www.biorxiv.org/content/10.1101/2023.09.07.556549v1
EpiSegMix first estimates the parameters of a hidden Markov model, where each state corresponds to a different combination of epigenetic modifications and thus represents a functional role, such as enhancer, transcription start site, active or silent gene.
The spatial relations are captured via the transition probabolities. After the parameter estimation, each region in the genome is annotated w/ the most likely chromatin state. The implementation allows to choose for each histone modification a different distributional assumption.

□ Xenomake: a pipeline for processing and sorting xenograft reads from spatial transcriptomic experiments
>> https://www.biorxiv.org/content/10.1101/2023.09.04.556109v1
Xenomake is a xenograft reads sorting and processing pipeline. It consists of the following steps: read tagging/trimming, alignment, annotation of genomic features, xenograft read sorting, subsetting bam, filtering multi mapping reads, and gene quantifications.
Xenomake contains a policy regarding handling reads classified as both and ambiguous by Xengsort. Xenomake differs from others in that it adopts a flexible strategy to resolve both/ambiguous categories to make reads in these categories usable, rather than removing them.
Xenomake uses the genomic location (exonic, intronic, intergenic, or pseudogene) to determine the best aligned location of a multimapping read. A multimapper favors the exonic alignment over intergenic, pseudogenic, and any other secondary alignments.

□ Multimodal learning of noncoding variant effects using genome sequence and chromatin structure
>> https://academic.oup.com/bioinformatics/advance-article/doi/10.1093/bioinformatics/btad541/7260506
A multimodal deep learning scheme that incorporates both data of 1D genome sequence and 3D chromatin structure for predicting noncoding variant effects.
Specifically, they have integrated convolutional and recurrent neural networks for sequence embedding and graph neural networks for structure embedding despite the resolution gap between the two types of data, while utilizing recent DNA language models.
Numerical results show that our models outperform competing sequence-only models in predicting epigenetic profiles and their use of long-range interactions complement sequence-only models in extracting regulatory motifs.
They prove to be excellent predictors for noncoding variant effects in gene expression and pathogenicity, whether in unsupervised “zero-shot” learning or supervised “few-shot” learning.

□ PFGM++: Unlocking the Potential of Physics-Inspired Generative Models
>> https://arxiv.org/abs/2302.04265
PFGM++ unifies diffusion models and Poisson Flow Generative Models. These models realize generative trajectories for N dimensional data by embedding paths in N+D dimensional space while still controlling the progression with a simple scalar norm of the D additional variables.
PFGM++ models reduce to PFGM when D=1 and to diffusion models when D→∞. present an align-after the phase alignment. PFGM++ uses an alignment method that enables a "zero-shot" transfer of hyper-parameters across different Ds.

□ GWAS of random glucose in 476,326 individuals provide insights into diabetes pathophysiology, complications and treatment stratification
>> https://www.nature.com/articles/s41588-023-01462-3
While random glucose (RG) is inherently more variable than standardized measures, they reasoned that, across a very large number of individuals, it gives a more comprehensive representation of complex glucoregulatory processes occurring in different organ systems.
In the near future, larger well-phenotyped datasets will enable high-dimensional GWAS investigations, disentangling the role of diet composition, physical activity and lifestyle on RG level variability in relation to genetic effects.

□ phyloGAN: Phylogenetic inference using Generative Adversarial Networks
>> https://academic.oup.com/bioinformatics/advance-article/doi/10.1093/bioinformatics/btad543/7260504
phyloGAN is a Generative Adversarial Network (GAN) that infers phylognetic relationships. phyloGAN takes as input a concatenated alignments, or a set of gene alignments, and then infers a phylogenetic tree either considering or ignoring gene tree heterogeneity.
phyloGAN heuristically explores phylogenetic tree space to find a tree topology that produces generated data that are similar to observed data. The generator generates a tree topology and branch lengths, which are used as input into an evolutionary simulator (AliSim).
At each iteration, new topologies are proposed using nearest neighbor interchange (NNI) and subtree pruning and regrafting (SPR). The discriminator is a CNN trained to differentiate real and generated data.

