
我昔所造諸悪業
皆由無始貪瞋癡
従身口意之所生
一切我今皆懺悔
響きは発生した刹那から静寂へ吸い込まれていく。明滅する現象界の狭間に、儚い願いと共に信号を送るのように。

□ Hyperspherical Dirac Mixture Reapproximation
>> https://arxiv.org/pdf/2110.10411.pdf
Hyperspherical localized cumulative distribution (HLCD) is introduced as a local and smooth characterization of the underlying continuous density in hyperspherical domains.
a manifold-adapted modification of the Cram ́er–von Mises distance measures the statistical divergence b/w two Dirac mixtures. the hyperspherical Dirac mixture reapproximation (HDMR), for efficient discrete probabilistic modeling on unit hyperspheres of arbitrary dimensions.

□ Tangent Space and Dimension Estimation with the Wasserstein Distance
>> https://arxiv.org/pdf/2110.06357.pdf
The estimators arise from a local version of principal component analysis (PCA). This approach directly estimates covariance matrices locally, which simultaneously allows estimating both the tangent spaces and the intrinsic dimension of a manifold.
A matrix concentration inequality, a Wasserstein bound for flattening a manifold, and a Lipschitz relation for the covariance matrix with respect to the Wasserstein distance.

□ hifiasm-meta: Metagenome assembly of high-fidelity long reads
>> https://arxiv.org/pdf/2110.08457.pdf
hifiasm-meta has an optional read selection step that reduces the coverage of highly abundant strains without losing reads on low abundant strains. hifiasm-meta tries to protect reads in genomes of low coverage, which may be treated as chimeric reads.
hifiasm-meta only drops a contained read if other reads exactly overlapping with the read are inferred to come from the same haplotype. This reduces contig breakpoints caused by contained reads.
hifiasm-meta uses the coverage information to prune unitig overlaps, assuming unitigs from the same strain tend to have similar coverage. It also tries to join unitigs from different haplotypes to patch the remaining assembly gaps.
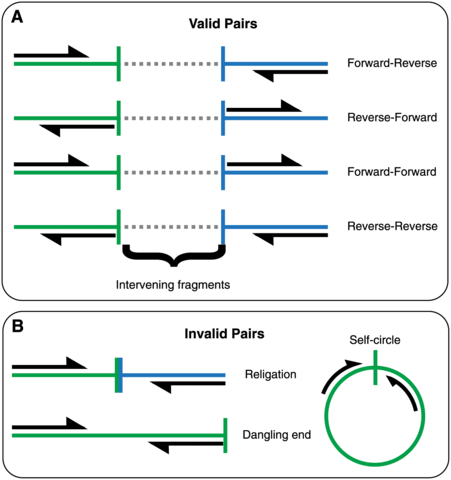
□ qc3C: Reference-free quality control for Hi-C sequencing data
>> https://journals.plos.org/ploscompbiol/article?id=10.1371/journal.pcbi.1008839
qc3C can be done without access to a reference sequence, which until now has been a significant stopping point for projects not involving model organisms.
qc3C can also perform reference-based analysis. Statistics obtained from “bam mode” include such details as the number of read-through events and HiCPro style pair categorisation e.g. dangling-end, self-circle.

□ Circall: fast and accurate methodology for discovery of circular RNAs from paired-end RNA-sequencing data
>> https://bmcbioinformatics.biomedcentral.com/articles/10.1186/s12859-021-04418-8
Circall builds the back-splicing junction (BSJ) database based on the annotated reference, thus depends on the completion of the annotation.
Circall controls the FPs using a robust multidimensional local false discovery rate method based on the length and expression of circRNAs. It is computationally highly efficient by using a quasi-mapping algorithm for fast and accurate RNA read alignments.

□ scGAD: single-cell gene associating domain scores for exploratory analysis of scHi-C data
>> https://www.biorxiv.org/content/10.1101/2021.10.22.465520v1.full.pdf
scGAD enables summarization at the gene level while accounting for inherent gene-level genomic biases. Low-dimensional projections with scGAD capture clustering of cells based on their 3D structures.
Projection onto the scRNA-seq embedding from the same system revealed that the cells originating from the same cell type but quantified by different data modalities were tightly clustered. scGAD facilitated an accurate projection of cells onto this larger space.

□ SMURF: End-to-end learning of multiple sequence alignments with differentiable Smith-Waterman
>> https://www.biorxiv.org/content/10.1101/2021.10.23.465204v1.full.pdf
SMURF (Smooth Markov Unaligned Random Field), a new method that jointly learns an alignment and the parameters of a Markov Random Field for unsupervised contact prediction.
SMURF begins with a learned alignment module (LAM). For each sequence, a convolutional architecture produces a matrix of match scores between the sequence and a reference. A similarity tensor is constructed for each sequence with the vectors for the query sequence.

