

□ TrEMOLO: Accurate transposable element allele frequency estimation using long-read sequencing data combining assembly and mapping-based approaches
>> https://www.biorxiv.org/content/10.1101/2022.07.21.500944v1.full.pdf
Transposable Element MOvement detection using LOng-reads (TrEMOLO) combines the advantages offered by LR sequencing (i.e., highly contiguous assembly and unambiguous mapping) to identify TE insertion (and deletion) variations, for TE detection and frequency estimation.
TrEMOLO accuracy in TE identification and the TSD detection system allow predicting the insertion site within a 2-base pair window. Assemblers provide the most frequent haplotype at each locus, and thus an assembly represent just the "consensus" of all haplotypes at each locus.
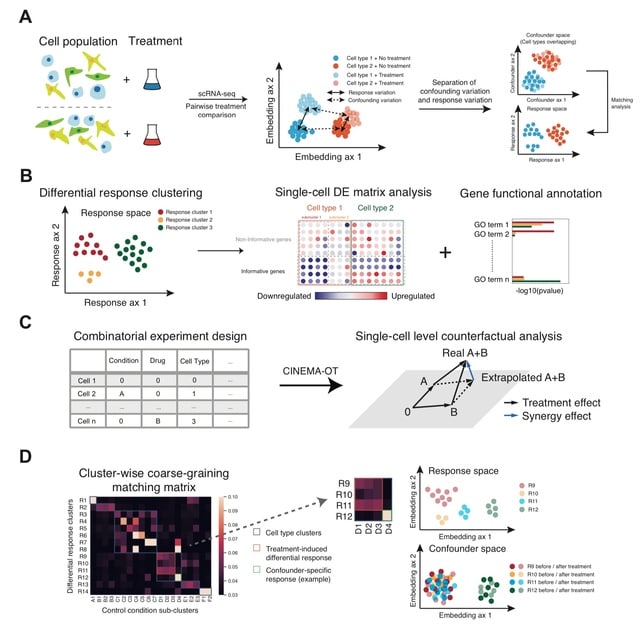
□ Causal identification of single-cell experimental perturbation effects with CINEMA-OT
>> https://www.biorxiv.org/content/10.1101/2022.07.31.502173v1.full.pdf
CINEMA-OT (Causal INdependent Effect Module Attribution + Optimal Transport) separates confounding sources of variation from perturbation effects to obtain an optimal transport matching that reflects counterfactual cell pairs.
The algorithm is based on a causal inference framework for modeling confounding signals and conditional perturbation. CINEMA-OT can attribute divergent treatment effects to either explicit confounders, or latent confounders by cluster-wise coarse-graining of the matching matrix.

□ AIFS: A novel perspective, Artificial Intelligence infused wrapper based Feature Selection Algorithm on High Dimensional data analysis
>> https://www.biorxiv.org/content/10.1101/2022.07.21.501053v1.full.pdf
AIFS creates a Performance Prediction Model (PPM) using artificial intelligence (AI) which predicts the performance of any feature set and allows wrapper based methods to predict and evaluate the feature subset model performance without building actual model.
AIFS can identify both marginal features and interaction terms without using interaction terms in PPM, which could be critical in reducing the feature space an algorithm has to process.

□ MVCPM: Multiview clustering of multi-omics data integration by using a penalty model
>> https://bmcbioinformatics.biomedcentral.com/articles/10.1186/s12859-022-04826-4
MVCPM has the highest silhouette score for common clusters and the average silhouette score. MVCPM provides more detailed information within each data type, is better for integrating different types of omics data and simultaneously has consistent and differential cluster patterns.
MVCPM can be considered the best approach for integration and clustering. MVCPM uses k-NN to assign patients that are originally clustered into different clusters into one cluster and compute silhouette scores. MVCPM determines the significance of difference in survival times.