□ CoLA: Exploiting Compositional Structure for Automatic and Efficient Numerical Linear Algebra
>> https://arxiv.org/abs/2309.03060
CoLA (Compositional Linear Algebra) combines a linear operator abstraction with compositional dispatch rules. CoLA automatically constructs memory and runtime efficient numerical algorithms.
CoLA can accelerate many algebraic operations, while making it easy to prototype matrix structures and algorithms, providing an appealing drop-in tool for virtually any computational effort that requires linear algebra.

□ evopython: a Python package for feature-focused, comparative genomic data exploration
>> https://www.biorxiv.org/content/10.1101/2023.09.02.556042v1
evopython is a modular, object-oriented Python package, specifically designed for parsing features at genome-scale and resolving their alignments from whole-genome alignment data.
The fundamental capabilities of evopython are encapsulated within two key class-level functionalities: Parser and Resolver. The Parser class provides a dictionary-like interface for interacting with feature-storing formats, such as TF or BED.
The Resolver class then resolves these features from within the context of the whole-genome alignment. It performs the task of mapping the features onto the alignment and returns a nested dictionary representation that reflects the alignment structure.

□ ChromGene: gene-based modeling of epigenomic data
>> https://genomebiology.biomedcentral.com/articles/10.1186/s13059-023-03041-5
ChromGene models the set of epigenomic data across genes with a mixture of Hidden Markov Models. The set of epigenomic data for each gene, along with a flanking region at each end, is binarized at fixed-width bins, indicating observations of each epigenomic mark.
ChromGene does not directly model gene position information. The prior probability that a gene belongs to a specific mixture component, that is, an individual HMM, corresponds to the sum of initial probabilities of the states of that component.

□ Regulatory Transposable Elements in the Encyclopedia of DNA Elements
>> https://www.biorxiv.org/content/10.1101/2023.09.05.556380v1
TE-derived cCREs are enriched for GWAS variants, albeit to a lesser extent than non-TE cCREs. While this could indicate that TEs are less likely to be physiologically relevant, it could also reflect technical shortcomings associated with genotyping within TE sequences.
Genotyping arrays, which use short oligonucleotide probes to discern SNPs, are designed to avoid repetitive regions of the genome.
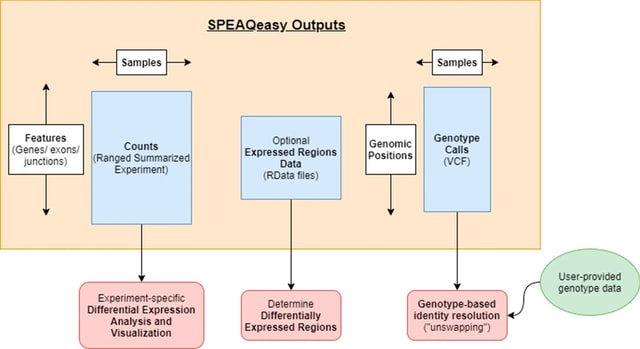
□ SPEAQeasy: a scalable pipeline for expression analysis and quantification for R/bioconductor-powered RNA-seq analyses
>> https://bmcbioinformatics.biomedcentral.com/articles/10.1186/s12859-021-04142-3
SPEAQeasy (a Scalable Pipeline for Expression Analysis and Quantification) ultimately generates RangedSummarizedExperiment R objects that are the foundation block for many Bioconductor R packages and the statistical methods they provide.
SPEAQeasy produces the information that coupled with DNA genotyping information can be used for detecting and fixing sample swaps, RNA-seq processing quality metrics that are helpful for statistically adjusting for quality differences across samples.

□ SpatialPrompt: spatially aware scalable and accurate tool for spot deconvolution and clustering in spatial transcriptomics
>> https://www.biorxiv.org/content/10.1101/2023.09.07.556641v1
SpatialPrompt, a spatially aware and scalable method for spot deconvolution as well as domain identification for spatial transcriptomics. SpatialPrompt integrates gene expression, spatial location, and scRNA-seq reference data to infer cell-type proportions of spatial spots accurately.
At the core, SpatialPrompt uses non-negative ridge regression and an iterative approach inspired by graph neural network (GNN) to capture the local microenvironment information in the spatial data.
Spatial Prompt takes spatial matrix with coordinate information and scRNA-seg matrix with cell type annotations as input for spot deconvolution and clustering.
The spatial spot simulation pipeline utilises scRNA-seq expression matrix and cell type annotations to generate simulated expression matrix with known cell type mixture.

