
"What should happen in the future" is nothing but "what is happening at this moment"
「未来に起こるべきこと」は「今起きていること」に他ならない
「統計によって何を知るか」ではなく、「統計されている構造を知ること」が重要である。

□ SELMA: Accurate estimation of intrinsic biases for improved analysis of chromatin accessibility sequencing data
>> https://www.biorxiv.org/content/10.1101/2021.10.22.465530v1.full.pdf
SELMA (Simplex Encoded Linear Model for Accessible Chromatin), a computational framework for the accurate estimation of intrinsic cleavage biases and improved analysis of DNase/ATAC-seq data for both bulk and single-cell experiments.
SELMA generates more robust bias estimation from bulk data than the naïve k-mer model. SELMA encodes each k-mer as a vector in the Hadamard Matrix, derived from a simplex encoding model, in which the k-mer sequences are encoded as the vertices of a regular 0-centered simplex.

□ NanoSplicer: Accurate identification of splice junctions using Oxford Nanopore sequencing
>> https://www.biorxiv.org/content/10.1101/2021.10.23.465402v1.full.pdf
NanoSplicer utilises the raw ouput from nanopore sequencing (measures of electrical current commonly known as squiggles) to improve the identification of splice junctions. Instead of identifying splice junctions by mapping basecalled reads.
nanosplicer compares the squiggle from a read with the predicted squiggles of potential splice junctions to identify the best match and likely junction. nanosplicer uses the support in the junction squiggle for the model as a measure of similarity in Dynamic Time Warping.

□ VSS-Hi-C: Variance-stabilized signals for chromatin 3D contacts
>> https://www.biorxiv.org/content/10.1101/2021.10.19.465027v1.full.pdf
VSS-Hi-C stabilizes the variance of Hi-C contact strength. This method learns the empirical mean-variance relationship of the Hi-C matrices and transforms the Hi-C contact strength using a transformation based on this learned mean-variance relationship.
VSS-Hi-C transformed matrices have a fully stabilized mean-variance relationship, in contrast to other transformation methods. Variance-stabilized signals are beneficial for downstream analyses like identifying topological domains and subcompartments.

□ PeakBot: Machine learning based chromatographic peak picking
>> https://www.biorxiv.org/content/10.1101/2021.10.11.463887v1.full.pdf
These are subsequently inspected by a custom-trained convolutional neural network that forms the basis of PeakBot’s architecture. This is achieved by first searching for chromatographic peaks using a smoothing and gradient-descend algorithm.
PeakBot detects all local signal maxima in a chromatogram, which are then extracted as super-sampled standardized areas. The model reports if the respective local maximum is the apex of a chromatographic peak or not as well as its peak center and bounding box.

□ ReFeaFi: Genome-wide prediction of regulatory elements driving transcription initiation
>> https://journals.plos.org/ploscompbiol/article?id=10.1371/journal.pcbi.1009376
ReFeaFi, a dynamic negative set updating scheme with a two-model approach, using one model for scanning the genome and the other one for testing candidate positions.
Empty vector and random sequences were used as negative controls, while GAPDH promoter is used as positive control. ReFeaFi achieves outstanding performance on discriminating VISTA enhancers and 100 times as many random genomic regions.
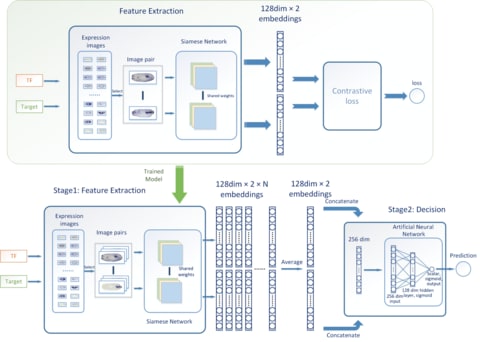
□ ConGRI: Accurate inference of gene regulatory interactions from spatial gene expression with deep contrastive learning
>> https://academic.oup.com/bioinformatics/advance-article-abstract/doi/10.1093/bioinformatics/btab718/6401998
The high-throughput spatial gene expression data, like in situ hybridization images that exhibit temporal and spatial expression patterns, has provided abundant and reliable information for the inference of GRNs.
ConGRI is featured by a contrastive learning scheme and deep Siamese CNN architecture, which automatically learns high-level feature embeddings for the expression images and feeds the embeddings to an artificial neural network to determine whether or not the interaction exists.