□ Hybrid Rank Aggregation (HRA): A novel rank aggregation method for ensemble-based feature selection
>> https://www.biorxiv.org/content/10.1101/2022.07.21.501057v1.full.pdf
the ensemble-based feature selection (EFS) approach relies on using a single RA algorithm to pool feature performance and select features. However, a single RA algorithm may not always give optimal performance across all datasets.
A novel hybrid rank aggregation (HRA) method allows creation of a RA matrix which contains feature performance or importance in each RA technique followed by an unsupervised learning-based selection of features based on their performance/importance in RA matrix.

□ Benchmarking long-read RNA-sequencing analysis tools using in silico mixtures
>> https://www.biorxiv.org/content/10.1101/2022.07.22.501076v1.full.pdf
ONT long reads from pure RNA samples were used for isoform detection using bambu, FLAIR, FLAMES, SQANTI3, StringTie2 and TALON. Both pure RNA samples and in silico mixture samples were mapped against the GENCODE human annotation and sequins annotation.
This silico mixture strategy provides extra levels of ground-truth without extra cost. The transcript-level count matrix was used as input to downstream steps such as DTE (fDESeq2, EBSeq, edgeR, limma, NOISeq) and DTU (DEXSeq, DRIMSeq, edgeR, limma and satuRn).

□ ccImpute: an accurate and scalable consensus clustering based algorithm to impute dropout events in the single-cell RNA-seq data
>> https://bmcbioinformatics.biomedcentral.com/articles/10.1186/s12859-022-04814-8
ccImpute has a polynomial runtime that compares favorably to imputation algorithms with polynomial (DrImpute, DCA, DeepImpute) and exponential runtime (scImpute).
ccImpute relies on a consensus matrix to approximate how likely a given pair of cells is to be clustered together and considered to be of the same type. Applying mini-batch K-means and the possibility of using a more efficient centroid selection scheme than random restarts.

□ CMIC: an efficient quality score compressor with random access functionality
>> https://bmcbioinformatics.biomedcentral.com/articles/10.1186/s12859-022-04837-1
CMIC (classification, mapping, indexing and compression), an adaptive and random access supported compressor for lossless compression. In terms of random access speed, the CMIC is faster than the LCQS.
The algorithm realizes the parallelization of the compression process by using SIMD. CMIC makes full use of the correlation between adjacent quality scores and improves the efficiency of context modeling entropy encoding.

□ orsum: a Python package for filtering and comparing enrichment analyses using a simple principle
>> https://bmcbioinformatics.biomedcentral.com/articles/10.1186/s12859-022-04828-2
Filtering in orsum is based on a simple principle: a term is discarded if there is a more significant term that annotates at least the same genes; the remaining more significant term becomes the representative term for the discarded term.
The inputs for orsum are enrichment analysis results containing term IDs ordered by statistical significance and Gene Matrix Transposed (GMT) file. This makes it possible to use the same annotations as the ones used in the enrichment analysis.

□ dRFEtools: Dynamic recursive feature elimination for omics
>> https://www.biorxiv.org/content/10.1101/2022.07.27.501227v1.full.pdf
Dynamic recursive feature elimination (RFE) decreases computational time compared to the current RFE function available with scikit-learn, while maintaining high accuracy in simulated data for both classification and regression models.
Dynamic RFE analysis is based on the random forest algorithm with Out-of-Bag scoring and 100 n estimators similar to simulation data. StratifiedKFold is used to generate cross-validation folds for all scenarios to maintain even distribution of patient diagnosis across folds.

□ McAN: an ultrafast haplotype network construction algorithm
>> https://www.biorxiv.org/content/10.1101/2022.07.23.501111v1.full.pdf
McAN, a minimum-cost arborescence based haplotype network construction algorithm, by considering mutation spectrum history (mutations in ancestry haplotype should be contained in descendant haplotype), node size and sampling time.
McAN calculates distances b/n adjacent haplotypes instead of any two haplotypes. All haplotypes are sorted by mutation count and sequence count in descending order and the earliest sampling time in ascending order. The closest ancestor is determined and minimized for each haplotype.