□ A Quantitative Genetic Model of Background Selection in Humans
>> https://www.biorxiv.org/content/10.1101/2023.09.07.556762v1
A statistical method based on a quantitative genetics view of linked selection, that models how polygenic additive fitness variance distributed along the genome increases the rate of stochastic allele frequency change.
By jointly predicting the equilibrium fitness variance and substitution rate due to both strong and weakly deleterious mutations, they estimate the distribution of fitness effects (DFE) and mutation rate across three geographically distinct human samples.
While the model can accommodate weaker selection, they find evidence of strong selection operating similarly across all human samples. Although the model fits better than previous models, substitution rates of the most constrained sites disagree w/ observed divergence levels.

□ An Extensive Benchmark Study on Biomedical Text Generation and Mining with ChatGPT
>> https://academic.oup.com/bioinformatics/advance-article/doi/10.1093/bioinformatics/btad557/7264174
Typical NLP tasks like named entity recognization, relation extraction, sentence similarity, question and answering, and document classification are included. Overall, ChatGPT got a BLURB score of 58.50 while the state-of-the-art model had a score of 84.30.
Among all types of task, QA task is the only type of task that ChatGPT is comparative to the baselines. In this case, ChatGPT (82.5) outperforms PubMedBERT (71.7) and BioLinkBERT-Base (80.8) and is very close to the BioLinkBERT-Large (83.5).

□ Nicholas Larus-Stone
>> https://sphinxbio.com/post/introducing-sphinx
🧬🛠 Introducing @sphinx_bio: Empowering Scientists to Make Better Decisions, Faster 🛠🧬
"What is #techbio apart from an anagram of #biotech?"
Read on below or see the full post here: sphinxbio.com/post/introduci…
I’m excited to share more about our vision for Sphinx. 👩🔬👨💻

□ Trackplot: A flexible toolkit for combinatorial analysis of genomic data
>> https://journals.plos.org/ploscompbiol/article?id=10.1371/journal.pcbi.1011477
Trackplot, a comprehensive tool that delivers high-quality plots via a programmable and interactive web-based platform.
Trackplot seamlessly integrates diverse data sources and utilizes a multi-threaded process, enabling users to explore genomic signal in large-scale sequencing datasets.

□ COLLAGENE enables privacy-aware federated and collaborative genomic data analysis
>> https://genomebiology.biomedcentral.com/articles/10.1186/s13059-023-03039-z
COLLAGENE integrates components of MPC, HE, and matrix masking that is motivated by matrix-level differential privacy for performing complex operations (e.g., matrix inversion) efficiently while preserving privacy.
COLLAGENE provides ready-to-run implementations for encryption, collective decryption, matrix masking, a suite of secure matrix arithmetic operations, and network file input/output tools for sharing encrypted intermediate datasets among collaborating sites.

□ scDECAF: Identification of cell types, states and programs by learning gene set representations
>> https://www.biorxiv.org/content/10.1101/2023.09.08.556842v1
scDECAF (Single-cell disentanglement by canonical factors) enables reference-free automated annotation of cells with either discrete labels, such as cell types and states, or continuous phenotype scores for gene expression programs.
scDECAF can learn disentangled representations of gene expression profiles and select the most relevant subset of gene programs among a collection of gene sets. scDECAF constructs a shared lower-dimensional space b/n binarised gene lists and unlabelled gene expression profiles.
scDECAF provides vector representations of gene sets and gene expression profiles while simultaneously maximizing the correlation between the two. The association between individual cells and phenotpe is determined based on the similarity of their representations in CCA space.

□ DelSIEVE: joint inference of single-nucleotide variants, somatic deletions, and cell phylogeny from single-cell DNA sequencing data
>> https://www.biorxiv.org/content/10.1101/2023.09.09.556903v1
DeISIEVE (somatic Deletions enabled SIngle-cell EVolution Explorer), a statistical phylogenetic model that includes all features of SIEVE, namely correcting branch lengths of the cell phylogeny for the acquisition bias, incorporating a trunk to model the establishment of the tumor clone.
DeISIEVE employs a Dirichlet-multinomial distribution to model the raw read counts for all nucleotides, as well as modeling the sequencing coverage using a negative binomial distribution, and extends them with the more versatile capacity of calling somatic deletions.