□ A novel algorithm to flag columns associated in any way with others or a dependent variable is computationally tractable in large data matrices and has much higher power when columns are linked like mutations in chromosomes.
>> https://www.biorxiv.org/content/10.1101/2021.09.15.460360v1.full.pdf
When a data matrix DM has many independent variables IVs, it is not computationally tractable to assess the association of every distinct IV subset with the dependent variable DV of the DM, because the number of subsets explodes combinatorially as IVs increase.
a computationally tractable, fully parallelizable Participation in Association Score (PAS) that in a DM with markers detects one by one every column that is strongly associated in any way with others.

□ Identifying common and novel cell types in single-cell RNA-sequencing data using FR-Match
>> https://www.biorxiv.org/content/10.1101/2021.10.17.464718v1.full.pdf
FR-Match matches query datasets to reference atlases with robust and accurate performance for identifying novel cell types and non-optimally clustered cell types in the query data.
FR-Match is an iterative procedure that allows each cell in the query cluster to be assigned a summary p-value, quantifying the confidence of matching, to a reference cluster. FR-Match forms a clean diagonal alignment of cell types and assigned unmatched cells as “unassigned”.
□ AlphaDesign: A de novo protein design framework based on AlphaFold
>> https://www.biorxiv.org/content/10.1101/2021.10.11.463937v1.full.pdf
AlphaDesign, a computational framework for de novo protein design that embeds AF as an oracle within an optimisable design process. This framework enables rapid prediction of completely novel protein monomers starting from random sequences.
Structural integrity of predicted structures is validated by ab initio folding / structural analysis as well as extensively by rigorous all-atom molecular dynamics simulations and analysing the corresponding structural flexibility, intramonomer / interfacial amino-acid contacts.
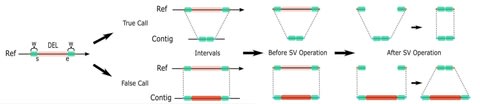
□ TT-Mars: Structural Variants Assessment Based on Haplotype-resolved Assemblies
>> https://www.biorxiv.org/content/10.1101/2021.09.27.462044v1.full.pdf
TT- Mars, that takes advantage of the recent production of high-quality haplotype-resolved genome assemblies by evaluating variant calls based on how well their call reflects the content of the assembly, rather than comparing calls themselves.
Compared with validation using dipcall variants, TT-Mars analyzes 1,497-2,229 more calls on long read callsets and has favorable results when candidate calls are fragmented into multiple calls in alignments.

□ motif_prob: Fast and exact quantification of motif occurrences in biological sequences
>> https://bmcbioinformatics.biomedcentral.com/articles/10.1186/s12859-021-04355-6
Exact formulae for motif occurrence, under Bernoullian or Markovian models, have exponential complexity, thus can be cumbersome to be implemented efficiently, but approximations can be calculated with constant cost.
‘motif_prob’, a fast implementation of an exact formula for motif count distribution through progressive approximation with arbitrary precision. motif_prob is 50–1000× faster than MoSDi exact and 60–120× faster than MoSDi compound Poisson.
Given the motif m and genome g lengths, one can set a tolerance level ε such that P(0, m, n) > (1 − ε), and in general each case where (1 − P(S))(m−m+1) > (1 − ε). This is equal to (n − m + 1)∙log(1 − P(S)) > log(1 − ε), which implies n > m − 1 + log(1 − ε)/log(1 − P(S)).
□ vcf2gwas—python API for comprehensive GWAS analysis using GEMMA https://academic.oup.com/bioinformatics/advance-article/doi/10.1093/bioinformatics/btab710/6390796
GEMMA can fit a univariate linear mixed model, a multivariate mixed model,, and a Bayesian sparse linear mixed model for testing marker associations with a trait of interest in different organisms.
vcf2gwas is especially helpful when analyzing large numbers of phenotypes or different sets of individuals because it can perform the analyses in parallel with a single .csv file with all the phenotypes. And offers features like analyzing reduced phenotypic space.