□ SparkGC: Spark based genome compression for large collections of genomes
>> https://bmcbioinformatics.biomedcentral.com/articles/10.1186/s12859-022-04825-5
SparkGC uses Spark’s in-memory computation capabilities to reduce compression time by keeping data active in memory between the first-order and second-order compression.
SparkGC is a lossless genome compression method, the auxiliary data of the to-be-compressed sequence cannot be lost.
The compression algorithm is deployed on the master node, but the scheduling mechanism of Spark is migrating the computing tasks to nodes closest to the data, so the compression tasks will be scheduled to worker nodes.

□ ColocQuiaL: A QTL-GWAS colocalization pipeline
>> https://academic.oup.com/bioinformatics/advance-article/doi/10.1093/bioinformatics/btac512/6650620
ColocQuiaL automates the execution of COLOC to perform colocalization analyses between GWAS signals for any trait of interest and single-tissue eQTL and sQTL signals.
The input loci to ColocQuiaL can be a single GWAS locus, a list of GWAS loci of interest, or just the summary statistics across the entire genome.

□ Canary: an automated tool for the conversion of MaCH imputed dosage files to PLINK files
>> https://bmcbioinformatics.biomedcentral.com/articles/10.1186/s12859-022-04822-8
Canary uses singularity container technology to allow users to automatically convert these MaCH files into PLINK compatible files. Canary is a singularity container which comes w/ many preinstalled software, incl. dose2plink.c, which allows users to use directly on any system.
The convert-mac module of Canary deals with a single sub-study at a time. Canary combines the consent groups by combining each of chromosome dose files i.e., consent group 1 chromosome 1 with consent group 2 with chromosome 1.

□ Haisu: Hierarchically supervised nonlinear dimensionality reduction
>> https://journals.plos.org/ploscompbiol/article?id=10.1371/journal.pcbi.1010351
Haisu is a generalizable extension to nonlinear dimensionality reduction for visualization that incorporates an input hierarchy to influence a resulting embedding.
Haisu mirrors the limitations of the integrated NLDR approach spatially and temporally. Haisu formulates a direct relationship between the distance of two graph nodes in the hierarchy and the resulting pairwise distance in high-dimensional space.

□ CGAN-Cmap: protein contact map prediction using deep generative adversarial neural networks
>> https://www.biorxiv.org/content/10.1101/2022.07.26.501607v1.full.pdf
CGAN-Cmap is constructed via integration of a modified squeeze excitation residual neural network (SE-ResNet), SE-Concat, and a conditional GAN.
CGAN-Cmap uses a dynamic weighted binary cross-entropy (BCE) loss function, which assigns a dynamic weight for classes based on the ratio of the uncontacted class to the contacted class in each iteration.

□ JBrowse 2: A modular genome browser with views of synteny and structural variation
>> https://www.biorxiv.org/content/10.1101/2022.07.28.501447v1.full.pdf
JBrowse 2 retains the core features of the open-source JavaScript genome browser JBrowse while adding new views for synteny, dotplots, breakpoints, gene fusions, and whole-genome overviews.
JBrowse 2 features several specialized synteny views, incl. the Dotplot View and the Linear Synteny View. These views can display data from Synteny Tracks, which themselves can load data from formats including MUMmer, minimap2, MashMap, UCSC chain files, and MCScan.

□ HyMSMK: Integrate multiscale module kernel for disease-gene discovery in biological networks
>> https://www.biorxiv.org/content/10.1101/2022.07.28.501869v1.full.pdf
HyMSMK, a type of novel hybrid methods for disease-gene discovery by integrating multiscale module kernel (MSMK) derived from multiscale module profile (MSMP).
HyMSMK extracts MSMP with local to global structural information by multiscale modularity optimization with exponential sampling, and construct MSMK by using the MSMP as a feature matrix, combining with the relative information content of features and kernel sparsification.