□ MUSTANG: MUlti-sample Spatial Transcriptomics data ANalysis with cross-sample transcriptional similarity Guidance
>> https://www.biorxiv.org/content/10.1101/2023.09.08.556895v1
MUSTANG (MUlti-sample Spatial Transcriptomics data ANalysis with cross-sample transcriptional similarity Guidance) simultaneousIy derives the spot cellular deconvolution of multiple tissue samples without the need for reference cell type expression profiles.
MUSTANG adjusts for potential batch effects as crucial multi-sample experiments considerations to enable cross-sample transcriptional information sharing to aid in parameter estimation.
MUSTANG is designed based on the assumption that the same or similar cell types exhibit consistent gene expression profiles across samples. MUSTANG allows both intra-sample and inter-sample information sharing by introducing a new spot similarity graph.

□ BiocMAP: a Bioconductor-friendly, GPU-accelerated pipeline for bisulfite-sequencing data
>> https://bmcbioinformatics.biomedcentral.com/articles/10.1186/s12859-023-05461-3
The BiocMAP workflow consists of a set of two modules—alignment and extraction, which together process raw WGBS reads in FASTQ format into Bioconductor-friendly R objects containing DNA methylation proportions essentially as a cytosine-by-sample matrix.
The first BiocMAP module performs speedy alignment to a reference genome by Arioc, and requires GPU resources. Methylation extraction and remaining steps are performed in the second module, optionally on a different computing system where GPUs need not be available.
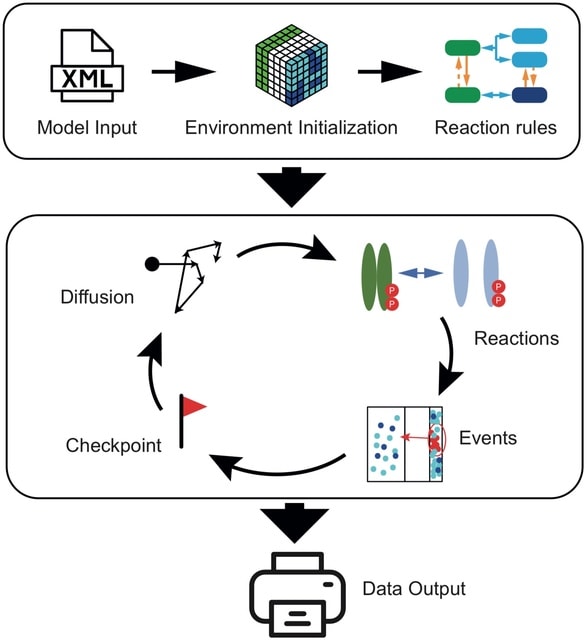
□ Cell4D: A general purpose spatial stochastic simulator for cellular pathways
>> https://www.biorxiv.org/content/10.1101/2023.09.10.557076v1
Cell4D is a C++-based graphical spatial stochatic cell simulator capable of simulating a wide variety of cellular pathways. Molecules are simulated as particles w/in a user-defined simulation space under a Smoluchowski-based reaction-diffusion system on a static time-step basis.
At each timestep, particles will diffuse under Brownian-like motion and any potential reactions between molecules will be resolved.
Simulation space is divided into cubic sub-partitions called c-voxels, groups of these c-voxels can be used to define spatial compartments that can have optional rules that govern particle permeability, and reactions can be compartment-specific as well.

□ INTEGRATE-Circ and INTEGRATE-Vis: Unbiased Detection and Visualization of Fusion-Derived Circular RNA
>> https://academic.oup.com/bioinformatics/advance-article/doi/10.1093/bioinformatics/btad569/7273782
INTEGRATE-Circ is an open-source software tool capable of integrating both RNA and whole genome sequencing data to perform unbiased detection of novel gene fusions and report the presence of splice variants in gene fusion transcripts, including backsplicing events.
Recurrent gene fusions were identified from the COSMIC and theoretical backsolice junctions were randomly introduced to the selected fusions. Linear fusion transcripts and linearized versions of the regions that spanned the simulated backsplices were used to simulate reads.