□ GBSmode: a pipeline for haplotype-aware analysis of genotyping-by-sequencing data
>> https://www.biorxiv.org/content/10.1101/2021.09.20.461130v1.full.pdf
Genotyping-by-sequencing (GBS) enables simultaneous genotyping of thousands of DNA markers in the genome of any species. GBS exploits a restriction enzyme to reduce genome complexity and directs the sequencing to begin at fixed digestion sites.
GBSmode, a dedicated pipeline to call DNA sequence variants using whole-read information from GBS data. It removes false positives by incorporating biological features such as the ploidy level and the number of possible alleles in the population under investigation.

□ BindVAE: Dirichlet variational autoencoders for de novo motif discovery from accessible chromatin
>> https://www.biorxiv.org/content/10.1101/2021.09.23.461564v1.full.pdf
BindVAE, based on Dirichlet variational autoencoders, for jointly decoding multiple TF binding signals from open chromatin regions. BindVAE automatically learns distinct groups of k-mer patterns that correspond to cell type-specific in vivo binding signals.
BindVAE uses 8-mers with wildcards, which allows us to interpret the learned latent factors. Of the 102 distinct patterns learned over the latent dimensions, BindVAE found specific patterns for some TFs and were able to map the latent factors to unique TFs.

□ BionetBF: A Novel Bloom Filter for Faster Membership Identification of Paired Biological Network Data
>> https://www.biorxiv.org/content/10.1101/2021.09.23.461527v1.full.pdf
BionetBF is capable of executing millions of operations within a second on datasets having millions of paired biological data while occupying tiny amount of main memory.
BionetBF is also compared with other filters: Cuckoo Filter and Libbloom, where BionetBF proves its supremacy by exhibiting higher performance with a smaller sized memory compared with large sized filters of Cuckoo Filter and Libbloom.

□ MONTI: A Multi-Omics Non-negative Tensor Decomposition Framework for Gene-Level Integrative Analysis https://www.frontiersin.org/articles/10.3389/fgene.2021.682841/full
SNF (Similarity Network Fusion) integrates multi-omics data by constructing networks for each omics data in terms of the sample similarity using the omics data and then fusing the networks iteratively using the message-passing method.
MONTI (Multi-Omics Non-negative Tensor Decomposition Integration) that learns hidden features through tensor decomposition for the integration of multi-omics data. The omics matrices are stacked to form a 3-dimensional tensor structure all sharing the same genes.
□ Improving structural variant clustering to reduce the negative effect of the breakpoint uncertainty problem
>> https://bmcbioinformatics.biomedcentral.com/articles/10.1186/s12859-021-04374-3
a statistically significant enrichment of the pattern of decomposed SVs during the evaluation of conventional clustering strategies.
It can be argued that MEI-based quantities, especially Nic, have limited informative values in this case because maximization of Nic is implicitly included in the constrained clustering algorithm.

□ LoHaMMer: Evaluation of Vicinity-based Hidden Markov Models for Genotype Imputation
>> https://www.biorxiv.org/content/10.1101/2021.09.28.462261v1.full.pdf
the HMM evaluates the paths over only a short stretch of variants around the untyped variants. LoHaMMer can perform the computations in the logarithmic domain or it scales the ML and forward-backward variables by a scaling factor.
LoHaMMer keeps track of any overflow and underflow at each computation step. If an array value becomes too high or too low, the values are re-scaled to ensure numerical stability.

□ Evolutionary strategies applied to artificial gene regulatory networks
>> https://www.biorxiv.org/content/10.1101/2021.09.28.462218v1.full.pdf
a population of computational robotic models controlled by artificial gene regulatory networks (AGRNs) to evaluate the impact of different genetic modification strategies in the course of evolution.
a gradual increase in the complexity of the performed tasks is beneficial for the evolution of the model.

□ STRATISFIMAL LAYOUT: A modular optimization model for laying out layered node-link network visualizations
>> https://ieeexplore.ieee.org/document/9556579/
Using a layout optimization model that prioritizes optimality – as compared to scalability – because an optimal solution not only represents the best attainable result, but can also serve as a baseline to evaluate the effectiveness of layout heuristics.
STRATISFIMAL LAYOUT, a modular integer-linear-programming formulation that can consider several important readability criteria simultaneously – crossing reduction, edge bendiness, and nested and multi-layer groups.
□ Incomplete Multiple Kernel Alignment Maximization for Clustering
>> https://ieeexplore.ieee.org/document/9556554/
Multiple kernel alignment (MKA) maximization criterion has been widely applied into multiple kernel clustering (MKC) and many variants have been recently developed.
The clustering of MKA maximization guides the imputation of incomplete kernel elements, and the completed kernel matrices are in turn combined to conduct the subsequent Multiple kernel alignment.