□ Graphia: A platform for the graph-based visualisation and analysis of high dimensional data
>> https://journals.plos.org/ploscompbiol/article?id=10.1371/journal.pcbi.1010310
Graph layout is an iterative process. Many programs only display the results of a layout algorithm after it has run a defined number of iterations. With Graphia, the layout is shown live, such that graphs ‘unfold’ in real time.
Core to Graphia’s functionality is support for the calculation of correlation matrices from any tabular matrix of continuous or discrete values, whereupon the software is designed to rapidly visualise the often very large graphs that result in 2D or 3D space.

□ Cookie: Selecting Representative Samples From Complex Biological Datasets Using K-Medoids Clustering
>> https://www.frontiersin.org/articles/10.3389/fgene.2022.954024/full
Cookie can efficiently select out the most representative samples from a massive single-cell population with diverse properties. This method quantifies the relationships/similarities among samples using their Manhattan distances by vectorizing all given properties.
Cookie determines an appropriate sample size by evaluating the coverage of key properties from multiple candidate sizes, following by a k-medoids clustering to group samples into several clusters and selects centers from each cluster as the most representatives.

□ FLAIR-fusion: Detection of alternative isoforms of gene fusions from long-read RNA-seq
>> https://www.biorxiv.org/content/10.1101/2022.08.01.502364v1.full.pdf
FLAIR-fusion can detect simulated fusions and their isoforms with high precision and recall even with error-prone reads. This tool is able to do splice site correction of all reads, gather chimeric reads, and then apply a number of specific filters to identify true fusion reads.
FLAIR-fusion identifies the isoforms at each locus involved in a fusion, then combines those to identify full-length fusion isoforms matched across the fusion breakpoint.

□ sc-SHC: Significance Analysis for Clustering with Single-Cell RNA-Sequencing Data
>> https://www.biorxiv.org/content/10.1101/2022.08.01.502383v1.full.pdf
Over-clustering can be particularly insidious because clustering algorithms will partition data even in cases where there is only uninteresting random variation present.
Extending a method for Gaussian data, Significance of Hierarchical Clustering (SHC), to propose a model-based hypothesis testing that incorporates significance analysis into the clustering algorithm and permits statistical evaluation of clusters as distinct cell populations.

□ SPA: Optimal Sparsity Selection Based on an Information Criterion for Accurate Gene Regulatory Network Inference
>> https://www.frontiersin.org/articles/10.3389/fgene.2022.855770/full
SPA, a sparsity selection algorithm that is inspired by the AIC and BIC in terms of introducing a penalty term to the goodness of fit, but is developed particularly for GRN inference to identify the most mathematically optimal and accurate GRN.
SPA takes a set of inferred GRNs with varying sparsities, the measured gene expression in fold changes, and the perturbation design as input. It then uses the GRN Information Criterion (GRNIC) and identifies the GRN that minimizes GRNIC as the best GRN.

□ EI: Integrating multimodal data through interpretable heterogeneous ensembles
>> https://www.biorxiv.org/content/10.1101/2020.05.29.123497v3.full.pdf
Existing data integration approaches do not sufficiently address the heterogeneous semantics of multimodal data. Early approaches that rely on a uniform integrated representation reinforce the consensus among the modalities, but may lose exclusive local information.
Ensemble Integration (EI) infers local predictive models from the individual data modalities using appropriate algorithms, and uses effective heterogeneous ensemble algorithms to integrate these local models into a global predictive model.

□ BASS: multi-scale and multi-sample analysis enables accurate cell type clustering and spatial domain detection in spatial transcriptomic studies
>> https://genomebiology.biomedcentral.com/articles/10.1186/s13059-022-02734-7
BASS (Bayesian Analytics for Spatial Segmentation) performs multi-scale transcriptomic analyses in the form of joint cell type clustering and spatial domain detection, with the two analytic tasks carried out simultaneously within a Bayesian hierarchical modeling framework.
BASS is capable of multi-sample analysis that jointly models multiple tissue sections/samples, facilitating the integration of spatial transcriptomic data across tissue samples.