□ MERINGUE: Characterizing spatial gene expression heterogeneity in spatially resolved single-cell transcriptomic data with nonuniform cellular densities
>> https://genome.cshlp.org/content/31/10/1843
MERINGUE, a density-agnostic method for identifying spatial gene expression heterogeneity using spatial autocorrelation and cross-correlation analyses.
MERINGUE first represents these cells as neighborhoods using Voronoi tessellation. In Voronoi tessellation, planes are partitioned into neighborhoods where a neighborhood for a cell consists of all points closer to that cell than any other.

□ scMRA: A robust deep learning method to annotate scRNA-seq data with multiple reference datasets
>> https://academic.oup.com/bioinformatics/advance-article/doi/10.1093/bioinformatics/btab700/6384568
In scMRA, a knowledge graph is constructed to represent the characteristics of cell types in different datasets, and a graphic convolutional network (GCN) serves as a discriminator. scMRA keeps intra-cell-type closeness and the relative position of cell types across datasets.
Single-cell Multiple Reference Annotator (scMRA) is tailored to transform knowledges from multiple well-annotated data to the target unlabeled data. scMRA integrate information in those extra cell types into the adjacency matrix to better learn the embeddings of sequencing data.

□ FastqCLS: a FASTQ Compressor for Long-read Sequencing via read reordering using a novel scoring model
>> https://academic.oup.com/bioinformatics/advance-article/doi/10.1093/bioinformatics/btab696/6384565
Various compression techniques have been proposed to reduce the size of original FASTQ raw sequencing data, but these remain suboptimal. Long-read sequencing has become dominant in genomics, whereas most existing compression methods focus on short-read sequencing only.
FastqCLS, a new FASTQ compression tool specialized for long-read sequencing data of large genomes using read reordering and zpaq, which employs arithmetic coding, a form of an entropy encoding.

□ Efficient inference for agent-based models of real-world phenomena
>> https://www.biorxiv.org/content/10.1101/2021.10.04.462980v1.full.pdf
While some methods generally produce more robust results than others, no algorithm offers a one-size-fits-all solution when attempting to infer model parameters from observations.
The predictions of the emulators are directly compared to the mock observations, i.e. the synthetic data. And infer the underlying model parameters (Θ) using rejection Approximate Bayesian computation and Markov Chain Monte Carlo.

□ DiviSSR: Simple arithmetic for efficient identification of tandem repeats
>> https://www.biorxiv.org/content/10.1101/2021.10.05.462997v1.full.pdf
DiviSSR identifies tandem repeats by applying a division rule on the binary numbers resultant after 2-bit transformations of DNA sequences. DiviSSR is on average 5-10 fold faster than the next best tools and takes just ~30 secs to identify all perfect microsatellites in the human genome.
DiviSSR merges repeats as it scans through the input sequence by storing the location of the previous repeat. The time complexity of DiviSSR is O(nm), where n is the input data size and m is the number of desired motif sizes.

□ NN-RNALoc: neural network-based model for prediction of mRNA sub-cellular localization using distance-based sub-sequence profiles
>> https://www.biorxiv.org/content/10.1101/2021.10.06.463397v1.full.pdf
NN-RNALoc is a machine-learning based model to predict the sub-cellular location of mRNAs which is evaluated on two following datasets: Cefra-seq and RNALocate.
The results demonstrate that by employement of the distance-based sub-sequence profiles along with k-mer frequencies and with inclusion of PPI matrix data, NN-RNALoc which has simple and transparent neural network architecture.