□ Open Imputation Server provides secure Imputation services with provable genomic privacy
>> https://www.biorxiv.org/content/10.1101/2021.09.30.462262v1.full.pdf
a client-server-based outsourcing framework for genotype imputation, an important step in genomic data analyses.
Genotype data is encrypted once at the client and submitted to the server, which securely imputes the untyped variants without decrypting the genotypes.

□ ssNet: Integration of probabilistic functional networks without an external Gold Standard
>> https://www.biorxiv.org/content/10.1101/2021.10.01.462727v1.full.pdf
ssNet is easier and faster, overcoming the challenges of data redundancy, Gold Standard bias and ID mapping, while producing comparable performance. In addition ssNet results in less loss of data and produces a more complete network.
The ssNet method provides a computationally amenable one-step PFIN integration method for functional interaction data. ssnet takes a BioGRID file of functional interaction data for a species and produces a probabilitistic functional integrated network.

□ CellDepot: A unified repository for scRNA-seq data and visual exploration
>> https://www.biorxiv.org/content/10.1101/2021.09.30.462602v1.full.pdf
CellDepot integrates with advanced single-cell transcriptomic data explorer to conduct all analytical tasks on the webserver while presenting interactive results on the webpage through leveraging modern web development techniques.
CellDepot requires scRNA-seq data in h5ad file where the expression matrix is stored in CSC (compressed sparse column) instead of CSR (compressed sparse row) format to improve the speed of data retrieving.

□ Productive visualization of high-throughput sequencing data using the SeqCode open portable platform
>> https://www.nature.com/articles/s41598-021-98889-7
SeqCode is entirely focused on the graphical analysis of 1D genomic data. t has been implemented in ANSI C following a modular architecture of blocks.
□ DisCovER: distance- and orientation-based covariational threading for weakly homologous proteins
>> https://pubmed.ncbi.nlm.nih.gov/34599831/
DisCovER, new distance- and orientation-based covariational threading method by effectively integrating information from inter-residue distance and orientation along with the topological network neighborhood of a query-template alignment.
DisCovER selects a subset of templates using standard profile-based threading coupled with topological network similarity terms to account, and subsequently performs distance- and orientation-based query-template alignment using an iterative double dynamic programming framework.

□ SamQL: a structured query language and filtering tool for the SAM/BAM file format
>> https://bmcbioinformatics.biomedcentral.com/articles/10.1186/s12859-021-04390-3
SamQL has intuitive syntax allowing complex queries and takes advantage of parallelizable handling of BAM files.
SamQL builds an abstract syntax tree (AST) corresponding to the query. The AST is then parsed, depth-first, to progressively build a function closure that encapsulates the whole query.
□ Spatial rank-based multifactor dimensionality reduction to detect gene–gene interactions for multivariate phenotypes
>> https://bmcbioinformatics.biomedcentral.com/articles/10.1186/s12859-021-04395-y
The new multivariate rank-based MDR (MR-MDR) is mainly suitable for analyzing multiple continuous phenotypes and is less sensitive to skewed distributions and outliers.
MR-MDR utilizes fuzzy k-means clustering and classifies multi-locus genotypes into two groups. Then, MR-MDR calculates a spatial rank-sum statistic as an evaluation measure and selects the best interaction model with the largest statistic.