□ Cogito: automated and generic comparison of annotated genomic intervals
>> https://bmcbioinformatics.biomedcentral.com/articles/10.1186/s12859-022-04853-1
Cogito “COmpare annotated Genomic Intervals TOol” provides a workflow for an unbiased, structured overview and systematic analysis of complex genomic datasets consisting of different data types (e.g. RNA-seq, ChIP-seq) and conditions.
Cogito is able to visualize valuable key information of genomic or epigenomic interval-based data. Within Cogito gene expression in reads per kilo base per million mapped reads (RPKM) from RNA-seq and Homer ChIP-seq peak scores were interpreted as rational values.
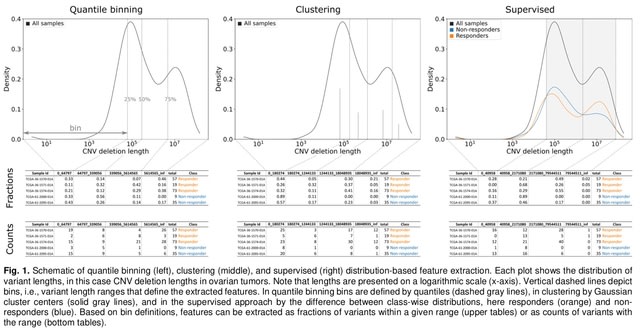
□ DBFE: Distribution-based feature extraction from structural variants in whole-genome data
>> https://academic.oup.com/bioinformatics/advance-article-abstract/doi/10.1093/bioinformatics/btac513/6656344
The core contributions of DBFE include: (1) strategies for determining features using variant length binning, clustering, and density estimation; (2) a programming library for automating distribution-based feature extraction in machine learning pipelines.
DBFE uses an approach based on Kernel Density Estimation. DBFE can be applied to other variant types (e.g., small insertions/deletions). One would possibly need to limit the range of lengths taken into account and analyze distributions on a linear rather than a logarithmic scale.

□ ChromTransfer: Transfer learning reveals sequence determinants of regulatory element accessibility
>> https://www.biorxiv.org/content/10.1101/2022.08.05.502903v1.full.pdf
The ENCODE rDHSs were assembled using consensus calling from 93 million DHSs called across a wide range of human cell lines, cell types, cellular states, and tissues, and are therefore likely capturing the great majority of possible sequences associated with human open chromatin.
ChromTransfer, a transfer learning scheme for single-task modeling of the DNA sequence determinants of regulatory element activities. ChromTransfer uses a cell-type agnostic model of open chromatin regions across human cell types to fine-tune models for specific tasks.
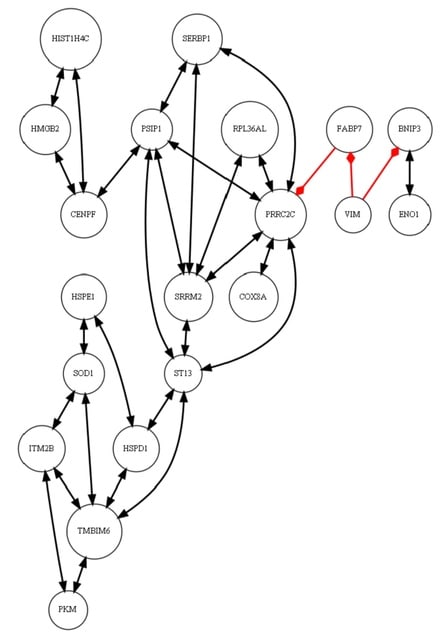
□ Detecting boolean asymmetric relationships with a loop counting technique and its implications for analyzing heterogeneity within gene expression datasets
>> https://www.biorxiv.org/content/10.1101/2022.08.04.502792v1.full.pdf
A very general method that can be used to detect biclusters within gene-expression data that involve subsets of genes which are enriched for these ‘boolean-asymmetric’ relationships (BARs).
This strategy can make use of any method which finds BSR-biclusters, but for demonstration we make use of the LCLR method for finding BSR-biclusters. combine the column-splitting technique with the LCLR algorithm to form what we call the Loop Counting Asymmetric algorithm.