□ mmbam: Memory mapped parallel BAM file access API for high throughput sequence analysis informatics
>> https://www.biorxiv.org/content/10.1101/2021.10.05.463280v1.full.pdf
mmbam, a library to allow sequence analysis informatics software to access raw sequencing data stored in BAM files extremely fast.
Mmbam enables parallel processing of alignment data via memory mapped file access, and utilizes the scatter / gather paradigm to parallelize computation tasks across many genomic regions before combining the regional results to produce global results.
□ CoMM-S 4: A Collaborative Mixed Model Using Summary-Level eQTL and GWAS Datasets in Transcriptome-Wide Association Studies
>> https://www.frontiersin.org/articles/10.3389/fgene.2021.704538/full
CoMM-S4, a likelihood-based method which uses individual-level eQTL data to assess expression-trait association, and propose a probabilistic model, Collaborative Mixed Models using Summary Statistics from eQTL and GWAS.
CoMM-S4, like S-PrediXcan, is not able to distinguish between causal relationship and horizontal pleiotropy. CoMM-S4 uses an efficient algorithm based on variational Bayes expectation-maximization and parameter expansion (PX-VBEM).
□ Fast and compact matching statistics analytics
>> https://www.biorxiv.org/content/10.1101/2021.10.05.463202v1.full.pdf
a lossy compression scheme that can reduce the size of our compact encoding to much less than 2|S| bits when S and T are dissimilar, by replacing small match- ing statistics values (that typically arise from random matches) with other, suitably chosen small values.
a practical variant of the algorithm that computes MS in parallel on a shared-memory machine, and that achieves approximately a 41-fold speedup of the core procedures and a 30-fold speedup of the entire program with 48 cores on the instances that are most difficult to parallelize.

□ Lpnet: Reconstructing Phylogenetic Networks from Distances Using Integer Linear Programming
>> https://www.biorxiv.org/content/10.1101/2021.10.08.463657v1.full.pdf
the Lpnet algorithm uses a distance matrix as its input. First it constructs a phylogenetic tree from the distances, then it uses Linear Programming to find a circular ordering which maximizes the sum of all quartet weights consistent with the circular ordering.
Lpnet, a variant of Neighbor- net that does not apply the second heuristic step of the agglomeration. the integer linear programming problem in Lpnet uses a quadratic number of variables and a cubic number of constraints.

□ RDBKE: Enhancing breakpoint resolution with deep segmentation model: A general refinement method for read-depth based structural variant callers
>> https://journals.plos.org/ploscompbiol/article?id=10.1371/journal.pcbi.1009186
deepIntraSV, UNet model for segmenting intra-bin structural variants with base-pair read-depth data of WGS. RDBKE uses the deep segmentation model UNet to learn base-wise Read Depth (RD) patterns surrounding breakpoints of known SVs.
the UNet model could also be applied for one-dimensional genomic data. RDBKE formalizes the breakpoint prediction as a segmentation task and inferred breakpoints in single-nucleotide resolution from predicted label marks.

□ scREMOTE: Using multimodal single cell data to predict regulatory gene relationships and to build a computational cell reprogramming model
>> https://www.biorxiv.org/content/10.1101/2021.10.11.463798v1.full.pdf
scREMOTE, a novel computational model for cell reprogramming that leverages single cell multiomics data, enabling a more holistic view of the regulatory mechanisms at cellular resolution.
This is achieved by first identifying the regulatory potential of each transcription factor and gene to uncover regulatory relationships, then a regression model is built to estimate the effect of transcription factor perturbations.
□ Translation procedures in descriptive inner model theory
>> https://arxiv.org/pdf/2110.06091v1.pdf
if there is a stationary class of λ such that λ is a limit of Woodin cardinals and the derived model at λ satisfies AD+ + θ0 < Θ then there is a transitive model M such that Ord ⊆ M and M “there is a proper class of Woodin cardinals and a strong cardinal”.
Using a theorem of Woodin on derived models it is not hard to see that the reverse of the aforementioned theorem is also true, thus proving that the two theories are in fact equiconsistent.

□ ONTdeCIPHER: An amplicon-based nanopore sequencing pipeline for tracking pathogen variants
>> https://www.biorxiv.org/content/10.1101/2021.10.13.464242v1.full.pdf
ONTdeCIPHER is an Oxford Nanopore Technology (ONT) amplicon-based sequencing pipeline to perform key downstream analyses on raw sequencing data from quality testing to SNPs effect to phylogenetic analysis.
ONTdeCIPHER integrates 13 bioinformatics tools, including Seqkit, ARTIC bioinformatics tool, PycoQC, MultiQC, Minimap2, Medaka, Nanopolish, Pangolin (with the model database pangoLEARN), Deeptools (PlotCoverage, BamCoverage), Sniffles, MAFFT, RaxML and snpEff.
□ Incomplete Multiple Kernel Alignment Maximization for Clustering
>> https://ieeexplore.ieee.org/document/9556554
Integrating the imputation of incomplete kernel matrices and Multiple Kernel Alignment maximization for clustering into a unified learning framework.
The clustering of Multiple Kernel Alignment maximization guides the imputation of incomplete kernel elements, and the completed kernel matrices are in turn combined to conduct the subsequent Multiple Kernel Clustering.
These two procedures are alternately performed until convergence. By this way, the imputation and Multiple Kernel Clustering processes are seamlessly connected.
□ LFMKC-PGR: Late Fusion Multiple Kernel Clustering With Proxy Graph Refinement
>> https://ieeexplore.ieee.org/document/9573366/
the kernel partition learning and late fusion processes are separated from each other in the existing mechanism, which may lead to suboptimal solutions and adversely affect the clustering performance.
LFMKC-PGR, a novel late fusion multiple kernel clustering with proxy graph refinement framework to address these issues. LFMKC-PGR constructs a proxy self-expressive graph from kernel base partitions.
The proxy graph in return refines the individual kernel partitions and also captures partition relations in graph structure rather than simple linear transformation.
LFMKC-PGR provides theoretical connections and considerations between the proposed framework and the multiple kernel subspace clustering. An alternate algorithm with proved convergence is then developed to solve the resultant optimization problem.