□ SingleCellMultiModal: Curated single cell multimodal landmark datasets for R/Bioconductor
>> https://journals.plos.org/ploscompbiol/article?id=10.1371/journal.pcbi.1011324
Collecting publicly available landmark datasets from important single-cell multimodal protocols, including CITE-Seq, ECCITE-Seq, SCoPE2, scNMT, 10X Multiome, seqFISH, and G&T.
SingleCellMultiModal R/Bioconductor package that provides single-command access to landmark datasets from seven different technologies, storing datasets using HDF5 and sparse arrays for memory efficiency and integrating data modalities via the MultiAssayExperiment class.

□ BioThings Explorer: a query engine for a federated knowledge graph of biomedical APIs
>> https://academic.oup.com/bioinformatics/advance-article/doi/10.1093/bioinformatics/btad570/7273783
BioThings Explorer (BTE) is an engine for autonomously querying a distributed knowledge graph. The distributed knowledge graph is made up of biomedical APIs that have been annotated with semantically-precise descriptions of their inputs and outputs in the SmartAPI registry.
BioThings Explorer leverages semantically precise annotations of the inputs and outputs for each resource, and automates the chaining of web service calls to execute multi-step graph queries.

□ The tidyomics ecosystem: Enhancing omic data analyses
>> https://www.biorxiv.org/content/10.1101/2023.09.10.557072v1
tidyomics, an interoperable software ecosystem that bridges Bioconductor and the tidyverse. tidyomics is easily installable with a single homonymous meta-package.
This ecosystem includes three new R packages: tidySummarizedExperiment, tidySingleCell Experiment, and tidySpatialExperiment, and five that are publicly available: plyranges", nullranges, tidyseura, tidybulk, tidytof.

□ EHE: Dissecting the high-resolution genetic architecture of complex phenotypes by accurately estimating gene-based conditional heritability
>> https://www.cell.com/ajhg/fulltext/S0002-9297(23)00282-3
EHE (the effective heritability estimator) can use p values from genome-wide association studies (GWASs) for local heritability estimation by directly converting marginal heritability estimates of SNPs to a non-redundant heritability estimate of a gene or a small genomic region.
EHE estimates the conditional heritability of nearby genes, where redundant heritability among the genes can be removed further. The conditional estimation can be guided by tissue-specific expression profiles to quantify more functionally important genes of complex phenotypes.

□ BG2: Bayesian variable selection in generalized linear mixed models with nonlocal priors for non-Gaussian GWAS data
>> https://bmcbioinformatics.biomedcentral.com/articles/10.1186/s12859-023-05468-w
A novel Bayesian method to find SNPs associated with non-Gaussian phenotypes. Using generalized linear mixed models (GLMMs) and, thus, the method called Bayesian GLMMs for GWAS (BG2). This is the first time that nonlocal priors are proposed for regression coefficients in GLMMs.
BG2 uses a two-step procedure: first, BG2 screens for candidate SNPs; second, BG2 performs model selection that considers all screened candidate SNPs as possible regressors.
BG2 uses a pseudo-likelihood approach to facilitate integrating out the random effects. Such pseudo-likelihood approach leads to a Gaussian approximation for adjusted observations that allows analytically integrating out the random effects.

□ DNA sequencing at the picogram level to investigate life on Mars and Earth
>> https://www.nature.com/articles/s41598-023-42170-6
In this research, it is assumed that if there is a living organism within the returned Mars Sample Collection with the possibility to replicate, and thus, the type of organism that background planetary protection protocols need to contain and control.
It relies on the same chemical processes as terrestrial organisms and it codes its genetic information with the known bases (ATGC for DNA, and AUGC for RNA) that are ubiquitously used by life on Earth.

□ cdsBERT - Extending Protein Language Models with Codon Awareness
>> https://www.biorxiv.org/content/10.1101/2023.09.15.558027v1
cdsBERT (CoDing Sequence Bidirectional Encoder Representation Transformer) was seeded with ProtBERT and further trained on 4 million CoDing Sequences (CDS) compiled from the NIH and Ensembl databases.
MELD (Masked Extended Language Distillation) is a vocabulary extension pipeline that was trained w/ Knowledge Distillation. The hypothesis was that a shift in synonymous codon embeddings w/in the TEM would indicate a nontrivial addition of protein information after applying MELD.
※コメント投稿者のブログIDはブログ作成者のみに通知されます