□ BioKIT: a versatile toolkit for processing and analyzing diverse types of sequence data
>> https://www.biorxiv.org/content/10.1101/2021.10.02.462868v1.full.pdf
BioKIT, a versatile toolkit with 40 functions, several of which were community sourced, that conduct routine and novel processing and analysis of diverse sequence files including genome assemblies, multiple sequence alignments, protein coding sequences, and sequencing data.
Functions implemented in BioKIT facilitate a wide variety of standard bioinformatic analyses, including genome assembly quality assessment, the calculation of multiple sequence alignment properties; number of taxa, alignment length, the number of parsimony-informative sites.
□ iDNA-ABT : advanced deep learning model for detecting DNA methylation with adaptive features and transductive information maximization
>> https://academic.oup.com/bioinformatics/advance-article-abstract/doi/10.1093/bioinformatics/btab677/6380543
iDNA-ABT, an advanced deep learning model that utilizes adaptive embedding based on bidirectional transformers for language understanding together with a novel transductive information maximization (TIM) loss.
iDNA-ABT can automatically and adaptively learn the distinguishing features of biological sequences from multiple species. iDNA-ABT has strong adaptability and robustness to different species through comparison of adaptive embedding and six handcrafted feature encodings.
□ Efficient Change-Points Detection For Genomic Sequences Via Cumulative Segmented Regression
>> https://academic.oup.com/bioinformatics/advance-article-abstract/doi/10.1093/bioinformatics/btab685/6380564
The cumulative segmented algorithm (cumSeg) has been recently proposed as a computationally efficient approach for multiple change-points detection, which is based on a simple transformation of data and provides results quite robust to model mis-specifications.
Two new change-points detection procedures in the framework of cumulative segmented regression. the proposed methods not only improve the efficiency of each change point estimator substantially but also provide the estimators with similar variations for all the change points.
□ K2Mem: Discovering Discriminative K-mers from Sequencing Data for Metagenomic Reads Classification
>> https://ieeexplore.ieee.org/document/9557831/
Studying the problem of metagenomic reads classification by improving the reference k-mers library with novel discriminative k-mers from the input sequencing reads and is proposed a metagenomics classification tool, named K2Mem.
K2 is based, not only on a set of reference genomes, but also it uses discriminative k-mers from the input metagenomics reads in order to improve the classification.

□ Mining hidden knowledge: Embedding models of cause-effect relationships curated from the biomedical literature
>> https://www.biorxiv.org/content/10.1101/2021.10.07.463598v1.full.pdf
Gene embeddings are based on literature-derived downstream ex- pression signatures in contrast to embeddings obtained with existing approaches that leverage either co-expression, or protein binding networks.
Using the QIAGEN Knowledge Base (QKB), a structured collection of biomedical content. Function embeddings are constructed using gene embedding vectors with a linear model trained on signed gene-function relationships.

□ NS-Forest 2.0: A machine learning method for the discovery of minimum marker gene combinations for cell type identification from single-cell RNA sequencing
>> https://genome.cshlp.org/content/31/10/1767.full
Necessary and Sufficient Forest (NS-Forest) version 2.0 leverages the nonlinear attributes of random forest feature selection and a binary expression scoring approach to discover the minimal marker gene expression combinations that optimally capture the cell type identity.
In NS-Forest v2.0, all permutations of the selected top-ranked genes are tested and their performance assessed using the weighted F-beta score. The F-beta score contains a weighting term, beta, that allows for emphasizing either precision or recall.
By weighting for precision (the contributions of false positives) versus recall (the contributions of false negatives), limit the impact of zero inflation (or drop-out), a known technical artifact with scRNA-seq data, on marker gene assessment.

□ BioVAE: a pre-trained latent variable language model for biomedical text mining
>> https://academic.oup.com/bioinformatics/advance-article/doi/10.1093/bioinformatics/btab702/6390793
OPTIMUS has successfully combined BERT-based PLMs and GPT-2 with variational autoencoders (VAEs), achieving SOTA in both representation learning and language generation tasks. However, they are trained only on general domain text, and biomedical models are still missing.
BioVAE, the first large scale pre-trained latent variable language model for the biomedical domain, which uses the OPTIMUS framework to train on large volumes of biomedical text. BioVAE can generate more accurate biomedical sentences than the original OPTIMUS output.
□ pLMMGMM: A penalized linear mixed model with generalized method of moments for complex phenotype prediction
>> https://www.biorxiv.org/content/10.1101/2021.10.11.463997v1.full.pdf
pLM- MGMM is built within the linear mixed model framework, where random effects are used to model the joint predictive effects from all genetic variants within a region.
pLMMGMM can jointly consider a large number of genetic regions and efficiently select those harboring variants with both linear and non-linear predictive effects.
□ NAToRA, a relatedness-pruning method to minimize the loss of dataset size in genetic and omics analyses
>> https://www.biorxiv.org/content/10.1101/2021.10.21.465343v1.full.pdf
NAToRA is an algorithm that minimizes the number of individuals to be removed from a dataset. In the context of complex network theory, NAToRA finds the maximum clique in the complement networks.
NAToRA is also compatible with relatedness metrics calculated by the REAP method, which is more appropriate for admixed populations than PLINK and KING.
※コメント投稿者のブログIDはブログ作成者のみに通知されます