□ BASE: A novel workflow to integrate nonubiquitous genes in comparative genomics analyses for selection
>> https://onlinelibrary.wiley.com/doi/10.1002/ece3.7959
BASE is a workflow for analyses on selection regimes that integrates several popular pieces of software, with CodeML at its core. BASE allows to seamlessly carry out a user-specified number of replicate analyses, incorporating random omega starting values.
This circumstance can underlie a wide range of technical and biological phenomena—such as sequence misalignment, nonorthology, and incomplete lineage sorting—which can ultimately bias evolutionary rate inference.
In order to account for such possibility, when a fixed species tree is specified BASE will report its normalized Robinson–Foulds distances with each gene tree, calculated using ete3.
□ Eoulsan 2: an efficient workflow manager for reproducible bulk, long-read and single-cell transcriptomics analyses
>> https://www.biorxiv.org/content/10.1101/2021.10.13.464219v1.full.pdf
Eoulsan is a versatile framework based on the Hadoop implementation of the MapReduce algorithm, dedicated to high throughput sequencing data analysis on distributed computers.
Eoulsan 2, a major update that (i) enhances the workflow manager itself, (ii) facilitates the development of new modules, and (iii) expands its applications to long reads RNA-seq (Oxford Nanopore Technologies) and scRNA-seq (Smart-seq2 and 10x Genomics).
□ Polish topologies on groups of non-singular transformations
>> https://arxiv.org/pdf/2110.07289v1.pdf
the group of measure-preserving transformations of the real line whose support has finite measure carries no Polish group topology.
Characterize the Borel σ-finite measures λ on a standard Borel space for which the group of λ-preserving transformations has the automatic continuity property. the natural Polish topology on the group of all non-singular transformations is actually its only Polish group topology.

□ Tailored graphical lasso for data integration in gene network reconstruction
>> https://bmcbioinformatics.biomedcentral.com/articles/10.1186/s12859-021-04413-z
Assuming a Gaussian graphical model, a gene association network may be estimated from multiomic data based on the non-zero entries of the inverse covariance matrix.
The method also has a nice interpretability through the estimated value of k, giving us a “usefulness score” for the prior information, where k close to zero indicates that the prior information does not provide any useful information while larger k indicates that it does.
the tailored graphical is the most suitable for network inference from high-dimensional data with prior information of unknown accuracy.

□ Fractional Calderón problem on a closed Riemannian manifold
>> https://arxiv.org/pdf/2110.07500v1.pdf
the inverse problem of re-covering the isometry class of a smooth closed and connected Riemannian manifold (M,g),
Given the knowledge of a source-to-solution map for the fractional Laplace equation (−∆ )αu = f on the manifold subject to an garbitrarily small observation region O where sources can be placed and solutions can be measured.
Assuming only a local property on the a priori known observation region O while making no geometric assumptions on the inaccessible region of the manifold, namely M \ O.
Thia proof is based on discovering a hidden connection to a variant of Carlson’s theorem in complex analysis that allows us to reduce the non-local inverse problem to the Gel’fand inverse spectral problem.
□ Minimax extrapolation problem for periodically correlated stochastic sequences with missing observations
>> https://arxiv.org/pdf/2110.06675.pdf
Formulas that determine the least favorable spectral densities and the minimax-robust spectral characteristics of the optimal estimates of functionals are proposed in the case of spectral uncertainty,
where the spectral densities are not exactly known while some sets of admissible spectral densities are specified.