□ matchRanges: Generating null hypothesis genomic ranges via covariate-matched sampling
>> https://www.biorxiv.org/content/10.1101/2022.08.05.502985v1.full.pdf
matchRanges, a propensity score-based covariate matching method for the efficient generation of matched null ranges from a set of background ranges. matchRanges function takes as input a “focal” set of data to be matched and a “pool” set of background ranges to select from.
matchRanges performs subset selection based on the provided covariates and returns a null set of ranges with distributions of covariates. This allows for an unbiased comparison between features of interest in the focal and matched sets without confounding by matched covariates.

□ RNA-Bloom2: Reference-free assembly of long-read transcriptome sequencing data
>> https://www.biorxiv.org/content/10.1101/2022.08.07.503110v1.full.pdf
RNA-Bloom2 extends support for reference-free transcriptome assembly of bulk RNA long sequencing reads. RNA-Bloom2 offers both memory- and time-efficient assembly by utilizing digital normalization of long reads with strobemers.
RNA-Bloom2 assemblies have higher BUSCO completeness than input reads and a RATTLE assembly. A portion of our assembled transcripts have split alignments across genome scaffolds, but the majority of them are supported by paired-end short reads.
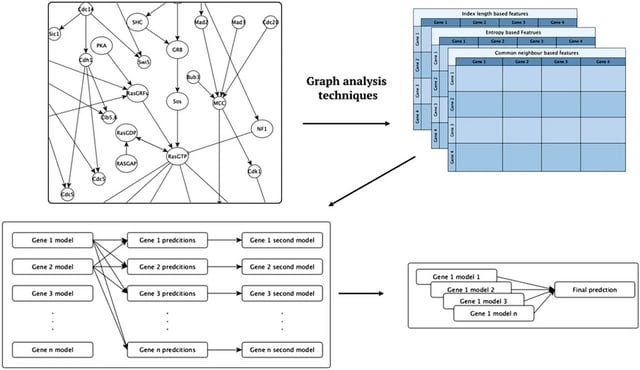
□ Improved prediction of gene expression through integrating cell signalling models with machine learning
>> https://bmcbioinformatics.biomedcentral.com/articles/10.1186/s12859-022-04787-8
An approach to integration is to augment ML with similarity features computed from cell signalling models. Each set of features was in turn used to learn multi-target regression models. All the features have significantly improved accuracy over the baseline model.
The baseline model is a random forest model trained as Multi-target regressor stacking (MTRS) without the extra features generated from graph processing. This implementation directly combines the predictions without using an extra meta model.

□ Completing Single-Cell DNA Methylome Profiles via Transfer Learning Together With KL-Divergence
>> https://www.frontiersin.org/articles/10.3389/fgene.2022.910439/full
Using transfer learning together with Kullback-Leibler (KL) divergence to train DNNs for completing DNA methylome profiles with extremely low coverage rate by leveraging those with higher coverage.
Employing a hybrid network architecture adapted from DeepGpG, a mixture of convolutional neural network and recurrent neural network. The CNN learns predictive DNA sequence patterns and the RNN exploits known methylation state of neighboring CpGs in the target profile.

□ PWCoCo: Pair-wise Conditional and Colocalisation: An efficient and robust tool for colocalisation
>> https://www.biorxiv.org/content/10.1101/2022.08.08.503158v1.full.pdf
PWCoCo performs conditional analyses to identify independent signals for the two tested traits in a genomic region and then conducts colocalisation of each pair of conditionally independent signals for the two traits using summary-level data.
This allows for the stringent single-variant assumption to hold for each pair of colocalisation analysis. the computational efficiency of PWCoCo is better than colocalisation with Sum of Single Effects Regression using Summary Stats, with greater gains in efficiency for analysis.
※コメント投稿者のブログIDはブログ作成者のみに通知されます