□ SIMBA: SIngle-cell eMBedding Along with features
>> https://www.biorxiv.org/content/10.1101/2021.10.17.464750v1.full.pdf
SIMBA is a single-cell embedding method with support for single- or multi- modality analyses that embeds cells and their associated genomic features into a shared latent space, generating interpretable and comparable embeddings of cells and features.
SIMBA readily corrects batch effects and produces joint embeddings of cells and features across multiple datasets with different sequencing platforms and cell type compositions.
SIMBA works as a stand-alone package obviating the need for prior input data correction when applied to multi-batch scRNA-seq dataset. In SIMBA, batch correction is accomplished by encoding multiple scRNA-seq datasets into a single graph.
□ ORTHOSCOPE*: a phylogenetic pipeline to infer gene histories from genome-wide data
>> https://academic.oup.com/mbe/advance-article/doi/10.1093/molbev/msab301/6400256
ORTHOSCOPE* estimates a tree for a specified gene, detects speciation/gene duplication events that occurred at nodes belonging to only one lineage leading to a species of interest, and integrates results derived from gene trees estimated for all query genes in genome-wide data.
ORTHOSCOPE* can offer a set of orthology-confirmed gene markers for environmental DNA analyses. By using an amino acid file defined in the control.txt file, ORTHOSCOPE* automatically creates an amino acid database for each species by MAKEBLASTDB with -dbtype prot option.

□ REViewer: Haplotype-resolved visualization of read alignments in and around tandem repeats
>> https://www.biorxiv.org/content/10.1101/2021.10.20.465046v1.full.pdf
Repeat Expansion Viewer (REViewer) has been designed to work with the read alignments produced by ExpansionHunter, though it will work with any repeat genotyping software that produces output in the appropriate format.
REViewer constructs all possible pairs of haplotype sequences from the STR genotypes. REViewer reconstructs local haplotype sequences and distributes reads to these haplotypes in a way that is most consistent with the fragment lengths and evenness of read coverage.
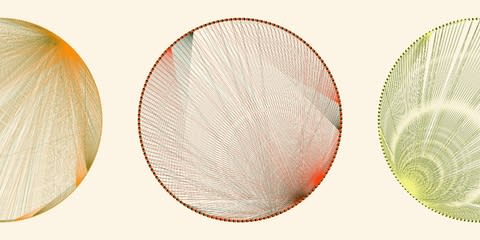
□ Creating Generative Art NFTs from Genomic Data
>> https://towardsdatascience.com/creating-generative-art-nfts-from-genomic-data-16a48ae4df99
a dynamic NFT on the Ethereum blockchain with IPFS and discuss the possible use cases for scientific data.
function _mint(address to, uint256 tokenId) internal virtual {
require(to != address(0), "ERC721: mint to the zero address");
require(!_exists(tokenId), "ERC721: token already minted");
_beforeTokenTransfer(address(0), to, tokenId);
_balances[to] += 1;
_owners[tokenId] = to;
emit Transfer(address(0), to, tokenId);
}

□ SINBAD: a flexible tool for single cell DNA methylation data https://www.biorxiv.org/content/10.1101/2021.10.23.465577v1.full.pdf
SINBAD demultiplexes the raw reads using cell barcode sequence information, which is technology dependent. The indexed reads, which are defined as those that match the given indices, are generated for each individual cell as the output.
the dimensionality of the methylation matrix is reduced by the multivariate analysis module and cell populations are detected by clustering analysis.

□ ProPIP: a tool for progressive multiple sequence alignment with Poisson Indel Process
>> https://bmcbioinformatics.biomedcentral.com/articles/10.1186/s12859-021-04442-8
ProPIP - The process of insertions and deletions is described using an explicit evolutionary model—the Poisson Indel Process or PIP. The method is based on dynamic programming and is implemented in a frequentist framework.
Instead of the arbitrary gap penalties, the parameters used by ProPIP are the insertion and deletion rates, which have biological interpretation and are contextualized in a probabilistic environment.
ProPIP implements the originally published progressive MSA inference method based on PIP, and also introduces new features, such as stochastic backtracking and parallelisation.

□ TPSC: a module detection method based on topology potential and spectral clustering in weighted networks and its application in gene co-expression module discovery
>> https://bmcbioinformatics.biomedcentral.com/articles/10.1186/s12859-021-03964-5
the Topology Potential-based Spectral Clustering (TPSC) Algorithm, an improved module detection algorithm based on topology potential and spectral clustering and use it to detect co-expression modules.
TPSC algorithm found that the module related to extracellular matrix and structure organization does not identified by both lmQCM and WGCNA algorithm. The method improved upon a previous method for full-connected network and asymmetric Laplacian matrix.
※コメント投稿者のブログIDはブログ作成者のみに通知されます